Practical Applications of Chatbot
Running LLM Model Locally
Frameworks for local LLM

Frameworks
GPT4All
LM Studio
Jan
llamafile
llama.cpp
ollama
NextChat
and more...

框架 | 主要用途 | 使用難度 | 模型格式 | GPU | 最佳化技術 | API支援 | GUI |
---|---|---|---|---|---|---|---|
Ollama | 簡單易用的 LLM 運行框架 | ⭐️⭐️⭐️⭐️⭐️ 最簡單 |
GGUF |
✅ 自動選擇最佳資源 | GGUF 內建最佳化 |
✅ REST API & Python API | ❌ |
LLama.cpp | 高效能 LLM 推理 | ⭐️⭐️⭐️ 需手動調整 |
GGUF | ✅ 需手動設置 | GGUF Metal/ROCM 支援 | ❌ (無內建 API,但可手動添加) | ❌ |
Nextchat | LLM 桌面端 GUI | ⭐️⭐️⭐️⭐️ (簡單) | GGUF | ✅ | GGUF | ❌ | ✅ |
vLLM | 高吞吐量的伺服器級推理 | ⭐️⭐️ (較難) | Hugging Face Transformers (HF) | ✅ 強大 GPU 支援 | PagedAttention、FlashAttention | ✅ (REST API) | ❌ |
LM Studio | GUI 工具,類似 Ollama 但有 UI | ⭐️⭐️⭐️⭐️ (簡單) | GGUF | ✅ | GGUF | ✅ (API 支援) | ✅ |
Jan | LLM 伺服器,適合微調 | ⭐️⭐️ (需安裝設定) | GGUF, HF | ✅ | 量化、微調功能 | ✅ (REST API) | ❌ |
Llamafile | 單一二進位文件運行 LLM | ⭐️⭐️ (需安裝設定) | GGUF | ✅ | GGUF | ❌ | ❌ |
GPT4All | GUI 本地 LLM 運行工具 | ⭐️⭐️⭐️⭐️⭐️ 最簡單 |
GGUF, GPTQ | ✅ | GGUF、GPTQ | ✅ (REST API) | ✅ |
來源:GPT-4-turbo 2024/06版本
Ollama Installation

Ollama download
Get up and running with large language models.

Llama 3.3
DeepSeek R1 1776

Phi-4
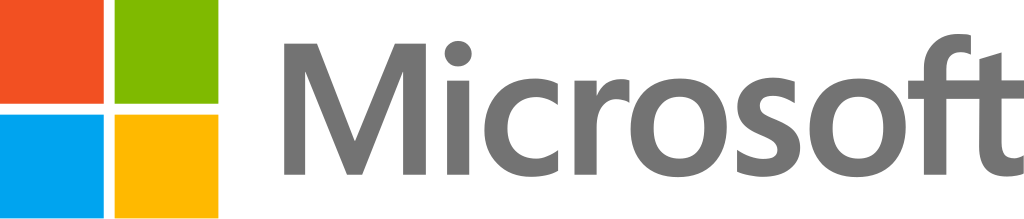
Mistral 0.3

Gemma 2

Ollama Installation

Ollama Command

執行cmd, 輸入ollama
Ollama model支援列表








Ollama model支援列表










Open Source models
LLM releases by year: blue cards = pre-trained models, orange cards = instruction-tuned. Top half shows open-source models, bottom half contains closed-source ones. source: Naveed, H. et. al, 2023, A Comprehensive Overview of Large Language Models





Open Source models

and more...
Ollama model下載/執行
ollama run model-name

執行:
尋找model-name
範例
Ollama model下載/執行

Ollama model下載/執行
結束:

