One Network to Rule Them All
Multi-Modal Foundation Models for Survey Astronomy
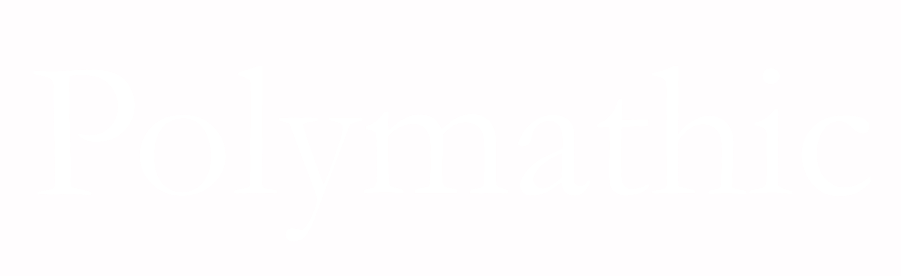
Francois Lanusse
CNRS

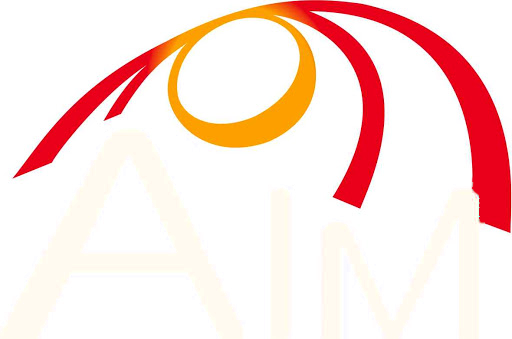

The Deep Learning Boom in Astrophysics

astro-ph abstracts mentioning Deep Learning, CNN, or Neural Networks
The vast majority of these results has relied on supervised learning and networks trained from scratch.

The Limits of Traditional Deep Learning
-
Limited Supervised Training Data
- Rare or novel objects have by definition few labeled examples
- In Simulation Based Inference (SBI), training a neural compression model requires many simulations
- Rare or novel objects have by definition few labeled examples
-
Limited Reusability
- Existing models are trained supervised on a specific task, and specific data.


=> Limits in practice the ease of using deep learning for analysis and discovery

Meanwhile, in Computer Science...

The Rise of The Foundation Model Paradigm
-
Foundation Model approach
- Pretrain models on pretext tasks, without supervision, on very large scale datasets.
- Adapt pretrained models to downstream tasks.
- Combine pretrained modules in more complex systems.



The Advantage of Scale of Data and Compute


Linearly Accessible Information

- Backbone of modern architectures embed input images as vectors in where d can typically be between 512 to 2048.
- Linear probing refers to training a single matrix to adapt this vector representation to the desired downstream task.


What This New Paradigm Could Mean for Us
-
Never have to retrain my own neural networks from scratch
-
Existing pre-trained models would already be near optimal, no matter the task at hand
-
Existing pre-trained models would already be near optimal, no matter the task at hand
- Practical large scale Deep Learning even in very few example regime
-
Searching for very rare objects in large surveys like Euclid or LSST becomes possible
-
Searching for very rare objects in large surveys like Euclid or LSST becomes possible
- If the information is embedded in a space where it becomes linearly accessible, very simple analysis tools are enough for downstream analysis
- In the future, survey pipelines may add vector embedding of detected objects into catalogs, these would be enough for most tasks, without the need to go back to pixels

=> Alright, lets make it happen!
Step I: Data!

The Multimodal Universe
Enabling Large-Scale Machine Learning with 100TBs of Astronomical Scientific Data

Collaborative project with about 30 contributors
Presented at NeurIPS 2024 in Datasets & Benchmark track




The barrier to universal datasets
- Development of foundation models has relied on access to "web scale" datasets
- Astrophysics generates large amounts of publicly available data
BUT:- data is usually not stored or structured in an ML friendly way (e.g. postage stamps).
- data access varies significantly between survey
- Accessing and using scientific data requires significant expertise, for each dataset.


