Generative Solutions for Cosmic Problems
florpi
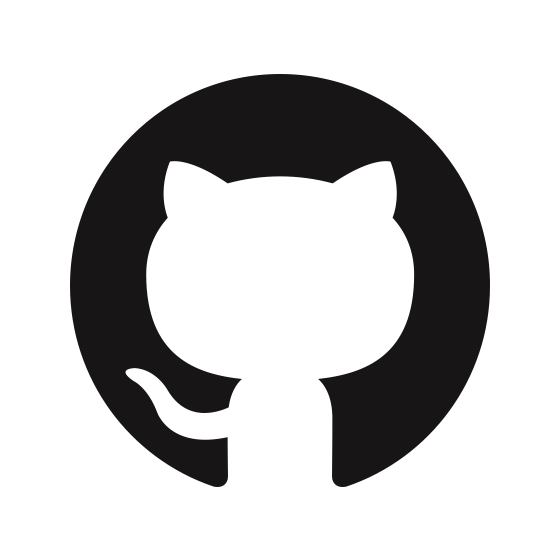
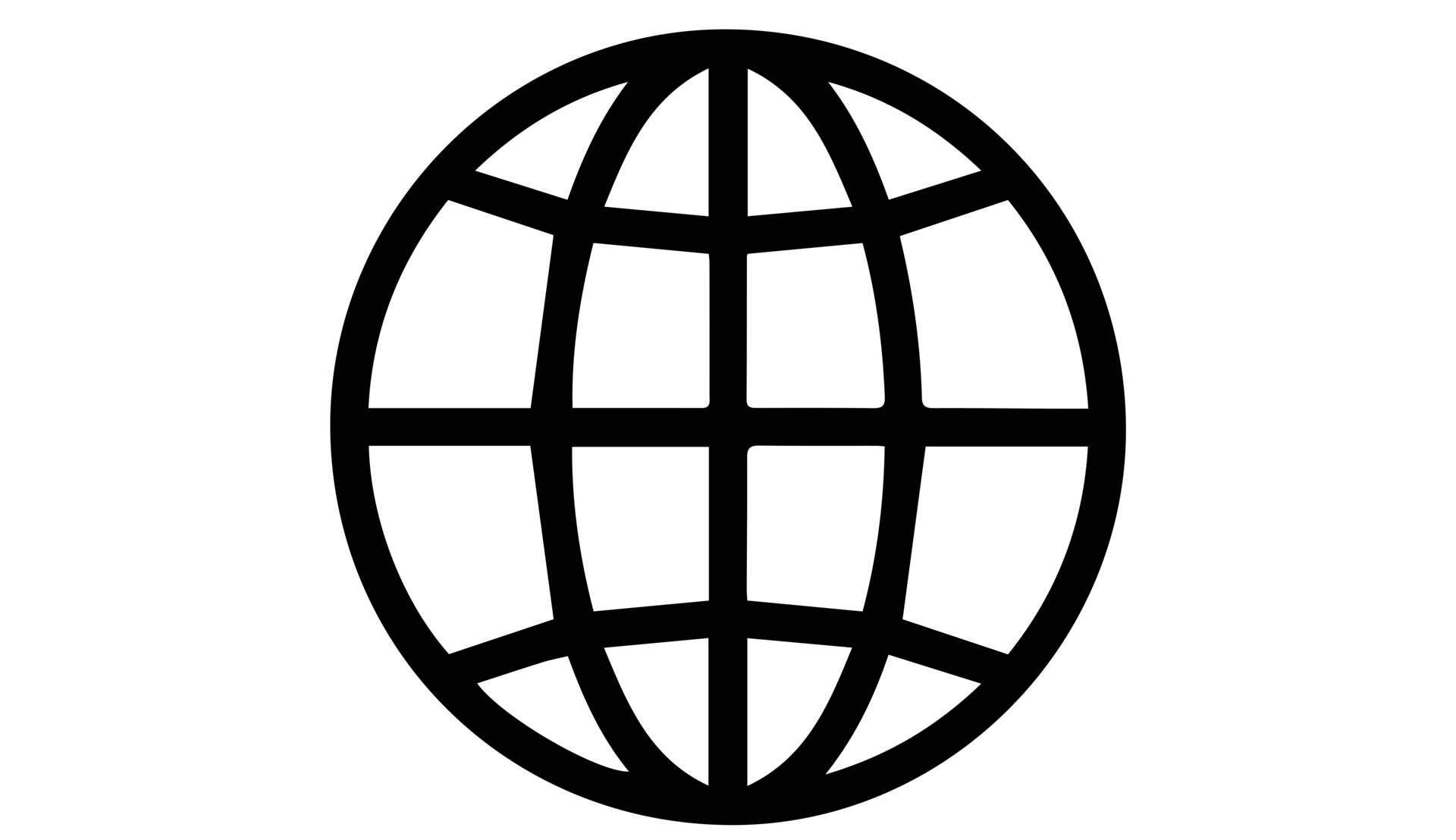
https://florpi.github.io/
IAIFI Fellow
Carol(ina) Cuesta-Lazaro