已經下載的model

Ollama local server

Ollama預設於背景執行中web service
Ollama local server
web service結束執行

Ollama local server
ollama serve
重啟web service

web service: http://127.0.0.1:11434 或http://localhost:11434
Ollama 其他指令

Calling Ollama HTTP API
import requests
# 設定 API Endpoint。11434是ollama預設listening port number
# POST /api/generate: 根據'prompt'生成內容
OLLAMA_API_URL = "http://127.0.0.1:11434/api/generate"
# 定義請求資料
data = {
"model": "llama3.2:1b", # 指定使用的模型,例如 'llama3'
"prompt": "Hello, how are you?",
"stream": False # disable streaming,避免收到JSON格式
}
# 發送請求
response = requests.post(OLLAMA_API_URL, json=data)
# 解析回應
if response.status_code == 200:
result = response.json()
print(result["response"]) # 印出回應的文字內容
else:
print("Error:", response.status_code, response.text)
Ollama API generate: 單一回應
import requests
OLLAMA_API_URL = "http://localhost:11434/api/generate"
MODEL = "llama3.2:1b" # 你可以換成 'llama3' 或其他支援的模型
# 初始化對話歷史
chat_history = []
print("💬 Ollama Chatbot 已啟動!輸入 'exit' 可離開對話。\n")
while True:
user_input = input("🧑💻 你:")
if user_input.lower() == "exit":
print("👋 再見!")
break
# 將使用者輸入加到對話歷史
chat_history.append({"role": "user", "content": user_input})
data = { # 構建請求資料
"model": MODEL,
"prompt": user_input,
"stream": False
}
response = requests.post(OLLAMA_API_URL, json=data) # 發送請求
if response.status_code == 200:
result = response.json()
bot_response = result["response"]
print(f"🧑💻 你:{user_input}")
print(f"🤖 Ollama:{bot_response}")
# 儲存機器人的回應到對話歷史
chat_history.append({"role": "assistant", "content": bot_response})
else:
print("❌ API 錯誤:", response.status_code, response.text)
Ollama API generate: 多次回應
import requests
# POST /api/chat: 對話形式
OLLAMA_API_URL = "http://127.0.0.1:11434/api/chat"
# 設定對話歷史(單次對話時,歷史只包含這次輸入)
user_input = "請介紹一下 Llama 3:1B 這個模型"
data = {
"model": "llama3.2:1b", # 指定使用的模型,例如 'mistral' 或 'llama3'
"messages": [
{"role": "user", "content": user_input}
],
"stream": False
}
# 發送請求
response = requests.post(OLLAMA_API_URL, json=data)
# 處理回應
if response.status_code == 200:
result = response.json()
bot_response = result["message"]["content"]
print(f"🤖 Ollama:{bot_response}")
else:
print("❌ API 錯誤:", response.status_code, response.text)
Ollama API chat: 一組Q-A
import requests
# POST /api/chat: 對話形式
OLLAMA_API_URL = "http://127.0.0.1:11434/api/chat"
MODEL = "llama3.2:1b" # 可以換成其他模型,例如 'mistral' 或 'llama3:8b'
chat_history = [] # 初始化對話歷史
print("💬 Ollama Chatbot (Llama3:1B) 已啟動!輸入 'exit' 可離開對話。\n")
while True:
user_input = input("🧑💻 你:")
if user_input.lower() == "exit":
print("👋 再見!")
break
# 將使用者輸入加到對話歷史
chat_history.append({"role": "user", "content": user_input})
data = { # 構建請求資料
"model": MODEL,
"messages": chat_history,
"stream": False
}
response = requests.post(OLLAMA_API_URL, json=data) # 發送請求
if response.status_code == 200:
result = response.json()
bot_response = result["message"]["content"]
print(f"🧑💻 你:{user_input}")
print(f"🤖 Ollama:{bot_response}")
# 儲存機器人的回應到對話歷史
chat_history.append({"role": "assistant", "content": bot_response})
else:
print("❌ API 錯誤:", response.status_code, response.text)
Ollama API chat: 多組Q-A
Ollama python package 呼叫generate api
!pip install ollama
import ollama
# 指定模型名稱
MODEL = "llama3.2:1b" # 可更換成其他模型,例如 'mistral' 或 'llama3:8b'
# 生成文字
response = ollama.generate(
model=MODEL,
prompt="請介紹一下 Llama 3.2:1B 這個模型"
)
# 輸出回應
print(response["response"])
Ollama python package 呼叫chat api
!pip install ollama
import ollama
# 指定模型
MODEL = "llama3.2:1b" # 可換成 'mistral' 或 'llama3:8b'
# 設定對話歷史
messages = [
{"role": "system", "content": "你是一個專業的 AI 助理,會以簡潔的方式回答問題。"},
{"role": "user", "content": "請介紹一下 Llama 3:1B 這個模型"}
]
# 發送聊天請求
response = ollama.chat(
model=MODEL,
messages=messages
)
# 取得機器人回應
print(f"🤖 Ollama:{response['message']['content']}")
Running LLM Locally
By Leuo-Hong Wang
Running LLM Locally
- 87