Enrique Paillas, Pauline Zarrouk, Yan-Chuan Cai, Will Percival, Sesh Nadathur, Mathilde Pinon, Arnaud de Mattia, Florian Beuler
Constraining vΛCDM:
beyond two-point functions
Carolina Cuesta-Lazaro

IAIFI fellow
arXiv:2209.04310












?

Early Universe
~linear

Gravity
Late Universe
Non-linear
Credit: S. Codis+16
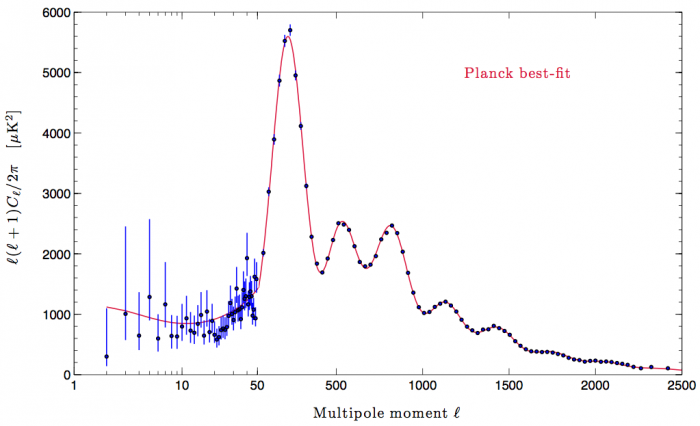
Non-Guassianity
Second moment not optimal













Autocorrelation
Cross-correlation with haloes
Monopole
Quadrupole
Voids
Clusters
Monopole


Where does the information come from?
?
Show comparison Quijote and GRF


# TODO: ADD GREEN AFTERWARDS

But, can we estimate densities realistically?
?
















Autocorrelation of quintiles
Cross-correlation between quintiles and haloes
Monopole
Monopole
Quadrupole
Quadrupole
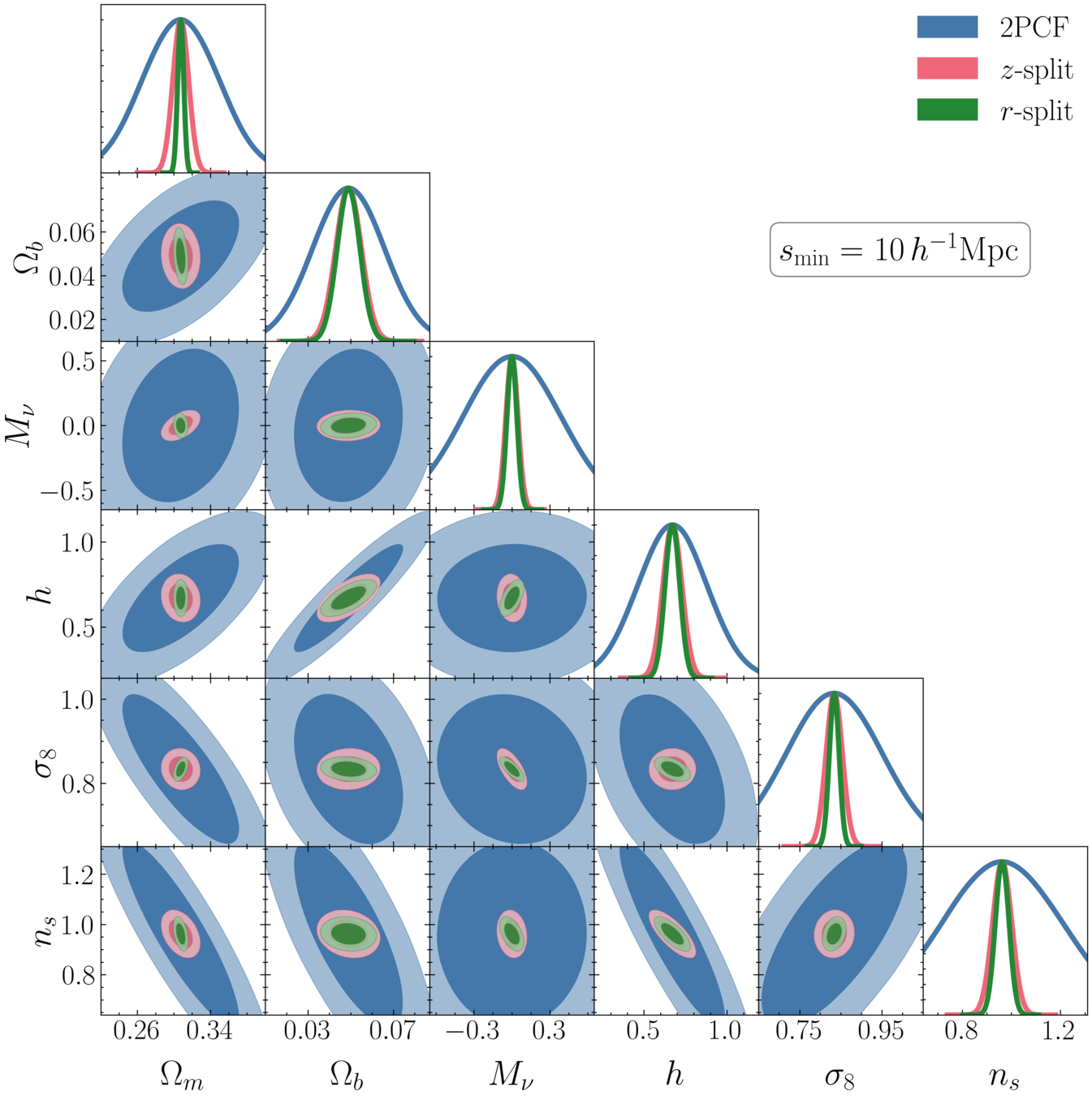
Simulated Data
Data


Prior
Posterior
Inverse problem
Forwards model
Density Estimation with normalising flows

No assumptions on the likelihood (likelihoods rarely Gaussian!)
No expensive MCMC chains needed to estimate posterior
Input
x
Neural network
f
Representation
(Summary statistic)
r = f(x)
Output



Increased interpretability through structured inputs
Modelling cross-correlations
What ML can do for cosmology
- ML to accelerate non-linear predictions and density estimation
- Can ML extract **all** the information that there is at the field-level in the non-linear regime?
- Compare data and simulations, point us to the missing pieces?
cuestalz@mit.edu
Contact me for anything related to Astro and ML!

DensitySplit
By carol cuesta
DensitySplit
- 361