how to use HOMOTOpic maps to improve Quantum optimization Approximation Algorithms
Institute of Theoretical and Applied Informatics, Polish Academy of Sciences
Ludmila Botelho
Quantum computing devices
Noisy
Intermediate-
Scale
Quantum computing
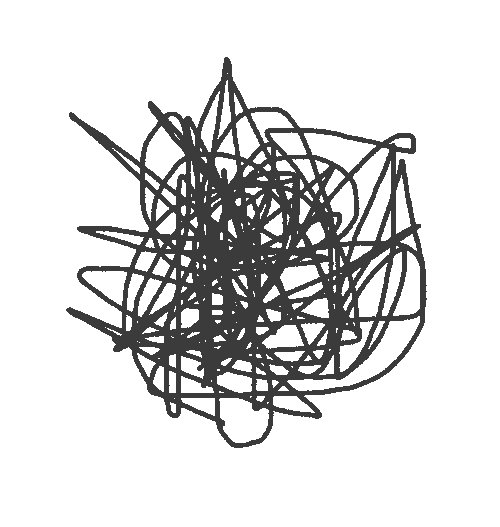
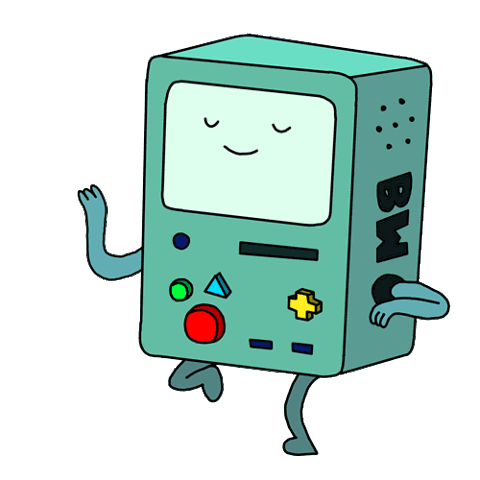
