Carol Cuesta-Lazaro (IAIFI Fellow)
and Siddarth Mishra-Sharma (IAIFI Fellow)

Diffusion generative modelling for galaxy surveys



Initial Conditions of the Universe
Gaussian RF
Laws of gravity
3-D distribution of galaxies
Which are the ICs of OUR Universe?
Primordial non-Gaussianity?

3-D distribution of dark matter
Is GR modified on large scales?
How do galaxies form?














Neutrino mass hierarchy?
ML for the Large Scale Structure of the Universe:
Carol's wish list
Generative models
Learn p(x)
Evaluate the likelihood of a 3D map, as a function of the parameters of interest
1
Combine different galaxy properties (such as velocities and positions)
2
Sample 3D maps from the posterior distribution
3
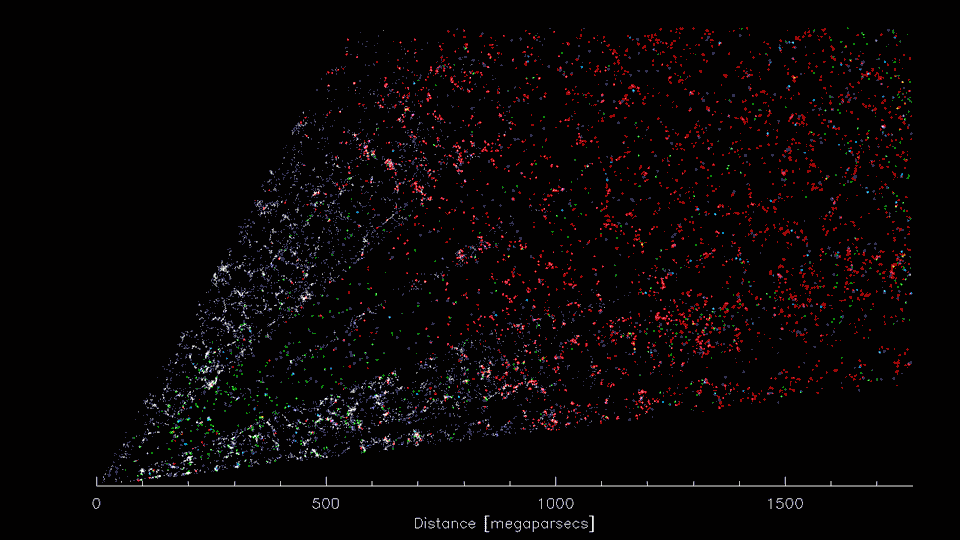

Reverse diffusion: Denoise previous step
Forward diffusion: Add Gaussian noise (fixed)



Diffusion models
A person half Yoda half Gandalf



Diffusion on point clouds
Reverse diffusion: Denoise previous step
Forward diffusion: Add Gaussian noise (fixed)

Cosmology
Node features coordinates (+mass, velocities)
Input
Noisy halo properties
Output
Noise prediction
Graph Neural Networks as score models
kNN (~20)




Halo Mass Function
Velocity
Mean pairwise velocity

Prior loss
Diffusion loss
Reconstruction loss
Be a true Bayesian: Always maximise the likelihood
arxiv:2107.00630
arxiv:2303.00848
Maximum Likelihood = Denoising
Setting tight constraints with only 5000 halo positions



+ Galaxy formation
+ Observational systematics (Cut-sky, Fiber collisions)
+ Lightcone, Redshift Space Distortions....




Forward Model
N-body simulations
Observations
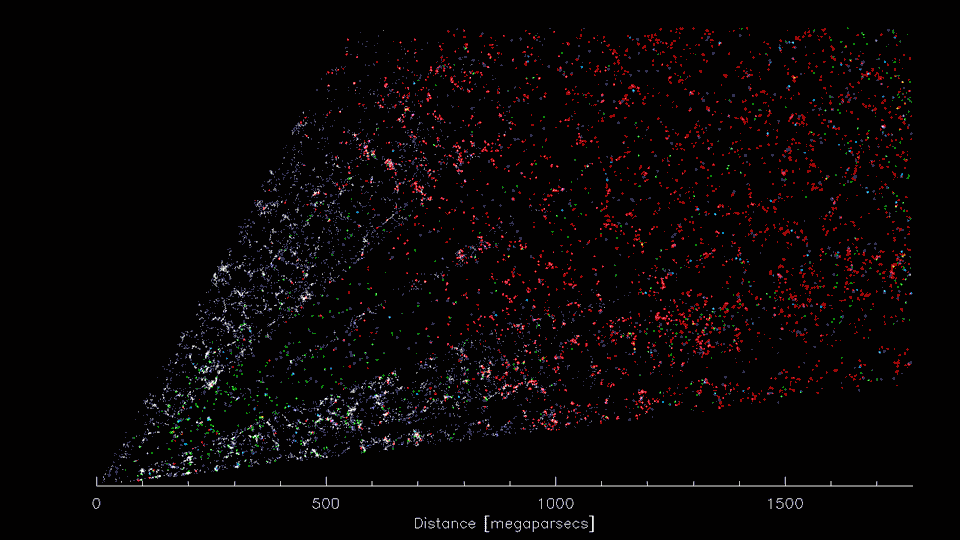
Optimise information on cosmological parameters
(robust) surprises
The challenge for field level inference
Copy of Copy of Copy of Copy of deck
By carol cuesta
Copy of Copy of Copy of Copy of deck
- 428