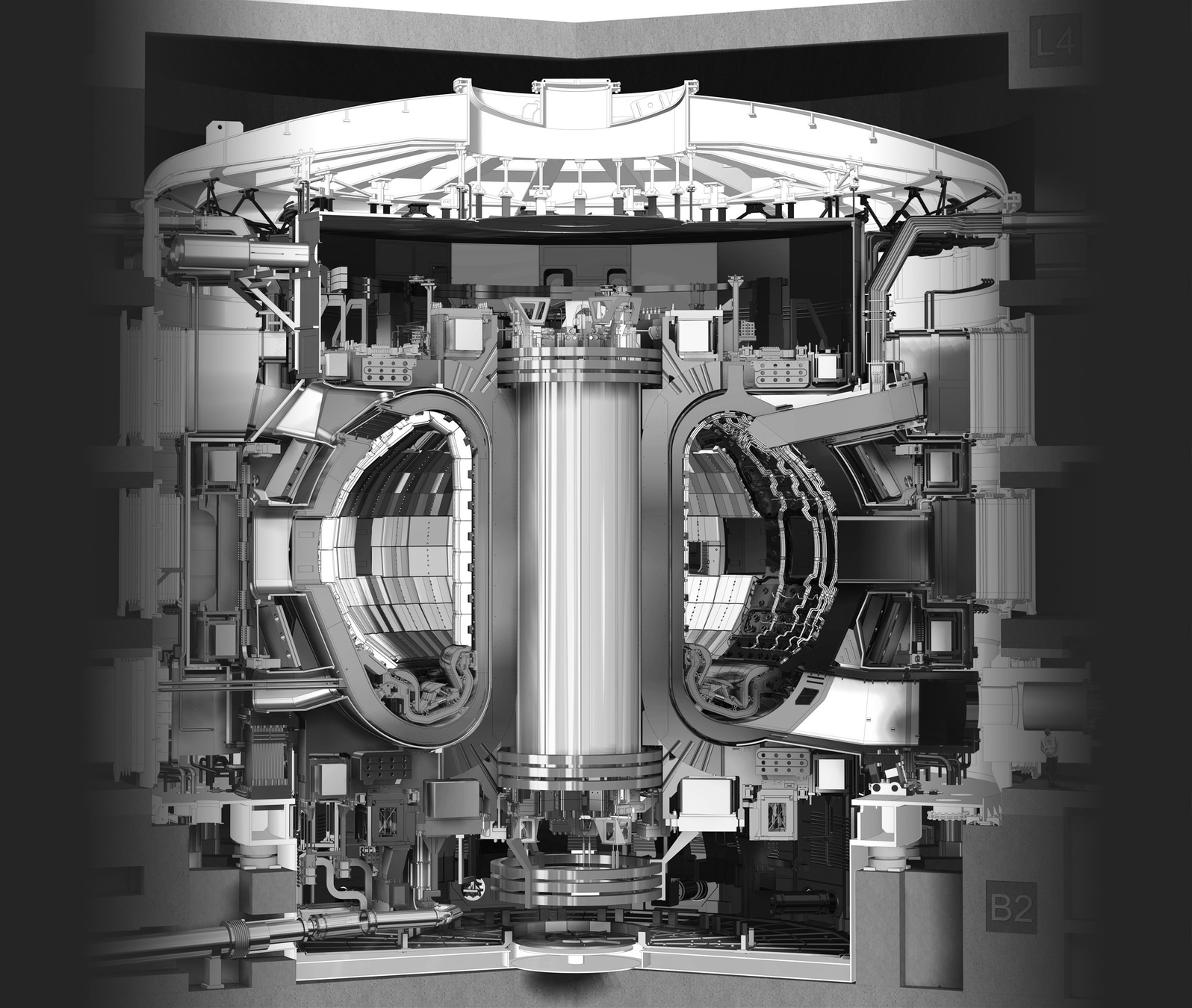
Hydrogen transport in tokamaks
Estimation of the ITER divertor tritium inventory and influence of helium exposure
Rémi Delaporte-Mathurin
17-10-2022
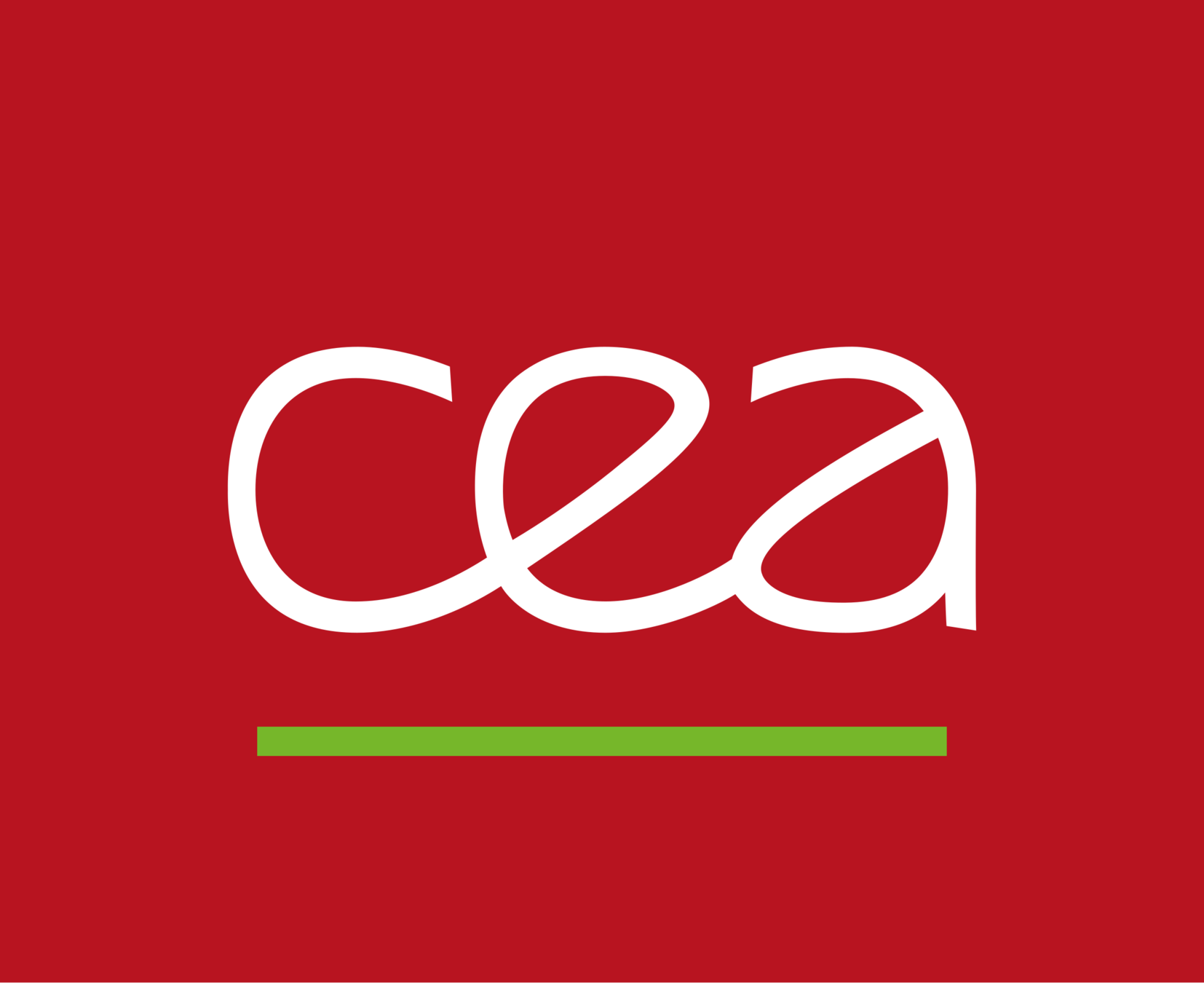
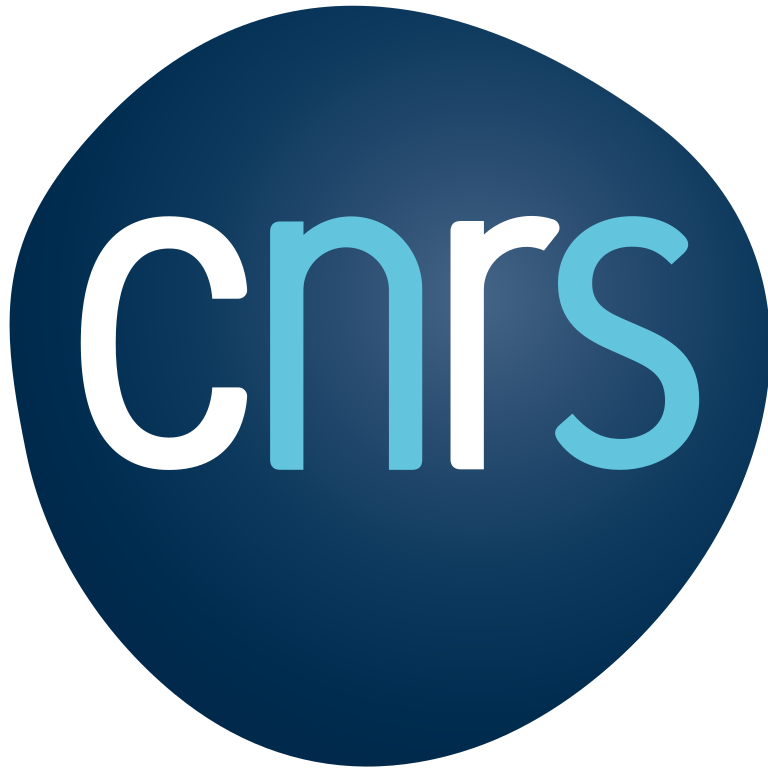
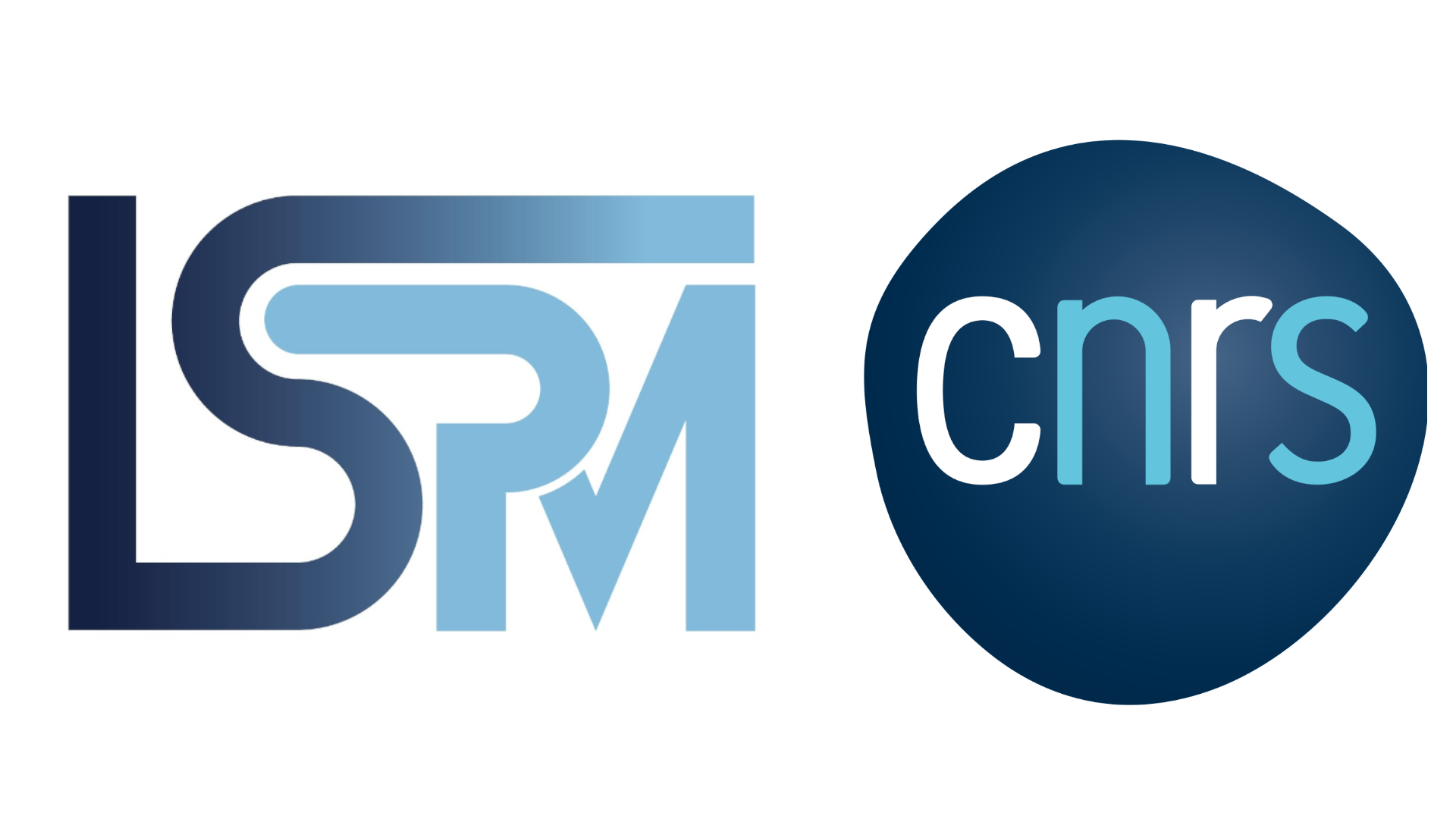
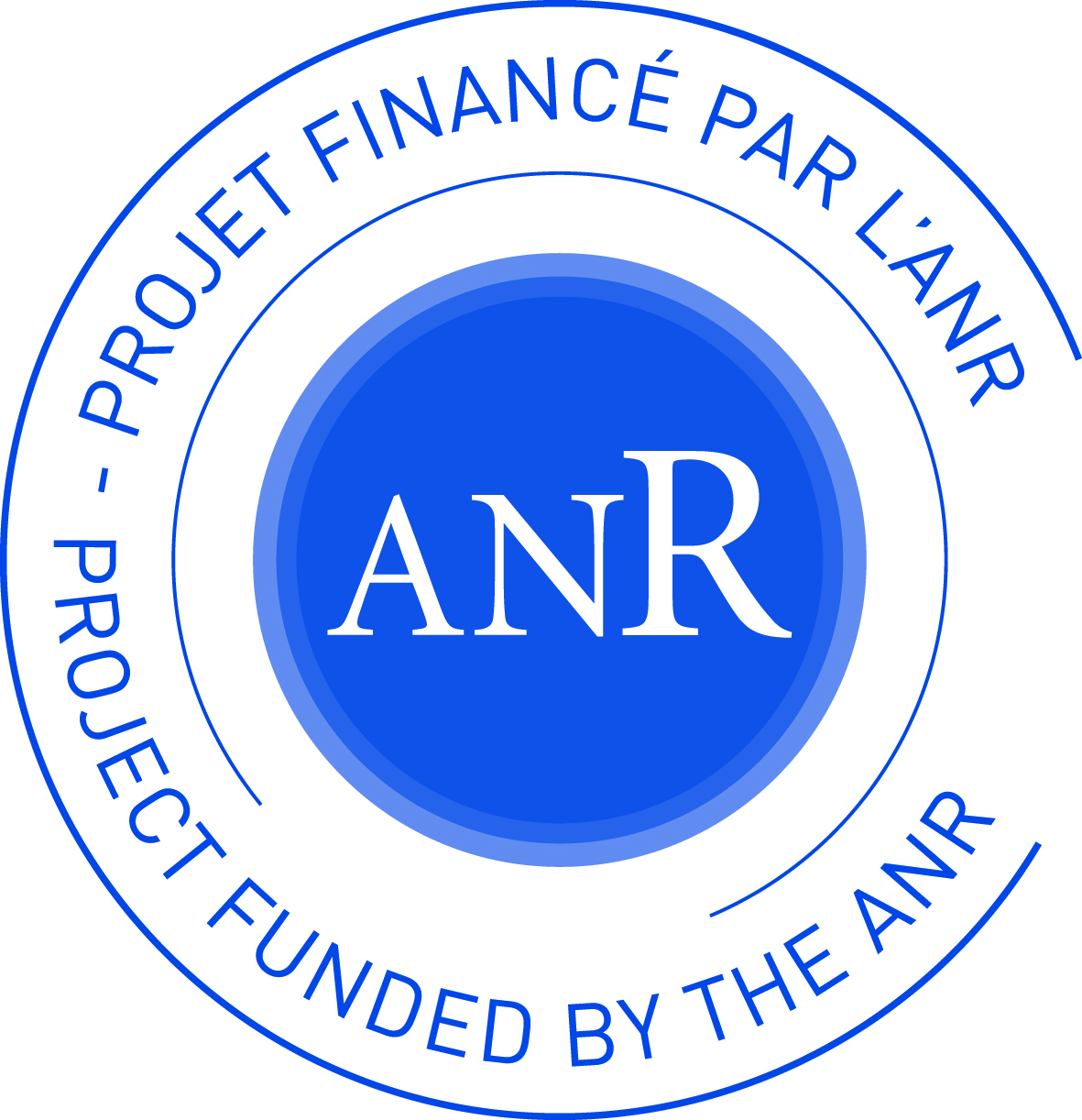
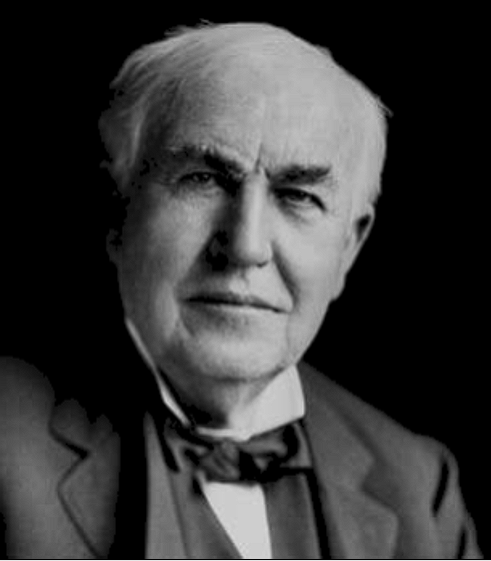
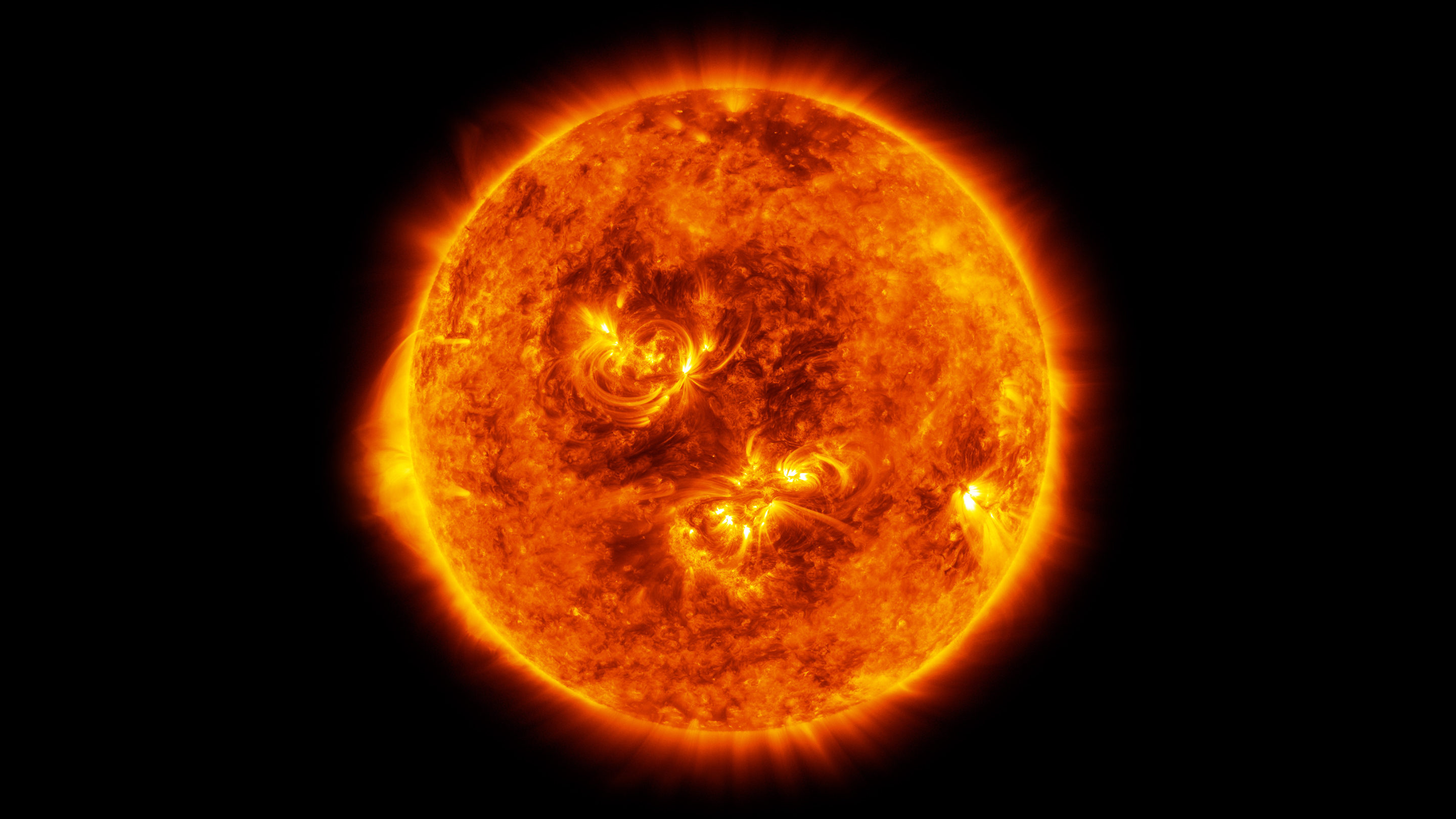