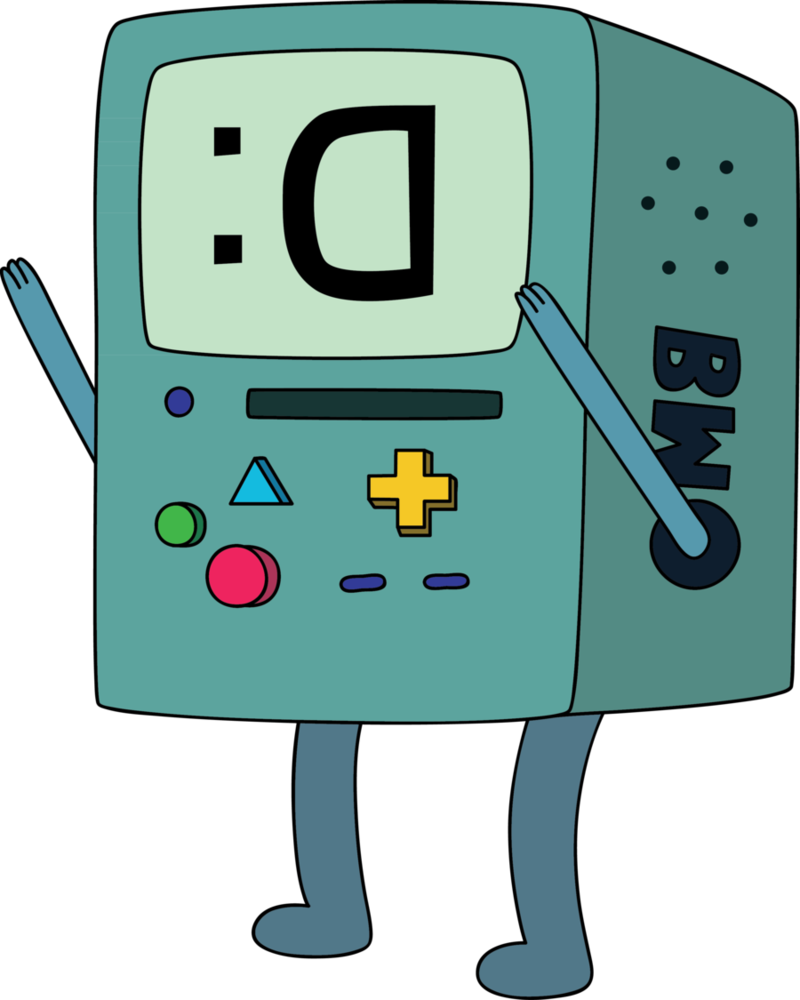
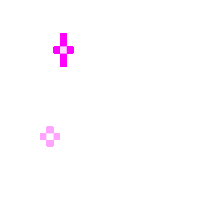
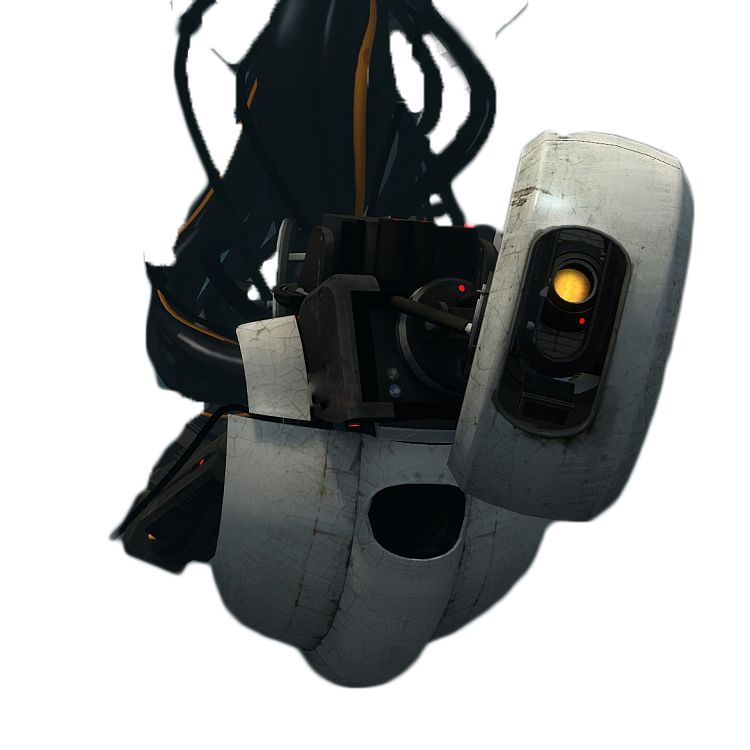
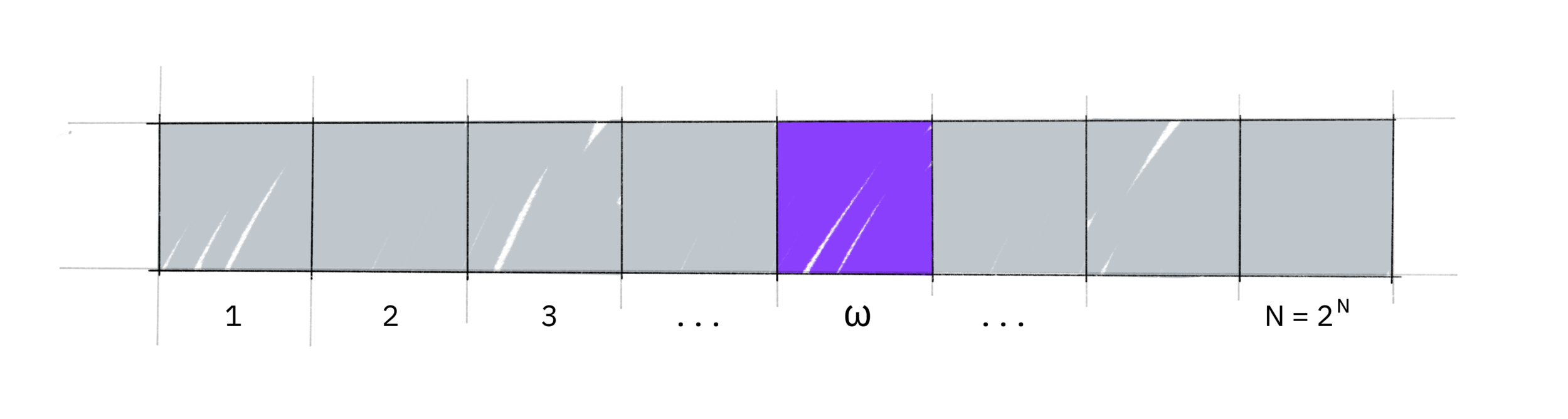
Where is ω?
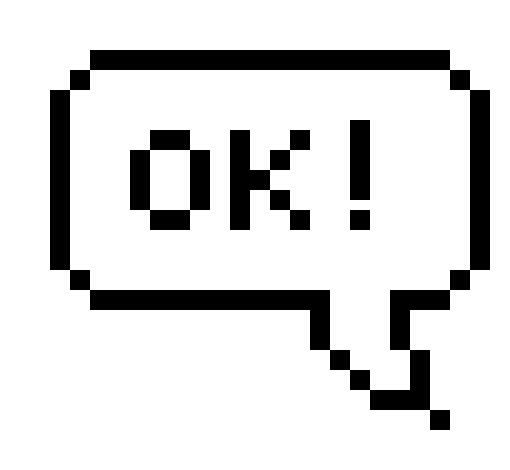
N
Prime factors of
?
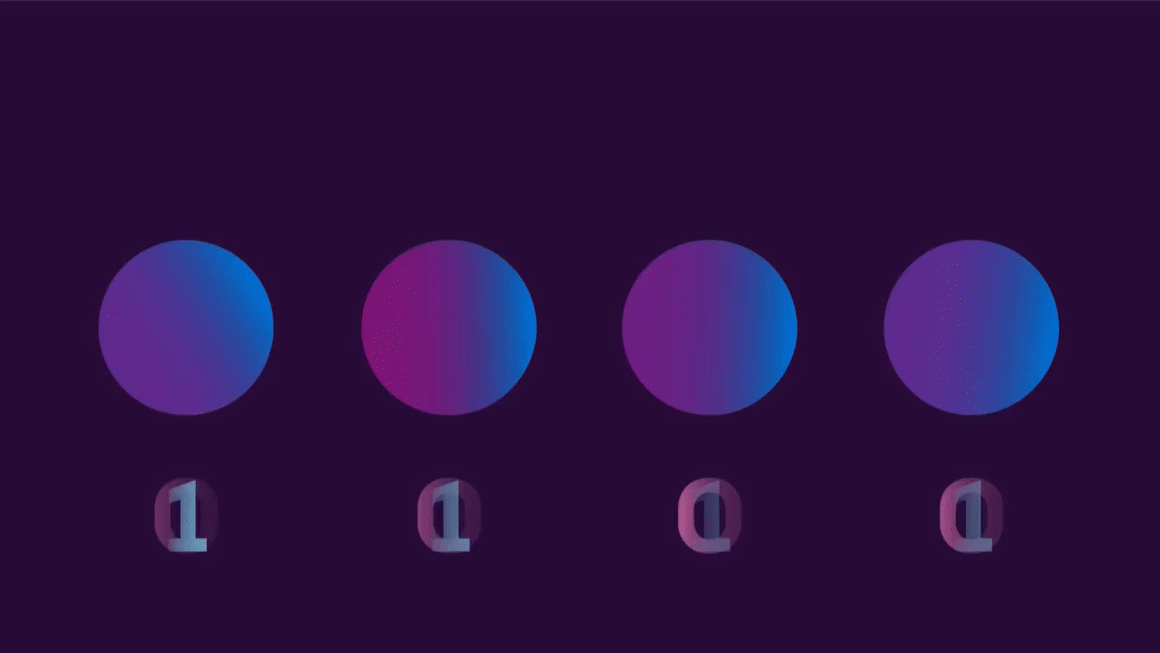
What can we do?
Hybrid solution
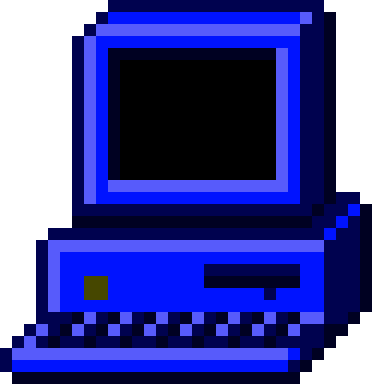

What can we do?