The MultiModal Universe Project
- Goal: Assemble the first large-scale multi-modal dataset for machine learning in astrophysics.
-
Main pillars:
- Engage with a broad community of AI+Astro experts.
- Adopt standardized conventions for storing and accessing data and metadata through mainstream tools (e.g. Hugging Face Datasets).
- Target large astronomical surveys, varied types of instruments, many different astrophysics sub-fields.






Multiband images from Legacy Survey


MMU Infrastructure

Data schema and storage
- For each example MMU expects a few mandatory fields:
- object_id, ra, dec
- object_id, ra, dec
- For each modality, MMU expects the data to be formatted according to a fixed schema which contains necessary metadata.
- Data is stored in HDF5 files, split according to HEALPix regions for efficient cross-matching and easy access

hsc
├── hsc.py
├── pdr3_dud_22.5
│ ├── healpix=1104
│ │ └── 001-of-001.hdf5
│ ├── healpix=1105
│ │ └── 001-of-001.hdf5
│ ├── healpix=1106
│ │ └── 001-of-001.hdf5
│ ├── healpix=1107
│ │ └── 001-of-001.hdf5
│ ├── healpix=1171
│ │ └── 001-of-001.hdf5
│ ├── healpix=1172
│ │ └── 001-of-001.hdf5
│ ├── healpix=1174
│ │ └── 001-of-001.hdf5
│ ├── healpix=1175
│ │ └── 001-of-001.hdf5
│ ├── healpix=1702
│ │ └── 001-of-001.hdf5
...

Content of v1


Usage example
from datasets import load_dataset
# Open Hugging Face dataset
dset_ls = load_dataset("MultimodalUniverse/legacysurvey",
streaming=True,
split='train')
dset_ls = dset_ls.with_format("numpy")
dset_iterator = iter(dset_ls)
# Draw one example from the dataset iterator
example = next(dset_iterator)
# Let's inspect what is contained in an example
print(example.keys())
figure(figsize=(12,5))
for i,b in enumerate(example['image']['band']):
subplot(1,4,i+1)
title(f'{b}')
imshow(example['image']['flux'][i], cmap='gray_r')
axis('off')

dict_keys(['image', 'blobmodel', 'rgb', 'object_mask', 'catalog', 'EBV', 'FLUX_G', 'FLUX_R', 'FLUX_I', 'FLUX_Z', 'FLUX_W1', 'FLUX_W2', 'FLUX_W3', 'FLUX_W4', 'SHAPE_R', 'SHAPE_E1', 'SHAPE_E2', 'object_id'])

Step II:
Architecture & Training

The Architecture Challenge for a Universal Model
Most General
Most Specific
Independent models for every type of observation
Single model capable of processing all types of observations



The Architecture Challenge for a Universal Model
Most General
Most Specific
Independent models for every type of observation
Single model capable of processing all types of observations



Bytes Are All You Need (Horton et al. 2023)

The Architecture Challenge for a Universal Model
Most General
Most Specific
Independent models for every type of observation
Single model capable of processing all types of observations



Bytes Are All You Need (Horton et al. 2023)
AstroCLIP

AstroCLIP
Cross-Modal Pre-Training for Astronomical Foundation Models


Project led by Liam Parker, Francois Lanusse, Leopoldo Sarra, Siavash Golkar, Miles Cranmer
Accepted contribution at the NeurIPS 2023 AI4Science Workshop
Published in the Monthly Notices of Royal Astronomical Society




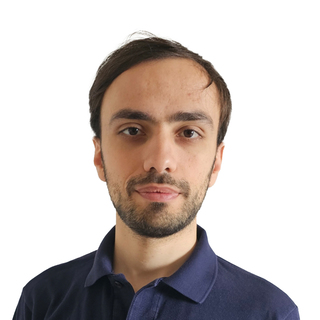
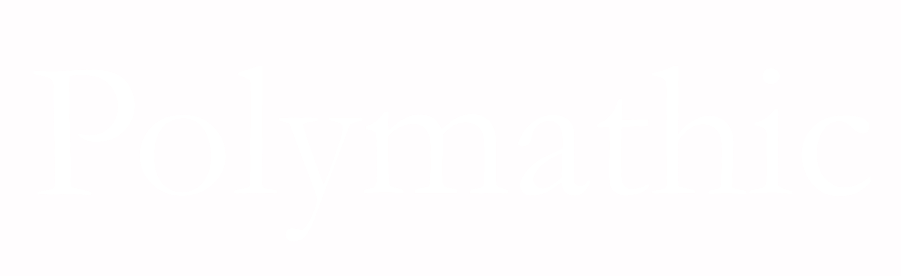
What is CLIP?
Contrastive Language Image Pretraining (CLIP)
(Radford et al. 2021)