“I’d put my money on the Sun. What a source of power!”
Thomas Edison, 1931
Every second:
→ Fuses 500 Mt of hydrogen
→ Produces a million times the world’s energy consumption
On Earth
Deuterium
Tritium
Neutron
Helium
✔️No CO2 emission
✔️No long lived radioactive waste
✔️Inherently safe
✔️Abundant fuel
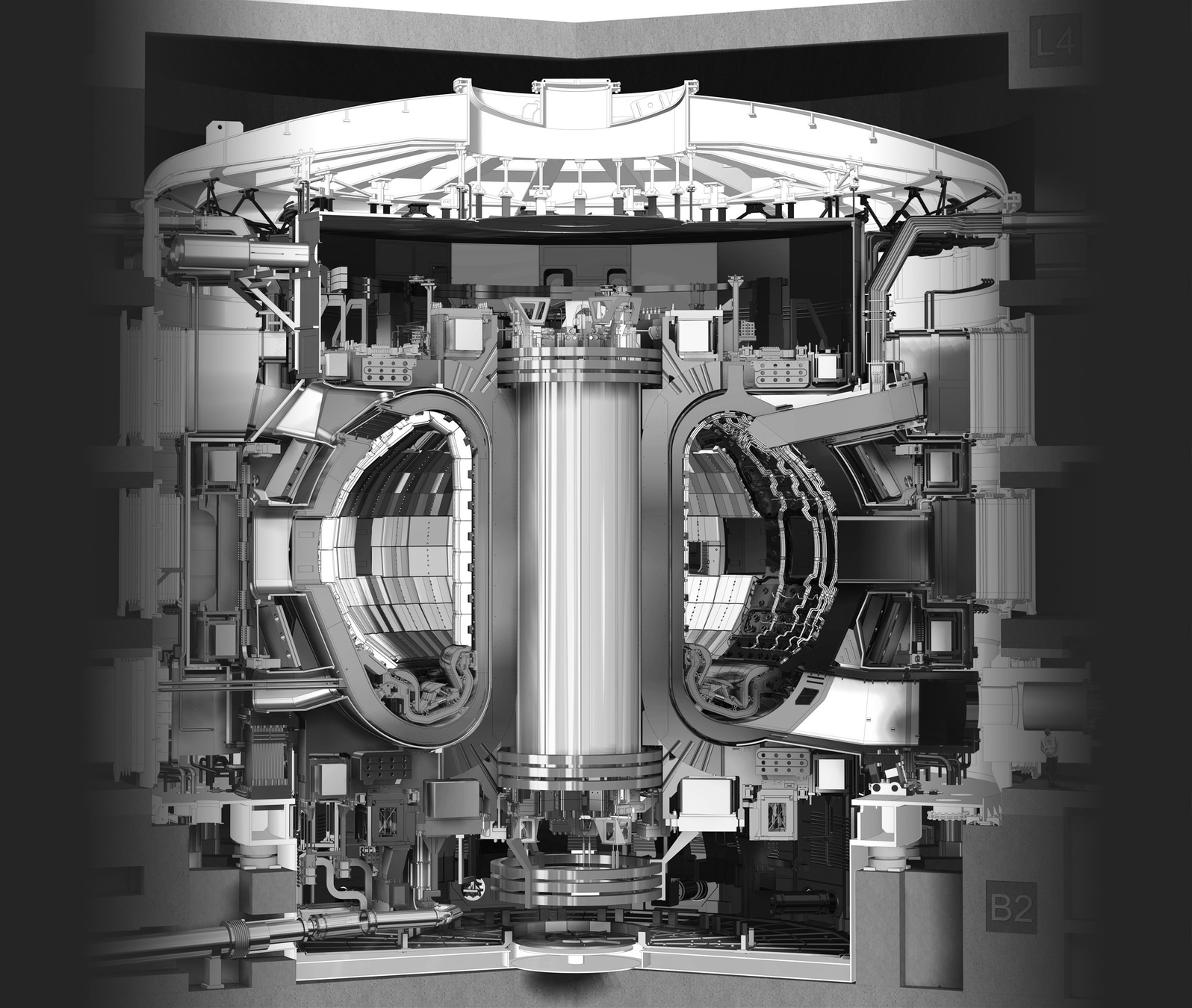
ITER
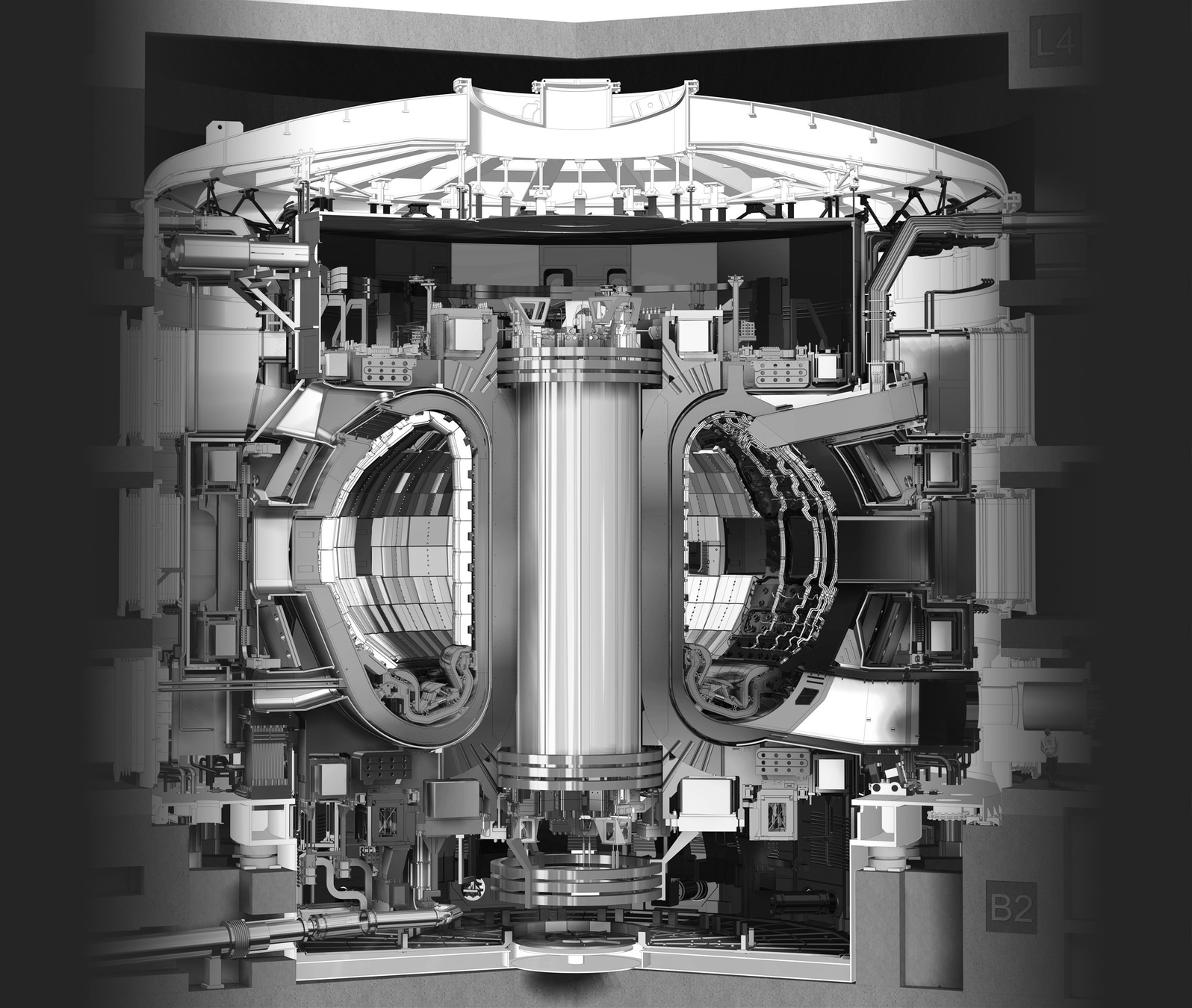
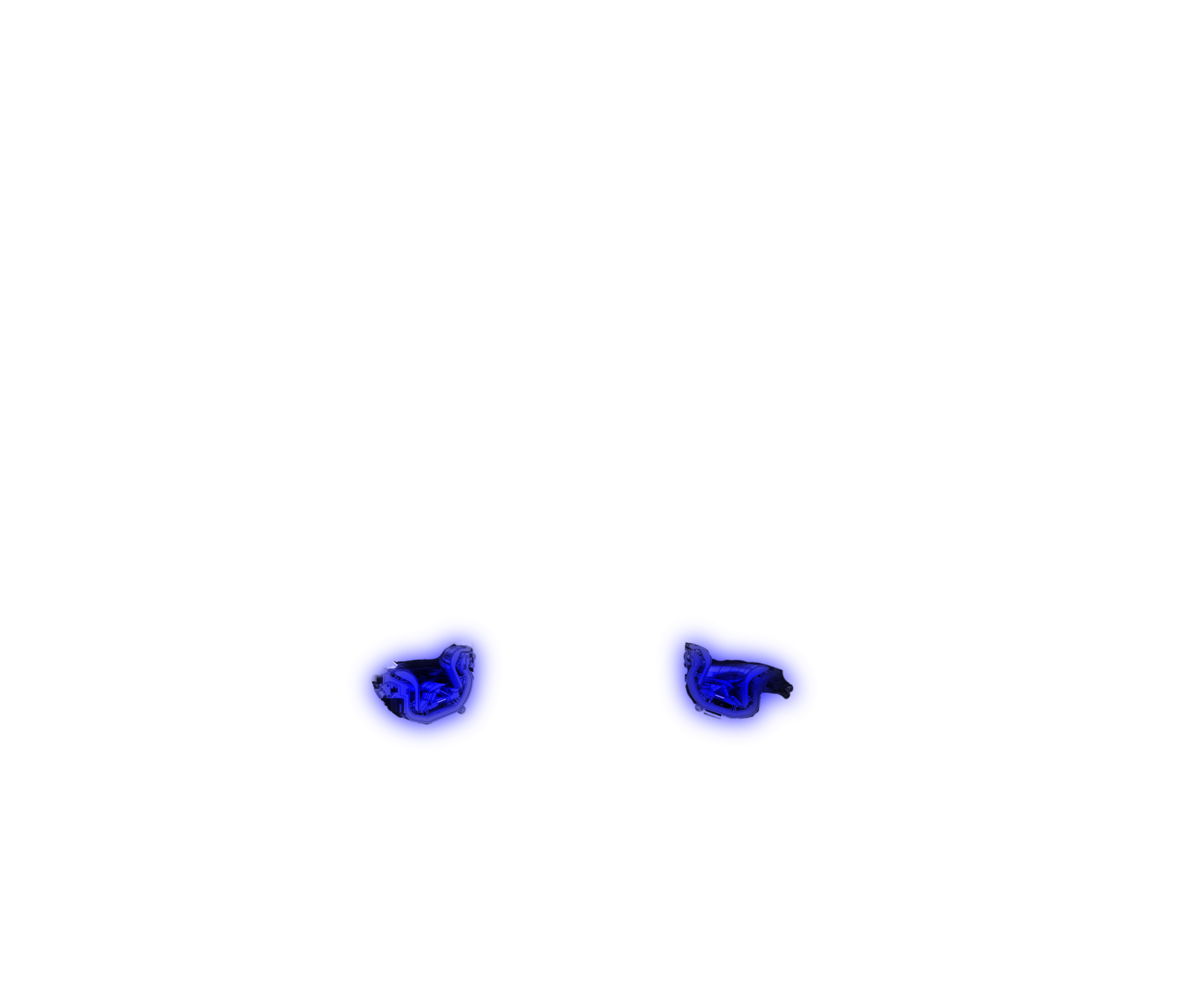
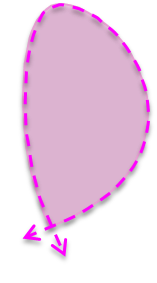