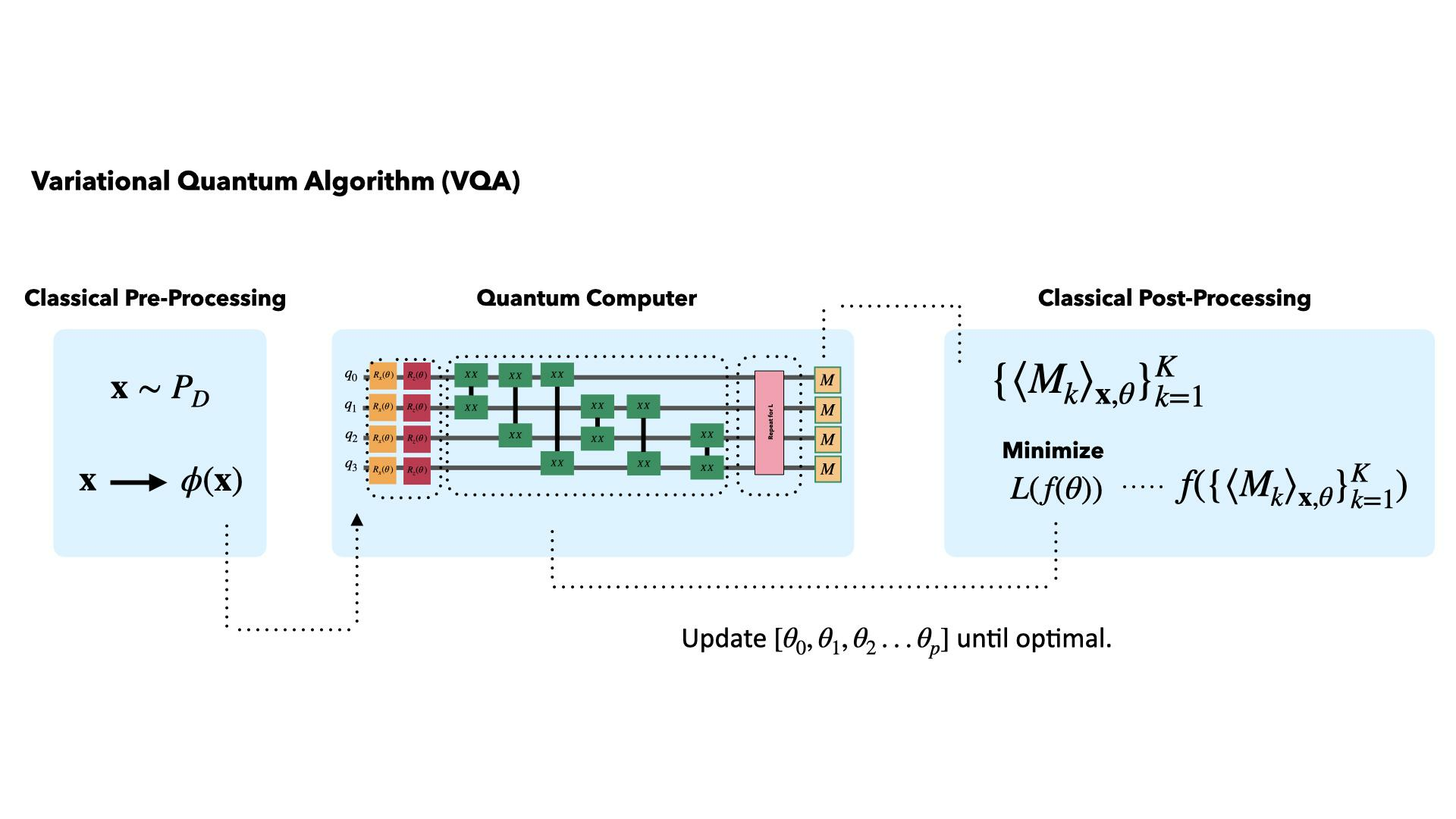
What can we do?
- Quantum Approximate Optimization Algorithm (QAOA)
Ground state = optimal solution
Hobj=−i>j∑JijZiZj−i∑hiZi,
H_{\text{obj}} = - \sum_{i > j} J_{ij}Z_i Z_j - \sum _i h_iZ_i,
Hmix=i∑Xi,
H_{\text{mix}} = \sum _i X_i,
∣γ,β⟩=i=1∏Lexp(−iβjHmix)exp(−iγjHobj)∣+⟩⊗N,
|\vec{\gamma}, \vec{\beta}\rangle=\prod_{i=1}^{L}\exp\left(-\mathrm{i}\beta_{j}H_{\mathrm{mix}}\right)\exp\left(-\mathrm{i}\gamma_{j}H_{\mathrm{obj}}\right)|+\rangle^{\otimes N},
