
Future-Proofing through
Open Source and
Data Management Policy
Terrell Russell, Ph.D.
@terrellrussell
Executive Director, iRODS Consortium
April 15-17, 2024
BioIT World 2024
Boston, MA




Our Membership



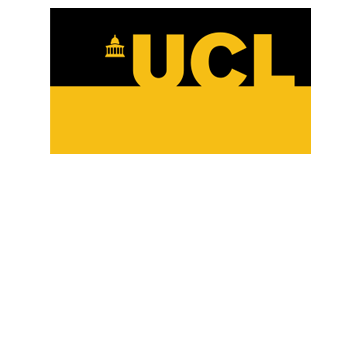













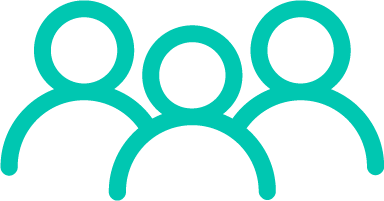
Consortium
Member
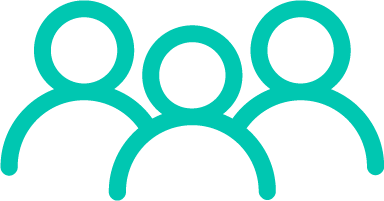
Consortium
Member
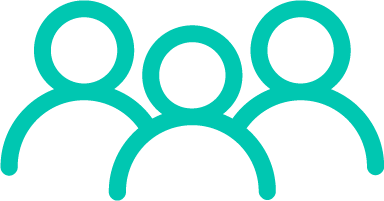
Consortium
Member





Our Business Model
Start with Proof of Concept
- Use Case Driven
- Hands on
- Service and Support Contract
- Master Services Agreement
Consortium Membership
- Four Levels - $11k to $165k, annually
- 10 to 300 hours of support
- Participation in Software roadmap
- Discounted hourly rate
Tier 3 Support
- Systems Integrators
- Compute Vendors
- Storage Vendors

Long-Term Thinking
The data management platforms being sold into the bio and pharmaceutical industries are expensive and incentivized to vertically integrate and capture the customer.
Long-term Data Management is best executed when policies are clear and infrastructure is abstracted and swappable.
iRODS provides an open-source example of how this approach can be implemented to sustain FAIR data practices, consistency, and cost-savings across your enterprise.

Today's Ecosystem
Types of Products
- sequencing
- imaging
- file conversion / transformation
- metadata extraction
- annotation
- lab notebooks
- inventory
- data movers
- storage
- visualization
- analytics

Today's Ecosystem
Types of Products
- sequencing
- imaging
- file conversion / transformation
- metadata extraction
- annotation
- lab notebooks
- inventory
- data movers
- storage
- visualization
- analytics

Data Lifecycle
- ingestion
- storage
- preparation
- analysis
- publication
- archiving
Ingest to Institutional Repository
As data matures and reaches a broader community, data management policy must also evolve to meet these additional requirements.


Pros and Cons

- Good, dynamic solutions are cobbled together from many of these pieces
- interoperable
- distinct
- proven
- Proprietary solutions generally live together in a black box with a support contract
- change faster, more likely to be cutting edge
- driven by market forces
- could lead to 'lock-in' or a 'rug pull'
- Open solutions generally require more knowledge and expertise and ownership
- backward and forward compatible
- can be extended / customized
- slower to advance
Not just a technical problem

- Solutions do not exist in a vacuum
- Have to satisfy
- a web of regulation and compliance
- national and international law
- internal policy

Data Management Mandates
National Institutes of Health (NIH)
- has issued the Data Management and Sharing (DMS) policy (effective January 25, 2023)
- requires management and sharing of scientific data generated from NIH-funded or conducted research
- https://grants.nih.gov/grants/guide/notice-files/NOT-OD-21-013.html
White House Office of Science and Technology Policy (OSTP)
- an update to the 2013 memorandum
- calls for free, immediate, and equitable access to all federally funded research (effective December 31, 2025)
- https://www.whitehouse.gov/wp-content/uploads/2022/08/08-2022-OSTP-Public-Access-Memo.pdf

Data Management
In the end, to be valuable, your data (and your process) need to be
- FAIR - Findable, Accessible, Interoperable, Reusable
- FAIR-TLC - Traceable, Licensed, and Connected
What is iRODS
Open Source
- C++ client-server architecture
- BSD-3 Licensed
Distributed
- Runs on a laptop, a cluster, on premises or geographically distributed
Data Centric & Metadata Driven
- Insulate both your users and your data from your infrastructure

iRODS as the Integration Layer


Why use iRODS?

