Precision Medicine: Where Small-Sample Clinical Trials Meet Big Health Data
Overview
Motivating case: NSCLC
Precision therapy challenges
Incorporating larger data sets for drug/population discovery
FOCUS ON TARGETED CANCER THERAPY
Subject Profile - 46 Yr Old Male NSCLC
- First diagnosed in November 2013
- 30 pack-year smoking history
- Required supplemental O2
- Significant pain and dyspnea due to widely metastatic disease
- Staging head CT also revealed numerous (15 to 20) asymptomatic brain metastases measuring up to 1.7 cm that had not been previously treated
- In hospice
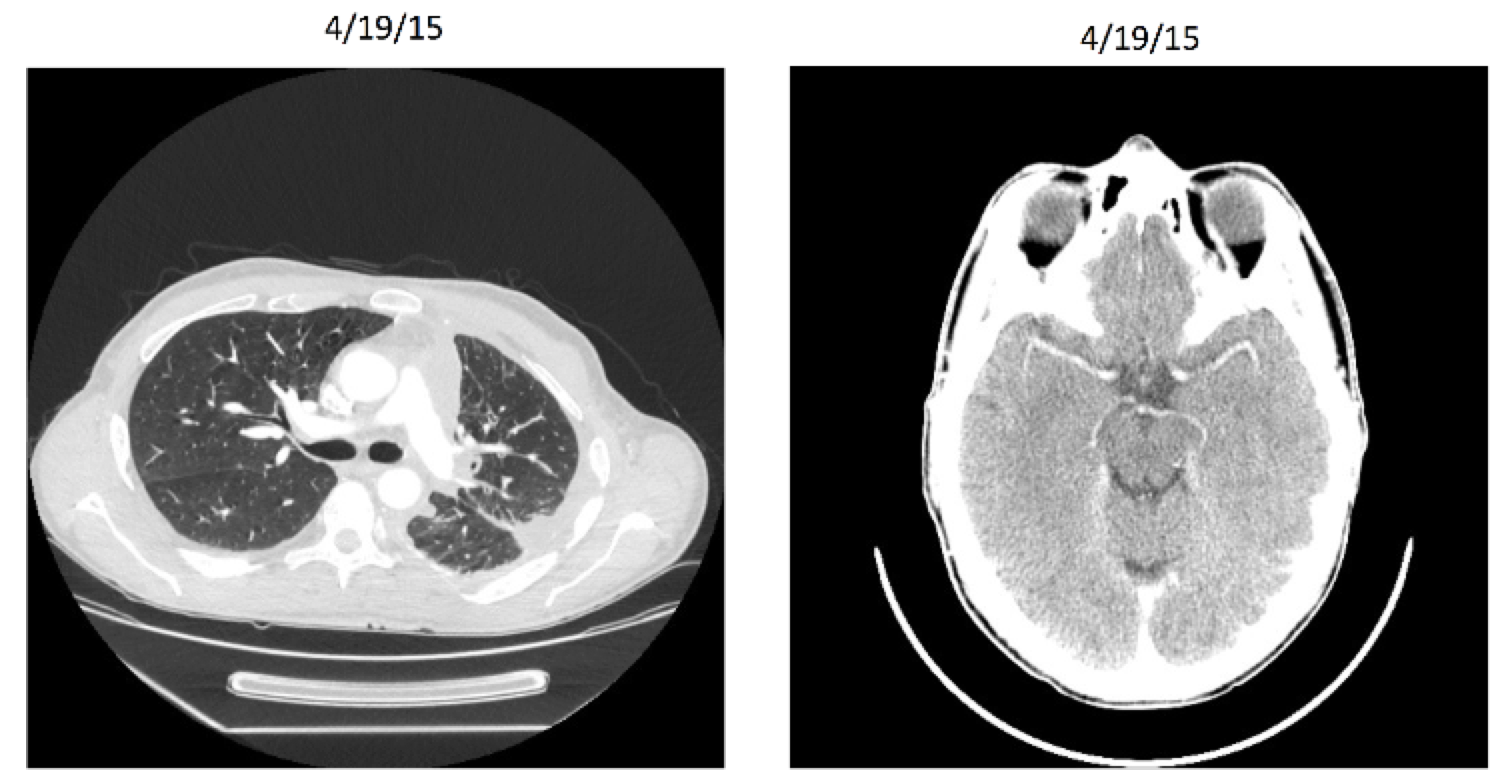
Ignyta Inc. Entrectinib Study: Before
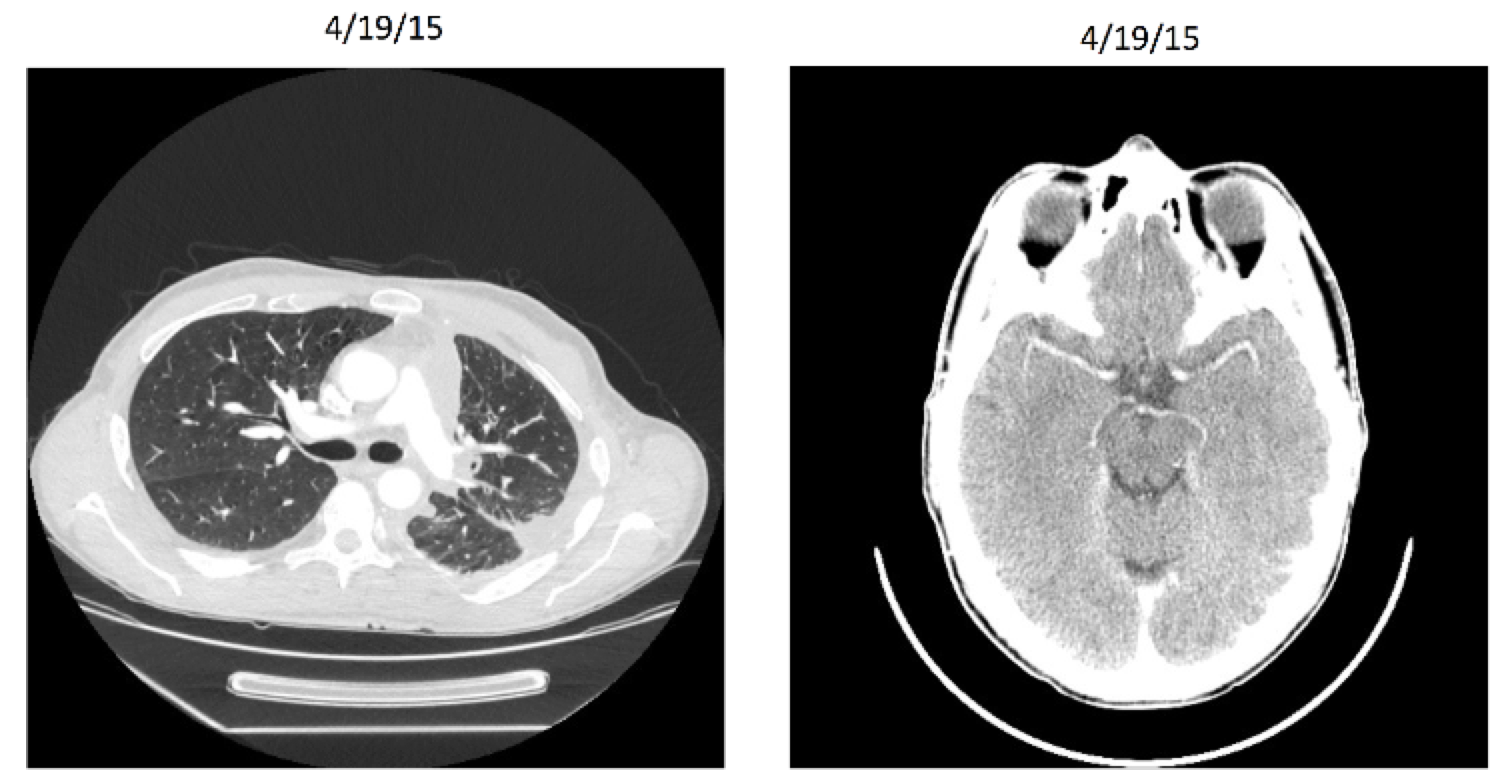
Ignyta Inc. Entrectinib Study: After
This example May extreme but shows what is possible
Precision Therapy Challenges
Traditional:
- Develop the drugs
- Evaluating dose
Current challenges:
- Identifying respsonsive subpopulations
- Powering efficacy trials with smaller sample sizes
Future challenges:
- Transitioning to a residual disease model
IDENTIFYING RESPSONSIVE POPULATIONS
Pre-clinical: cell line Experiments
- grow cancer cells and expose them to a potential therapy
- Measure half the maximal inhibitory concentration (ic50) - how much do I need to affect biological function?
Post-Trial: Subgroup analysis
Cell line experiments create data
REgressors are Biomarkers - Mutation, expression level, etc.
IC50 level is the response - Can we affect function with relatively low dose?
REgressions are high-dimensional