E(γ,β)=⟨γ,β∣Hobj∣γ,β⟩.
E(\vec{\gamma},\vec{\beta})\,=\,\langle\vec{\gamma},\vec{\beta}|H_{\text{obj}}|\vec{\gamma},\vec{\beta}\rangle.
What can we do?
- QAOA based on Adiabatic Quantum Computing
T≫Δ−2s∈[0,1]max[∂t∂H(s)]2
T\gg\Delta^{-2}\max_{s\in[0,1]}\left\|\left[\frac{\partial H(s)}{\partial t}\right]^2\right\|
Adiabatic theorem
H(s)=(1−s)Hmix+sHobj,
H(s) = (1-s)H_{\text{mix}} + sH_{\text{obj}},
Interpolation between Hamiltonians
Energy landscape
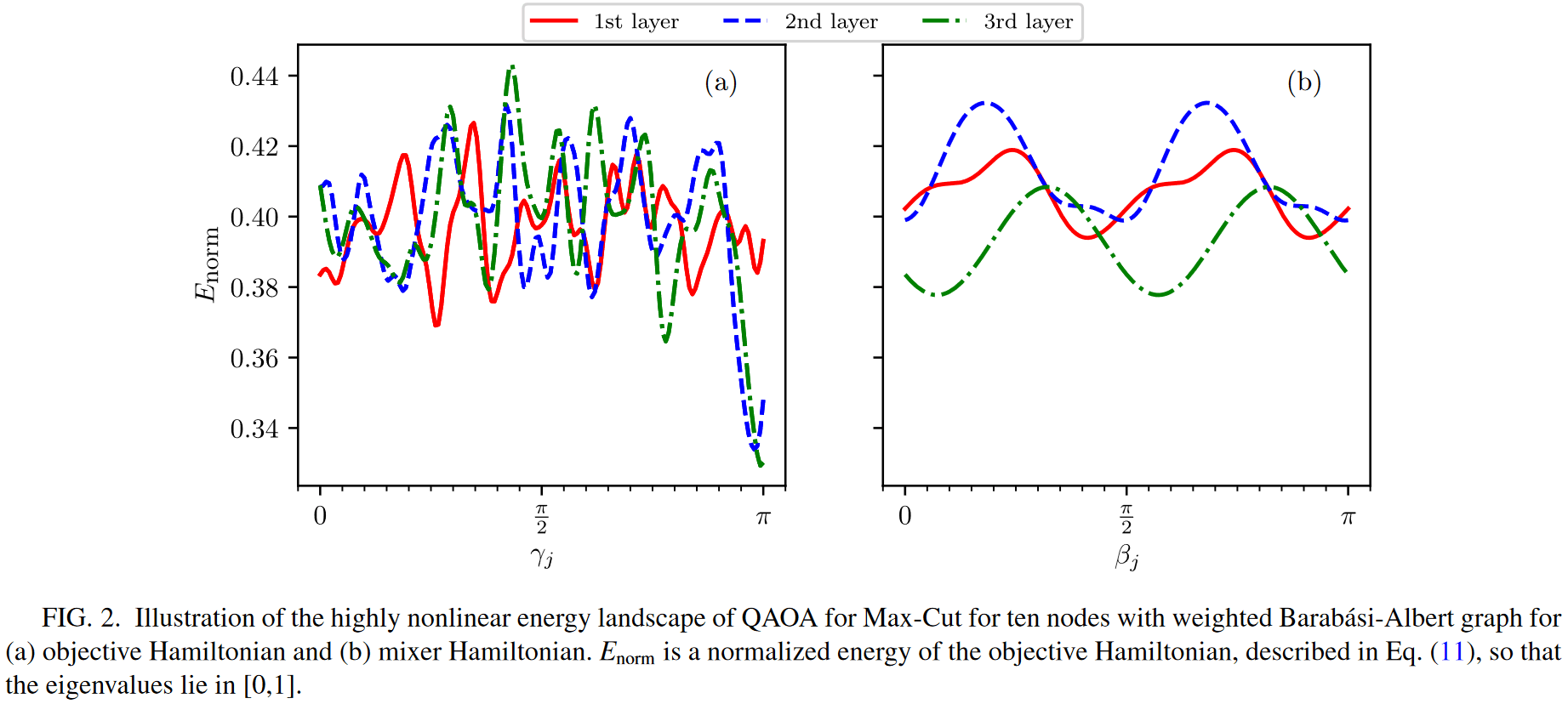