People need a solution for:
- Managing large amounts of data across various storage technologies
- Controlling access to data
- Searching their data quickly and efficiently
- Automation
The larger the organization, the more they need software like iRODS.
Working Groups

Imaging Working Group
- Goal: To provide a standardized suite of imaging policies and practices for integration with existing tools and pipelines
- Open Microscopy Environment (and OMERO)
- Neuroscience Microscopy Core at UNC School of Medicine
- New York University
- Santa Clara University
- UC San Diego
- UC Santa Cruz
- UMass
- Harvard
- Maastricht University
- Wellcome Sanger Institute
- CyVerse
- NIEHS
- Netherlands Cancer Institute (NKI)
- Francis Crick Institute
- Fritz Lipmann Institute
- Osnabrück University
- RIKEN

AWS Lambda for S3
Open Source:

- iRODS Client
- Developed in collaboration with BMS


Automated Ingest Capability

- iRODS Capability
- Developed in collaboration with Roche


Indexing Capability
Open Source:

- iRODS Capability
- Developed in collaboration with NIEHS and BMS



NFSRODS
Open Source:

- iRODS Client, NFSv4.1 Server
- Developed in collaboration with CU Boulder and BMS



Storage Tiering Capability

- iRODS Capability
- Developed in collaboration with Roche


Policy Composition

Consider Storage Tiering:
- Violating Object Identification
- Data Movement
- Data Replication
- Data Verification
- Data Retention

- Packaged and supported solutions
- Require configuration not code
- Derived from the majority of use cases observed in the user community








iRODS Capabilities


Big Picture

iRODS is a flexible platform for building long-term solutions
- Discovery - user-defined metadata catalog
- Auditing - bookkeeping across disparate systems
- Policy - full, programmatic environment
Big Picture

Proper data management requires policy enforcement.
These policies will change over time.
Open source is the best practice for a 100-year view.
Questions?
Thank you.


May 28-31, 2024
Automated Ingest - Landing Zone



Automated Ingest - Filesystem Scanning


Storage Tiering



Core Competencies
Policy
Capabilities
Indexing



Core Competencies
Policy
Capabilities
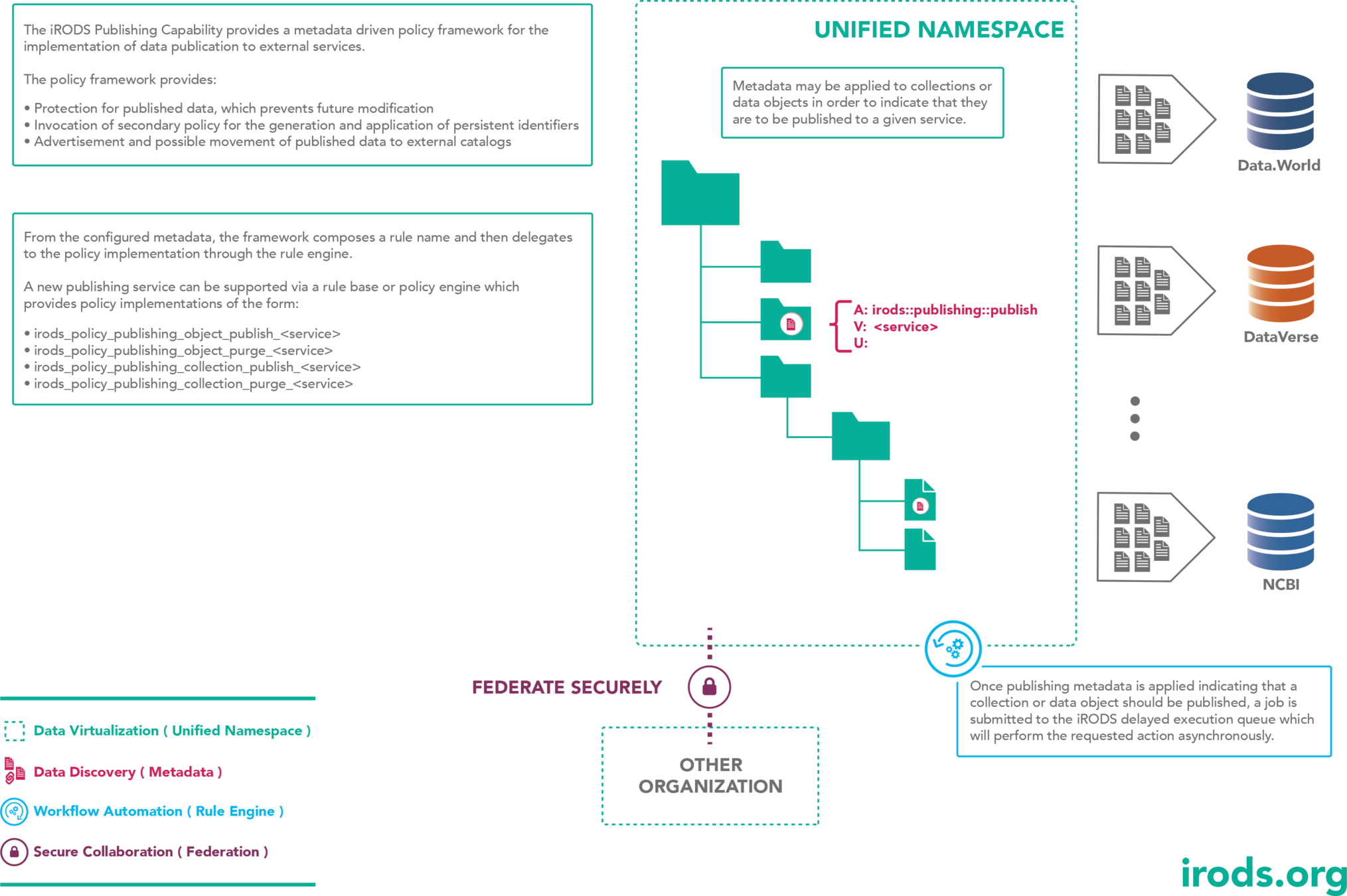
Publishing


