Carol Cuesta-Lazaro (IAIFI Fellow)
in collaboration with Siddarth Mishra-Sharma

Diffusion Models for Cosmology



Initial Conditions of the Universe
Laws of gravity
3-D distribution of galaxies
Which are the ICs of OUR Universe?
Primordial non-Gaussianity?
Probe Inflation
Galaxy formation

3-D distribution of dark matter
Is GR modified on large scales?
How do galaxies form?














Neutrino mass hierarchy?
ML for Large Scale Structure:
A wish list
Generative models
Learn p(x)
Evaluate the likelihood of a 3D map, as a function of the parameters of interest
1
Combine different galaxy properties (such as velocities and positions)
2
Sample 3D maps from the posterior distribution
3
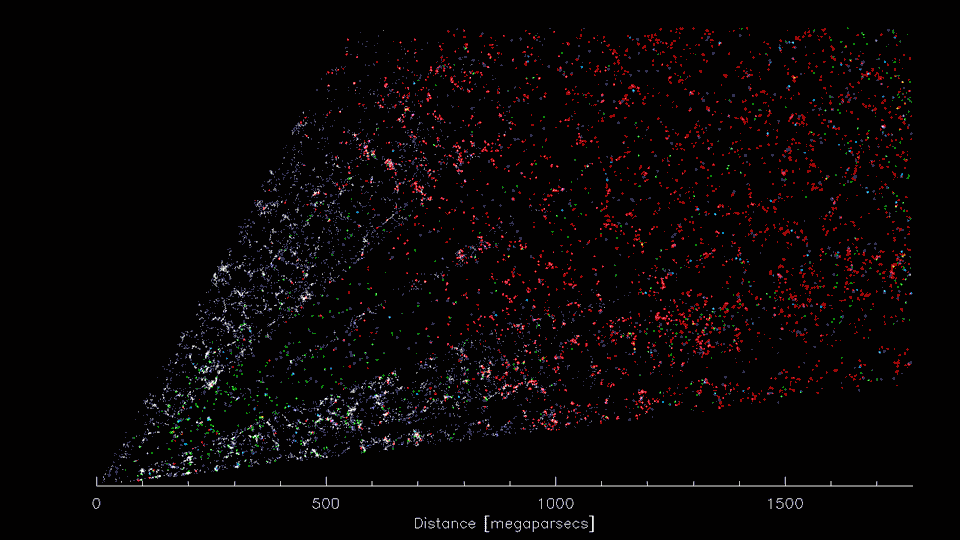
Explicit Density
Implicit Density
Tractable Density
Approximate Density
Normalising flows
Variational Autoencoders
Diffusion models
Generative Adversarial Networks
The zoo of generative models

Reverse diffusion: Denoise previous step
Forward diffusion: Add Gaussian noise (fixed)



Diffusion models
A person half Yoda half Gandalf



Diffusion on 3D coordinates
Reverse diffusion: Denoise previous step
Forward diffusion: Add Gaussian noise (fixed)

Cosmology






Setting tight constraints with only 5000 halos





Node features
Edge features
edge embedding
node embedding
Input
noisy halo properties
Output
noise prediction
A variational Lower Bound

Inference is hard....
known PDF with some free parameters
Kullback-Leibler divergence
distance between two distributions
Not symmetric!
A variational Lower Bound
Inference = Optimisation
only if q perfectly describes p!
Ensure consistency forward/reverse
Challenge Learning a well calibrated likelihood from only 2000 N-body simulations
All learnable functions
Equivariant functions
Data constraints
Credit: Adapted from Tess Smidt
Equivariant diffusion
Implications for robustness and interpretability?

+ Galaxy formation
+ Observational systematics (Cut-sky, Fiber collisions)
+ Lightcone, Redshift Space Distortions....




Forward Model
N-body simulations
Observations
SIMBIG arXiv:2211.00723
Copy of deck
By carol cuesta
Copy of deck
- 390