Enrique Paillas, Pauline Zarrouk, Yan-Chuan Cai, Will Percival, Sesh Nadathur, Mathilde Pinon, Arnaud de Mattia, Florian Beuler
Constraining vΛCDM:
beyond two-point functions
Carolina Cuesta-Lazaro

IAIFI fellow - MIT/CfA
arXiv:2209.04310












?
The golden days of Cosmology:
A five parameter Universe
Initial Conditions
Dynamics
Dark energy
Dark matter
Ordinary matter
Amplitude initial density field
Scale dependence

Neutrino mass first evidence beyond SM physics + LSS most accurate measurement
Primordial Non-gaussianity to probe the physics of inflation (single/multi field, particle content)
Galaxy formation not only a nuisance to margnilize over!
Large scale modifications of gravity using growth to detect the existence of fifth forces
What we expect to learn from the LSS


Linear


Early Universe
~linear

Gravity
Late Universe
Non-linear
Credit: S. Codis+16
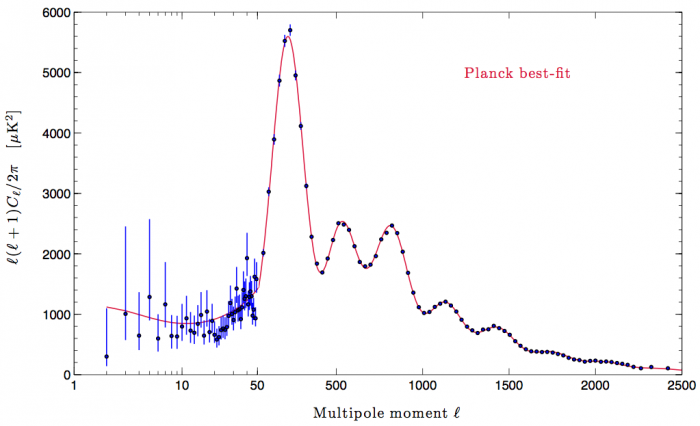
Non-Guassianity
Second moment not optimal












arxiv:1911.11158












Autocorrelation
Cross-correlation with haloes
Monopole
Quadrupole
Voids
Clusters


Estimating sensitivity to cosmology: Fisher information

The Quijote simulations
arXiv:1909.05273
Covariance
Derivatives

Finite differences



Where does the information come from?
?

Density split in a Gaussian Random Field



But, can we estimate densities realistically?
?














Real
Redshift

Cross-correlation between quintiles and haloes
Monopole
Quadrupole
Monopole
Quadrupole