The AstroCLIP approach
- We use spectra and multi-band images as our two different views for the same underlying object.
- DESI Legacy Surveys (g,r,z) images, and DESI EDR galaxy spectra.




Cosine similarity search

- Redshift Estimation From Images


Supervised baseline

- Zero-shot prediction
- k-NN regression
- Few-shot prediction
- MLP head trained on top of frozen backbone
Evaluation of the model: Parameter Inference

The Information Point of View
- The InfoNCE loss is a lower bound on the Mutual Information between modalities


Shared physical information about galaxies between images and spectra
=> We are building summary statistics for the physical parameters describing an object in a completely data driven way

The AstroCLIP Model
- For images, we use a ViT-L Transformer, pre-pretrained on 70M images using DiNOv2.
- For spectra, we use a decoder only Transformer working at the level of spectral patches.


DiNOv2 (Oquab et al. 2023) Image Pretraining
- Common practice for SOTA CLIP models is to initially pretrain the image encoder before CLIP alignment
- We adopt the DiNOv2 state of the art Self-Supervised Learning model for the initial large scale training of the model.
- We pretrain the DiNOv2 model on ~70 million postage stamps from DECaLS


PCA of patch features
Dense Semantic Segmentation
Dense Depth Estimation



The Architecture Challenge for a Universal Model
Most General
Most Specific
Independent models for every type of observation
Single model capable of processing all types of observations



Bytes Are All You Need (Horton et al. 2023)
AstroCLIP

The Architecture Challenge for a Universal Model
Most General
Most Specific
Independent models for every type of observation
Single model capable of processing all types of observations



Bytes Are All You Need (Horton et al. 2023)
AION-1

AION-1
AstronomIcal Omnimodal Network

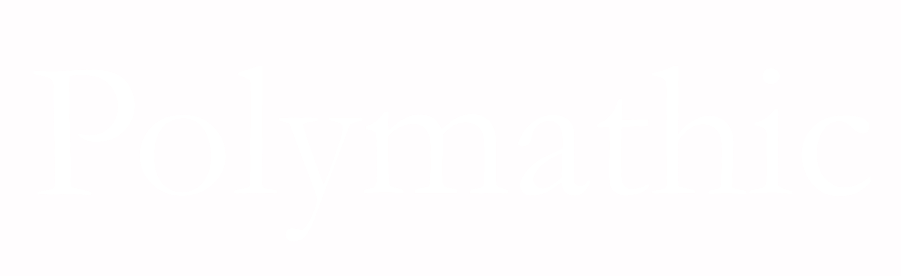
with extensive support from the rest of the team.


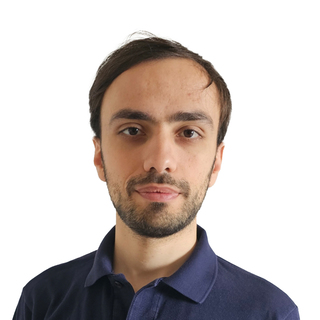







Project led by:
Francois
Lanusse
Liam
Parker
Jeff
Shen
Tom
Hehir
Ollie
Liu
Lucas
Meyer
Leopoldo
Sarra
Sebastian Wagner-Carena
Helen
Qu
Micah
Bowles
What will make Francois happy?
- Single pre-trained model which can operate on any input data type
- I no longer need to worry about what network to use on some data
- I no longer need to worry about what network to use on some data
- Emergent deep understanding of the data, informed by cross-modal information
- A downstream task could be specified with just a few examples

The AION-1 Data Pile


(Blanco Telescope and Dark Energy Camera.
Credit: Reidar Hahn/Fermi National Accelerator Laboratory)





