Reproducibility and beyond:
towards sustainable data science
Johannes Köster
Joint Meeting of the German
Research Training Groups
Dagstuhl 2023
dataset
results
Data analysis
"Let me do that by hand..."

dataset
results
dataset
dataset
dataset
dataset
dataset


"Let me do that by hand..."
Data analysis
workflow management
- check computational validity
- apply same to new data
- check methodological validity
- understand what was done
Data analysis
Reproducibility
Transparency
- modify
- extend
Adaptability
scientific workflows
scientific software
scientific workflows
scientific workflows
package management
Software installation is heterogeneous
source("https://bioconductor.org/biocLite.R")
biocLite("DESeq2")
easy_install snakemake
./configure --prefix=/usr/local
make
make install
cp lib/amd64/jli/*.so lib
cp lib/amd64/*.so lib
cp * $PREFIX
cpan -i bioperl
cmake ../../my_project \
-DCMAKE_MODULE_PATH=~/devel/seqan/util/cmake \
-DSEQAN_INCLUDE_PATH=~/devel/seqan/include
make
make install
apt-get install bwa
yum install python-h5py
install.packages("matrixpls")
Package management with Conda/Mamba
package:
name: seqtk
version: 1.2
source:
fn: v1.2.tar.gz
url: https://github.com/lh3/seqtk/archive/v1.2.tar.gz
requirements:
build:
- gcc
- zlib
run:
- zlib
about:
home: https://github.com/lh3/seqtk
license: MIT License
summary: Seqtk is a fast and lightweight tool for processing sequences
test:
commands:
- seqtk seq
#!/bin/bash
export C_INCLUDE_PATH=${PREFIX}/include
export LIBRARY_PATH=${PREFIX}/lib
make all
mkdir -p $PREFIX/bin
cp seqtk $PREFIX/bin
- source or binary
- recipe and build script
- package
Easy installation and maintenance:
no admin rights needed
mamba env create -f myenv.yaml -n myenv
Isolated environments:
channels:
- conda-forge
- nodefaults
dependencies:
- pandas ==0.20.3
- statsmodels ==0.8.0
- r-dplyr ==0.7.0
- r-base ==3.4.1
- python ==3.6.0
Package management with Conda/Mamba
- >8000 bioinformatics related packages (C, C++, Python, R, Perl, ...)
- >140 million downloads
- >650 contributors

Alternatives
- Nix
- Spack
- Containers
scientific workflows
workflow management




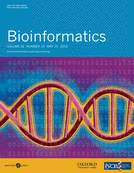













Snakemake:
>700k downloads since 2015
>2000 citations
>10 citations per week in 2022
Workflow management

dataset
results
dataset
dataset
dataset
dataset
dataset
Define workflows
in terms of rules
Define workflows
in terms of rules
rule mytask:
input:
"path/to/{dataset}.txt"
output:
"result/{dataset}.txt"
script:
"scripts/myscript.R"
rule myfiltration:
input:
"result/{dataset}.txt"
output:
"result/{dataset}.filtered.txt"
shell:
"mycommand {input} > {output}"
rule aggregate:
input:
"results/dataset1.filtered.txt",
"results/dataset2.filtered.txt"
output:
"plots/myplot.pdf"
script:
"scripts/myplot.R"
Define workflows
in terms of rules
Define workflows
in terms of rules
rule mytask:
input:
"data/{sample}.txt"
output:
"result/{sample}.txt"
conda:
"software-envs/some-tool.yaml"
shell:
"some-tool {input} > {output}"
rule name
how to create output from input
declare software environment
Boilerplate-free integration of scripts
rule mytask:
input:
"data/{sample}.txt"
output:
"result/{sample}.txt"
script:
"scripts/myscript.py"
reusable scripts:
- Python
- R
- Julia
- Rust
import pandas as pd
data = pd.read_table(snakemake.input[0])
data = data.sort_values("id")
data.to_csv(snakemake.output[0], sep="\t")
Python:
data <- read.table(snakemake@input[[1]])
data <- data[order(data$id),]
write.table(data, file = snakemake@output[[1]])
Boilerplate-free integration of scripts
R:
import polar as pl
pl.read_csv(&snakemake.input[0])
.sort()
.to_csv(&snakemake.output[0])
Rust:
Directed acyclic graph (DAG) of jobs
- MILP based scheduler
- graph partitioning
+
scale to any platform without adapting workflow
Alternatives
- Nextflow
- Galaxy
- Airflow
- KNIME
- Hadoop
- ...
Publication reproducibility
"The Reproducibility Project: Cancer Biology has so far managed to replicate the main findings in only 5 of 17 highly cited articles, and a replication of 21 social-sciences articles in Science and Nature had a success rate of between 57 and 67%."
Amaral & Neves, Nature 2021
Problem:
- rarely a topic in journal guidelines
- how to review?
- delegate to community (review or reproduction itself)?
- what could be incentives for reproducing the work of others?