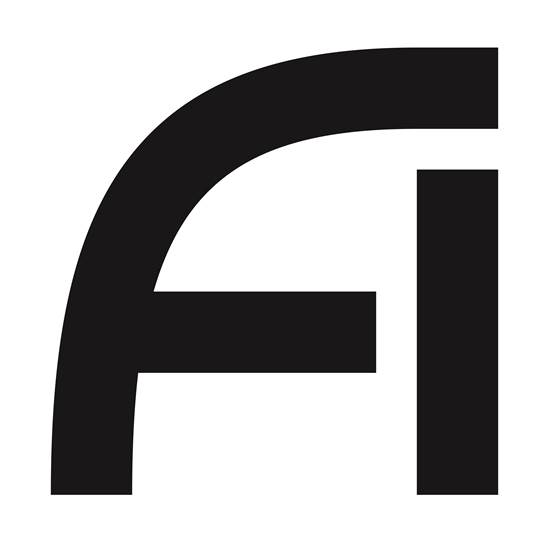
1. Probabilistic debiasing of the cosmic web
2. Physical priors on EFT bias parameters
3. How would a Machine learn a "bias expansion"?
Outline



Inference a la gradient descent
Base Distribution
Target Distribution

Bridging two distributions
Make the data as likely as possible




Prompt
A person half Yoda, half Gandalf

1 to Many:

["Debiasing with Diffusion: Probabilistic reconstruction of Dark Matter fields from galaxies"
Ono et al arXiv:2403.10648]


Victoria Ono
Core Park
1. From Galaxies to Dark Matter


Truth
Sampled


Observed
Small
Large
Scale (k)
Power Spectrum
Small
Large
Scale (k)
Cross correlation
TNG-300
True DM
Inferred DM




Size of training simulation
Galaxy Cluster
Void
[arXiv:2403.10648]
Model trained on Astrid subgrid model

["3D Reconstruction of Dark Matter Fields with Diffusion Models: Towards Application to Galaxy Surveys" Park, Mudur, Cuesta-Lazaro et al (in-prep)]
Posterior Sample
Posterior Mean
Debiasing Cosmic Flows
[Video credit: Francisco Villaescusa-Navarro]
Gas density
Gas temperature
Subgrid model 1
Subgrid model 2
Subgrid model 3
Subgrid model 4
2. But, Simulations are nonsense?

Mikhail Ivanov
Robust galaxy bias model: Effective field Theories
+ Simulation as priors


Field-level EFT
["Full-shape analysis with simulation-based priors: constraints on single field inflation from BOSS" Ivanov, Cuesta-Lazaro et al arXiv:2402.13310]

