Cosmology's Midlife Crisis
IAIFI Fellow, MIT
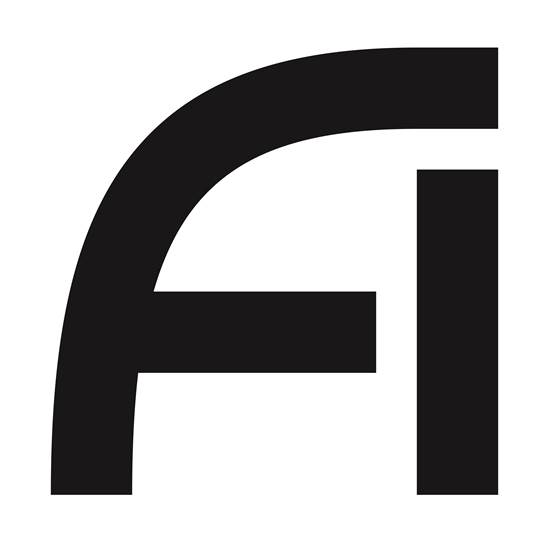
Carolina Cuesta-Lazaro
Art: "A Bar at the Folies-Bergère" by Édouard Manet

Embracing the Machine Learning Makeover
Robust
Very few assumptions about the mechanisms of galaxy formation
Generalizable
Physical Inductive biases
Symmetries: Homogeneous and isotropic Universe on large scales
Precise
(On large scales)
Information Loss
Galaxy formation = Nuisance
Due to being fast
The perks of Perturbation Theory
Small Scales
High dimensional inference
Carolina Cuesta-Lazaro IAIFI/MIT @ Split 2024
Galaxy properties
Astro parametrization
DM density + velocity fields
Target
1. Robust: Mapping dark matter into anything
Carolina Cuesta-Lazaro IAIFI/MIT @ Split 2024
MTNG
Astrid
UniverseMachine
CAMELS
Data
Hydro Sims
SAMS
Santa Cruz
Dark Matter
"Baryons"
Transformer
Carolina Cuesta-Lazaro IAIFI/MIT @ Split 2024
How many parameters can all these simulations share?
Carolina Cuesta-Lazaro IAIFI/MIT @ Split 2024
MTNG
Astrid
UniverseMachine
CAMELS
Data
Hydro Sims
SAMS
Santa Cruz
Can we fit the remaining to observations directly?
2. Generalizable: Representation learning
Learning to represent feedback
Carolina Cuesta-Lazaro IAIFI/MIT @ Split 2024
SIMBA
TNG
Astrid
Different Supernovae and AGN feedback mechanisms
+ Different parametrisations
Finding anomalies
Z


"A point cloud approach to generative modeling for galaxy surveys at the field level"
Cuesta-Lazaro and Mishra-Sharma
arXiv:2311.17141
Base Distribution
Target Distribution
- Sample
- Evaluate
3. Physical Inductive biases
Carolina Cuesta-Lazaro IAIFI/MIT @ Split 2024

Same model architecture (transformer) on direct inference wouldn't work at all!
Carolina Cuesta-Lazaro IAIFI/MIT @ Split 2024
Making homogeneous and isotropic Universes
Base Distribution
Denoiser
Invariant
Equivariant
Carolina Cuesta-Lazaro IAIFI/MIT @ Split 2024
All learnable functions
All learnable functions constrained by your data
All Equivariant functions
More data efficient!
Carolina Cuesta-Lazaro IAIFI/MIT @ Split 2024

Equivariant models
Non-equivariant
SEGNN: arXiv:2110.02905
NequIP: arXiv:2101.03164
Carolina Cuesta-Lazaro IAIFI/MIT @ Split 2024
Robust
Very few assumptions about the mechanisms of galaxy formation
Generalizable
Physical Inductive biases
Symmetries: Homogeneous and isotropic Universe on large scales
Precise
(On large scales)
Information Loss
Galaxy formation = Nuisance
Due to being fast
The perks of Machine Learning
Small Scales
High dimensional inference
Carolina Cuesta-Lazaro IAIFI/MIT @ Split 2024
Learned
Learned representations
Symmetries: Homogeneous and isotropic Universe on large scales
as long as we can sufficiently simulate
Interesting Physics
Perturbation Theory's retirement plan:
The Physics of Machine Learning
Carolina Cuesta-Lazaro IAIFI/MIT @ Split 2024