Plasma: mixture of Hydrogen (D-T) and Helium
Particle bombardment
Divertor
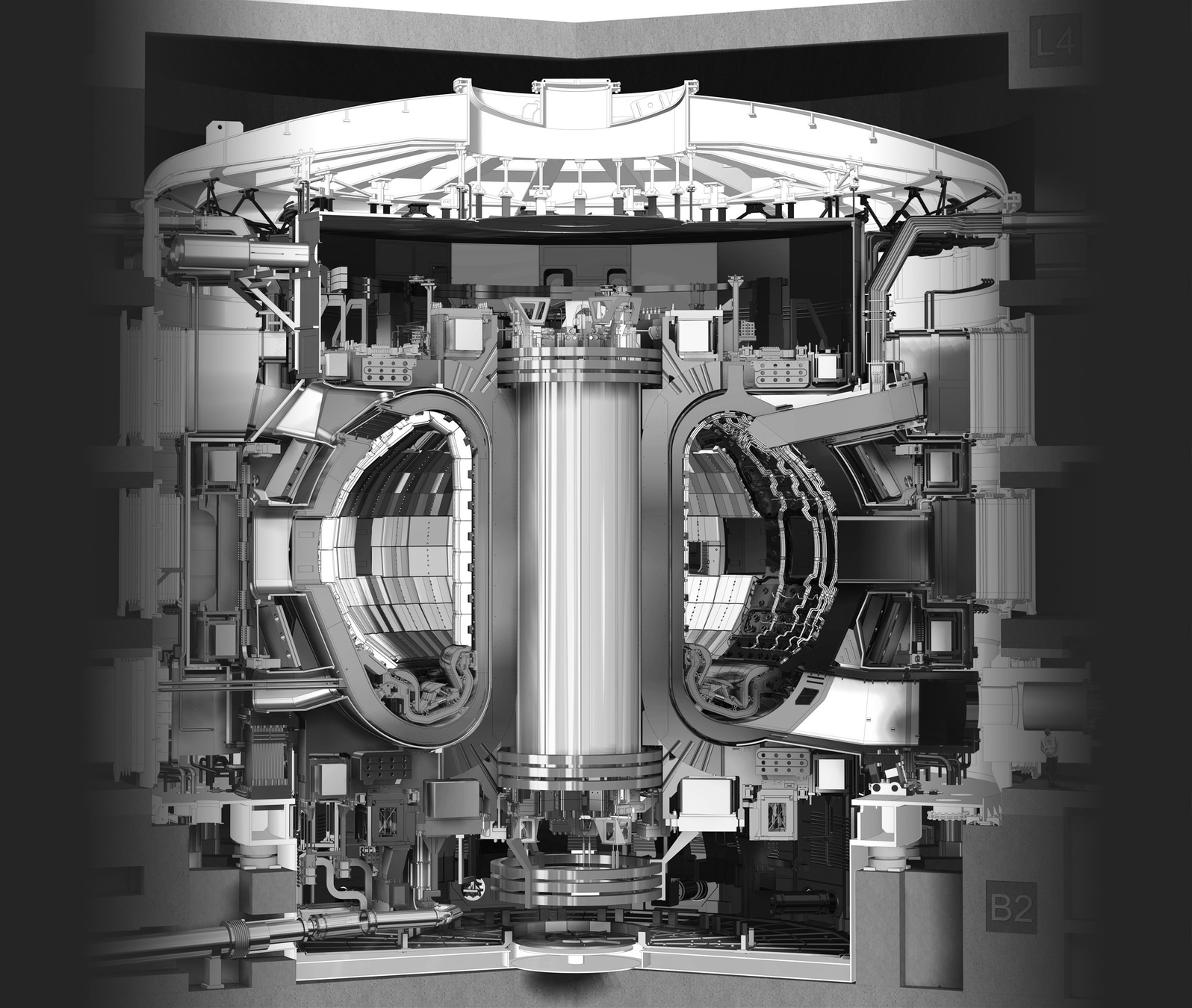
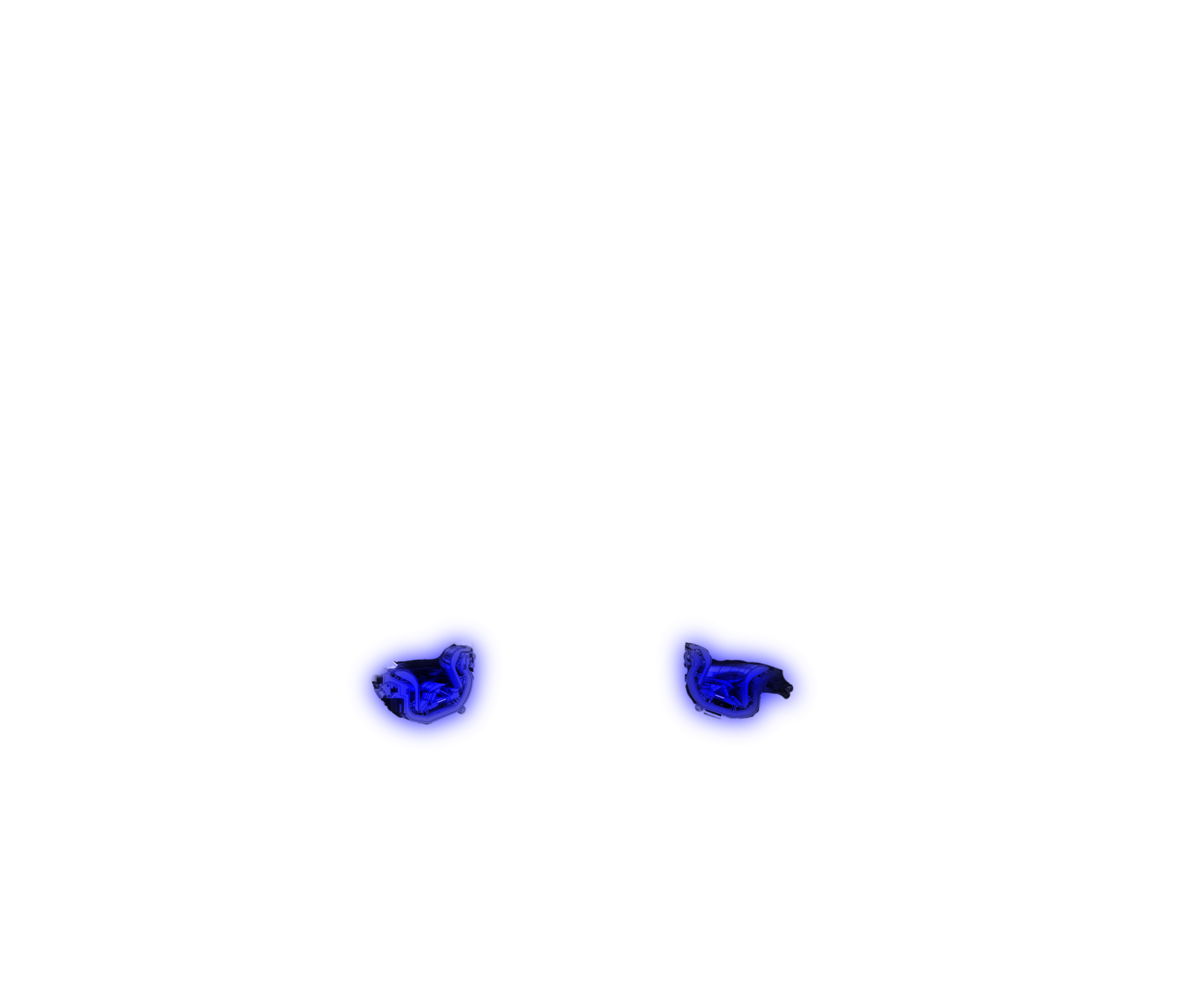
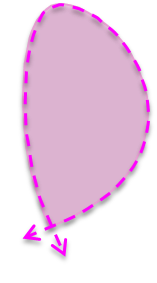
Why should we care?
T is rare
T is expensive
€£$
Material embrittlement
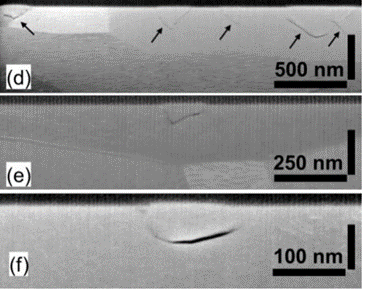
Gao et al, Nucl Fusion (2019)
What's the T inventory in the ITER divertor?
Does it remain within safety limits?
What's the influence of Helium?