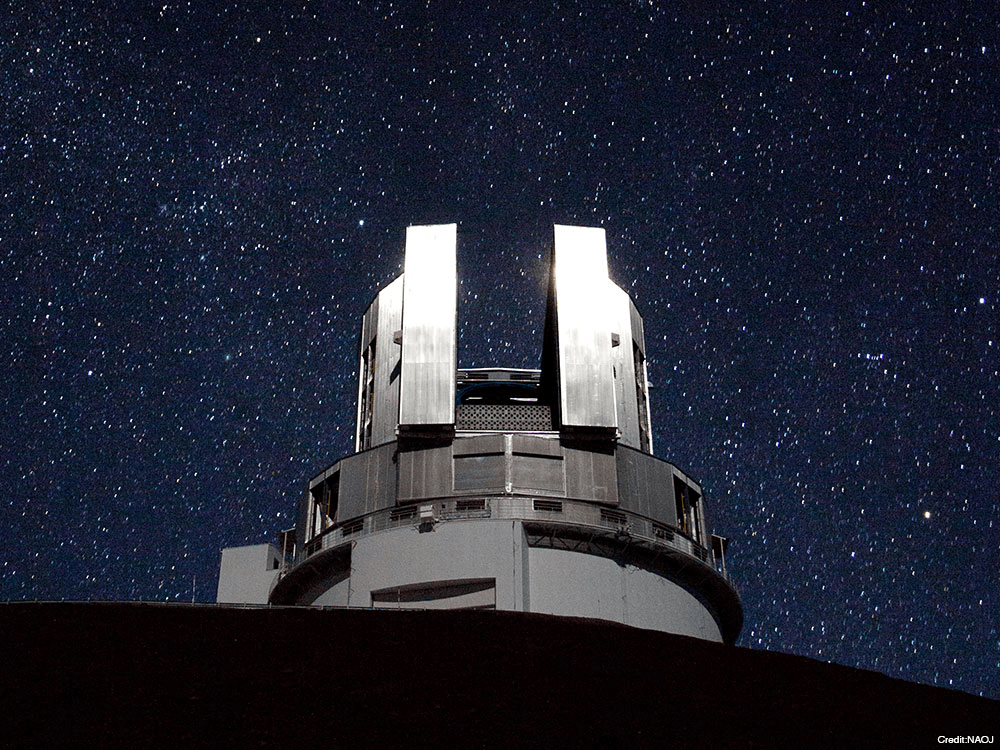
(Subaru Telescope and Hyper Suprime Cam. Credit: NAOJ)




(Dark Energy Spectroscopic Instrument)



(Sloan Digital Sky Survey. Credit: SDSS)


(Gaia Satellite. Credit: ESA/ATG)
Cuts: extended, full color griz, z < 21
Cuts: extended, full color grizy, z < 21
Cuts: parallax / parallax_error > 10
Standardizing all modalities through tokenization

- For each modality category (e.g. image, spectrum) we build dedicated tokenizers
=> Convert from any data to discrete tokens
- For Aion-1, we integrate 39 different modalities (different instruments, different measurements, etc.)


