CS6015: Linear Algebra and Random Processes
Lecture 11: A tiny bit of ML, vector norms, orthogonal vectors, orthogonal subspaces
Please join on https://iitmadras.webex.com/join/miteshk
Learning Objectives
How do vectors and matrices show up in Machine Learning?
What are orthogonal vectors?
How do you compute the norm of a vector?
(for today's lecture)
What do you do when Ax=b does not have a solution (intuition)?
What are orthogonal subspaces?
An example from Machine Learning
Salinity
How much oil can be recovered from a drilling site?
Site 1
Site 2
Site 3
Site m
Pressure
Density
Depth
Temp.
n var.
...
(Rely on past data)
Quantity
a11a21a31⋯⋯am1 a12a22a32⋯⋯am2 a13a23a33⋯⋯am3 a14a24a34⋯⋯am4 a15a25a35⋯⋯am5⋯⋯⋯⋯⋯⋯a1na2na3n⋯⋯amn
\begin{bmatrix}
a_{11}&~&a_{12}&~&a_{13}&~&a_{14}&~&a_{15}&\cdots&a_{1n}\\
a_{21}&~&a_{22}&~&a_{23}&~&a_{24}&~&a_{25}&\cdots&a_{2n}\\
a_{31}&~&a_{32}&~&a_{33}&~&a_{34}&~&a_{35}&\cdots&a_{3n}\\
\cdots&~&\cdots&~&\cdots&~&\cdots&~&\cdots&\cdots&\cdots\\
\cdots&~&\cdots&~&\cdots&~&\cdots&~&\cdots&\cdots&\cdots\\
a_{m1}&~&a_{m2}&~&a_{m3}&~&a_{m4}&~&a_{m5}&\cdots&a_{mn}\\
\end{bmatrix}
b1b2b3⋯⋯bm
\begin{bmatrix}
b_{1}\\
b_{2}\\
b_{3}\\
\cdots\\
\cdots\\
b_{m}\\
\end{bmatrix}
You know that some relation exists between 'Quantity' and the n variables
But you don't know what f is!
b1=f(a11,a12,a13,⋯,a1n)
b_{1} = f(a_{11}, a_{12}, a_{13}, \cdots, a_{1n})
So what do you do?
An example from Machine Learning
# of Degrees
How much salary should be offered to a candidate?
Emp. 1
Emp. 2
Emp. 3
Emp. m
# Exp.
# Projects
Univ. Rank
# Prog. Lang. known
n var.
...
(Rely on past employee data)
Salary
a11a21a31⋯⋯am1 a12a22a32⋯⋯am2 a13a23a33⋯⋯am3 a14a24a34⋯⋯am4 a15a25a35⋯⋯am5⋯⋯⋯⋯⋯⋯a1na2na3n⋯⋯amn
\begin{bmatrix}
a_{11}&~&a_{12}&~&a_{13}&~&a_{14}&~&a_{15}&\cdots&a_{1n}\\
a_{21}&~&a_{22}&~&a_{23}&~&a_{24}&~&a_{25}&\cdots&a_{2n}\\
a_{31}&~&a_{32}&~&a_{33}&~&a_{34}&~&a_{35}&\cdots&a_{3n}\\
\cdots&~&\cdots&~&\cdots&~&\cdots&~&\cdots&\cdots&\cdots\\
\cdots&~&\cdots&~&\cdots&~&\cdots&~&\cdots&\cdots&\cdots\\
a_{m1}&~&a_{m2}&~&a_{m3}&~&a_{m4}&~&a_{m5}&\cdots&a_{mn}\\
\end{bmatrix}
b1b2b3⋯⋯bm
\begin{bmatrix}
b_{1}\\
b_{2}\\
b_{3}\\
\cdots\\
\cdots\\
b_{m}\\
\end{bmatrix}
You know that some relation exists between 'Salary' and the n variables
But you don't know what f is!
b1=f(a11,a12,a13,⋯,a1n)
b_{1} = f(a_{11}, a_{12}, a_{13}, \cdots, a_{1n})
So what do you do?
An example from Machine Learning
Total Pop.
How many COVID19 cases in the next week in a locality?
Loc. 1
Loc. 2
Loc. 3
Loc. m
Pop. Density
Avg. Income
Last week's count
# masks sold
n var.
...
