Reproducible, transparent, and adaptable data analysis with Snakemake
Johannes Köster
2024

dataset
results
Data analysis
"Let me do that by hand..."

dataset
results
dataset
dataset
dataset
dataset
dataset


"Let me do that by hand..."
Data analysis
- check computational validity
- apply same analysis to new data
- check methodological validity
- understand analysis
Data analysis
Reproducibility
Transparency
- modify analysis
- extend analysis
Adaptability
>1 million downloads since 2015




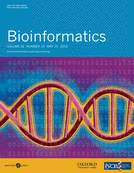













>2700 citations
>11 citations per week in 2023

- automation
- scalability
- portability
- readability
- documentation
- traceability
Data analysis
Reproducibility
Transparency
- readability
- portability
- scalability
Adaptability
Data analysis
- automation
- scalability
- portability
- readability
- documentation
- traceability
Reproducibility
Transparency
- readability
- portability
- scalability
Adaptability
dataset
results
dataset
dataset
dataset
dataset
dataset
Define workflows
in terms of rules
Define workflows
in terms of rules
rule mytask:
input:
"path/to/{dataset}.txt"
output:
"result/{dataset}.txt"
script:
"scripts/myscript.R"
rule myfiltration:
input:
"result/{dataset}.txt"
output:
"result/{dataset}.filtered.txt"
shell:
"mycommand {input} > {output}"
rule aggregate:
input:
"results/dataset1.filtered.txt",
"results/dataset2.filtered.txt"
output:
"plots/myplot.pdf"
script:
"scripts/myplot.R"
Define workflows
in terms of rules
Define workflows
in terms of rules
rule mytask:
input:
"data/{sample}.txt"
output:
"result/{sample}.txt"
shell:
"some-tool {input} > {output}"
rule name
how to create output from input
define
- input
- output
- log files
- parameters
- resources
rule mytask:
input:
"path/to/{dataset}.txt"
output:
"result/{dataset}.txt"
script:
"scripts/myscript.R"
rule myfiltration:
input:
"result/{dataset}.txt"
output:
"result/{dataset}.filtered.txt"
shell:
"mycommand {input} > {output}"
rule aggregate:
input:
"results/dataset1.filtered.txt",
"results/dataset2.filtered.txt"
output:
"plots/myplot.pdf"
script:
"scripts/myplot.R"
Automatic inference of DAG of jobs
Boilerplate-free integration of scripts
rule mytask:
input:
"data/{sample}.txt"
output:
"result/{sample}.txt"
script:
"scripts/myscript.py"
reusable scripts:
- Python
- R
- Julia
- Rust
- Bash
import pandas as pd
data = pd.read_table(snakemake.input[0])
data = data.sort_values("id")
data.to_csv(snakemake.output[0], sep="\t")
Python:
data <- read.table(snakemake@input[[1]])
data <- data[order(data$id),]
write.table(data, file = snakemake@output[[1]])
Boilerplate-free integration of scripts
R:
import polar as pl
pl.read_csv(&snakemake.input[0])
.sort()
.to_csv(&snakemake.output[0])
Rust:
Jupyter notebook integration
rule mytask:
input:
"data/{sample}.txt"
output:
"result/{sample}.txt"
notebook:
"notebooks/mynotebook.ipynb"
- Integrated interactive edit mode.
- Automatic generalization for reuse in other jobs.
Reusable wrappers
rule map_reads:
input:
"{sample}.bam"
output:
"{sample}.sorted.bam"
wrapper:
"0.22.0/bio/samtools/sort"
reuseable wrappers from central repository
Reusable wrappers
Output handling
rule mytask:
input:
"data/{sample}.txt"
output:
temp("result/{sample}.txt")
shell:
"some-tool {input} > {output}"
Output handling
rule mytask:
input:
"data/{sample}.txt"
output:
protected("result/{sample}.txt")
shell:
"some-tool {input} > {output}"
Output handling
rule mytask:
input:
"data/{sample}.txt"
output:
pipe("result/{sample}.txt")
shell:
"some-tool {input} > {output}"
Using and combining workflows
configfile: "config/config.yaml"
module some_workflow:
snakefile:
github("some-org/some-workflow", path="workflow/Snakefile", tag="v1.17.0")
config:
config["some-workflow"]
use rule * from some_workflow
# easily extend the workflow
rule some_plot:
input:
"results/some-table.h5"
output:
"results/plots/some-plot.pdf"
notebook:
"notebooks/some-plot.py.ipynb"
module some_other_workflow:
snakefile:
github("some-org/some-other-workflow", path="workflow/Snakefile", tag="v2.5.0")
config:
config["some-other-workflow"]
use rule * from some_other_workflow
use rule process_xy from some_other_workflow with:
params:
some_threshold=0.3
Data analysis
- automation
- scalability
- portability
- readability
- documentation
- traceability
Reproducibility
Transparency
- readability
- portability
- scalability
Adaptability
job selection
job resource usage
free resources
job temp file consumption
temp file lifetime fraction
job priority
job thread usage
Scheduling
temp file size
temp file deletion
DAG partitioning
--groups a=g1 b=g1
--groups a=g1 b=g1 --group-components g1=2
--groups a=g1 b=g1 --group-components g1=5
Scalable to any platform



workstation
compute server
cluster






grid computing
cloud computing

Data analysis
- automation
- scalability
- portability
- readability
- documentation
- traceability
Reproducibility
Transparency
- readability
- portability
- scalability
Adaptability
rule mytask:
input:
"path/to/{dataset}.txt"
output:
"result/{dataset}.txt"
conda:
"envs/some-tool.yaml"
shell:
"some-tool {input} > {output}"
Conda integration
channels:
- conda-forge
dependencies:
- some-tool =2.3.1
- some-lib =1.1.2
Container integration
rule mytask:
input:
"path/to/{dataset}.txt"
output:
"result/{dataset}.txt"
container:
"docker://biocontainers/some-tool#2.3.1"
shell:
"some-tool {input} > {output}"
Containerization
containerized:
"docker://username/myworkflow:1.0.0"
rule mytask:
input:
"path/to/{dataset}.txt"
output:
"result/{dataset}.txt"
conda:
"envs/some-tool.yaml"
shell:
"some-tool {input} > {output}"
snakemake --containerize > Dockerfile
Data analysis
- automation
- scalability
- portability
- readability
- documentation
- traceability
Reproducibility
Transparency
- readability
- portability
- scalability
Adaptability
Self-contained HTML reports
Many more features
- dynamic DAG rewiring
- service jobs (providing sockets, loading databases, or ramdisks)
- semantic helper functions for minimizing boilerplate code
- fallible rules
- caching of shared results across workflows
- transparent handling of remote storage
- interoperability (CWL tool wrappers, integration of Nextflow workflows)
Extensible architecture
Extensible architecture
Snakemake workflow catalog
Conclusion
Snakemake covers all aspects of fully reproducible, transparent, and adaptable data analysis, offering
- maximum readability
- ad-hoc integration with scripting and high performance languages
- an extensible architecture
- a plethora of advanced features
https://snakemake.github.io
Eager to chat and learn about use cases, issues, challenges!
Available today, tomorrow, Friday morning

Introduction to Snakemake
By Johannes Köster
Introduction to Snakemake
Introduction to Snakemake @ CERN
- 133