Deployment Patterns


Data to Compute
Compute to Data

Data Transfer Nodes

Filesystem Synchronization


Filesystem Synchronization



Data to Compute



Compute to Data



Data Transfer Nodes



The Data Management Model



iRODS S3 Functionality

The iRODS S3 storage resource plugin allows iRODS to use any S3-compatible storage device or service to hold iRODS Data Objects, on-premises or in the cloud.
This plugin can work as a standalone "cacheless" resource or as an archive resource under the iRODS compound resource. Either configuration provides a POSIX interface to data held on an object storage device or service.
The following S3 services and appliances (in no particular order) have been tested:
- Amazon (AWS) S3
- Fujifilm Object Archive
- MinIO S3
- Ceph S3
- Spectra Logic Vail
- Spectra Logic BlackPearl
- Google Cloud Storage (GCS)
- Wasabi S3
- Oracle OCI
- Quantum ActiveScale
- Garage S3

Protocol Plumbing - Presenting iRODS as other Protocols
- WebDAV
- FUSE
- HTTP
- NFS
- SFTP
- K8s CSI
- S3
Over the last few years, the ecosystem around the iRODS server has continued to expand.
Integration with other types of systems is a valuable way to increase accessibility without teaching existing tools about the iRODS protocol or introducing new tools to users.
With some plumbing, existing tools get the benefit of visibility into an iRODS deployment.
The iRODS Data Management Model

Core Competencies
Policy
Capabilities
Patterns

iRODS Core Competencies
The underlying technology categorized into four areas






Data Virtualization

Combine various distributed storage technologies into a Unified Namespace
- Existing file systems
- Cloud storage
- On premises object storage
- Archival storage systems
iRODS provides a logical view into the complex physical representation of your data, distributed geographically, and at scale.


Projection of the Physical into the Logical

Logical Path
Physical Path(s)

Data Discovery
Attach metadata to any first class entity within the iRODS Zone
- Data Objects
- Collections
- Users
- Storage Resources
- The Namespace
iRODS supports automated and user-provided metadata which makes your data and infrastructure more discoverable, operational, and valuable.



Metadata Everywhere


Workflow Automation
Policy Enforcement Points (PEPs) are triggered by every operation within the framework
- Authentication
- Storage Access
- Database Interaction
- Network Activity
- Extensible RPC API

The iRODS rule engine framework provides the ability to capture real world policy as computer actionable rules which may allow, deny, or add context to operations within the system.


Dynamic Policy Enforcement

The iRODS rule may:
- restrict access
- log for audit and reporting
- provide additional context
- send a notification

Dynamic Policy Enforcement

A single API call expands to many plugin operations all of which may invoke policy enforcement
Plugin Interfaces:
- Authentication
- Database
- Storage
- Network
- Rule Engine
- Microservice
- RPC API

Secure Collaboration
iRODS allows for collaboration across administrative boundaries after deployment
- No need for common infrastructure
- No need for shared funding
- Affords temporary collaborations
iRODS provides the ability to federate namespaces across organizations without pre-coordinated funding or effort.



iRODS as a Service Interface


Federation - Shared Data and Services


What is a Policy
A Definition of Policy
A set of ideas or a plan of what to do in particular situations that has been agreed to officially by a group of people...
So how does iRODS do this?


