The secret life of an IAIFI Fellow
florpi
Building a space for the intersection of Physics and AI
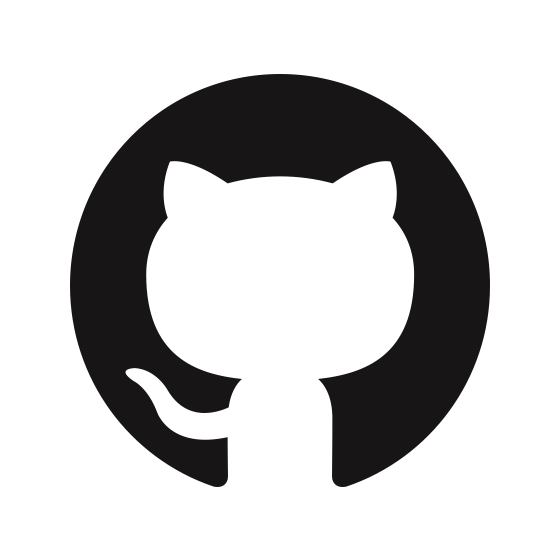
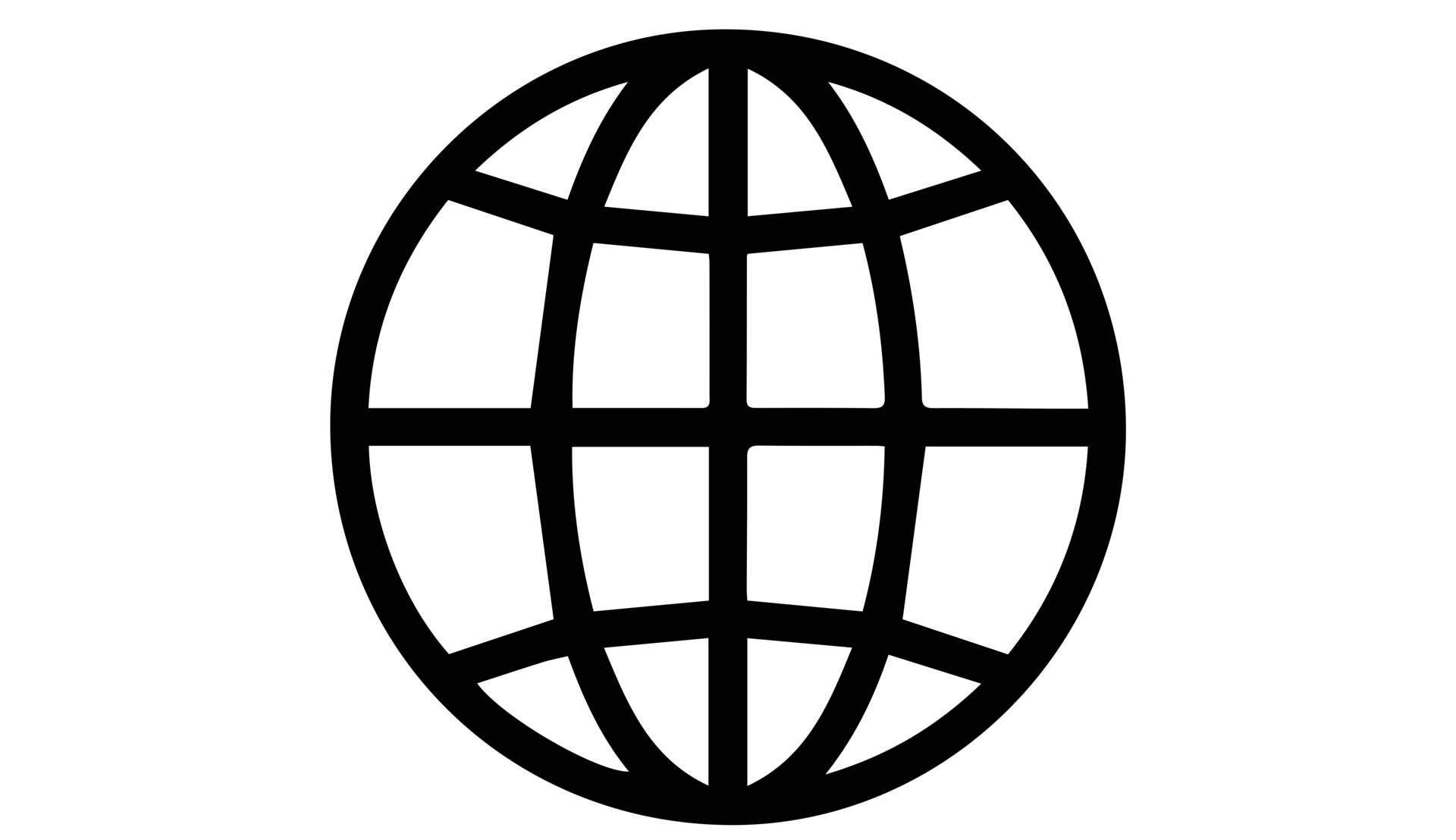
https://florpi.github.io/
IAIFI Fellow
Carol Cuesta-Lazaro