T is radioactive
☢
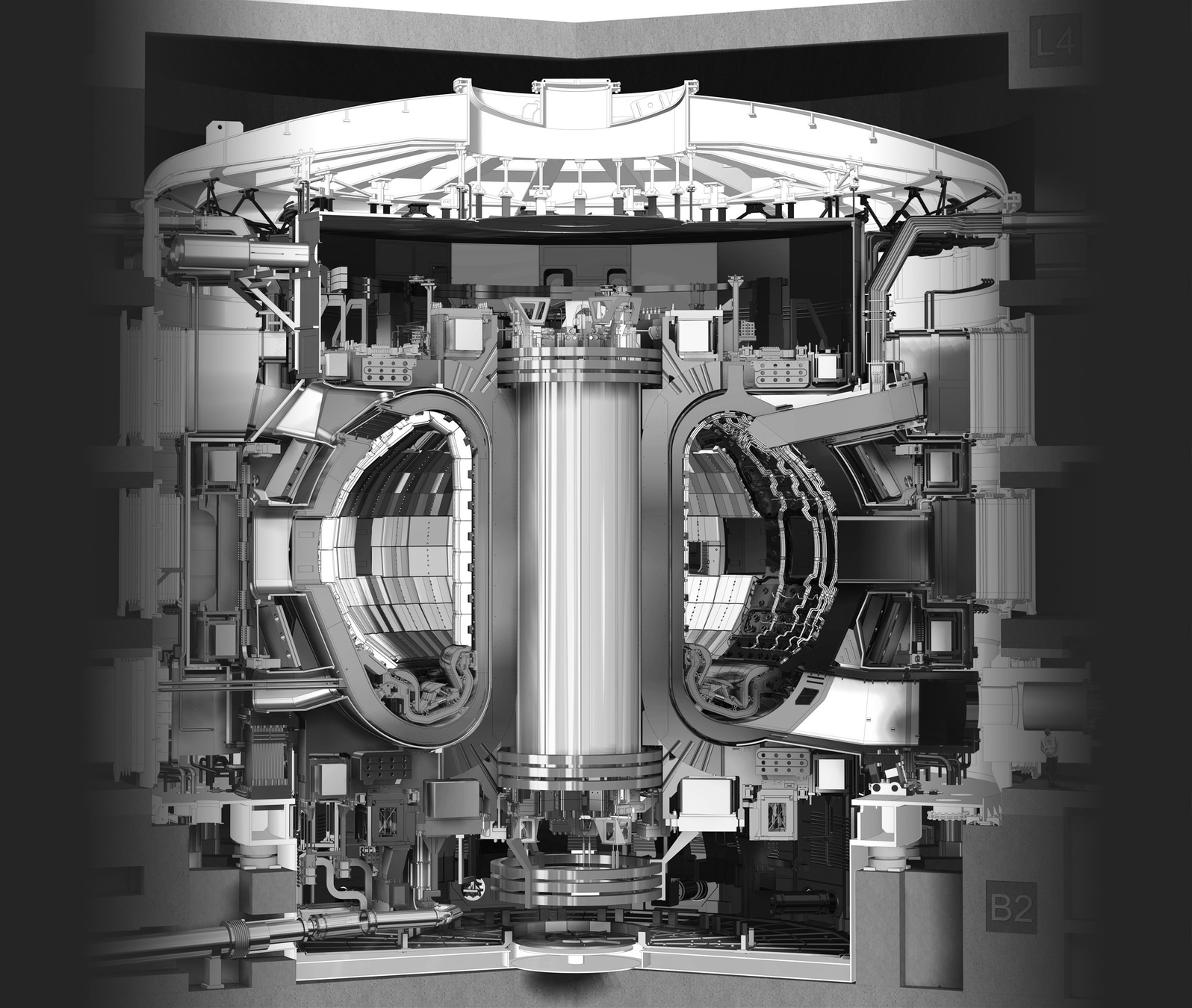
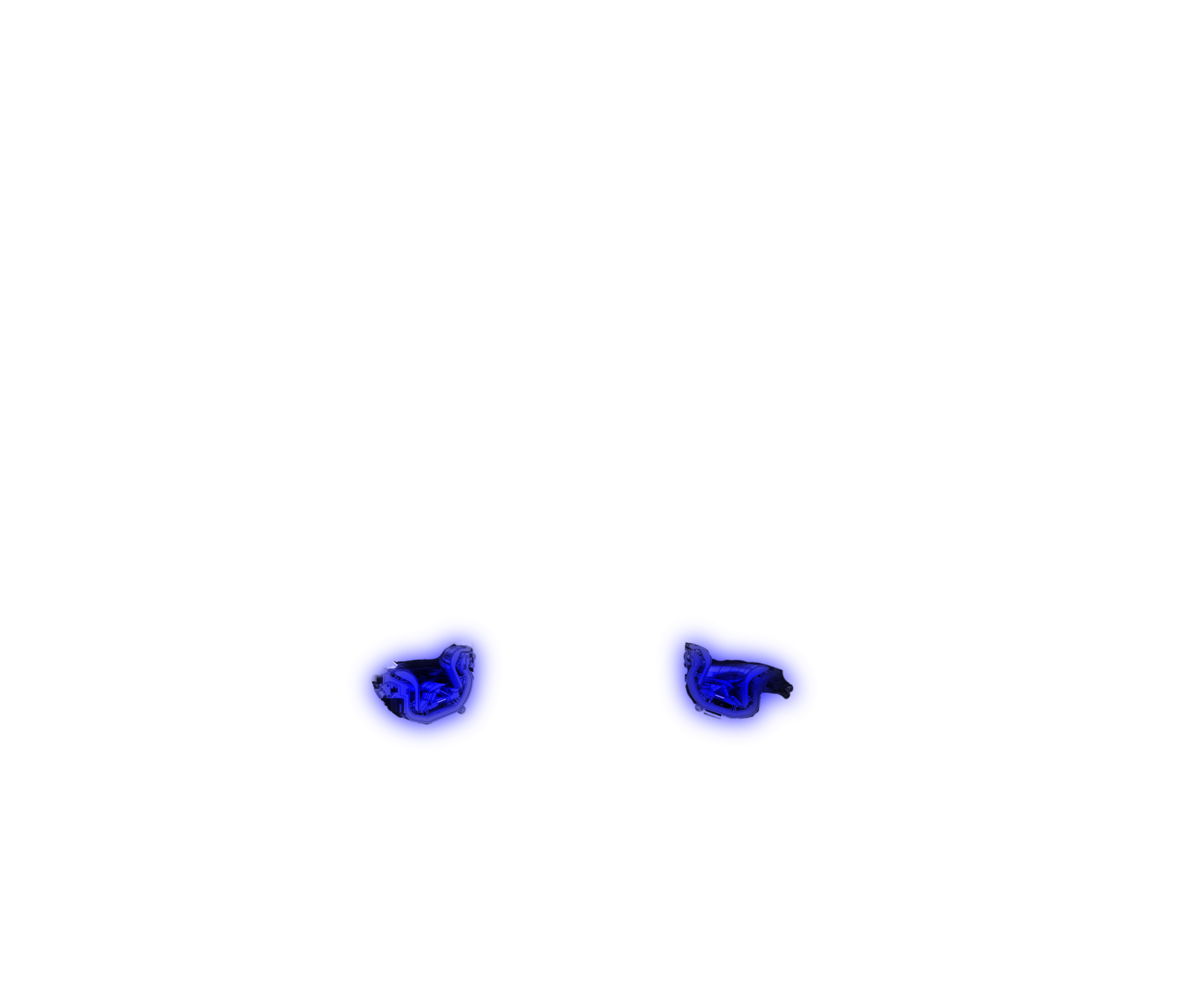
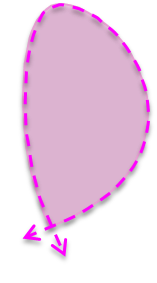
Why should we care?
T is rare
T is expensive
£
Material embrittlement

T is radioactive
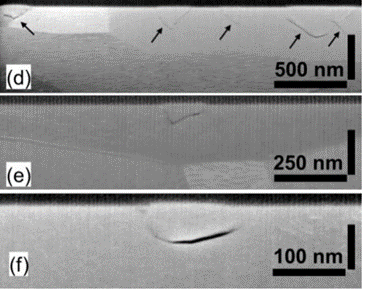
Gao et al, Nucl Fusion (2019)
What's the T inventory in the ITER divertor?
Does it remain within safety limits?
What's the influence of Helium?
Material embrittlement
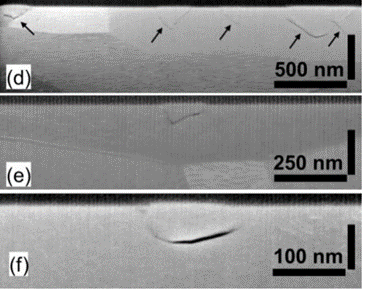
Gao et al, Nucl Fusion (2019)
Fuel recycling
£
☢
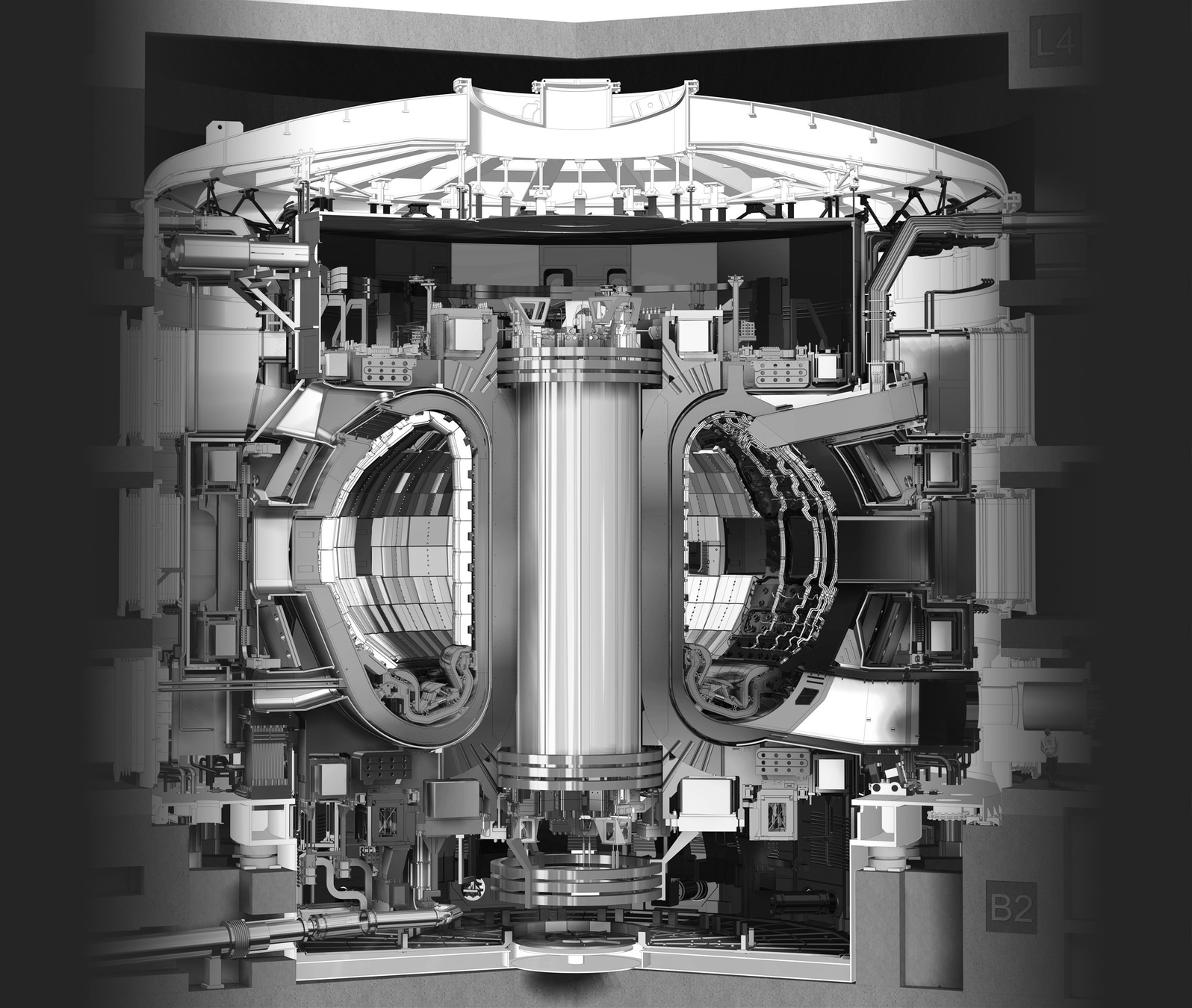
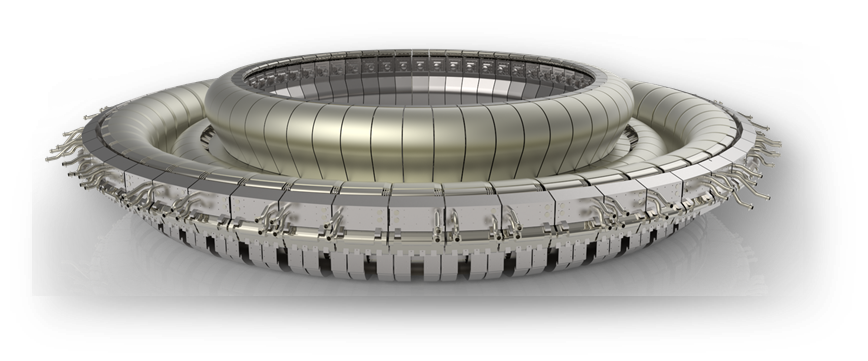
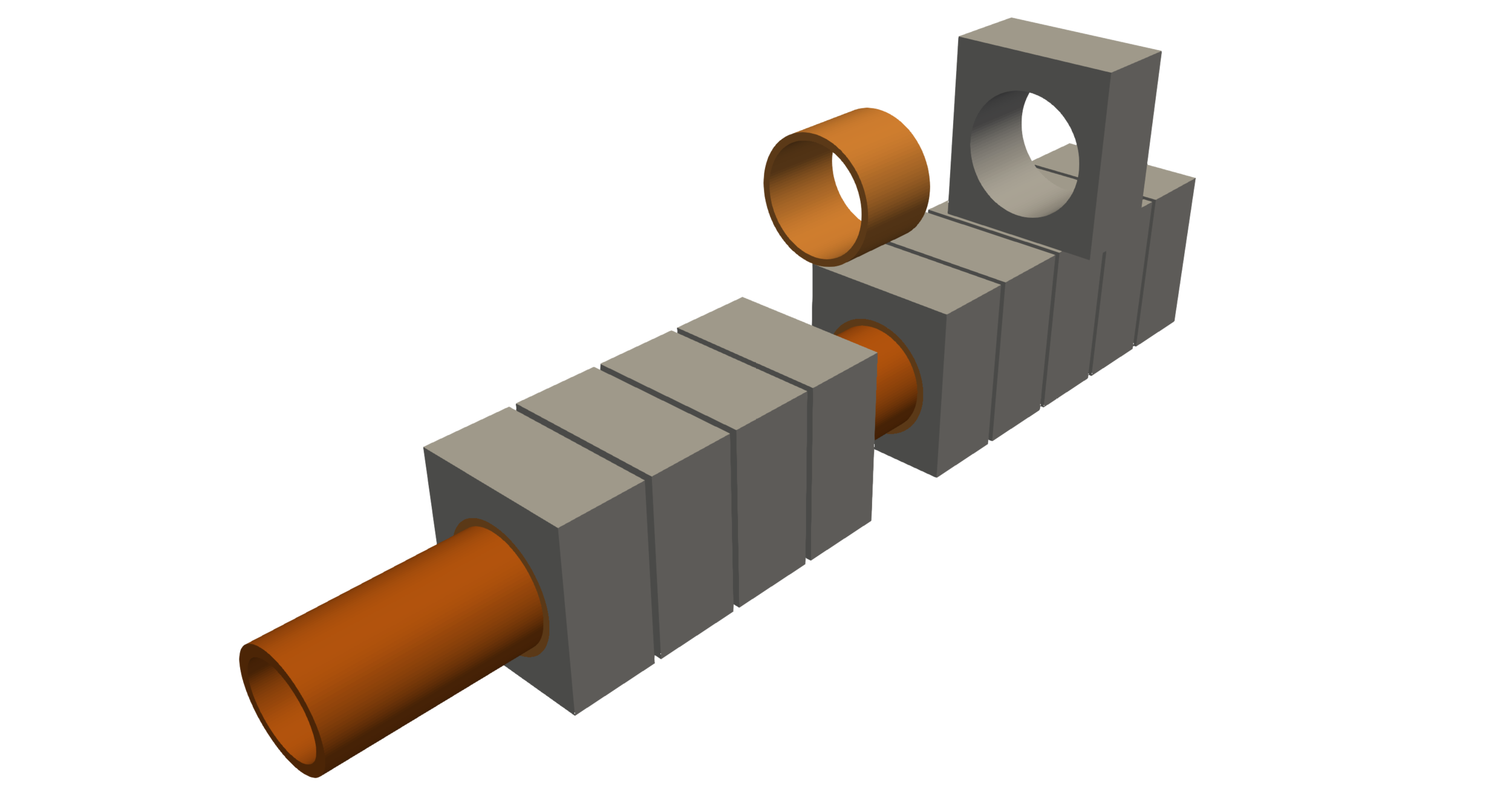
W
Cu
CuCrZr
Monoblocks
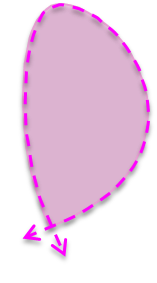
W
Cu
CuCrZr
Monoblock
Top surface exposed to extreme fluxes (particle, heat)
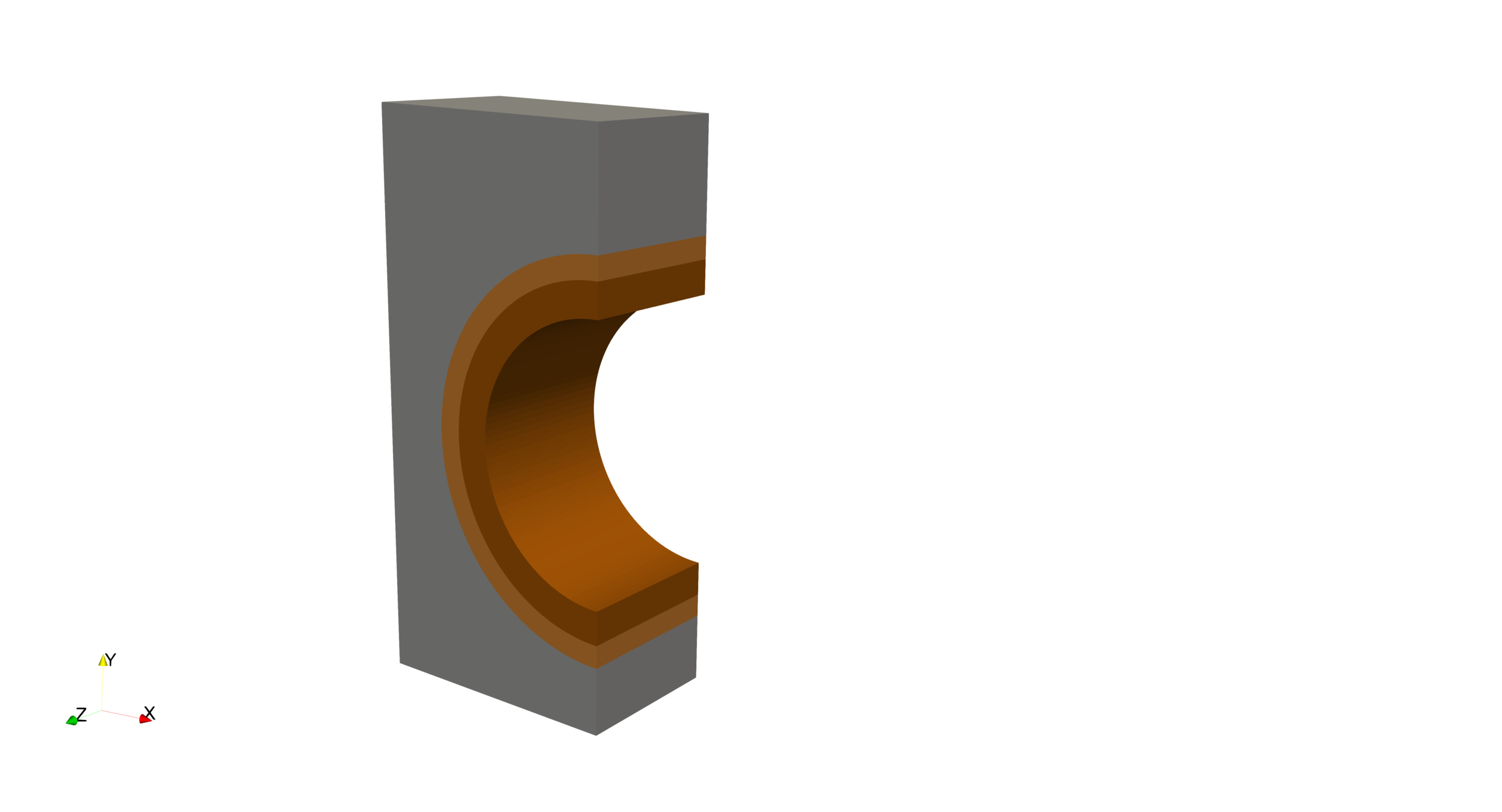
Pressurised water convection
14 mm
Outline
- Assessment of the tritium inventory in the ITER divertor
- Models & tools
- Monoblocks
- Divertor
- Influence of helium impurities
- Helium bubble model
- Interactions with hydrogen transport
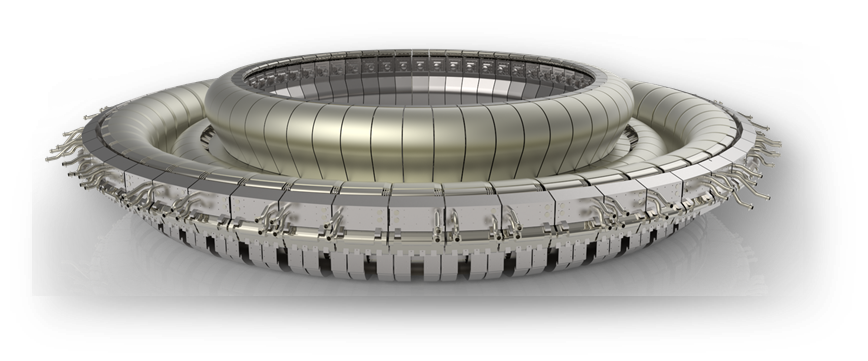
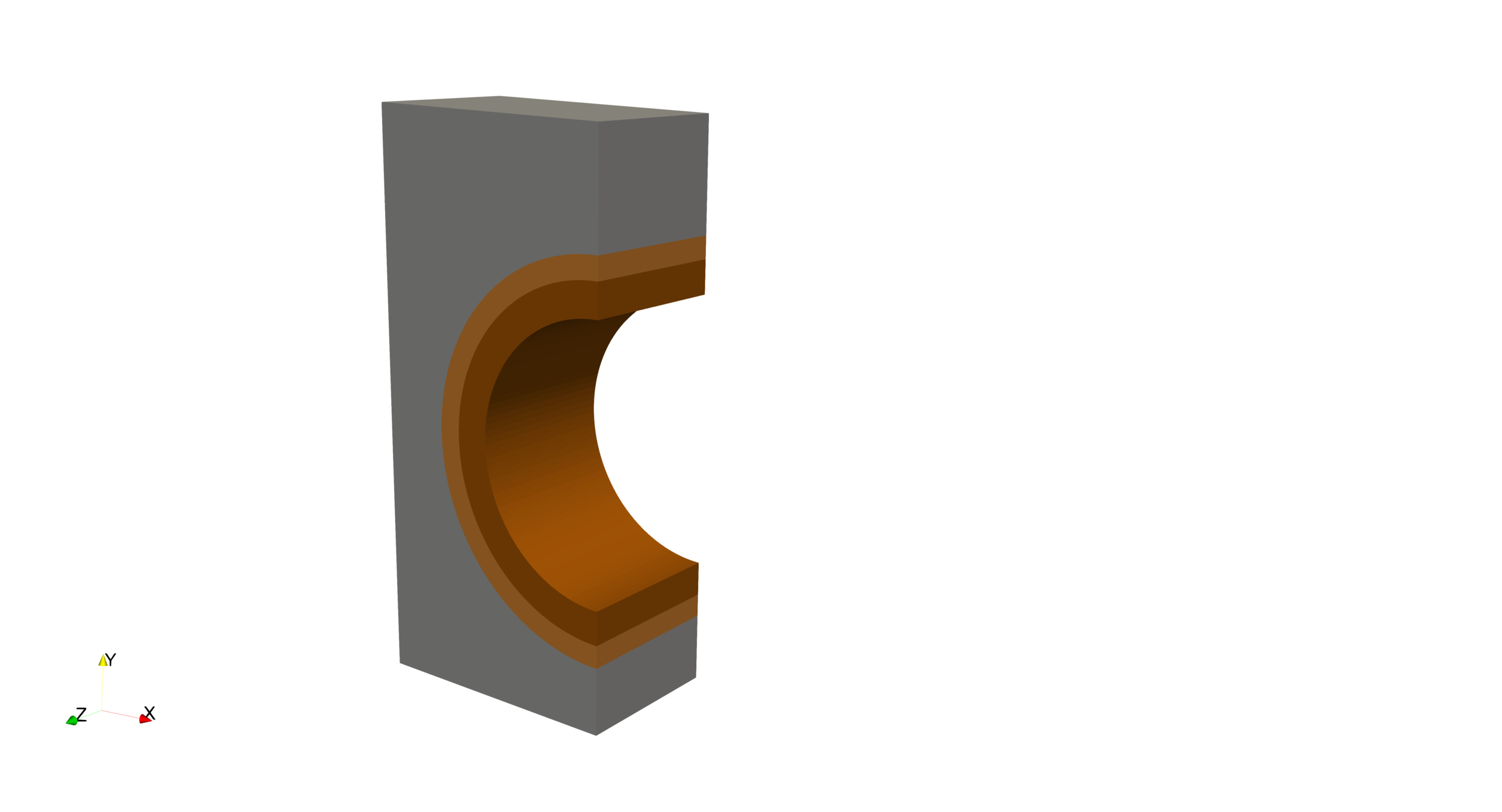
Models and tools
Hydrogen transport in metals
H transport equations
Fick's law
H transport equations