How do we prioritize investigations of HUndreds of samples and tens of thousands biomarkers per sample?
How do we define "response" vs. "non-response" when ic50 is a continuous measure?
How do we resolve results when data-driven analyses give one result and clinical investigators hypothesize another?
How can this be scaled to more markers and models?
PIRLS: Penalized iteratively reweighted least squares
STILL IN DEVELOPMENT BUT AVAILABLE ON GITHUB AT HTTPS://GITHUB.COM/KANEPLUSPLUS/PIRLS
Models supported:
- OLS, GLM, Ridge, LASSO, Elastic net
- need a little more infrastructure for "m" regressions
Plan for out-of-core
Going further: the interactome
Biomarkers are data summaries
- They are derived measurements from the nucleic acid sequence
- Regressions do not take into account the relationships between biomarkers
Create a graphical model from the correlation matrix (preprint here)
- Responsive subpopulations for a given therapy have shared structural differences in their interactomes when compared to a reference group
- Structural differences correspond to the genetic profiles of responders
- We derive specialized biomarkers for individual therapy/genome combinations
Interactome challenges
How can we quantify structural differences between interactomes?
How should we incorporate pathway information?
Thanks
Slides are available at http://slides.com/michaelkane/deck-14
My email is michael dot kane the at symbol yale dot edu.
Precision Medicine: Where Small-Sample Clinical Trials Meet Big Health Data
deck
By Michael Kane
deck
- 2,335