iRODS Policies

The reflection of real world data management decisions in computer actionable code.
(a plan of what to do in particular situations)

Possible Policies - The What

- Data Movement
- Data Verification
- Data Retention
- Data Replication
- Data Placement
- Checksum Validation
- Metadata Extraction
- Metadata Application
- Metadata Conformance
- Replica Verification
- Vault to Catalog Verification
- Catalog to Vault Verification
- ...

iRODS Scenario - S3 Eject

To "eject" a part of a user's data from an iRODS Zone...
- User adds metadata to a Collection, designating it as ready to be 'ejected' to a particular S3 resource
- The system recursively replicates all data objects under that Collection to the S3 resource
- The system writes all associated metadata into a manifest file on the S3 resource
- The system (optionally) recursively unregisters all data objects under that Collection from the S3 resource (and possibly all other resources)
Then, when ready...
- A separate script can put or ingest the files into another iRODS Zone and associate all the metadata stored in the manifest file
Questions?

Use Cases
iRODS

The Wellcome Sanger Institute


Sanger - Replication

- Data preferentially placed on resource servers in the green data center (fallback to red)
- Data replicated to the other room.
- Checksums applied
- Green and red centers both used for read access.

Sanger - Metadata
attribute: library
attribute: total_reads
attribute: type
attribute: lane
attribute: is_paired_read
attribute: study_accession_number
attribute: library_id
attribute: sample_accession_number
attribute: sample_public_name
attribute: manual_qc
attribute: tag
attribute: sample_common_name
attribute: md5
attribute: tag_index
attribute: study_title
attribute: study_id
attribute: reference
attribute: sample
attribute: target
attribute: sample_id
attribute: id_run
attribute: study
attribute: alignment
- Example metadata attributes
- Users query and access data from local compute clusters
- Users access iRODS locally via the command line interface

Sanger - Federation


Maastricht DataHub

Maastricht DataHub

SURF Scale Out Pilot



University Zone
Catalog Provider



University Zone


Server Hosting Environment
Catalog Consumer

Tape Archive
Disk Storage
Object Storage

Catalog Consumer
Catalog Provider
SURF EUDAT CDI
External Community Zones
Zone
Local Storage
CXFS

Tape Library


EUDAT University Zone


EUDAT University Zone
B2SAFE iRODS Federation
EUDAT Centers
iRODS Federation
ARCHIVE
GridFTP Data Movement


Catalog Provider

Catalog Provider

Catalog Provider

Catalog Provider
Questions?

Overview
iRODS Proof of Concept

Initial Goals
- Automatically Ingest data from a 'Landing Zone'
- Extract salient metadata - e.g. EXIF tags
- Tag Data Objects and Collections
- Makes them Actionable and Discoverable
- Discover and interact with data on the command line
- Discover and interact with data via Metalnx
- Share data via Metalnx
- Interact with data via NFS and WebDAV

Automated Ingest
Any data that is discovered during a scan
- Automatically registered to a storage resource
- Metadata extracted and applied to the object in the catalog
- Event possibly generated for audit trail
Users can view and access data and metadata from any client

Data Discovery with Metalnx
Automated Ingest has provided metadata for data discovery
The metadata can be directly inspected in Metalnx
The query builder can be used to identify data sets of interest via Attribute, Value, Unit matches
Queries to the system metadata may also be performed, searching on values such as file name, collection path, user, etc.

File System Presentations: Davrods
Davrods provides both a simple web based interface (via WebDAV) as well as the ability to mount a folder on the desktop
Davrods is an Apache Module implemented in C using the native iRODS POSIX API
Davrods can be used to edit data in-place, or to copy data to/from a user's collections

File System Presentations: NFSRODS
NFSRODS leverages the Java iRODS Client Library 'Jargon' and is implemented with NFS4J
NFSRODS acts as a Mid-Tier client to iRODS
NFSRODS projects iRODS ACLs into NFSv4 extended ACLs
NFSRODS can also be used to edit data in-place, or to copy data to/from a user's collections

Data Discovery with Command Line
Query using imeta, a command-line iRODS client utility:
imeta qu -d "Image Make" = Apple
iquest "%s/%s" "SELECT COLL_NAME, DATA_NAME WHERE META_DATA_ATTR_NAME = 'Image Make' AND META_DATA_ATTR_VALUE = 'Apple'"
Query using iquest, a command-line iRODS client utility:

Questions?

BioIT World 2024 - iRODS: Future-Proofing through Open Source and Data Management Policy
By iRODS Consortium
BioIT World 2024 - iRODS: Future-Proofing through Open Source and Data Management Policy
- 32