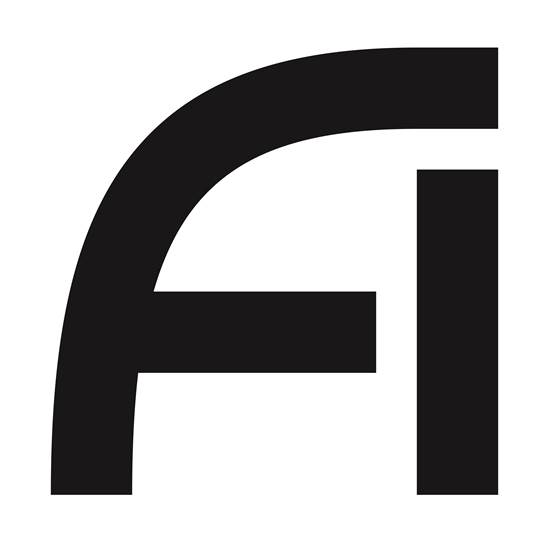
Undergrad in Physics
Madrid (Spain)
Heidelberg (Germany)
2017: first ML project as an assistant researcher
First time can combine all my interests!


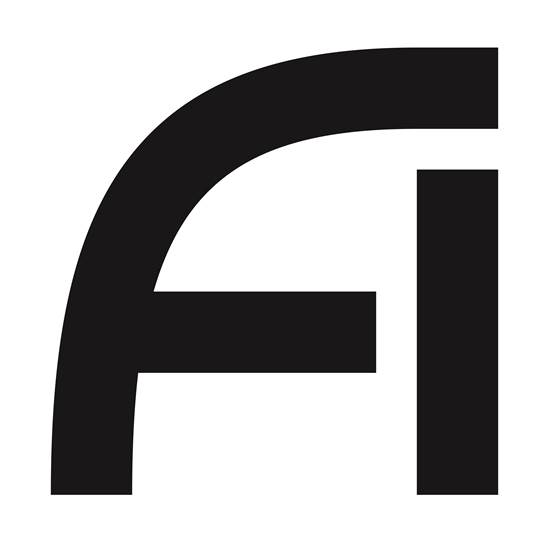
PhD in Data Intensive Science
Durham (UK)
Working on cosmology (theory)
+ Industry placements



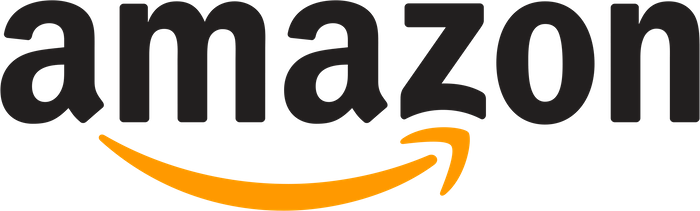



IAIFI Committees
How I thought it would look like
How it looks like
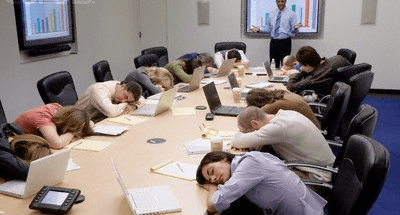
Industry Partnership
Helped organise industry lunches
Early Career and Equity
Moderated panel on Industry x Academia collaborations (IAIFI workshop 2024)
Yearly gathering feedback from ECRs
Pushed for fellows involvement in felowship hiring