concentration of mobile hydrogen
Fick's law
H transport equations
concentration of mobile hydrogen
diffusion coefficient
Fick's law
H transport equations
McNabb & Foster
H transport equations
concentration of trapped hydrogen
McNabb & Foster
H transport equations
trap concentration
concentration of trapped hydrogen
McNabb & Foster
H transport equations
trap concentration
concentration of trapped hydrogen
McNabb & Foster
trapping rate
detrapping rate
H transport equations
Conservation of chemical potential at interfaces
Material 1
Material 2
cm
S: solubility of H in the material
H transport equations
T : Temperature (K)
Thermally activated coefficients
D=D0exp(−ED/kBT)
ki=k0exp(−Ek/kBT)
pi=p0exp(−Ep/kBT)
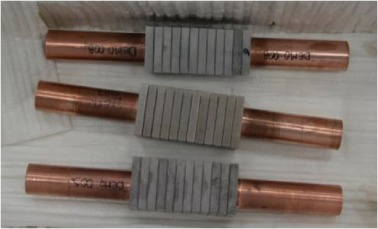
1800 °C
300 °C
20 MW/m2
kB: Boltzmann constant
We also need the heat equation
thermal conductivity
heat capacity
density
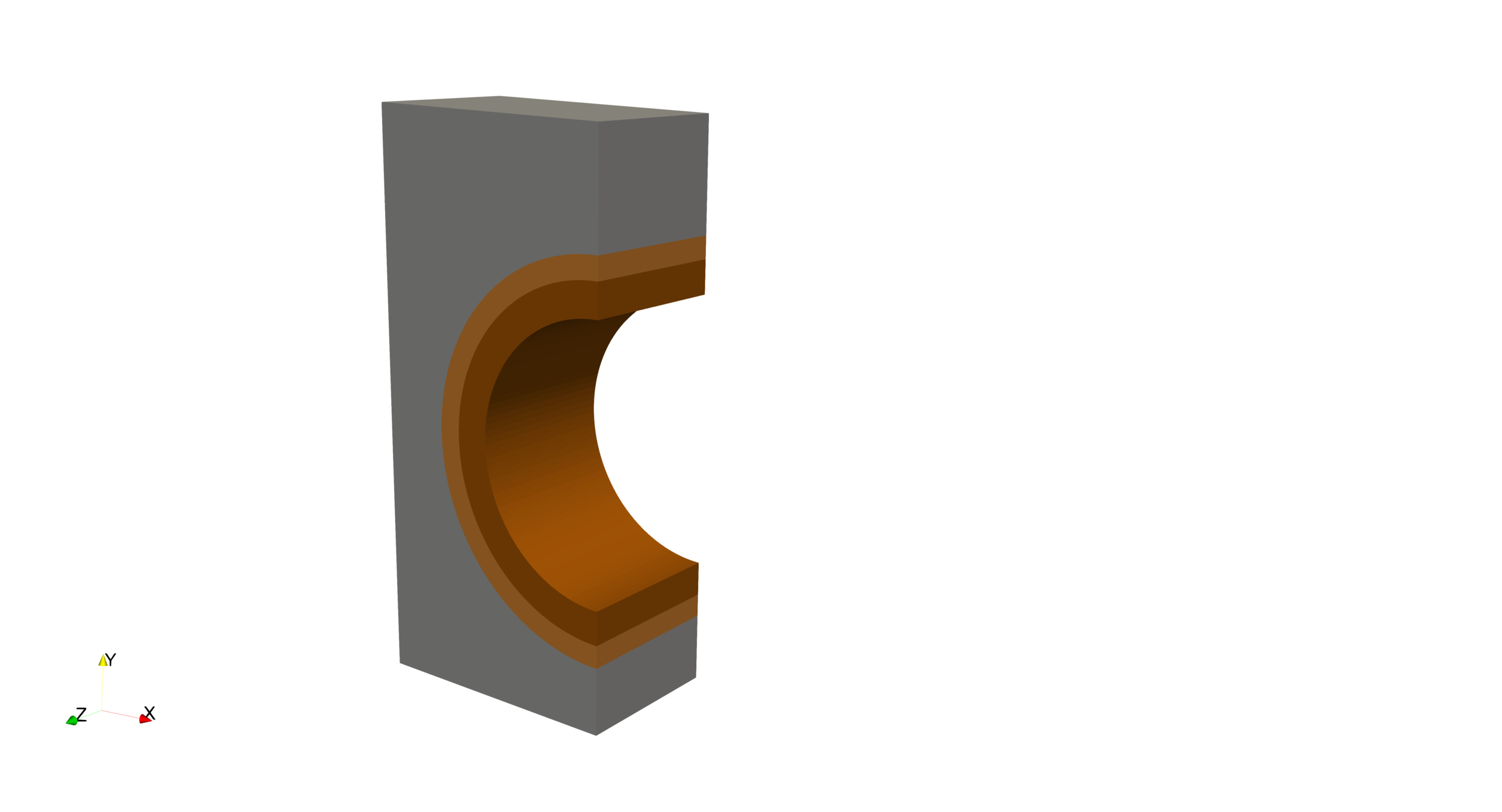
Wishlist
- Multi-materials
- Multi-dimensional
- Hydrogen transport
- Heat transfer
1D H transport | 2D/3D | Multi-material | Heat transfer | |
---|---|---|---|---|
TMAP7 | ✓ | ✓ | ||
HIIPC | ✓ | ✓ | ||
CRDS | ✓ | |||
MHIMS | ✓ | |||
TESSIM | ✓ | ✓ | ||
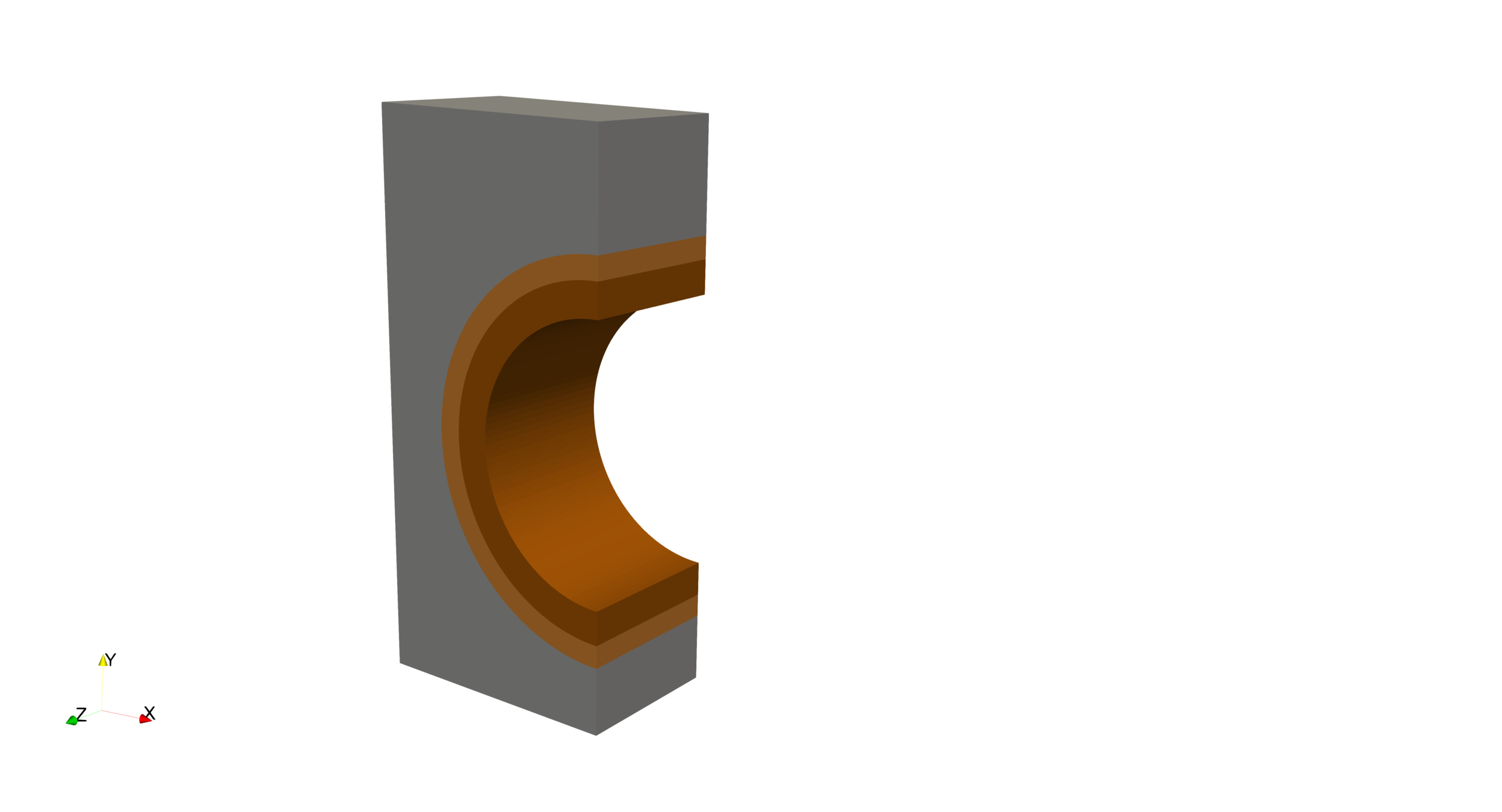
Wishlist
- Multi-materials
- Multi-dimensional