(Rely on past data)
Cases
a11a21a31⋯⋯am1 a12a22a32⋯⋯am2 a13a23a33⋯⋯am3 a14a24a34⋯⋯am4 a15a25a35⋯⋯am5⋯⋯⋯⋯⋯⋯a1na2na3n⋯⋯amn
\begin{bmatrix}
a_{11}&~&a_{12}&~&a_{13}&~&a_{14}&~&a_{15}&\cdots&a_{1n}\\
a_{21}&~&a_{22}&~&a_{23}&~&a_{24}&~&a_{25}&\cdots&a_{2n}\\
a_{31}&~&a_{32}&~&a_{33}&~&a_{34}&~&a_{35}&\cdots&a_{3n}\\
\cdots&~&\cdots&~&\cdots&~&\cdots&~&\cdots&\cdots&\cdots\\
\cdots&~&\cdots&~&\cdots&~&\cdots&~&\cdots&\cdots&\cdots\\
a_{m1}&~&a_{m2}&~&a_{m3}&~&a_{m4}&~&a_{m5}&\cdots&a_{mn}\\
\end{bmatrix}
b1b2b3⋯⋯bm
\begin{bmatrix}
b_{1}\\
b_{2}\\
b_{3}\\
\cdots\\
\cdots\\
b_{m}\\
\end{bmatrix}
You know that some relation exists between 'Cases' and the n variables
But you don't know what f is!
b1=f(a11,a12,a13,⋯,a1n)
b_{1} = f(a_{11}, a_{12}, a_{13}, \cdots, a_{1n})
So what do you do?
A typical Machine Learning setup
You know that some relation exists between the 'output' and the 'input' variables
But you don't know what f is!
b1=f(a11,a12,a13,⋯,a1n)
b_{1} = f(a_{11}, a_{12}, a_{13}, \cdots, a_{1n})
So what do you do?
You make some assumption about f
What is the simplest assumption you can make?
simple, interpretable, less predictive (less accurate)
Can you make more complex assumptions?
Yes,
b1=a11x1+a12x2+a13x3⋯+a1nxn
b_{1} = a_{11}x_1 + a_{12}x_2 + a_{13}x_3 \cdots+ a_{1n}x_n
b1=wkgk(⋯w3g3(w2g2(a11x1+a12x2+a13x3⋯,a1nxn)))
b_{1} = w_kg_k(\cdots w_3g_3(w_2g_2(a_{11}x_1 + a_{12}x_2 + a_{13}x_3 \cdots, a_{1n}x_n)))
complex, uninterpretable, more predictive (accurate)
(psst! psst! Deep Learning)
f is linear
In this course
What's the connection to Linear Algebra
a11x1+a12x2+a13x3⋯+a1nxn=b1
a_{11}x_1 + a_{12}x_2 + a_{13}x_3 \cdots+ a_{1n}x_n = b_{1}
a21x1+a22x2+a23x3⋯+a2nxn=b2
a_{21}x_1 + a_{22}x_2 + a_{23}x_3 \cdots+ a_{2n}x_n = b_{2}
a31x1+a32x2+a33x3⋯+a3nxn=b3
a_{31}x_1 + a_{32}x_2 + a_{33}x_3 \cdots+ a_{3n}x_n = b_{3}
am1x1+am2x2+am3x3⋯+amnxn=bm
a_{m1}x_1 + a_{m2}x_2 + a_{m3}x_3 \cdots+ a_{mn}x_n = b_{m}
⋯
\cdots
⋯
\cdots
⋯
\cdots
⋯
\cdots
⋯
\cdots
⋯
\cdots
⋯
\cdots
⋯
\cdots
Loc. 1
Loc. 2
Loc. 3
Loc. m
We are interested in finding the x's which best explain the relationship between the a's and the b's
In other words .....
What's the connection to Linear Algebra
Loc. 1
Loc. 2
Loc. 3
Loc. m
We are interested in finding the x's which best explain the relationship between the a's and the b's
In other words .....
..... we are interested in solving Ax=b
a11a21a31⋯⋯am1 a12a22a32⋯⋯am2 a13a23a33⋯⋯am3 a14a24a34⋯⋯am4 a15a25a35⋯⋯am5⋯⋯⋯⋯⋯⋯a1na2na3n⋯⋯amn
\begin{bmatrix}
a_{11}&~&a_{12}&~&a_{13}&~&a_{14}&~&a_{15}&\cdots&a_{1n}\\
a_{21}&~&a_{22}&~&a_{23}&~&a_{24}&~&a_{25}&\cdots&a_{2n}\\
a_{31}&~&a_{32}&~&a_{33}&~&a_{34}&~&a_{35}&\cdots&a_{3n}\\
\cdots&~&\cdots&~&\cdots&~&\cdots&~&\cdots&\cdots&\cdots\\
\cdots&~&\cdots&~&\cdots&~&\cdots&~&\cdots&\cdots&\cdots\\
a_{m1}&~&a_{m2}&~&a_{m3}&~&a_{m4}&~&a_{m5}&\cdots&a_{mn}\\
\end{bmatrix}
=b1b2b3⋯⋯bm
=\begin{bmatrix}
b_{1}\\
b_{2}\\
b_{3}\\
\cdots\\
\cdots\\
b_{m}\\
\end{bmatrix}
x1x2x3⋯⋯xn
\begin{bmatrix}
x_{1}\\
x_{2}\\
x_{3}\\
\cdots\\
\cdots\\
x_{n}\\
\end{bmatrix}
A
A
x
\mathbf{x}
b
\mathbf{b}
What could go wrong?