(Marisa and Thomas thinking about all the work this will really take that we are totally overlooking)
IAIFI Activities


Outreach
AstroxML Hackathon
Teaching




AstroML Hackathon 2024
LatamSummerSchool 2023
HerWILL 2024
IAIFISummerSchool2024
CambridgeScienceFestival2022
Ethics&ML2023
My research interests

AI4Science
Equivariance & Symmetries
Anomaly detection
Out-of-Distribution
Interpretability
Quantifying Uncertainties
Partial Observations
PDEs
Multimodal
Simulation-based-Inference
Foundation Models
Hierarchical
IAIFI Coffee
Hanging out at the penthouse


IAIFI Seminars
Hackathons



["A point cloud approach to generative modeling for galaxy surveys at the field level"
Cuesta-Lazaro and Mishra-Sharma
arXiv:2311.17141]
Base Distribution
Target Distribution
- Sample
- Evaluate

Siddharth Mishra-Sharma
IAIFI Fellow
Modelling Galaxy Point Clouds
ICML AstroML Spotlight 2023

Fixed Initial Conditions / Varying Cosmology

Diffusion model

CNN
Diffusion
Increasing Noise
["Diffusion-HMC: Parameter Inference with Diffusion Model driven Hamiltonian Monte Carlo"
Mudur, Cuesta-Lazaro and Finkbeiner]

Nayantara Mudur


CNN
Diffusion
Douglas Finkbeiner

IAIFI JI
IAIFI SI
NeurIPs ML for the Physical Sciences 2023


All learnable functions
All learnable functions constrained by your data
All Equivariant functions
More data efficient!
Incorporating symmetries in ML architectures