- Hydrogen transport
- Heat transfer
1D H transport | 2D/3D | Multi-material | Heat transfer | |
---|---|---|---|---|
TMAP7 | ✓ | ✓ | ||
HIIPC | ✓ | ✓ | ||
CRDS | ✓ | |||
MHIMS | ✓ | |||
TESSIM | ✓ | ✓ | ||
FESTIM | ✓ | ✓ | ✓ | ✓ |
FESTIM implements these models
FESTIM
Finite element
H transport
Heat transfer
Complex geometries
The finite element method
Steady state weak formulation:
Transient weak formulation:
In FESTIM
Type of finite elements:
- P1 (CG1) for cm
- P1 or DG1 for ct,i
FESTIM: a code verified & validated
Experimental validation
Analytical verification
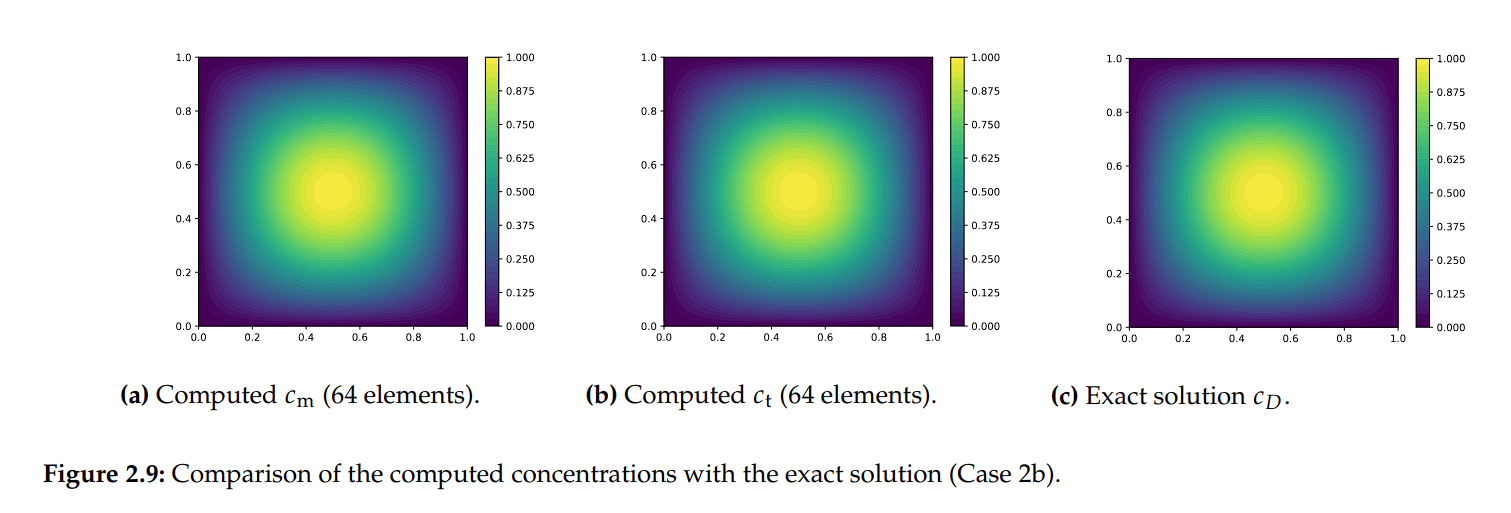
Exact
Computed concentration
Parametric optimisation technique
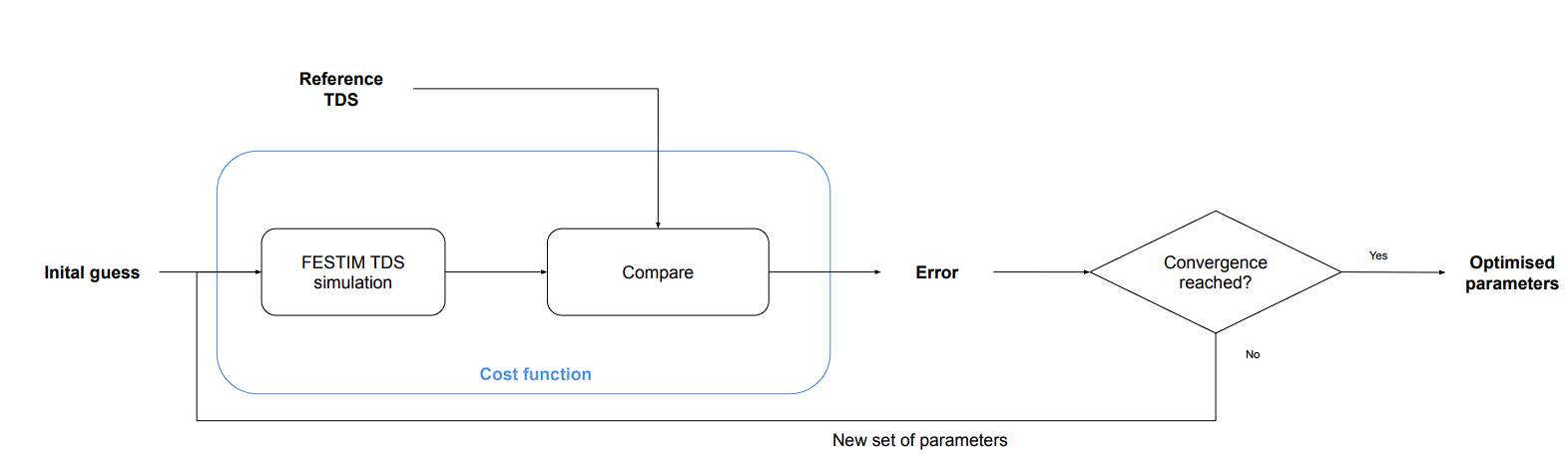
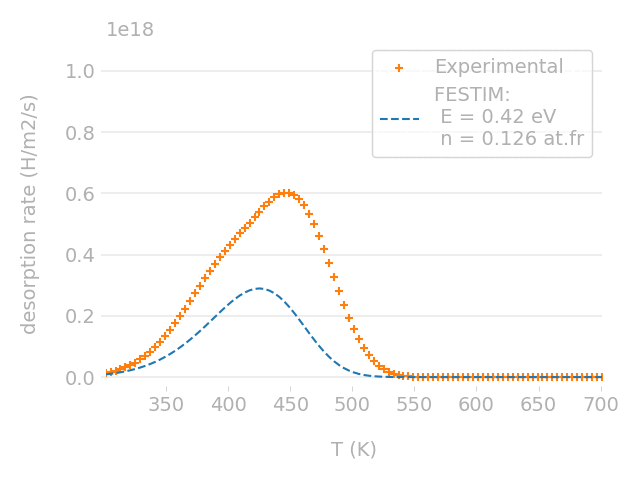
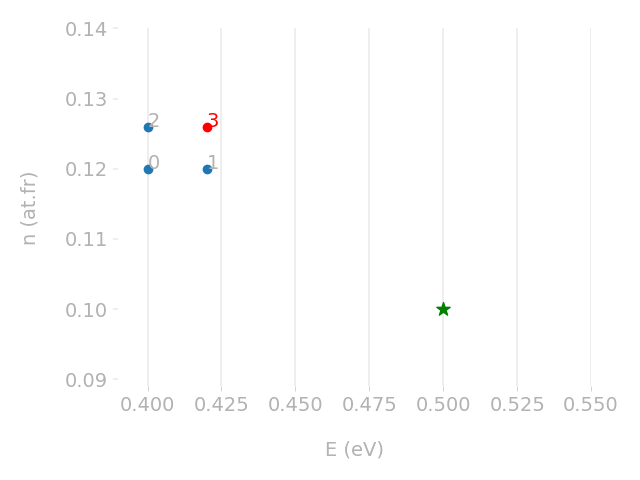
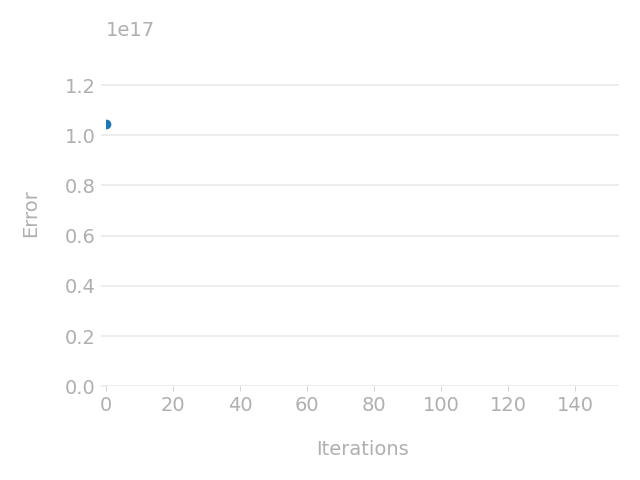
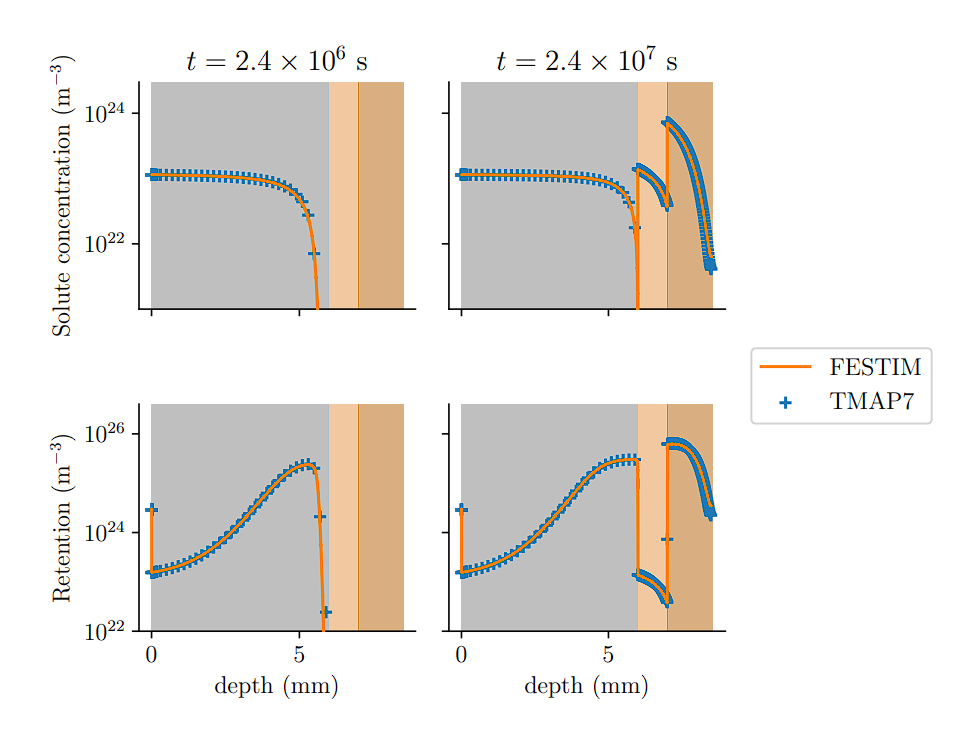
Cross-check with TMAP7
FESTIM main features
Physics
- Hydrogen diffusion
- Trapping (McNabb & Foster)
- Heat transfer
- Conservation of chemical potential
- Soret effect
Dimension
✅ 1D
✅ 2D
✅ 3D
Boundary conditions
- Imposed concentration/temperature
- Hydrogen/heat flux
- Recombination flux
- Dissociation flux
- Convective heat flux
- Sievert's law
- Plasma implantation approx.