Loc. 1
Loc. 2
Loc. 3
Loc. m
0 solutions
a11a21a31⋯⋯am1 a12a22a32⋯⋯am2 a13a23a33⋯⋯am3 a14a24a34⋯⋯am4 a15a25a35⋯⋯am5⋯⋯⋯⋯⋯⋯a1na2na3n⋯⋯amn
\begin{bmatrix}
a_{11}&~&a_{12}&~&a_{13}&~&a_{14}&~&a_{15}&\cdots&a_{1n}\\
a_{21}&~&a_{22}&~&a_{23}&~&a_{24}&~&a_{25}&\cdots&a_{2n}\\
a_{31}&~&a_{32}&~&a_{33}&~&a_{34}&~&a_{35}&\cdots&a_{3n}\\
\cdots&~&\cdots&~&\cdots&~&\cdots&~&\cdots&\cdots&\cdots\\
\cdots&~&\cdots&~&\cdots&~&\cdots&~&\cdots&\cdots&\cdots\\
a_{m1}&~&a_{m2}&~&a_{m3}&~&a_{m4}&~&a_{m5}&\cdots&a_{mn}\\
\end{bmatrix}
=b1b2b3⋯⋯bm
=\begin{bmatrix}
b_{1}\\
b_{2}\\
b_{3}\\
\cdots\\
\cdots\\
b_{m}\\
\end{bmatrix}
x1x2x3⋯⋯xn
\begin{bmatrix}
x_{1}\\
x_{2}\\
x_{3}\\
\cdots\\
\cdots\\
x_{n}\\
\end{bmatrix}
inconsistent equations
For example two patients with the same heart rate, blood sugar level, weight, height (inputs) but a different blood cholesterol level (output)
72x1+84x2+175x3⋯+78xn=110
72x_1 + 84x_2 + 175x_3 \cdots+ 78x_n =110
72x1+84x2+175x3⋯+78xn=120
72x_1 + 84x_2 + 175x_3 \cdots+ 78x_n =120
What can you do?
Loc. 1
Loc. 2
Loc. 3
Loc. m
0 solutions
a11a21a31⋯⋯am1 a12a22a32⋯⋯am2 a13a23a33⋯⋯am3 a14a24a34⋯⋯am4 a15a25a35⋯⋯am5⋯⋯⋯⋯⋯⋯a1na2na3n⋯⋯amn
\begin{bmatrix}
a_{11}&~&a_{12}&~&a_{13}&~&a_{14}&~&a_{15}&\cdots&a_{1n}\\
a_{21}&~&a_{22}&~&a_{23}&~&a_{24}&~&a_{25}&\cdots&a_{2n}\\
a_{31}&~&a_{32}&~&a_{33}&~&a_{34}&~&a_{35}&\cdots&a_{3n}\\
\cdots&~&\cdots&~&\cdots&~&\cdots&~&\cdots&\cdots&\cdots\\
\cdots&~&\cdots&~&\cdots&~&\cdots&~&\cdots&\cdots&\cdots\\
a_{m1}&~&a_{m2}&~&a_{m3}&~&a_{m4}&~&a_{m5}&\cdots&a_{mn}\\
\end{bmatrix}
=b1b2b3⋯⋯bm
=\begin{bmatrix}
b_{1}\\
b_{2}\\
b_{3}\\
\cdots\\
\cdots\\
b_{m}\\
\end{bmatrix}
x1x2x3⋯⋯xn
\begin{bmatrix}
x_{1}\\
x_{2}\\
x_{3}\\
\cdots\\
\cdots\\
x_{n}\\
\end{bmatrix}
inconsistent equations
Find the best possible solution by tolerating some noise
72x1+84x2+175x3⋯+78xn=110
72x_1 + 84x_2 + 175x_3 \cdots+ 78x_n =110
72x1+84x2+175x3⋯+78xn=120
72x_1 + 84x_2 + 175x_3 \cdots+ 78x_n =120
115
115
115
115
What is the geometric view?