Autocorrelation of quintiles
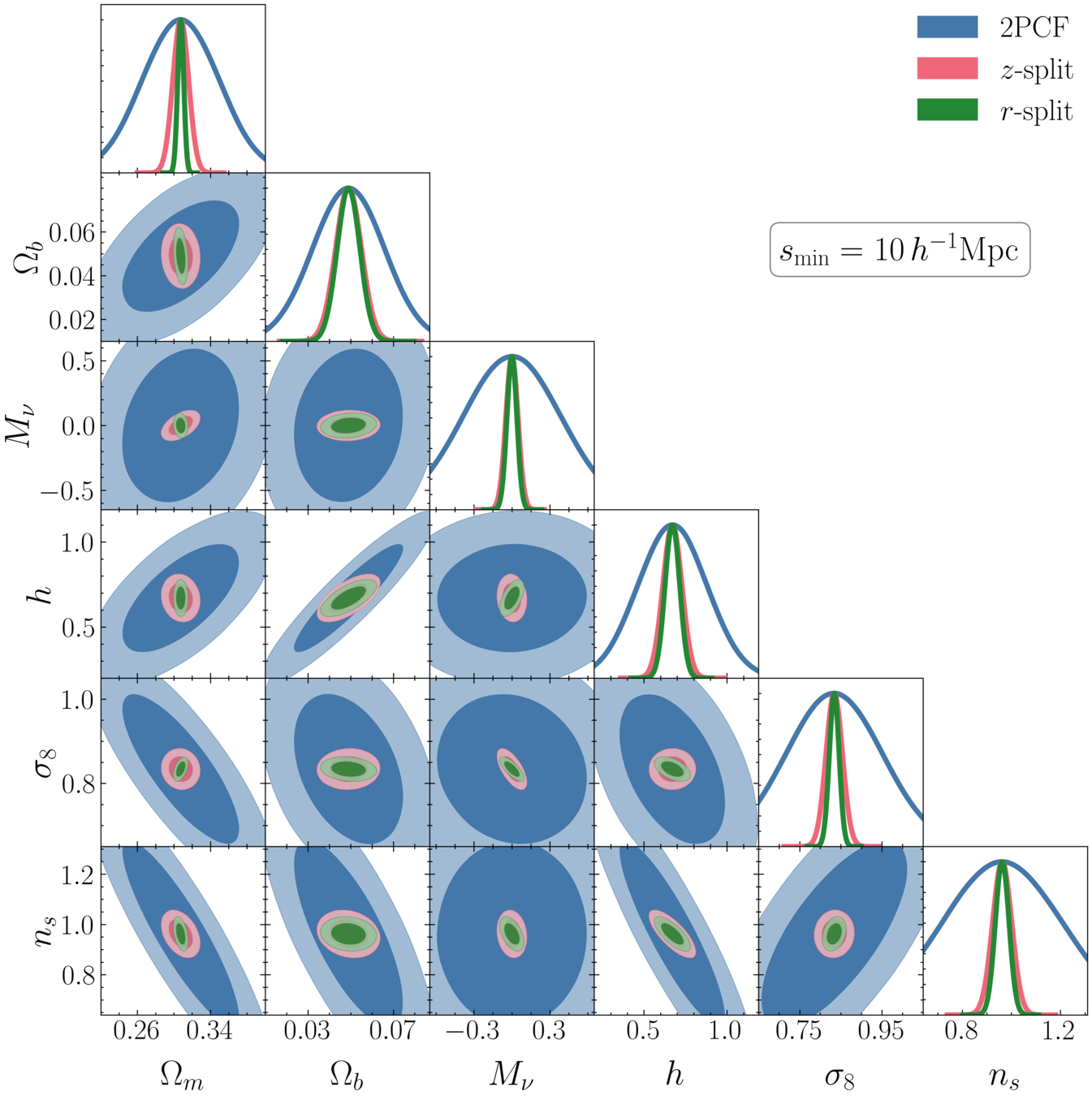

Comparison against other works that have used the same set of tracers
Simulated Data
Data


Prior
Posterior
Inverse problem
Forwards model

+ Galaxy-Halo connection
+ Cut-sky
+ Lightcone
+ Alcock-Paczinsky
+ Fiber collisions