C+∑Ai,jcos(θ(Ei−Ej)+Bi,j),
C+\sum A_{i,j}\cos(\theta(E_i-E_j)+B_{i,j}),
Text
Homotopic map
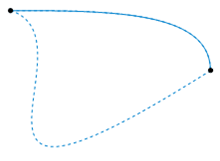
Hmix
H_{\text{mix}}
g2
g_2
F(α,x)=g1(α)ftarg(x)+g2(α)finit(x)
{\mathcal{F}}(\alpha,x)=g_{1}(\alpha)f_{\mathrm{targ}}(x)+g_{2}(\alpha)f_{\mathrm{init}}(x)
g1(0)=0,g1(1)=1,g2(0)=1,g2(1)=0.
\begin{array}{llllllllll}
{{g_1(0)=0,}} & {{g_2(0)=1,}} \\
{{g_1(1)=1,}} & {{g_2(1)=0.}}
\end{array}
g1
g_1
Hobj
H_{\text{obj}}
Hamiltonian-Oriented Homotopy QAOA
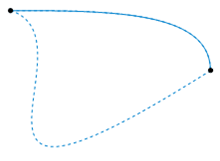
Hmix
H_{\text{mix}}
Hobj
H_{\text{obj}}
H(α)=g1(α)Hmix+g2(α)Hobj,0≤α≤1.
H(\alpha)=g_{1}(\alpha)H_{\mathrm{mix}}+g_{2}(\alpha)H_{\mathrm{obj}},\quad0\leq\alpha\leq1.
Eα(γ,β)=⟨γ,β∣H(α)∣γ,β⟩,
E_{\alpha}(\vec{\gamma},\vec{\beta})\,=\,\langle\vec{\gamma},\vec{\beta}|H(\alpha)|\vec{\gamma},\vec{\beta}\rangle,
g1(α)=1−α,g2(α)=α,
g_{1}(\alpha) = 1 -\alpha, \quad g_{2}(\alpha) = \alpha,
Hamiltonian-Oriented Homotopy QAOA
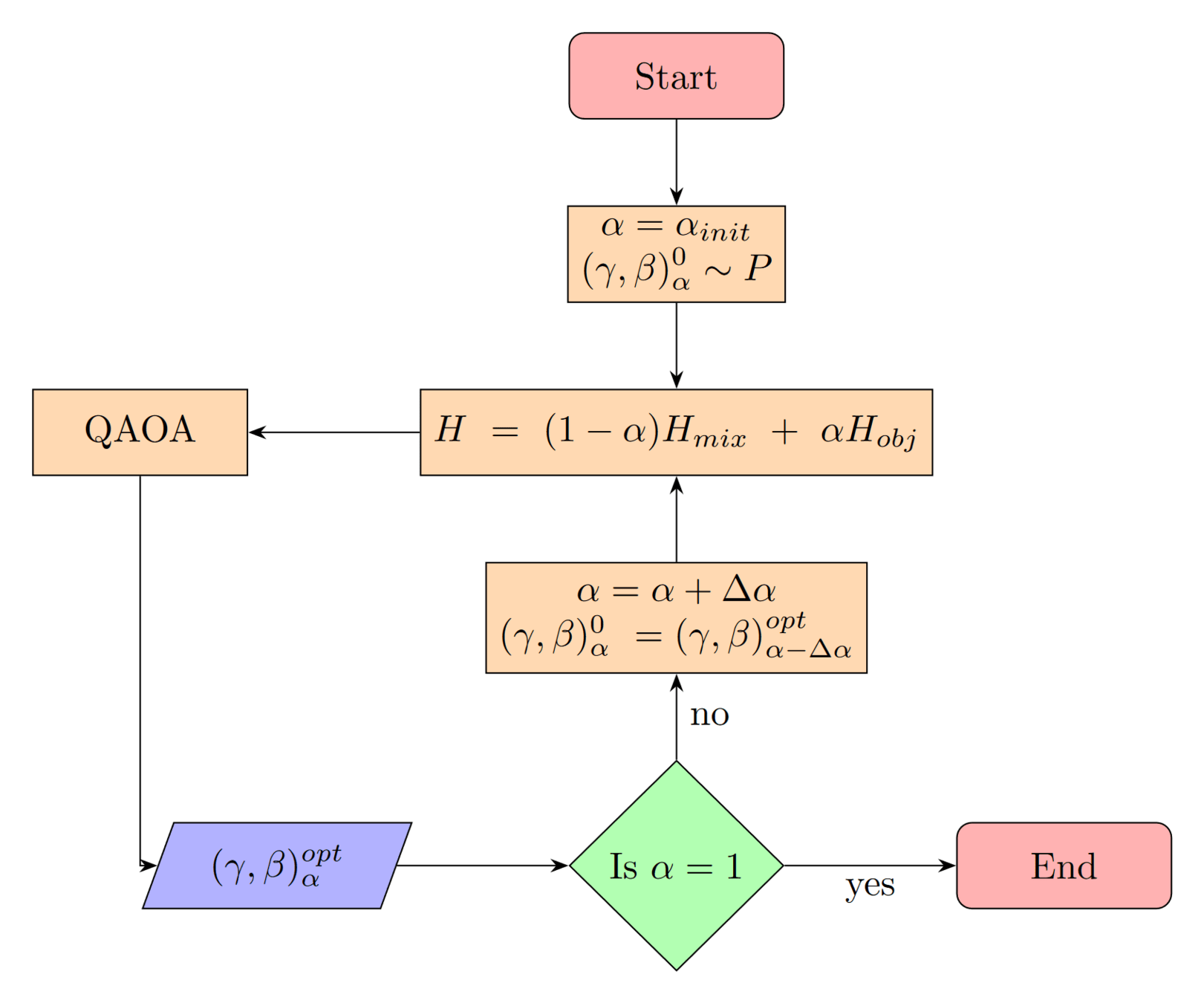
Initialization strategy