a117272⋯⋯am1 a128484⋯⋯am2 a13175175⋯⋯am3 a14⋯⋯⋯⋯am4 a15⋯⋯⋯⋯am5⋯⋯⋯⋯⋯⋯a1n7878⋯⋯amn
\begin{bmatrix}
a_{11}&~&a_{12}&~&a_{13}&~&a_{14}&~&a_{15}&\cdots&a_{1n}\\
72&~&84&~&175&~&\cdots&~&\cdots&\cdots&78\\
72&~&84&~&175&~&\cdots&~&\cdots&\cdots&78\\
\cdots&~&\cdots&~&\cdots&~&\cdots&~&\cdots&\cdots&\cdots\\
\cdots&~&\cdots&~&\cdots&~&\cdots&~&\cdots&\cdots&\cdots\\
a_{m1}&~&a_{m2}&~&a_{m3}&~&a_{m4}&~&a_{m5}&\cdots&a_{mn}\\
\end{bmatrix}
=b1110120⋯⋯bm
=\begin{bmatrix}
b_{1}\\
110\\
120\\
\cdots\\
\cdots\\
b_{m}\\
\end{bmatrix}
x1x2x3⋯⋯xm
\begin{bmatrix}
x_{1}\\
x_{2}\\
x_{3}\\
\cdots\\
\cdots\\
x_{m}\\
\end{bmatrix}
A
A
b
\mathbf{b}
b
\mathbf{b}
column space of A
p
\mathbf{p}
p1115115⋯⋯pm
\begin{bmatrix}
p_{1}\\
115\\
115\\
\cdots\\
\cdots\\
p_{m}\\
\end{bmatrix}
p
\mathbf{p}
"Project" b into the column space of A
Solve Ax^=p
x^ is the best possible approximation
b is not in the column space of A
Hence no solution to Ax=b
What next?
How do we project b into the column space of A?
But first a detour to build some concepts ...
What are orthogonal vectors?
What is the norm of a vector?
What are orthogonal subspaces?
The norm of a vector
Given a vector space V, the norm of a vector is a non-negative valued function p:V→R with the following properties
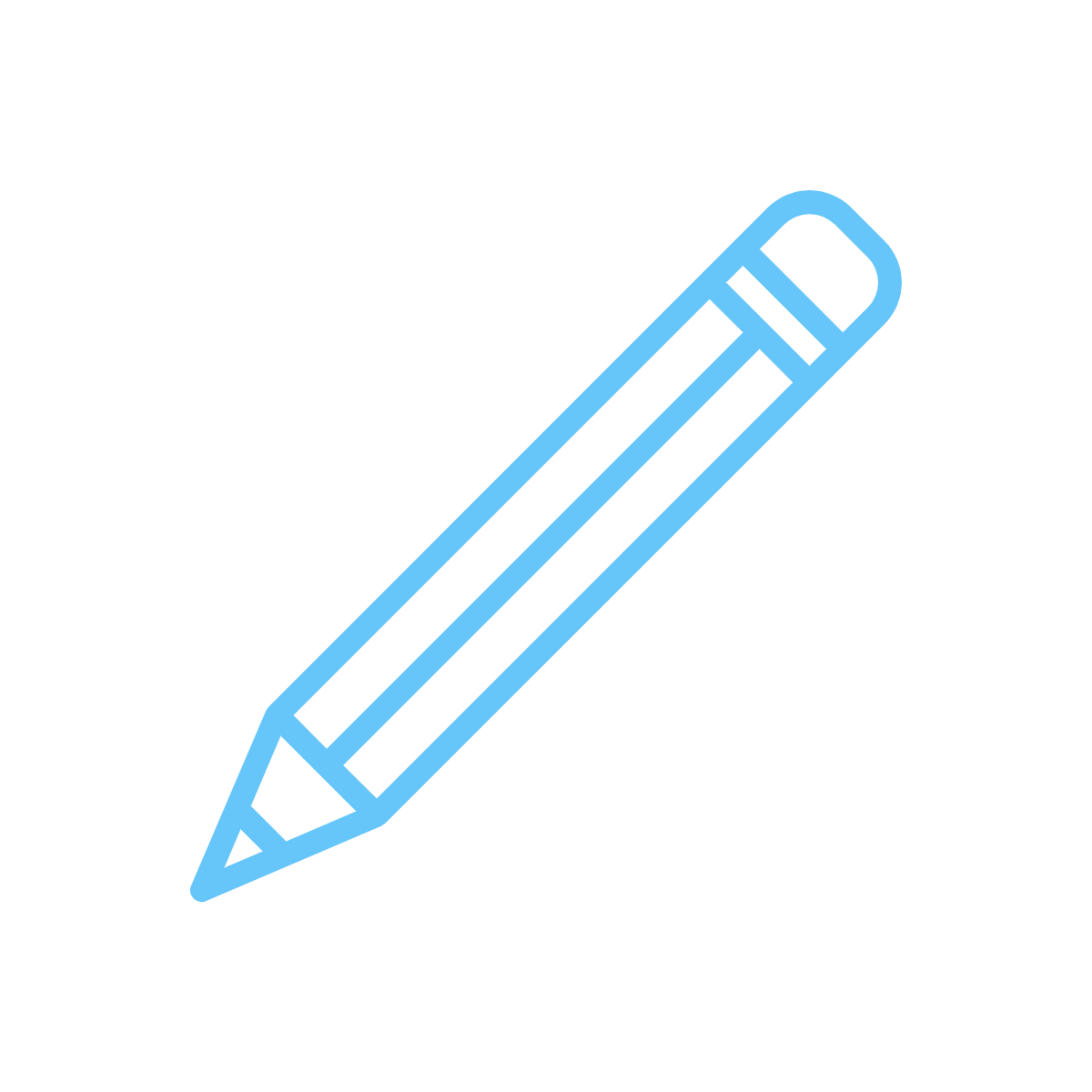