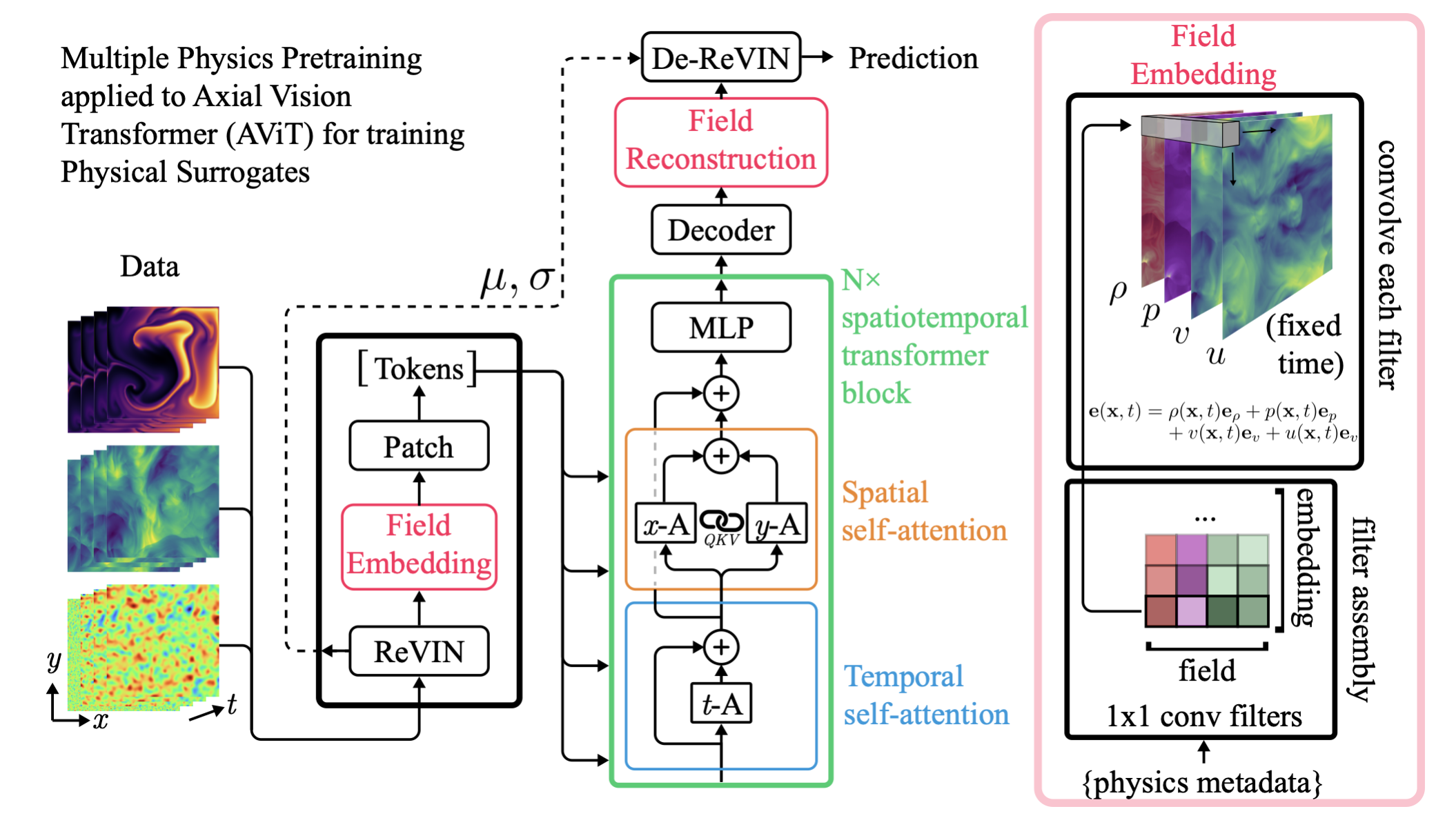
Field Embedding Strategy Developed for
Multiple Physics Pretraining (McCabe et al. 2023)

DES g
DES r
DES i
DES z
HSC g
HSC r
HSC i
HSC z
HSC y


Any-to-Any Modeling with Generative Masked Modeling
- Given standardized and cross-matched dataset, we can feed the data to a large Transformer Encoder Decoder
- Flexible to any combination of input data, can be prompted to generate any output.
- Model is trained by cross-modal generative masked modeling
=> Learns the joint and all conditional distributions of provided modalities:




AION-1 family of models



Models trained as part of the 2024 Jean Zay Grand Challenge
following an extension to a new partition of 1400 H100s
- AION-1 Base: 300 M parameters
- 64 H100s - 1.5 days
- AION-1 Large: 800 M parameters
- 100 H100s - 2.5 days
- AION-1 XLarge: 3B parameters
- 288 H100s - 3.5 days


Example of out-of-the-box capabilities


Survey translation

Spectrum super-resolution

Redshift estimation



Example of emergent multimodal understanding
- Direct association between DESI and HSC was excluded during pretraining
=> This task is out of distribution!