Forward Model
N-body simulations
Observations


Density split in a SDSS BOSS

CMASS 0.46 < z < 0.6


Galaxy-Halo connection

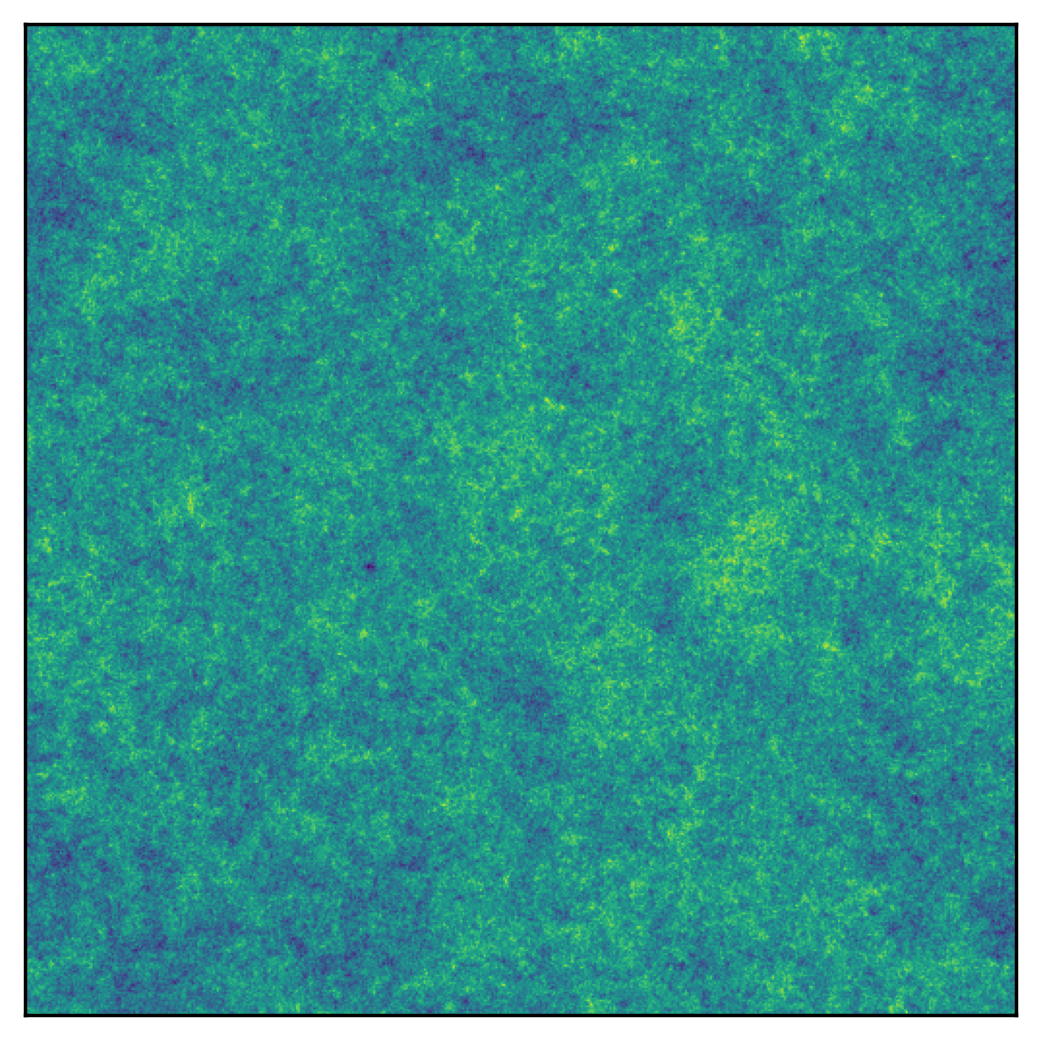














Main Assumptions
- Galaxies don't impact dark matter clustering
- Number of galaxies depends on halo mass only
Extensions: Get creative!
But these extensions might limit our constraining power
a) Use hydro simulations to limit options
b) Mask the data to optimise robustness?
Velocity bias
Assembly bias
Environment
Concentration
Formation time
...

Credit: https://cs231n.github.io/convolutional-networks/
Neural Network emulator
Implicit likelihood inference with normalising flows

No assumptions on the likelihood (likelihoods rarely Gaussian!)
No expensive MCMC chains needed to estimate posterior
Input
x
Neural network
f
Representation
(Summary statistic)
r = f(x)
Output



Increased interpretability through structured inputs
Modelling cross-correlations
What ML can do for cosmology
- ML to accelerate non-linear predictions and density estimation
- Can ML extract **all** the information that there is at the field-level in the non-linear regime?
- Compare data and simulations, point us to the missing pieces?
cuestalz@mit.edu

Copy of DensitySplit
By carol cuesta
Copy of DensitySplit
- 439