p(u+v)≤p(u)+p(v)
p(\mathbf{u} + \mathbf{v}) \leq p(\mathbf{u}) + p(\mathbf{v})
p(au)=∣a∣p(u)
p(a\mathbf{u}) = |a| p(\mathbf{u})
If p(u)=0 then u=0
If~p(\mathbf{u}) = 0~then~\mathbf{u}=\mathbf{0}
(triangular inequality)
u
\mathbf{u}
v
\mathbf{v}
u+v
\mathbf{u}+\mathbf{v}
Examples of norm
L1 norm
L_{1}~norm
∣∣x∣∣1=∣x1∣+∣x2∣+∣x3∣+⋯+∣xn∣
||\mathbf{x}||_1 = |x_1| + |x_2| + |x_3| + \cdots + |x_n|
(l1 norm)
(\mathcal{l}_{1}~norm)
L2 norm
L_{2}~norm
(l2 norm)
(\mathcal{l}_{2}~norm)
(Euclidean norm)
(Euclidean~norm)
(taxicab norm)
(taxicab~norm)
∣∣x∣∣2=(∣x1∣2+∣x2∣2+∣x3∣2+⋯+∣xn∣2)21
||\mathbf{x}||_2 = (|x_1|^2 + |x_2|^2 + |x_3|^2 + \cdots + |x_n|^2)^{\frac{1}{2}}
=x12+x22+x32+⋯+xn2
= \sqrt{x_1^2 + x_2^2 + x_3^2 + \cdots + x_n^2}
Lp norm
L_{p}~norm
(lp norm)
(\mathcal{l}_{p}~norm)
∣∣x∣∣p=(∣x1∣p+∣x2∣p+∣x3∣p+⋯+∣xn∣p)p1
||\mathbf{x}||_p = (|x_1|^p + |x_2|^p + |x_3|^p + \cdots + |x_n|^p)^{\frac{1}{p}}
=x⊤x
= \sqrt{\mathbf{x}^\top\mathbf{x}}
[x1 x2 x3 ⋯ xn]
[x_1~x_2~x_3~\cdots~x_n]
x1x2x3⋯xn
\begin{bmatrix}
x_1\\
x_2\\
x_3\\
\cdots\\
x_n
\end{bmatrix}
x⊤
\mathbf{x}^\top
x
\mathbf{x}
(most commonly used)
=∑i=1n(∣xi∣p)p1
= \sum_{i=1}^{n}(|x_i|^p)^{\frac{1}{p}}
Orthogonal vectors
Vectors u and v are orthogonal if the angle between them is 90°
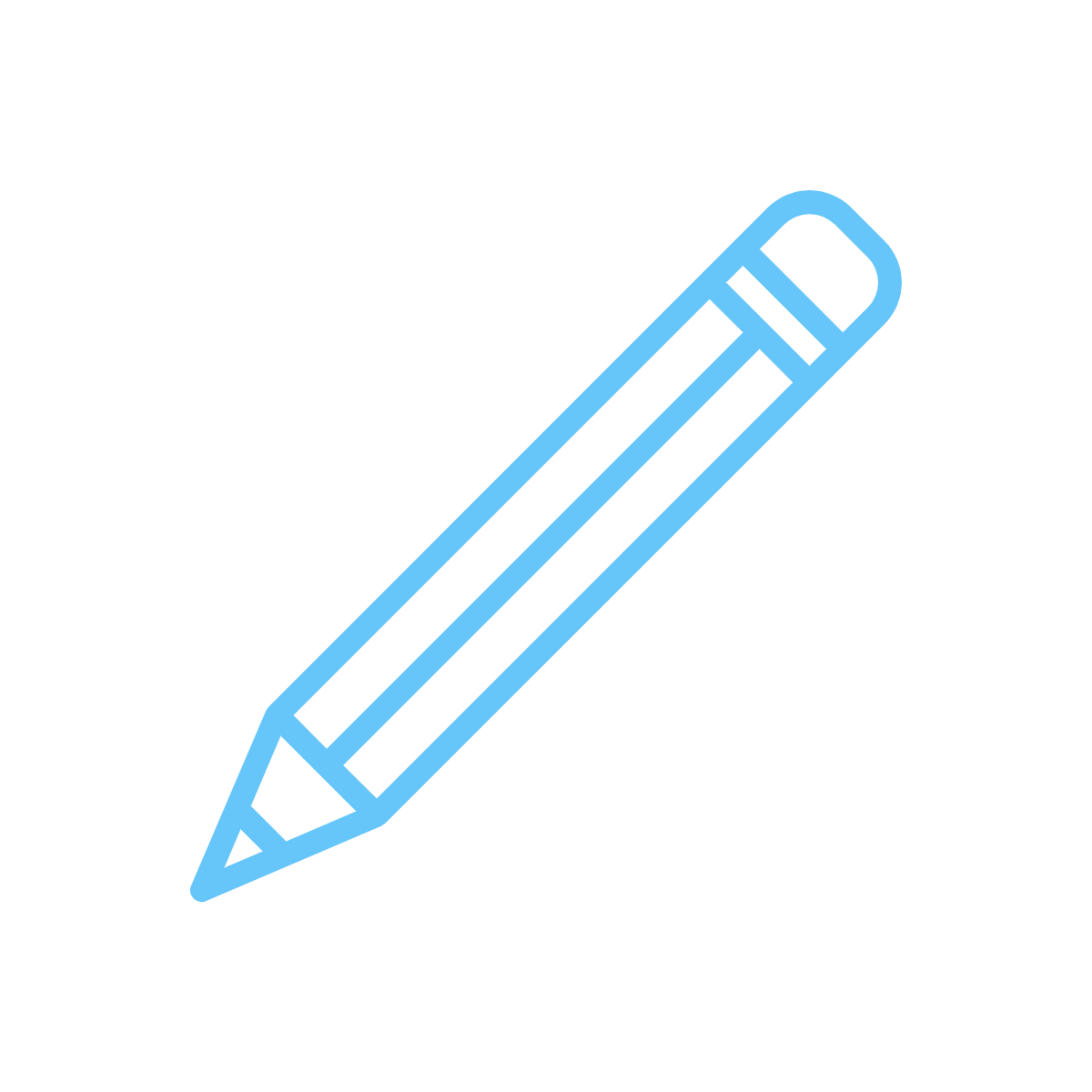