Step III: (Profit !)
Accelerating Downstream Science
Rethinking the way we use Deep Learning
Conventional scientific workflow with deep learning
- Build a large training set of realistic data
- Design a neural network architecture for your data
- Deal with data preprocessing/normalization issues
- Train your network on some GPUs for a day or so
- Apply your network to your problem
- Throw the network away...
=> Because it's completely specific to your data, and to the one task it's trained for.

Conventional researchers @ CMU
Circa 2016



CMU DeepLens (Lanusse et al 2017)

Rethinking the way we use Deep Learning
Foundation Model-based Scientific Workflow
- Build a small training set of realistic data
- Design a neural network architecture for your data
- Deal with data preprocessing/normalization issues
- Adapt a model in a matter of minutes
- Apply your model to your problem
- Throw the network away...
=> Because it's completely specific to your data, and to the one task it's trained for.
Already taken care of
=> Let's discuss embedding-based adaptation

Adaptation of AstroCLIP vector embedding models


Galaxy property estimation given the PROVABGS catalog (Hahn et al. 2023) as training data
of regression
from inquiry import Inquiry
from sklearn.neighbors import KNeighborsRegressor
# [Load training and testing data]
# Compute embeddings through the PolymathicAI API
client = Inquiry()
embeddings = client.embeddings(x_train, 'image-astroclip')
# Build regression model
model = KNeighborsRegressor().fit(embedding, y_train)
# Perform Inference
preds = model.predict(x_test)
- No local GPU needed
- Embeddings can even be pre-computed and included in data releases
Adaptation of AION embeddings


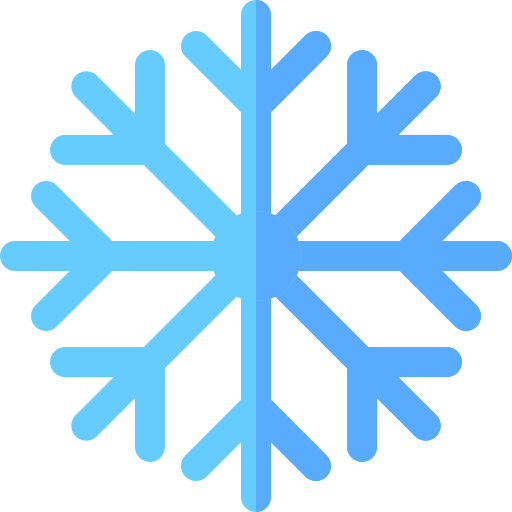
Adaptation at low cost
with simple strategies:
- Mean pooling + linear probing
- Attentive pooling
- Can be used trivially on any input data Aion was trained for
- Flexible to varying number/types of inputs
=> Allows for trivial data fusion

x_train = Tokenize(hsc_images, modality='HSC')
model = FineTunedModel(base='Aion-B',
adaptation='AttentivePooling')
model.fit(x_train, y_train)
y_test = model.predict(x_test)
Morphology classification by Linear Probing