Example Nature:
Publication reproducibility
Publication transparency
Problem:
How to connect results (i.e. figures and tables) with code, parameters, used software?
Snakemake reports
scientific software
The crisis of scientific software
- incomplete documentation
- inefficient programming
- incomplete testing
- little to no maintenance
Example
ISMB 2016 "Wall of Shame"

Of 47 open-access publications, ...
Reasons
- temporary employments
- time pressure
- code itself, software quality not a widely recognized scientific output
- PIs occupied with other duties
But
Many resources are available, we just have to use them!
Style guides
def make_complex(*args):
x, y = args
return dict(**locals())
def make_complex(x, y):
return {'x': x, 'y': y}
vs
Automatic code analysis tools:
- linter: e.g. Ruff (https://github.com/charliermarsh/ruff)
- auto-formatter: e.g. Black (https://github.com/psf/black)
Code duplication and complexity
Instead:
- inheritance
- delegation
- data classes
- singletons
Automatic code analysis tools:
e.g. Lizard (https://github.com/terryyin/lizard)
Reinventing wheels
data = dict()
with open("somefile.tsv") as f:
for line in f:
line = line.split("\t")
key, fields = line[0], line[1:]
data[key] = [float(field) for field in fields]
import pandas as pd
data = pd.read_table("sometable.tsv", index_col=0)
vs
The choice of the programming language
Goal:
efficient, error-free, readable code
Classical compiled languages (C, C++, ...):
- very efficient
- complex and error prone (memory management, thread safety, ...)
Wikipedia
The choice of the programming language
Scripting languages (Python, Ruby, ...):
- slow
- no type safety
- only runtime errors

https://attractivechaos.github.io/plb/
The choice of the programming language
Rust
- simplified, C++-like syntax
- automatic type inference
- elements from scripting languages
- very strict compiler with guaranteed memory and thread safety
- elected "most loved programming language" on stack overflow since 8 years
Result:
- Development time shifts from debugging to compiling.
- massively reduced maintenance effort

Continuous integration
Github Actions
jobs:
Formatting:
runs-on: ubuntu-latest
steps:
- uses: actions/checkout@v2
with:
# Full git history is needed to get a proper
# list of changed files within `super-linter`
fetch-depth: 0
- name: Formatting
uses: github/super-linter@v4
env:
VALIDATE_ALL_CODEBASE: false
DEFAULT_BRANCH: master
GITHUB_TOKEN: ${{ secrets.GITHUB_TOKEN }}
VALIDATE_SNAKEMAKE_SNAKEFMT: true
Linting:
runs-on: ubuntu-latest
steps:
- uses: actions/checkout@v2
- name: Lint workflow
uses: snakemake/snakemake-github-action@v1.24.0
with:
directory: .
snakefile: workflow/Snakefile
args: "--lint"
Testing:
runs-on: ubuntu-latest
needs:
- Linting
- Formatting
steps:
- uses: actions/checkout@v2
- name: Test workflow (local FASTQs)
uses: snakemake/snakemake-github-action@v1
with:
directory: .test
snakefile: workflow/Snakefile
args: "--configfile .test/config-simple/config.yaml --use-conda"

Conclusion
reproducibility
+ transparency
+ adaptability
= sustained value for authors and community
a long way to go
but the tools are there
It has never been easier!
deck
By Johannes Köster
deck
Keynote at Dagstuhl 2023
- 518