Condition for orthogonality
By Pythagoras' Theorem
(∣∣u+v∣∣2)2=(∣∣u∣∣2)2+(∣∣v∣∣2)2
(||\mathbf{u}+\mathbf{v}||_2)^2 = (||\mathbf{u}||_2)^2 + (||\mathbf{v}||_2)^2
⟹(u+v)⊤(u+v)=u⊤u+v⊤v
\implies (\mathbf{u}+\mathbf{v})^\top(\mathbf{u}+\mathbf{v}) = \mathbf{u}^\top\mathbf{u} + \mathbf{v}^\top\mathbf{v}
⟹(u⊤+v⊤)(u+v)=u⊤u+v⊤v
\implies (\mathbf{u}^\top+\mathbf{v}^\top)(\mathbf{u}+\mathbf{v}) = \mathbf{u}^\top\mathbf{u} + \mathbf{v}^\top\mathbf{v}
⟹u⊤u+v⊤u+u⊤v+v⊤v=u⊤u+v⊤v
\implies \mathbf{u}^\top\mathbf{u}+\mathbf{v}^\top\mathbf{u} + \mathbf{u}^\top\mathbf{v}+\mathbf{v}^\top\mathbf{v} = \mathbf{u}^\top\mathbf{u} + \mathbf{v}^\top\mathbf{v}
⟹u⊤v=0
\implies \mathbf{u}^\top\mathbf{v} = 0
(the dot product of the two vectors will be 0)
u
\mathbf{u}
v
\mathbf{v}
u+v
\mathbf{u}+\mathbf{v}
Orthogonal subspaces
Two subspaces S1 and S2 are orthogonal if every vector u∈S1 is orthogonal to every vector in v∈S2
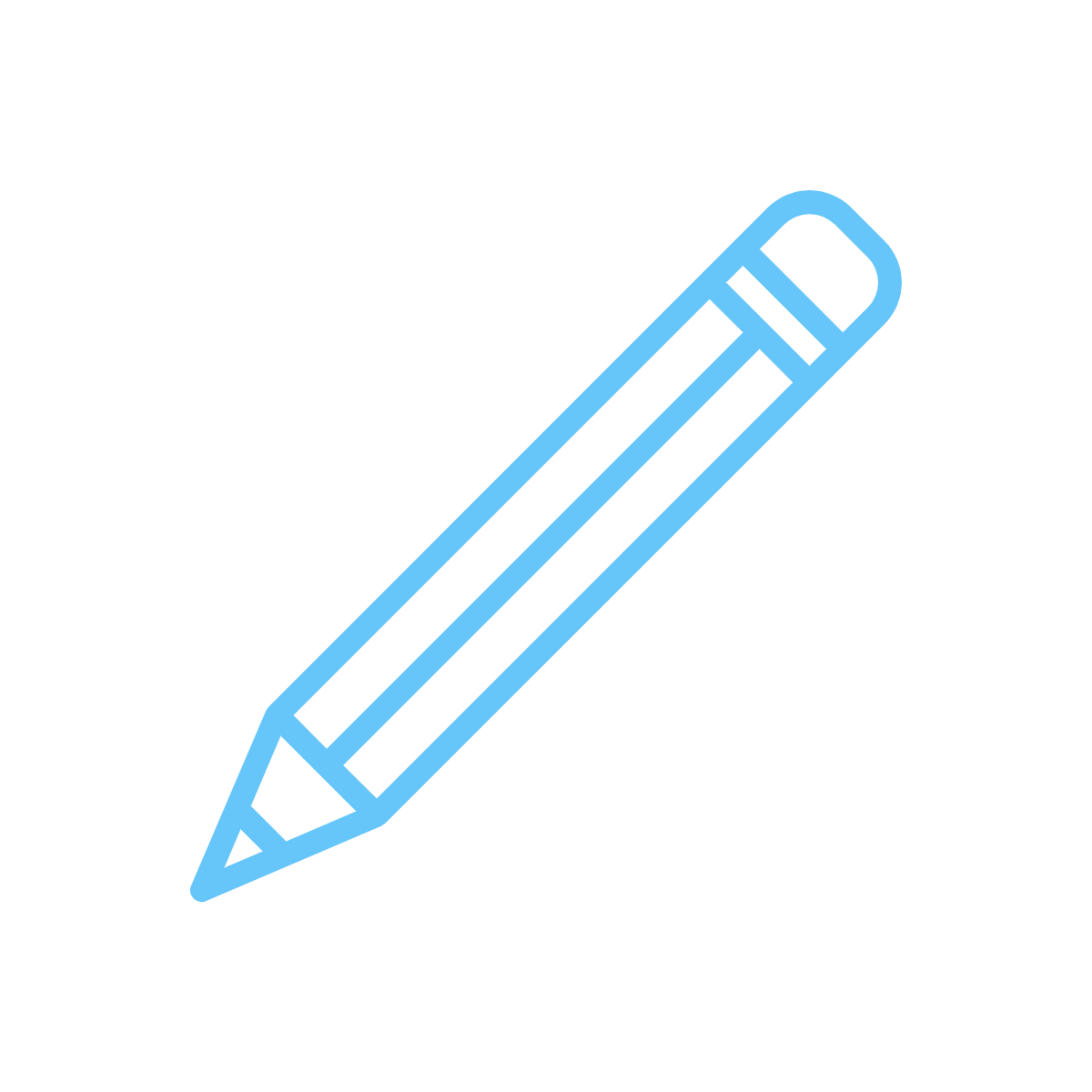
(switch to geogebra)
Orthogonal subspaces
C(A)
\mathcal{C}(A)
N(A⊤)
\mathcal{N}(A^\top)
inside Rm
inside~\mathbb{R}^m
←a1⊤→←a2⊤→←a3⊤→
\begin{bmatrix}
\leftarrow \mathbf{a_1}^\top \rightarrow\\
\leftarrow \mathbf{a_2}^\top \rightarrow\\
\leftarrow \mathbf{a_3}^\top \rightarrow\\
\end{bmatrix}
↑a1↓↑a2↓↑a3↓
\begin{bmatrix}
\uparrow&\uparrow&\uparrow\\