Grokking

Double Descent
Emergent abilities

Perturbation Theory's Midlife Crisis
Embracing the Machine Learning Makeover
IAIFI Fellow
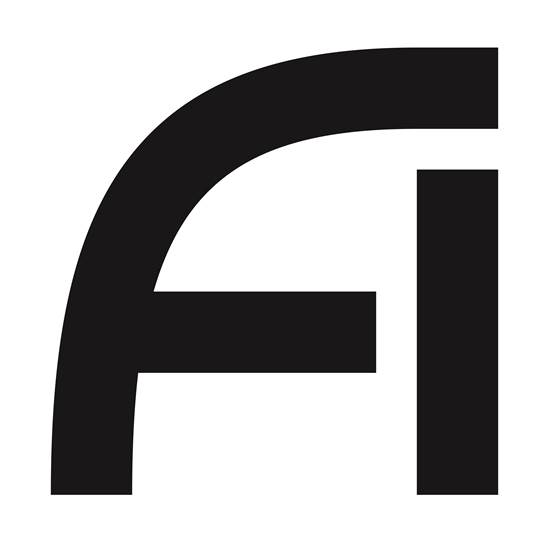
Carolina Cuesta-Lazaro
Art: "Melancholy" by Edvard Munch

Perturbation Theory's Midlife Crisis
Embracing the Machine Learning Makeover

IAIFI Fellow
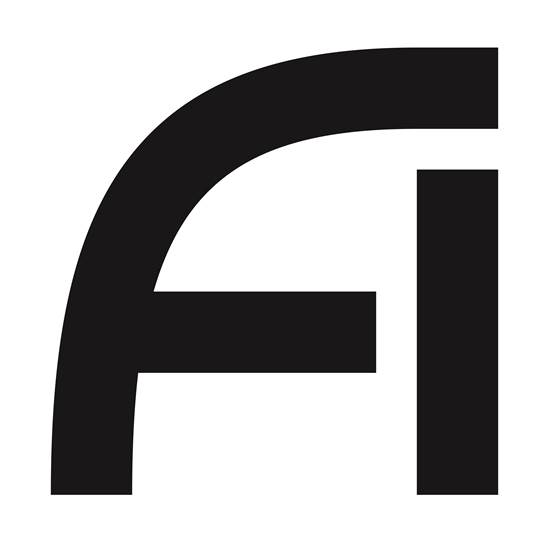
Carolina Cuesta-Lazaro
Art: "Midlife crisis" by Nik Ad

Fixed Initial Conditions
Varying Cosmology
Carolina Cuesta-Lazaro IAIFI/MIT @ Split 2024
- "Cosmic Plot Twist: When Algorithms Crash the Perturbation Party"
- "Perturbation Theory's Retirement Plan:
- Breaking Up with Perturbation Theory: It's Not You, It's AI
IAIFI Fellow, MIT
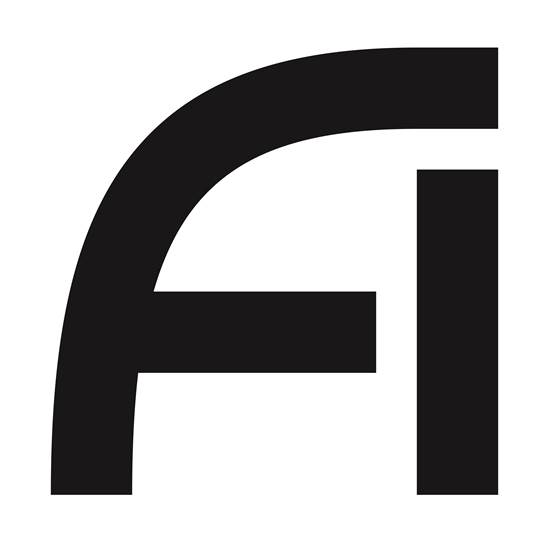
Carolina Cuesta-Lazaro
Art: "A Bar at the Folies-Bergère" by Édouard Manet

Embracing the Machine Learning Makeover
Split-Croatia2024
By carol cuesta
Split-Croatia2024
- 250