Carol Cuesta-Lazaro (IAIFI Fellow)
in collaboration with Siddarth Mishra-Sharma and Tess Smidt

Diffusion Models for Cosmology
Bridging simulations and observations of the Universe

Initial Conditions of the Universe
Laws of gravity
3-D distribution of galaxies
Which are the ICs of OUR Universe?
What is the origin of these fluctuations?
Probe Inflation
Galaxy formation

3-D distribution of dark matter
Is GR modified on large scales?
How do galaxies form?














ML for Large Scale Structure:
A wish list
Generative models
Learn p(x)
Evaluate the likelihood of a 3D map, as a function of the parameters of interest
1
Combine different galaxy properties (such as velocities and positions)
2
Sample 3D maps from the posterior distribution
3
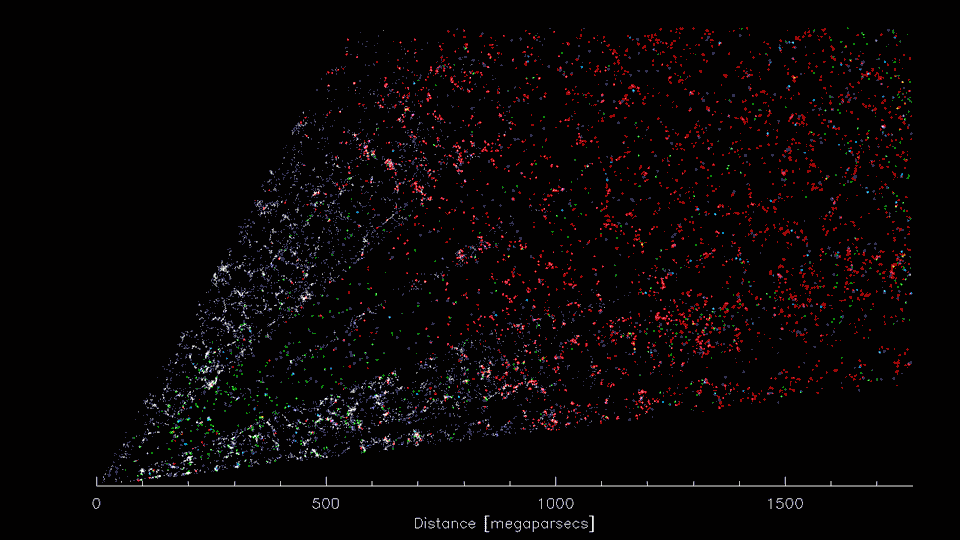

Reverse diffusion: Denoise previous step
Forward diffusion: Add Gaussian noise (fixed)



Diffusion models
A person half Yoda half Gandalf



Diffusion on 6D coordinates
Reverse diffusion: Denoise previous step
Forward diffusion: Add Gaussian noise (fixed)

Cosmology
Tractable likelihood!



Setting tight constraints with only 5000 galaxies
All learnable functions
Equivariant functions
Data constraints
Credit: Adapted from Tess Smidt
Equivariant diffusion
Implications for robustness and interpretability?

+ Galaxy formation
+ Observational systematics (Cut-sky, Fiber collisions)




Forward Model
N-body simulations
Observations


https://arxiv.org/pdf/1611.08606.pdf
Is the model robust to systematic errors?
How are simulations and data different?

deck
By carol cuesta
deck
- 214