Andrej Obuljen
Michael Toomey



["Full-shape analysis with simulation-based priors: cosmological parameters and the structure growth anomaly" Ivanov, Obuljen, Cuesta-Lazaro, Toomey arXiv:2409.10609]
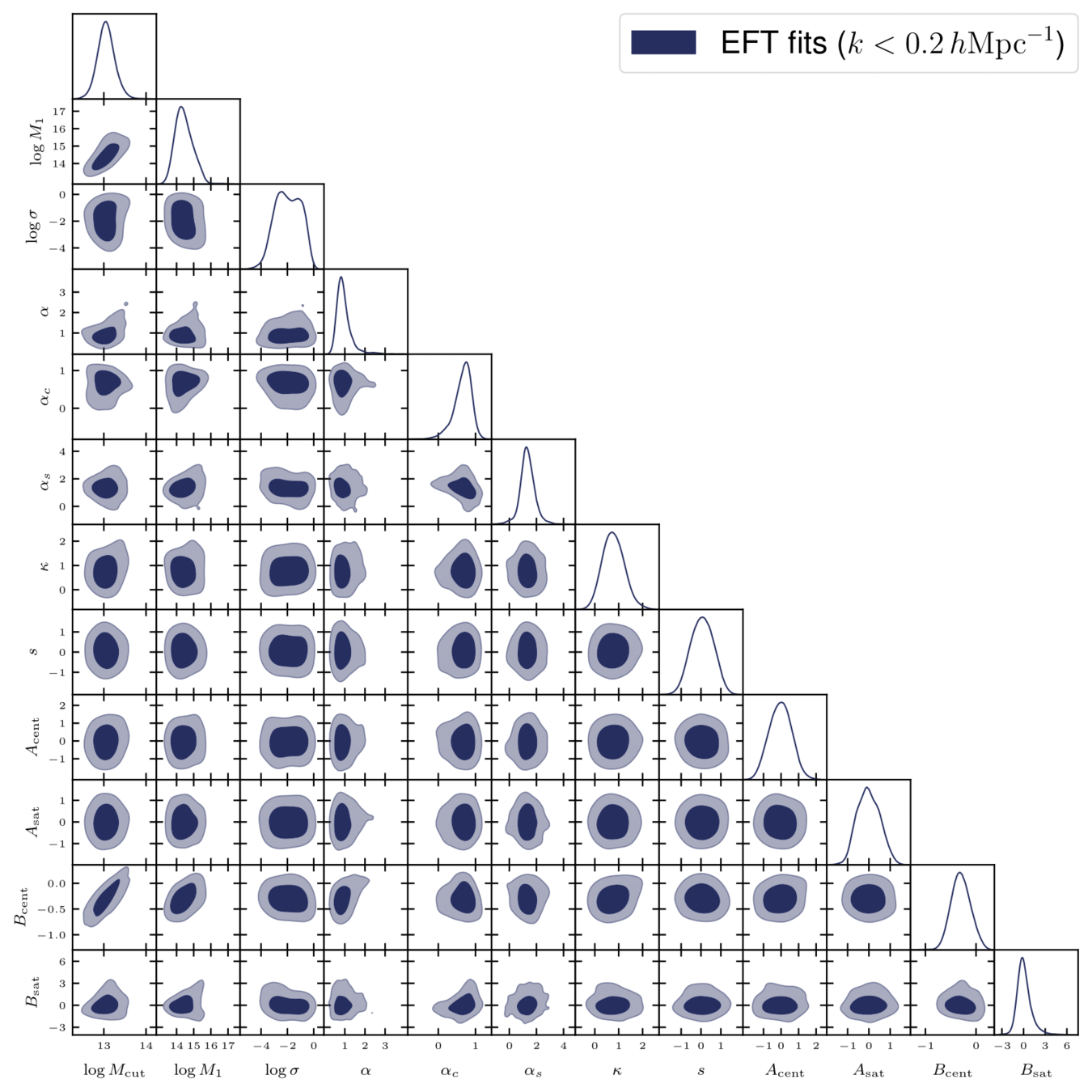


Simulator 1
Simulator 2


Dark Matter
Feedback
i) Contrastive
3. Learning the feedback manifold
Baryonic fields
ii) Generative


Baryonic fields
Dark Matter
Generative model
Total matter, gas temperature,
gas metalicity





Encoder


1. Probabilistic debiasing of the cosmic web
2. Physical priors on EFT bias parameters
3. How would a Machine learn a "bias expansion"?



Mock Barcelona
By carol cuesta
Mock Barcelona
- 227