\mathbf{a_1}&\mathbf{a_2}&\mathbf{a_3}\\
\downarrow&\downarrow&\downarrow\\
\end{bmatrix}
A
A
A⊤
A^\top
x1x2x3
\begin{bmatrix}
x_1\\
x_2\\
x_3
\end{bmatrix}
x
\mathbf{x}
=000
= \begin{bmatrix}
0\\0\\0
\end{bmatrix}
x∈N(A⊤)
\mathbf{x} \in \mathcal{N}(A^\top)
⟹a1⊤x=0
\implies \mathbf{a_1}^\top\mathbf{x} = 0
a2⊤x=0
\mathbf{a_2}^\top\mathbf{x} = 0
a3⊤x=0
\mathbf{a_3}^\top\mathbf{x} = 0
⟹(pa1+qa2+ra3)⊤x=0
\implies (p\mathbf{a_1}+q\mathbf{a_2}+r\mathbf{a_3})^\top\mathbf{x} = 0
⟹C(A)⊥N(A⊤)
\implies \mathcal{C}(A) \perp \mathcal{N}(A^\top)
The 4 fundamental subspaces
C(A)
\mathcal{C}(A)
N(A⊤)
\mathcal{N}(A^\top)
inside Rm
inside~\mathbb{R}^m
C(A⊤)
\mathcal{C}(A^\top)
N(A)
\mathcal{N}(A)
inside Rn
inside~\mathbb{R}^n
dim=r
dim=r
dim=n−r
dim=n-r
dim=r
dim=r
dim=m−r
dim=m-r
C(A)⊥N(A⊤)
\mathcal{C}(A) \perp \mathcal{N}(A^\top)
C(A⊤)⊥N(A)
\mathcal{C}(A^\top) \perp \mathcal{N}(A)
orthogonal complements
orthogonal complements
Example: C(A)⊥N(A⊤)
111123234
\begin{bmatrix}
1&1&2\\
1&2&3\\
1&3&4\\
\end{bmatrix}
A
A
100110210
\begin{bmatrix}
1&1&2\\
0&1&1\\
0&0&0\\
\end{bmatrix}
U
U
Basis(C(A))
Basis(\mathcal{C}(A))
=111
=\begin{bmatrix}
1\\
1\\
1\\
\end{bmatrix}
,123
,\begin{bmatrix}
1\\
2\\
3\\
\end{bmatrix}
Basis(N(A⊤))
Basis(\mathcal{N}(A^\top))
=11−1
=\begin{bmatrix}
1\\