Non-equivariant
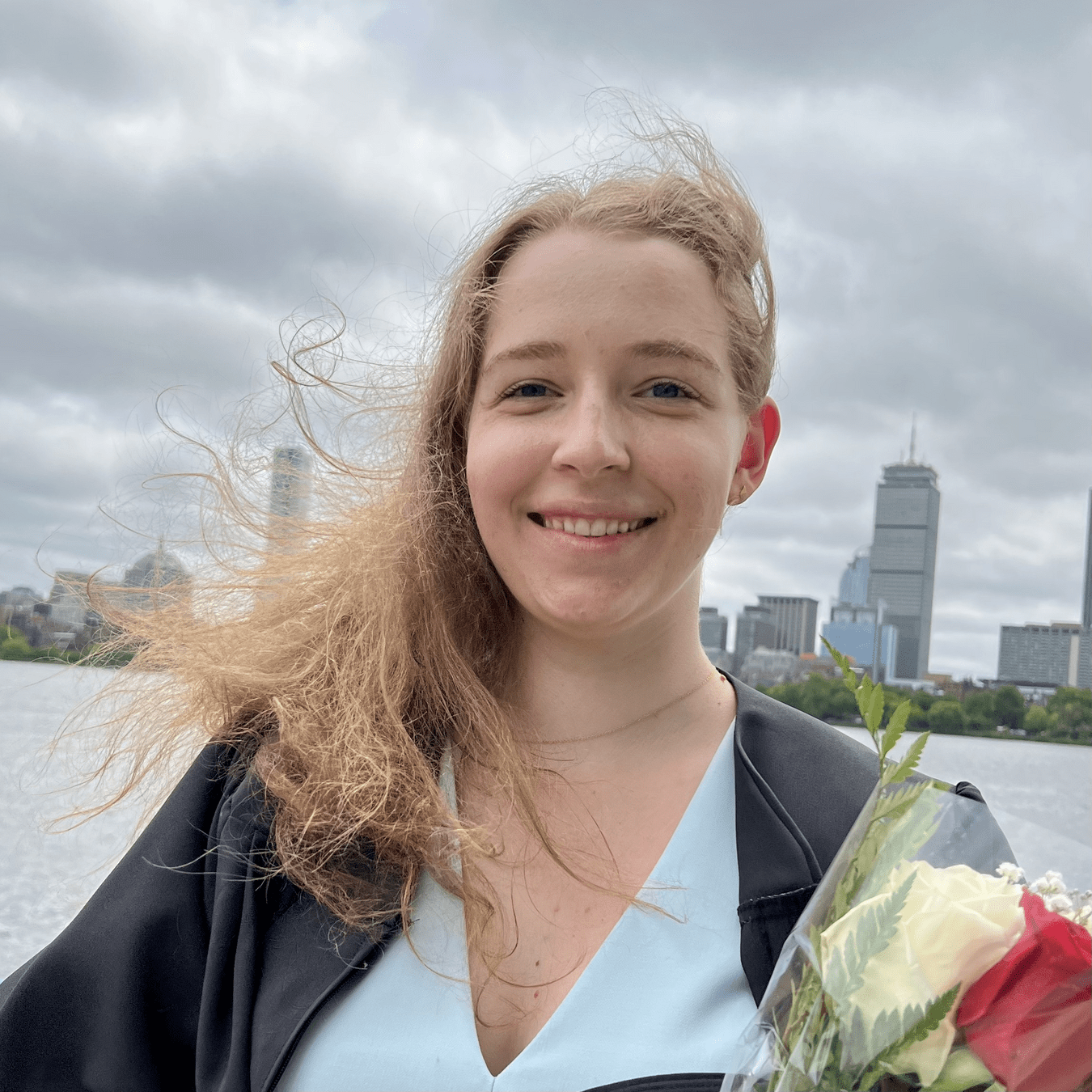

Julia Balla
Siddharth Mishra-Sharma
> x2 in constraining power
From Angstroms to Gigaparsecs
Tess Smidt
IAIFI JI
IAIFI Fellow
IAIFI SI



1 to Many:
["Debiasing with Diffusion: Probabilistic reconstruction of Dark Matter fields from galaxies"
Ono et al arXiv:2403.10648]


Victoria Ono
Core Park

Truth DM
Inferred DM


Observed

Nayantara Mudur
Probabilistic dark matter mapping
NeurIPs Physical Sciences 2023
IAIFI JI
Harvard students




["3D Reconstruction of Dark Matter Fields with Diffusion Models: Towards Application to Galaxy Surveys" Park, Mudur, Cuesta-Lazaro et al (in-prep)]
Posterior Sample
Posterior Mean
Debiasing Cosmic Flows
ICML AI4Science 2024
Rewinding the Universe
Stochastic Interpolants
NF

Michael Albergo
IAIFI Fellow

Daniel Eisenstein
IAIFI SI



Simulating what you need
(and sometimes what you want)

Compressing cosmological simulations

Brandon Y. Feng
(in Bill Freeman's group)
IAIFI SI
Ge Yang talking about NERFs at IAIFI colloquium

~ 10 trillion particles per snapshot stored
x Discrete snapshots
Can we learn compressed continuous representations with Neural Fields?



IAIFI Coffee
Learning priors from simulations

Mikhail Ivanov

IAIFI Affiliate
Why IAIFI works
Bring postdocs excited about AI+Physics
+ get them great junior collaborators
+ gpus
=
New ideas, best practices and state of the art ML
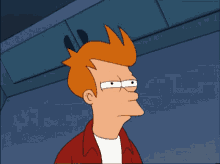

+ resources
New community at the intersection
summer schools, hackathons, workshops
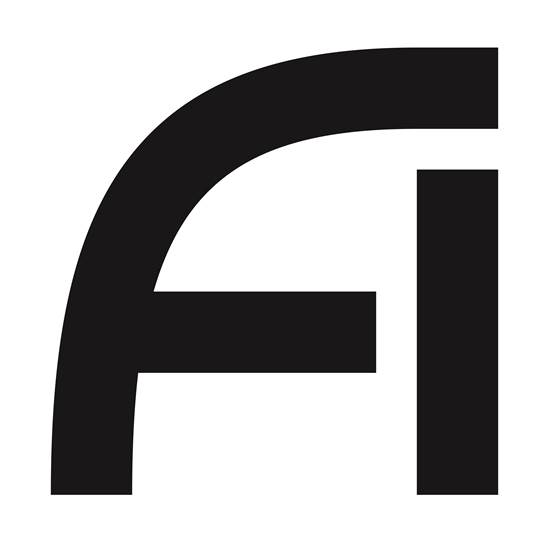

deck
By carol cuesta
deck
- 6