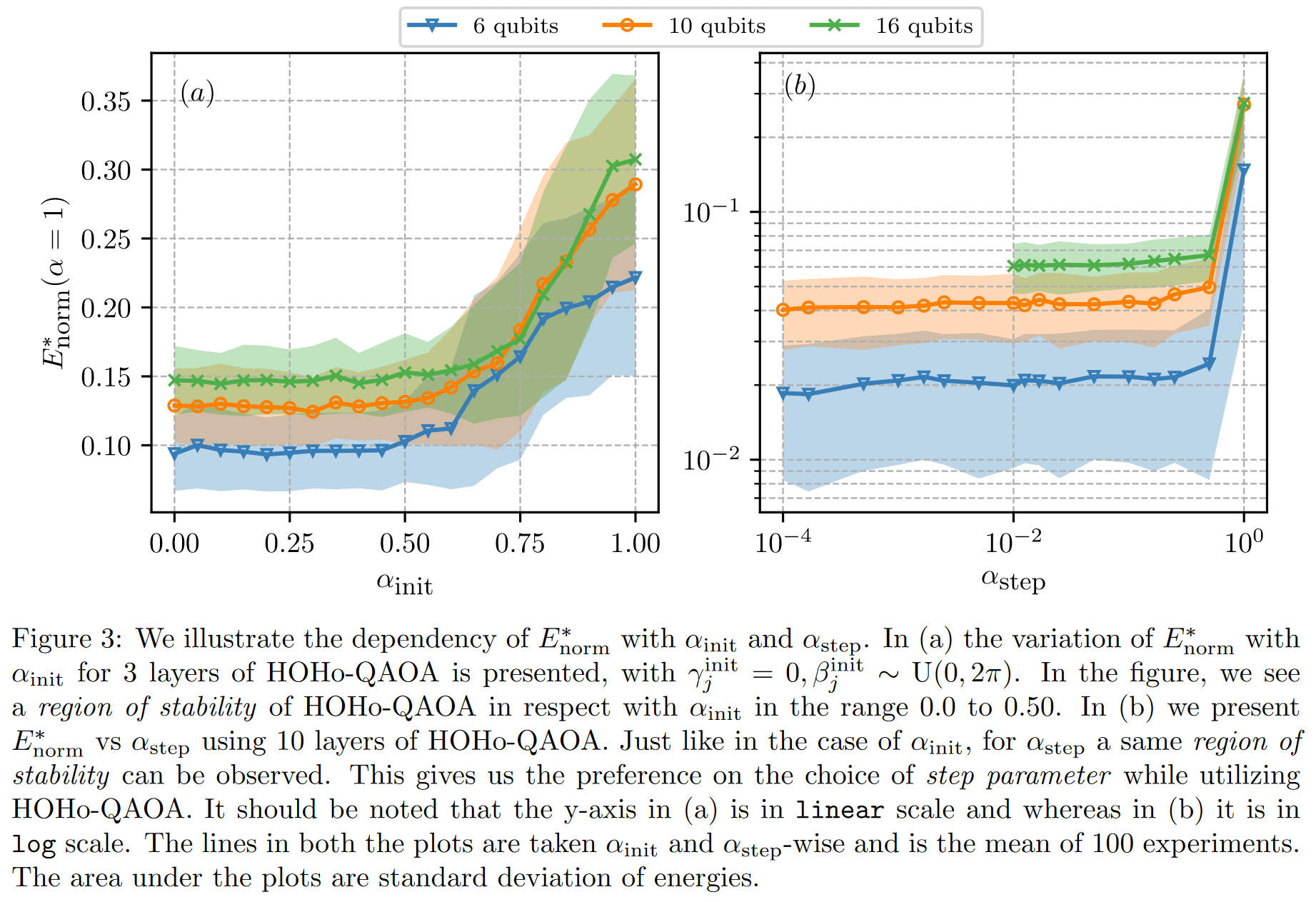
Results
Performance
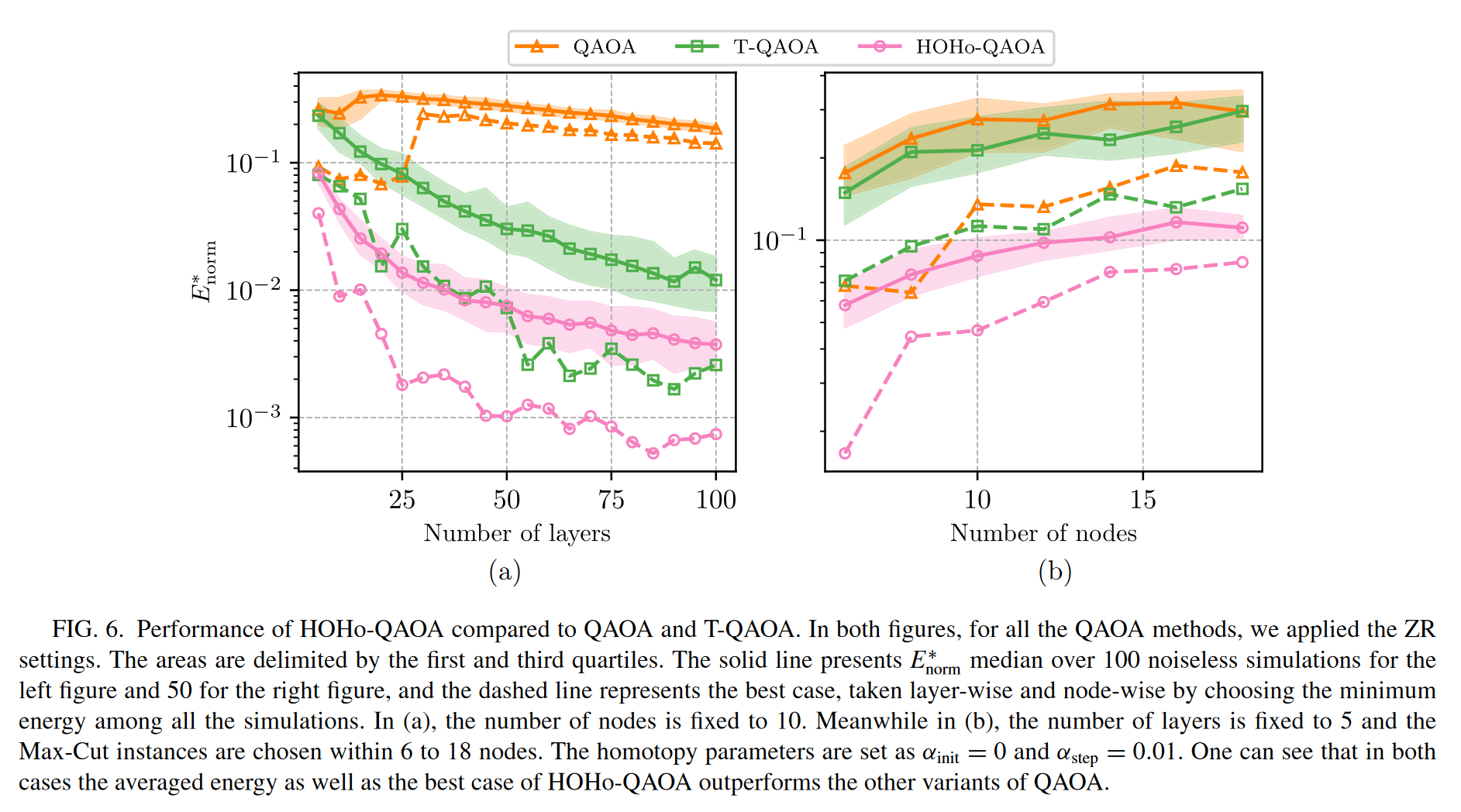
Conclusions
- The method does not increase the impact of the noise
- We numerically confirm that our method outperforms state-of-the-art approaches
- Our approach is well-motivated for nonlinear optimized functions
Thank you!
Dziękuję!
Obrigada!
धन्यवाद!
Teşekkür ederim!
Köszönöm!
ধন্যবাদ !
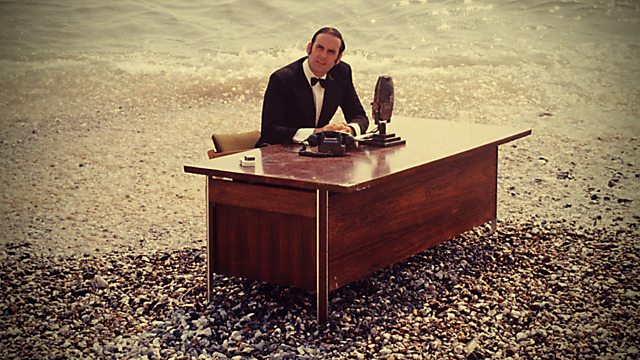
And Now for Something Completely Different
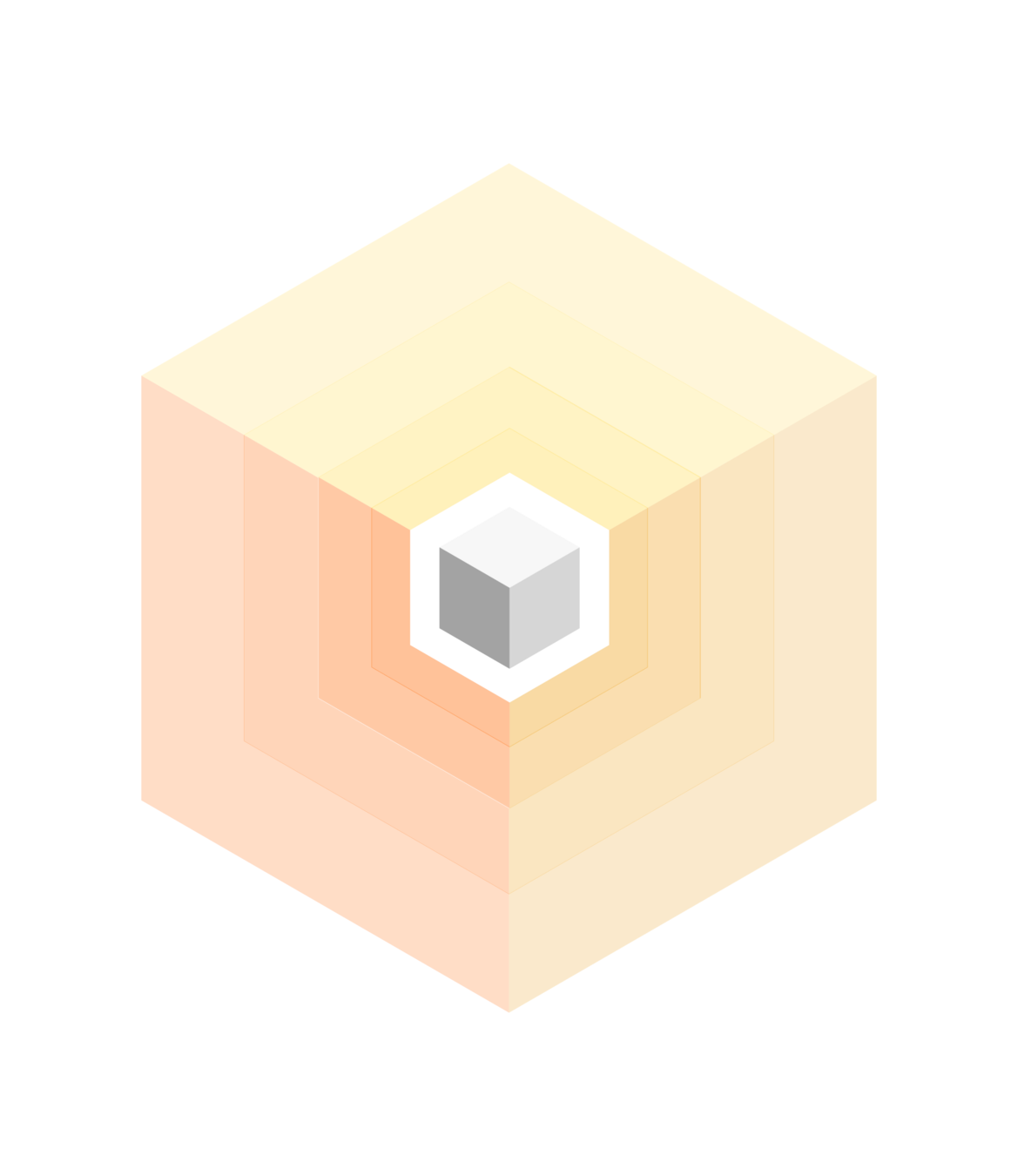