1\\
-1\\
\end{bmatrix}
112123134
\begin{bmatrix}
1&1&1\\
1&2&3\\
2&3&4\\
\end{bmatrix}
A⊤
A^\top
100110120
\begin{bmatrix}
1&1&1\\
0&1&2\\
0&0&0\\
\end{bmatrix}
A⊤x=0
A^\top\mathbf{x} = \mathbf{0}
x=1−21
\mathbf{x}=\begin{bmatrix}
1\\
-2\\
1\\
\end{bmatrix}
special solution
(switch to geogebra)
Practice Problems
For each of the above matrices, verify whether the null space of A is orthogonal to the column space of A⊤
112211320
\begin{bmatrix}
1&2&3\\
1&1&2\\
2&1&0
\end{bmatrix}
112220320311212122
\begin{bmatrix}
1&2&3&3&2&1\\
1&2&2&1&1&2\\
2&0&0&1&2&2
\end{bmatrix}
0123−122211112131−20
\begin{bmatrix}
0&2&2\\
1&2&1\\
2&1&3\\
3&1&1\\
-1&1&-2\\
2&1&0\\
\end{bmatrix}
124215306317226124
\begin{bmatrix}
1&2&3&3&2&1\\
2&1&0&1&2&2\\
4&5&6&7&6&4
\end{bmatrix}
Learning Objectives
(achieved)
How do vectors and matrices show up in Machine Learning?
What are orthogonal vectors?
How do you compute the norm of a vector?
What do you do when Ax=b does not have a solution (intuition)?
What are orthogonal subspaces?
CS6015: Linear Algebra and Random Processes Lecture 11: A tiny bit of ML, vector norms, orthogonal vectors, orthogonal subspaces
CS6015: Lecture 11
By Mitesh Khapra
CS6015: Lecture 11
Lecture 11: A tiny bit of ML, vector norms, orthogonal vectors, orthogonal subspaces
- 2,718