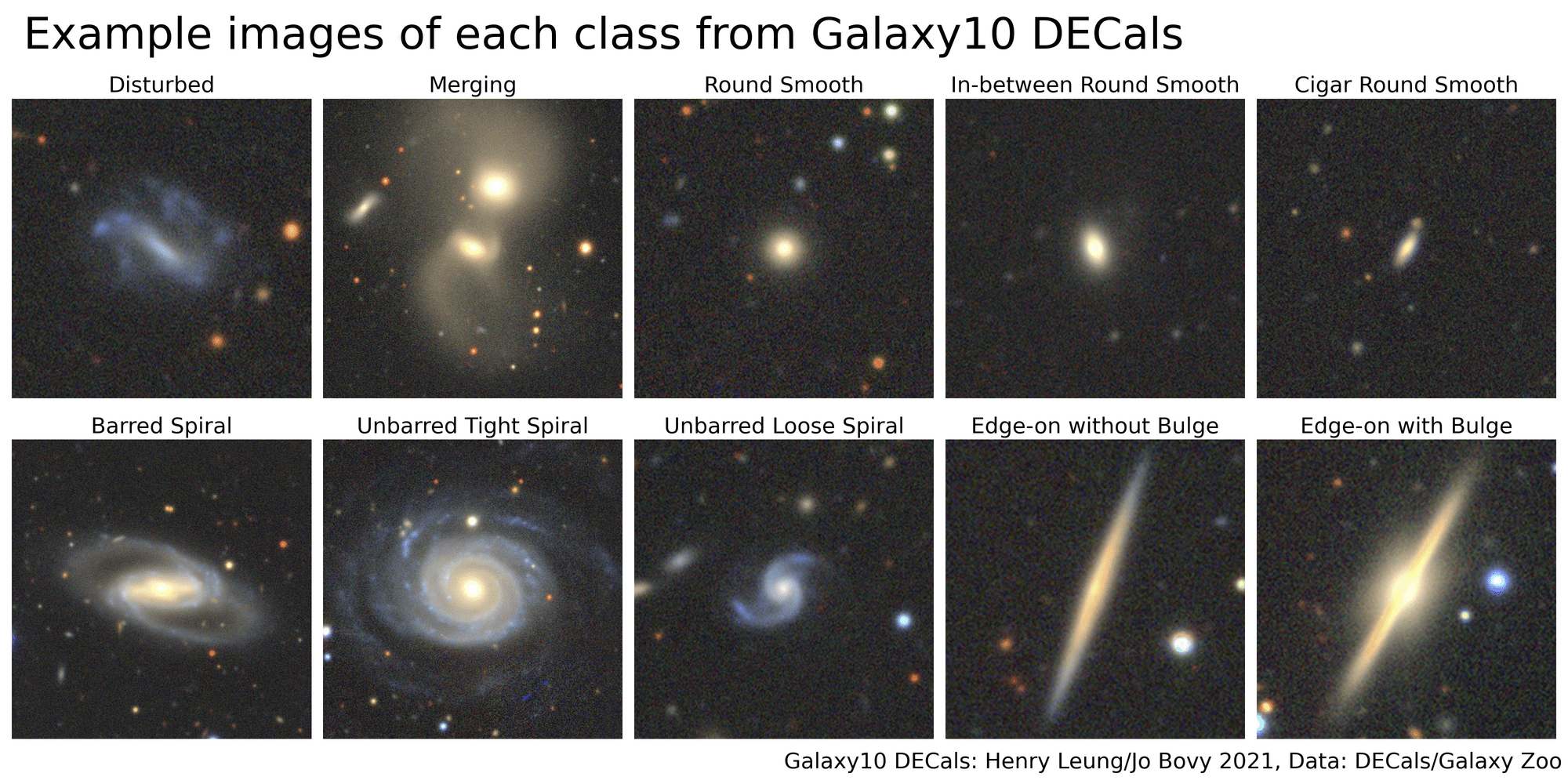

Trained on ->
Eval on ->
Semantic segmentation


Segmenting central bar and spiral arms in galaxy images based on Galaxy Zoo 3D
Physical parameter estimation



Example-based retrieval from mean pooling


Opportunities to engage
-
Beta testers program coming up next week!
=> Slack if you want to be notified
-
We are hiring in Paris!
- Postdoctoral positions (deadline this Friday)
https://aas.org/jobregister/ad/09aadf56
- Postdoctoral positions (deadline this Friday)



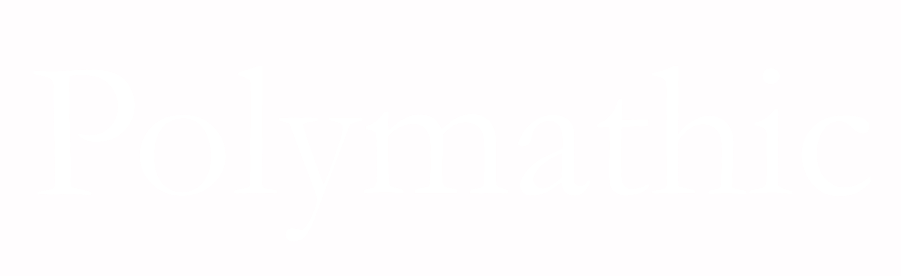
Follow us online!
Thank you for listening!
One Network to Rule Them All
By eiffl
One Network to Rule Them All
LINCC Tech Talk, Feb 2025
- 141