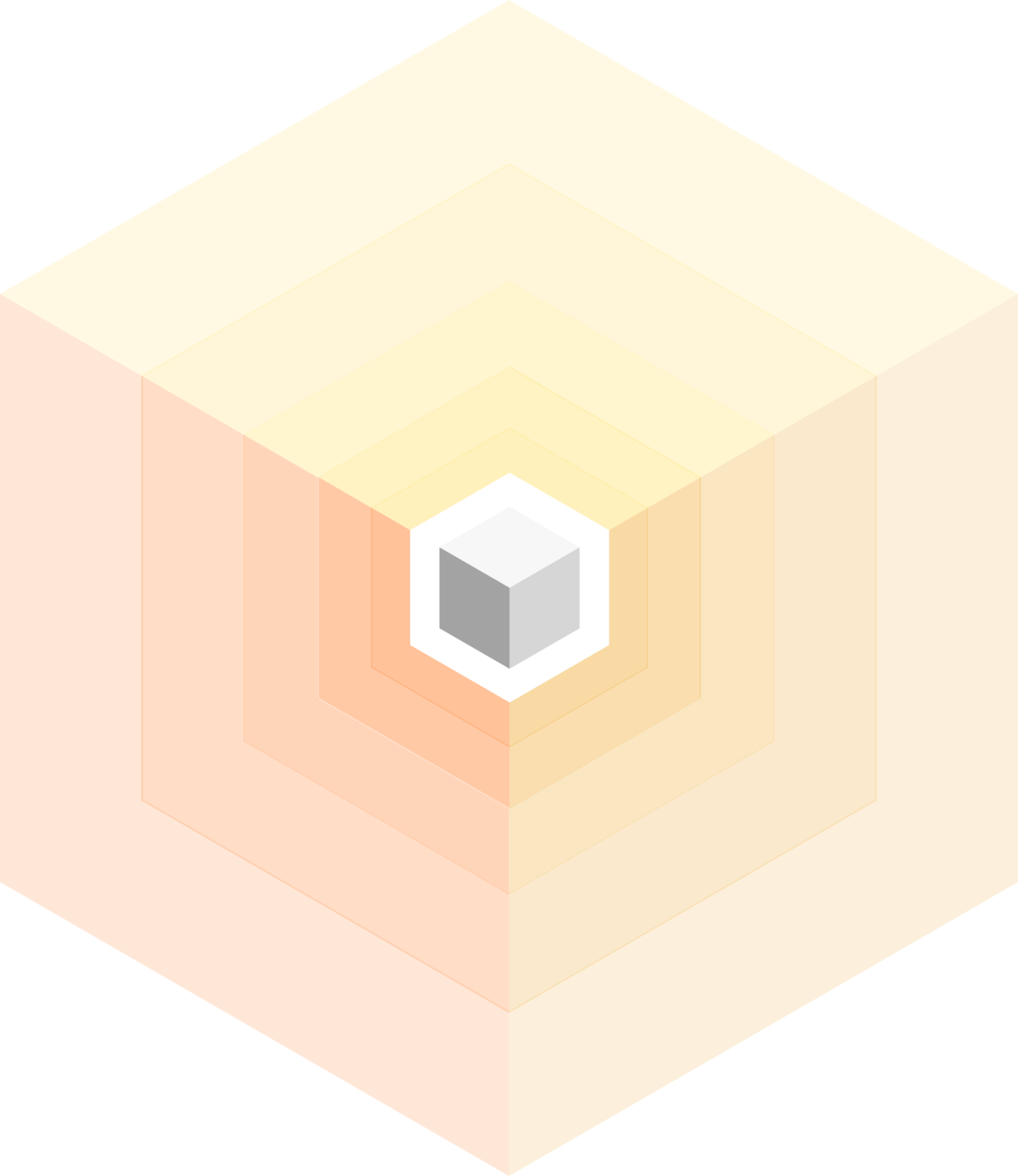
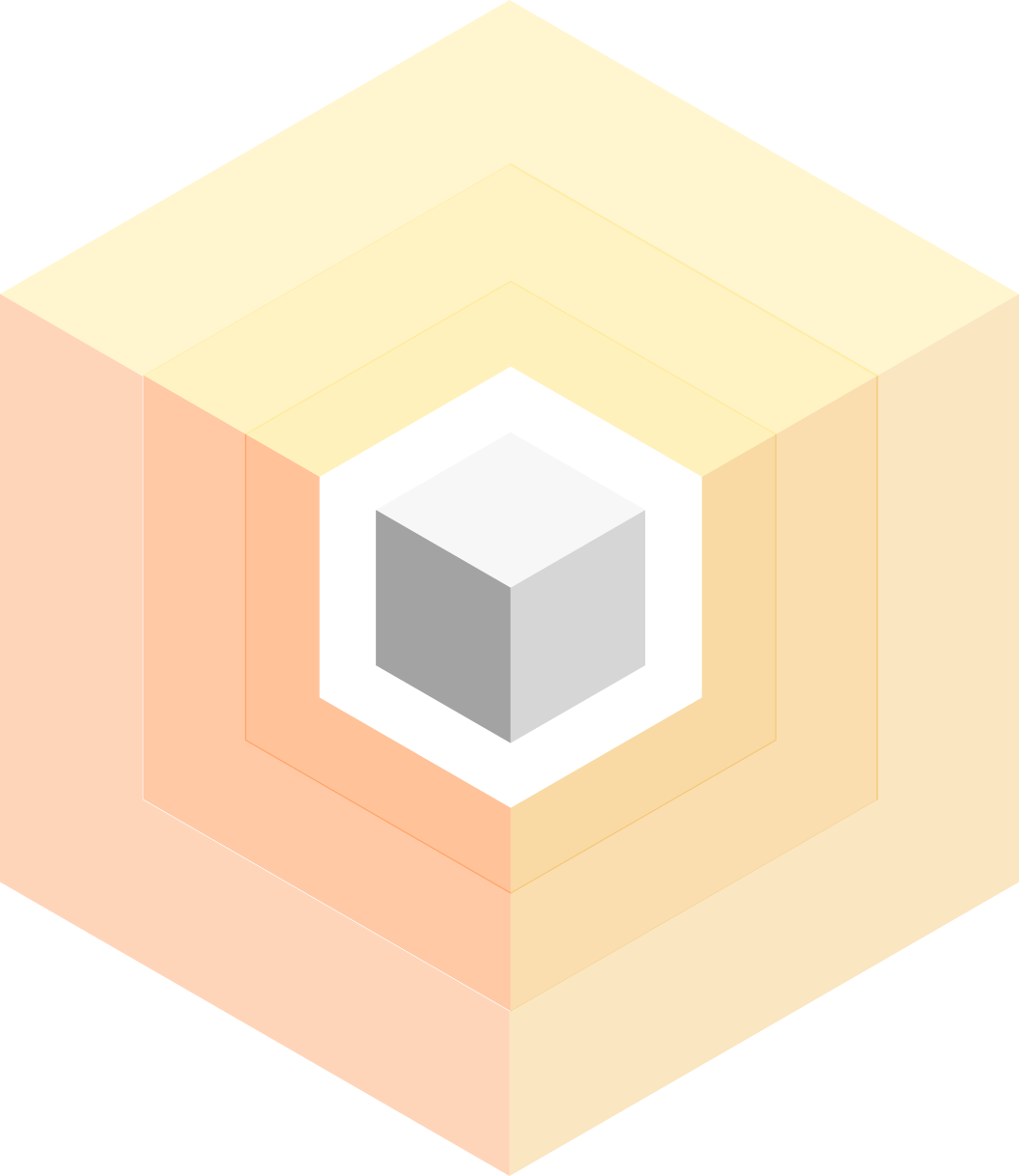
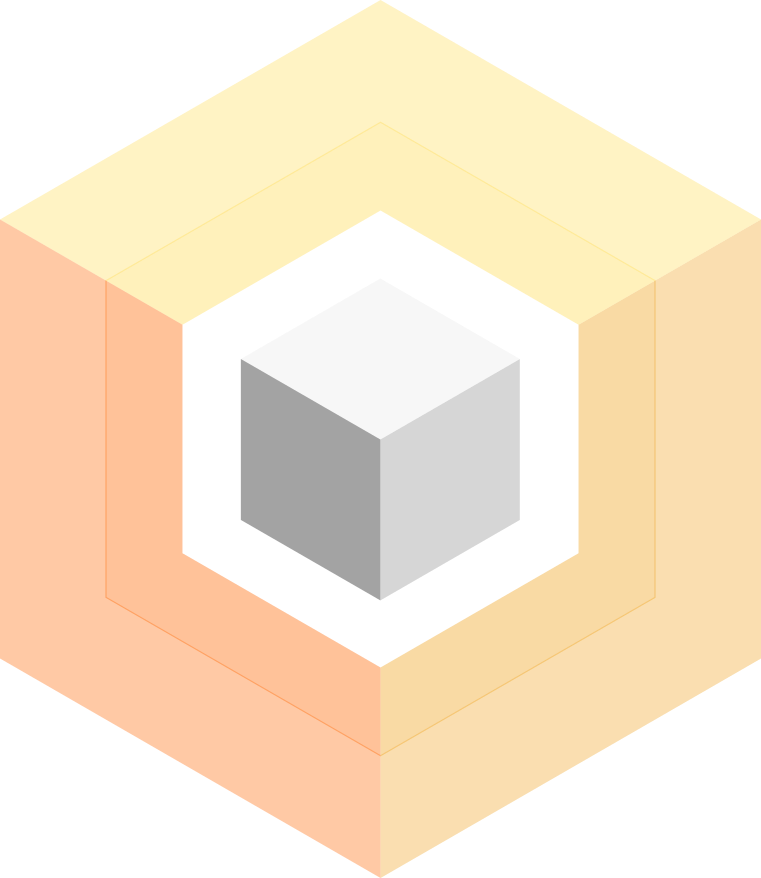
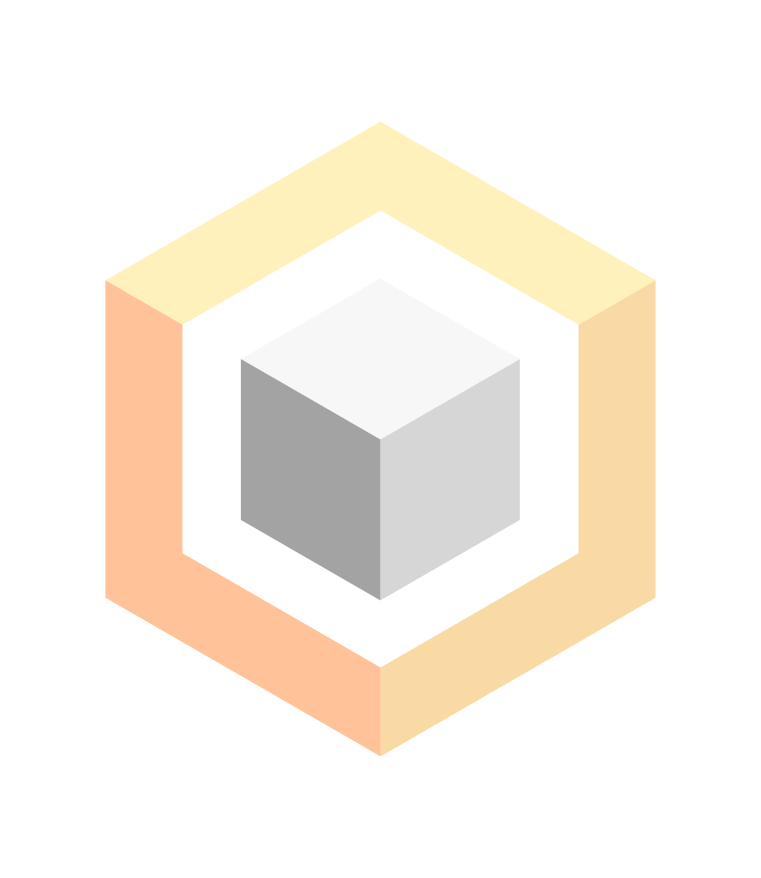
how to use HOMOTOpic maps to improve Quantum optimization Approximation Algorithms Institute of Theoretical and Applied Informatics, Polish Academy of Sciences Ludmila Botelho
Hamiltonian-Oriented Homotopy QAOA - Doctoral School Seminar
By ludmilaasb
Hamiltonian-Oriented Homotopy QAOA - Doctoral School Seminar
- 135