- ...
Traps
- Time/space dependent densities
- Extrinsic traps
FESTIM: an open-source code
✅ More transparency
✅ More collaborations
✅ More flexibility
Automated documentation
FESTIM workshop
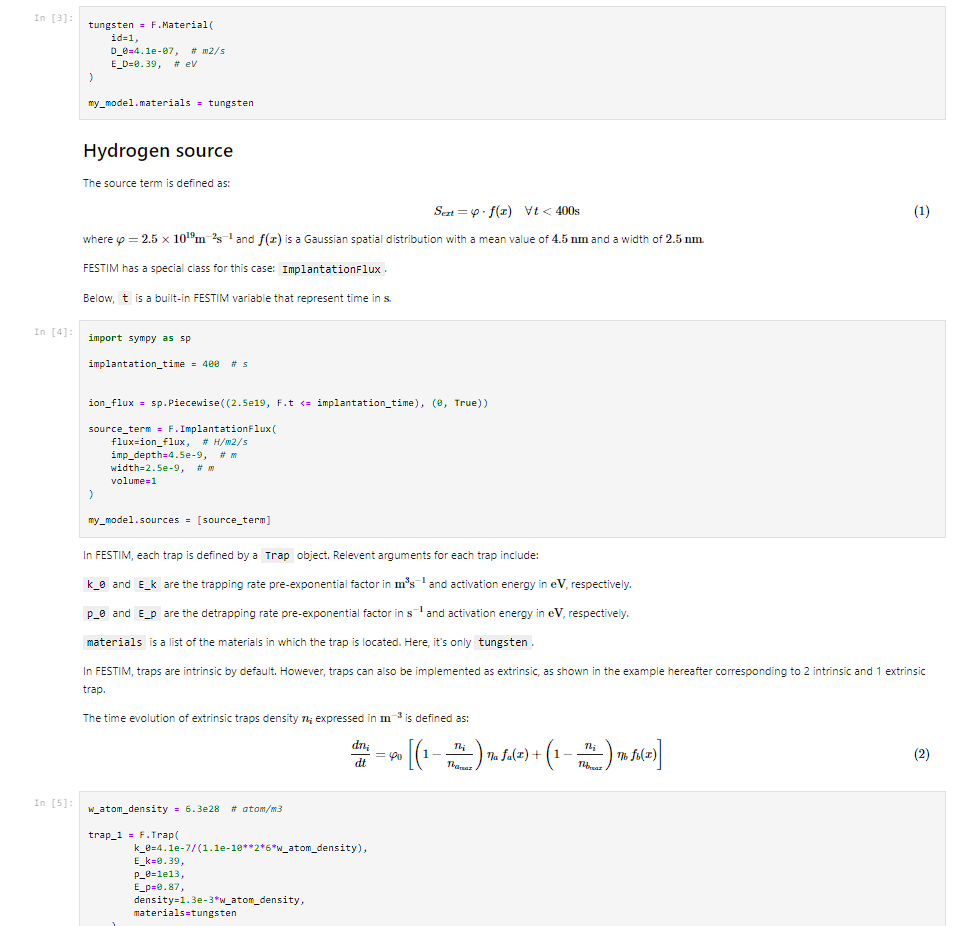
A FESTIM course to learn how to run H transport simulations
Monoblock simulations
FESTIM model
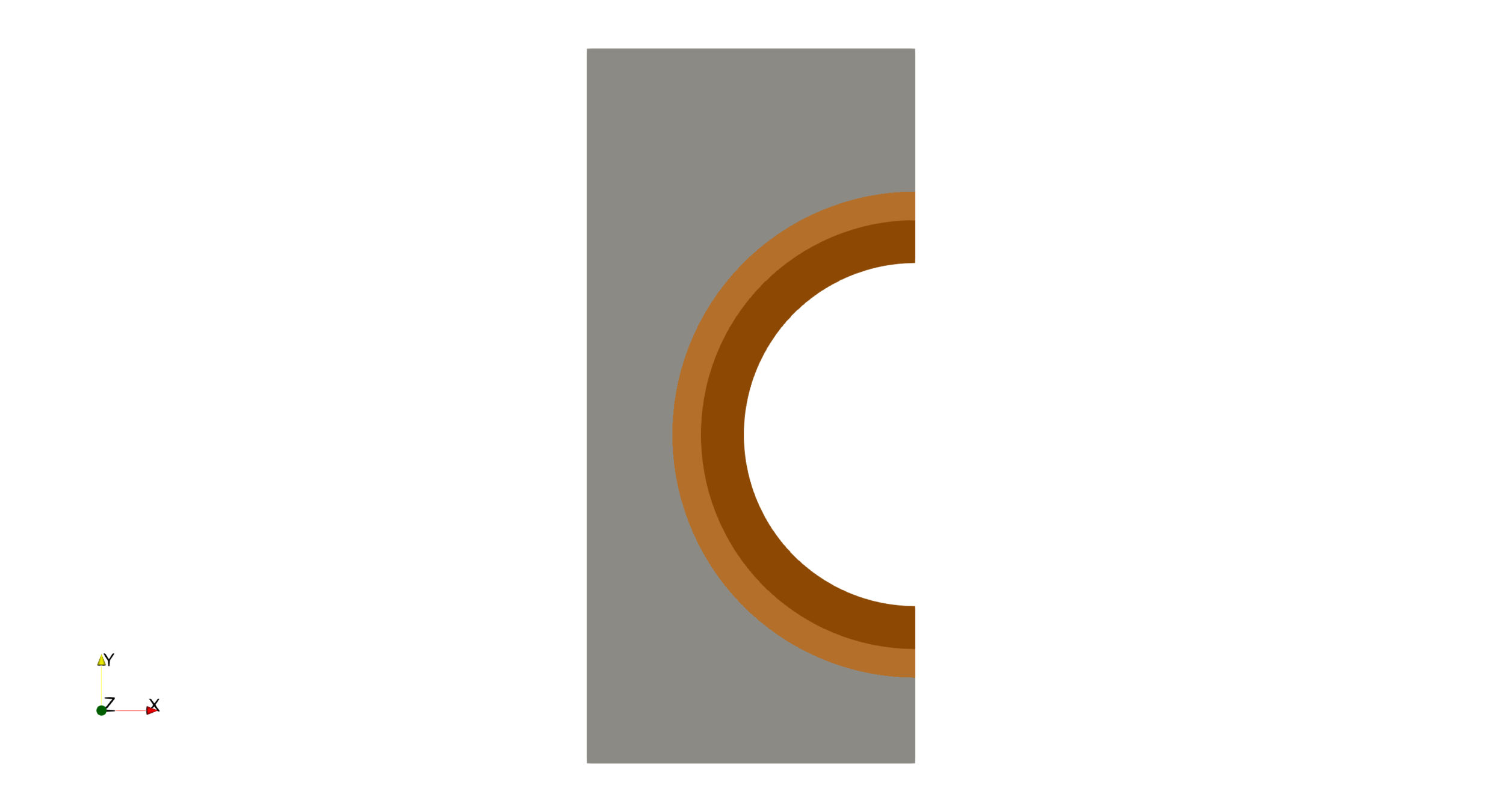
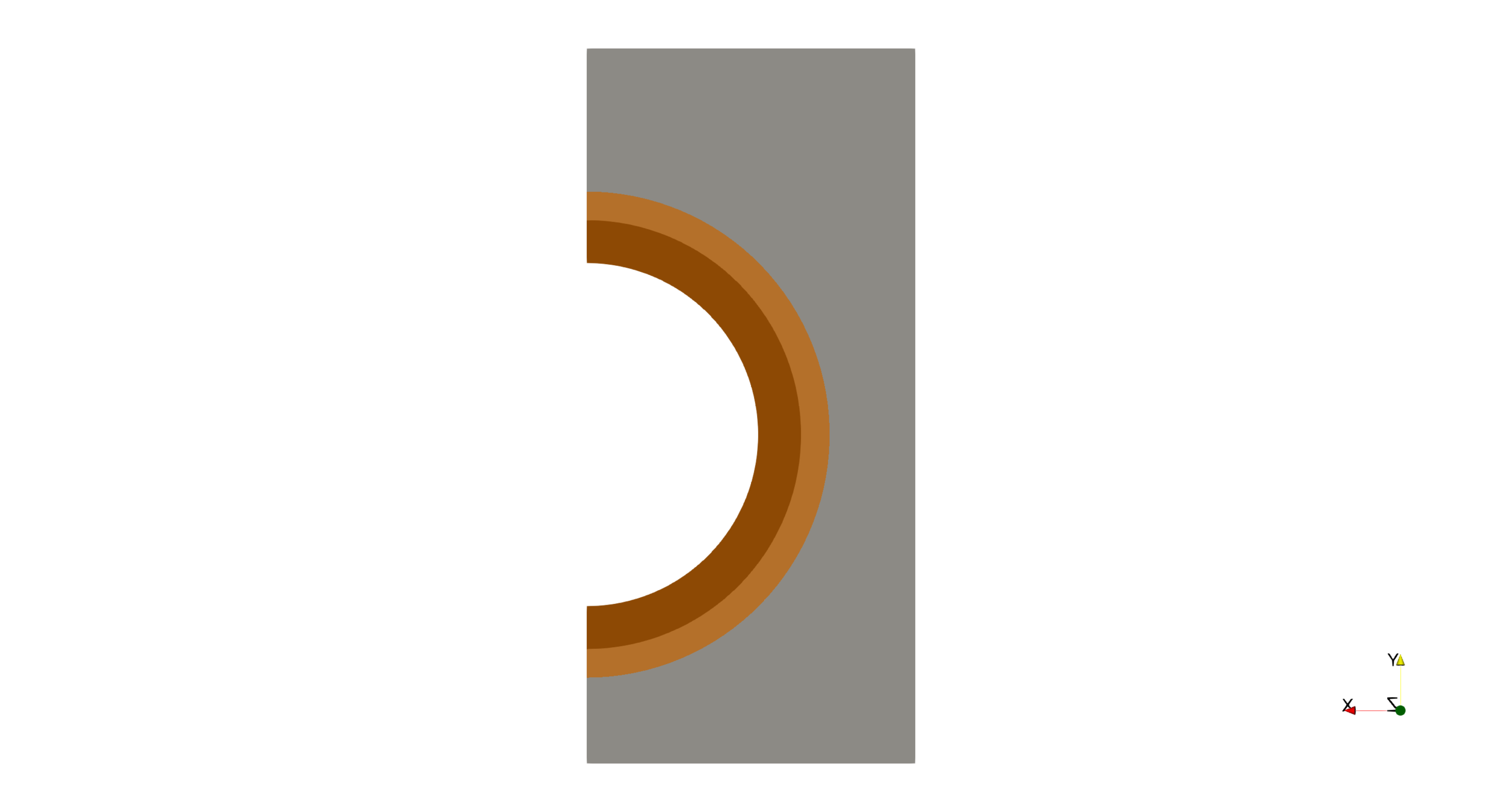
Heat flux φheat
Imposed concentration
Convection
H recombination
Conservative assumptions:
- 2D
- Continuous exposure
- No desorption on gaps
Influence of mechanical fields neglected
Boundary conditions
Convective flux: −λ∇T⋅n=h (T−Tcoolant)
Recombination flux: −D∇cm⋅n=Kr cm2
Incident heat flux: −λ∇T⋅n=10 MW m−2
Heat transfer coefficient: h=70,000 W m−2 K−1
Coolant temperature: Tcoolant=323 K
Recombination coefficient: Kr=2.9×10−14 exp(−1.92/kBT) (m4 s−1 )
FESTIM model
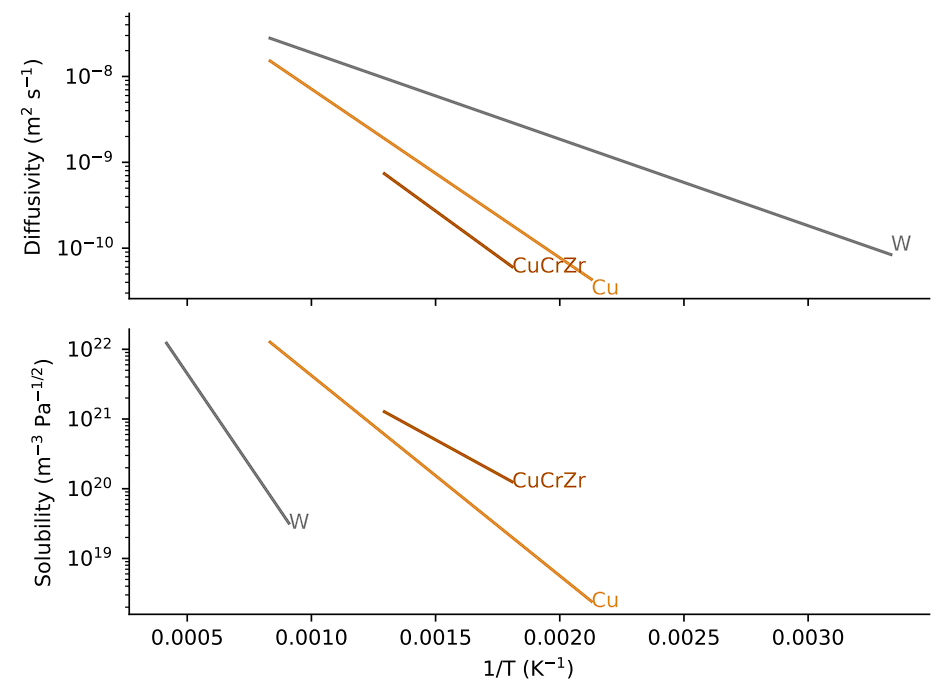
Materials properties
Trapping parameters
W | |||||
Cu | |||||
CuCrZr |
Simulation parameters
Thermal properties
W
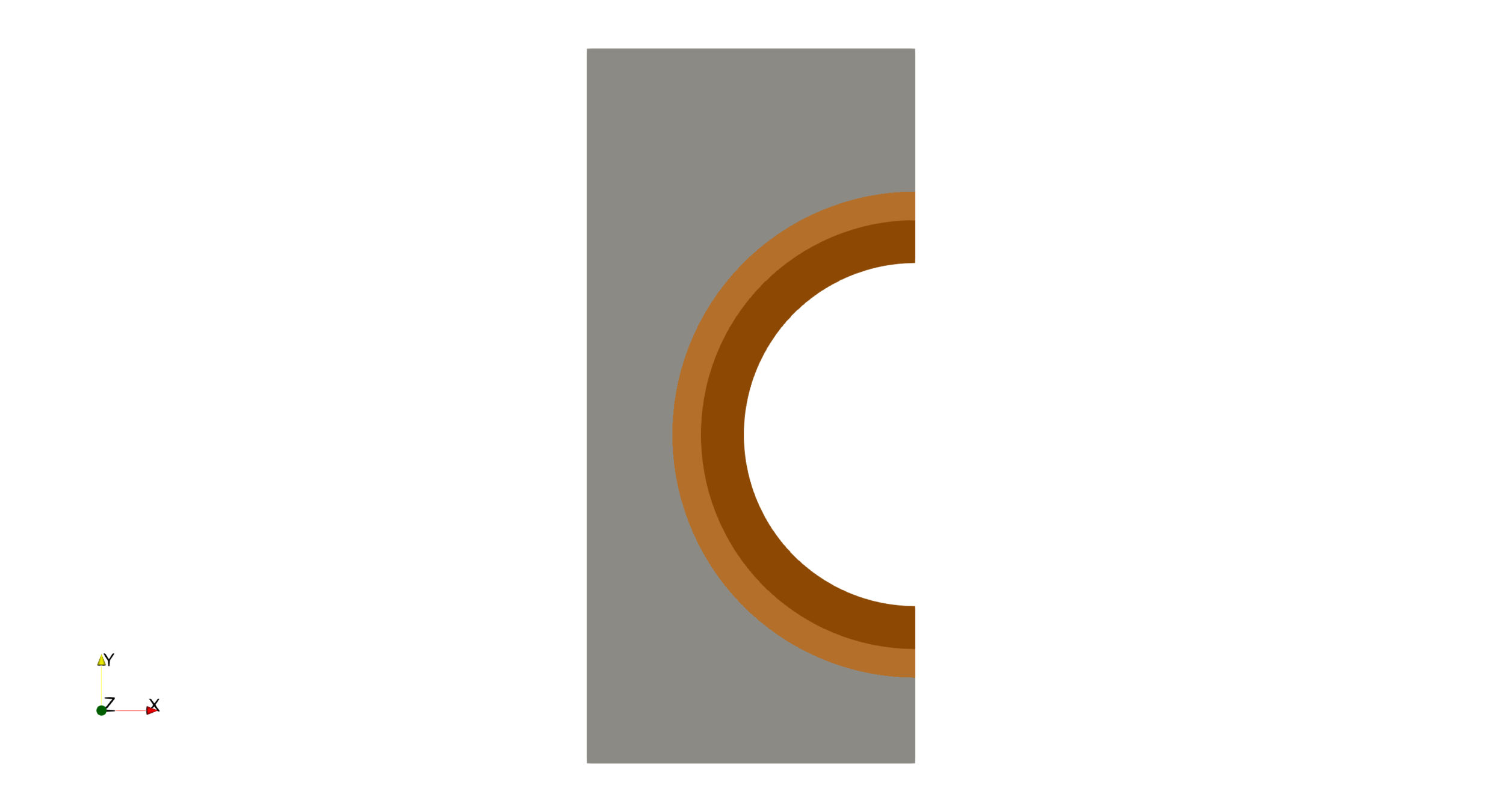
14 mm
⌀ 12 mm
1.5 mm
1 mm
Geometry
Cu
CuCrZr
- Maximum 77% difference
- Equivalent trap:
- n=n1+n2
- Ep=nEp,1 n1+Ep,2 n2
Approximations
Neglecting Trap 2
A 2D model is the best trade-off between performance and accuracy
When neglecting desorption on gaps
3D = 2D
H concentration
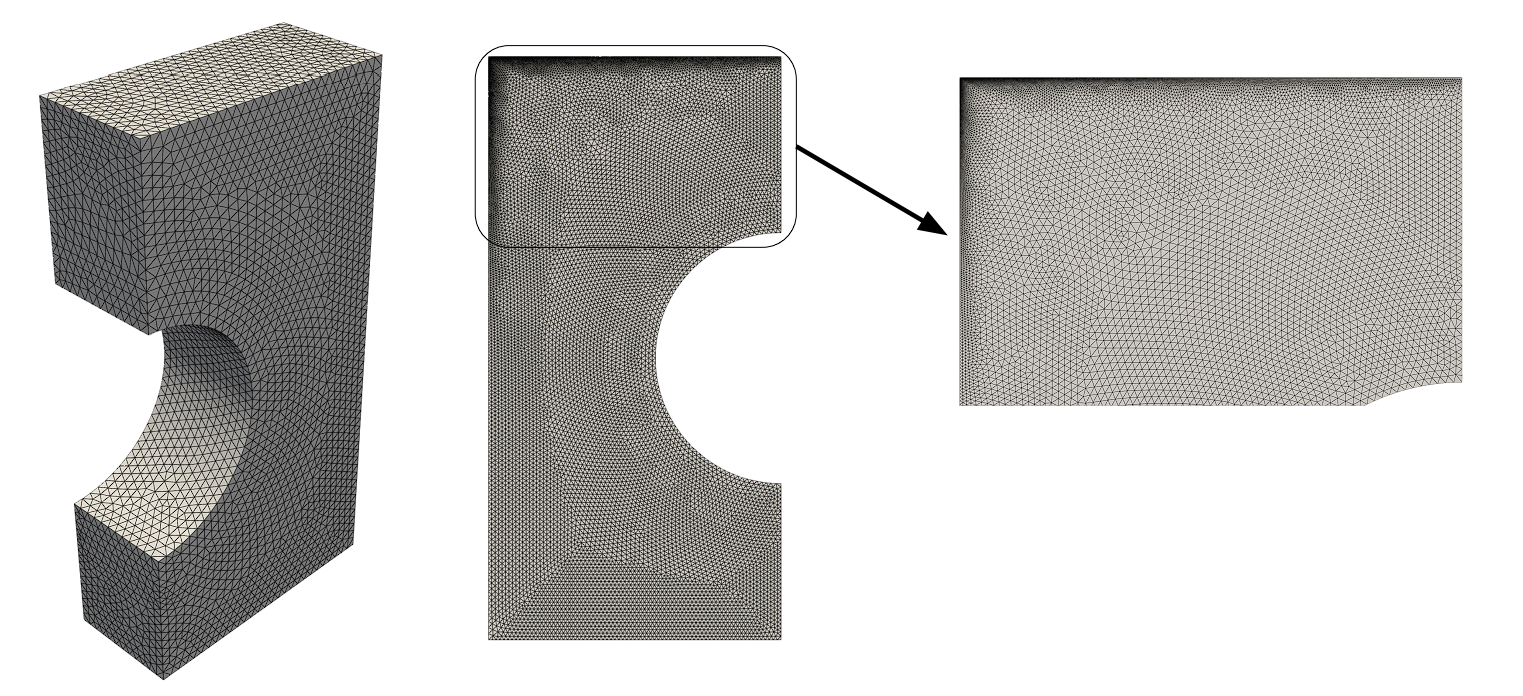
160k tetrahedrons
59k triangles
2D allows for a more refined model
ITER operations will be pulsed
Hot
Cold
The H inventory is influenced by the temperature evolution
Hot
Cold
H inventory (m−2 )
Assuming a continuous exposure allows to have a bigger stepsize
Cycling: 1500 timesteps
Continuous: 86 timesteps
H inventory (m−2 )
Low flux:
5.0 MW m−2
5.0×1021 m−2 s−1
High flux:
13 MW m−2
1.6×1022 m−2 s−1
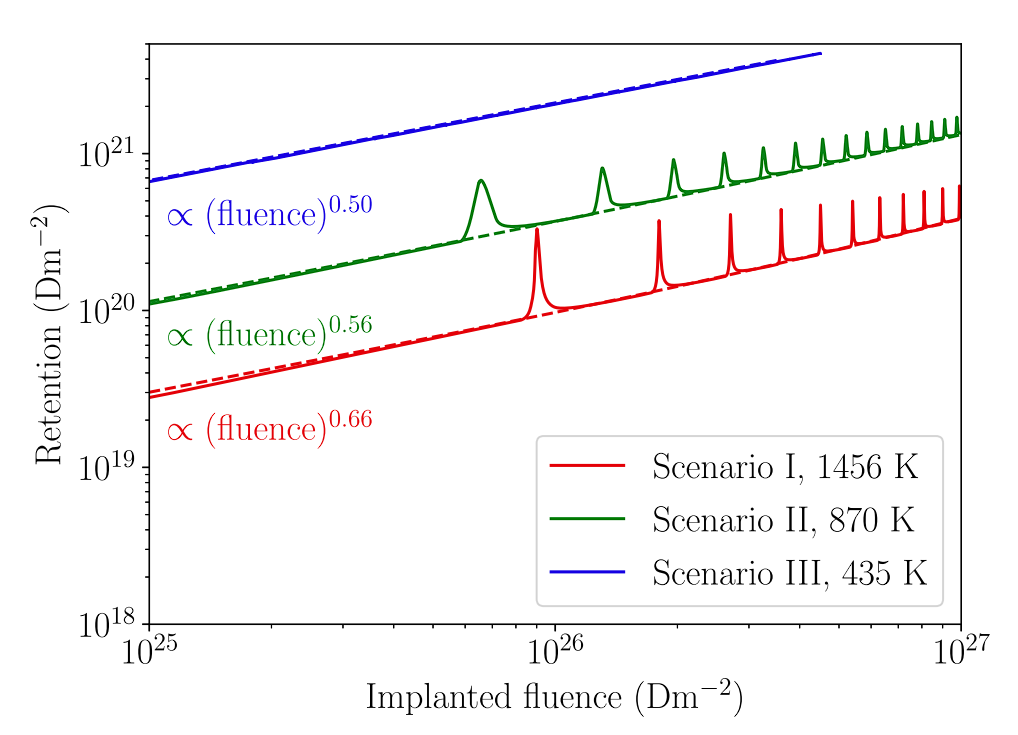
Similar results were obtained with MHIMS
Monoblock thermal response
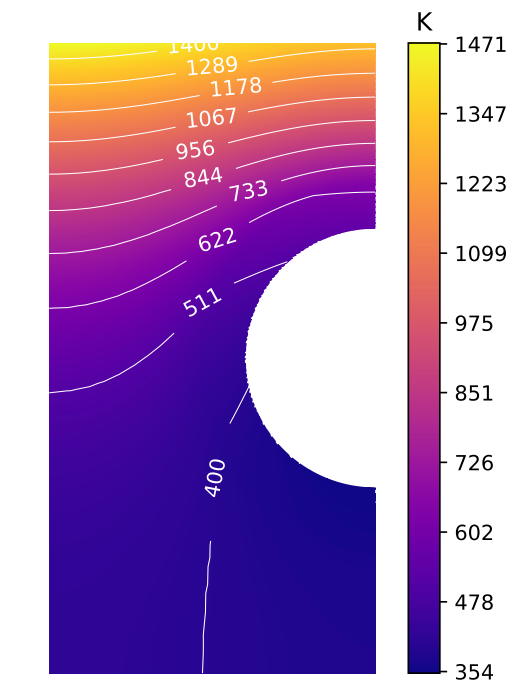
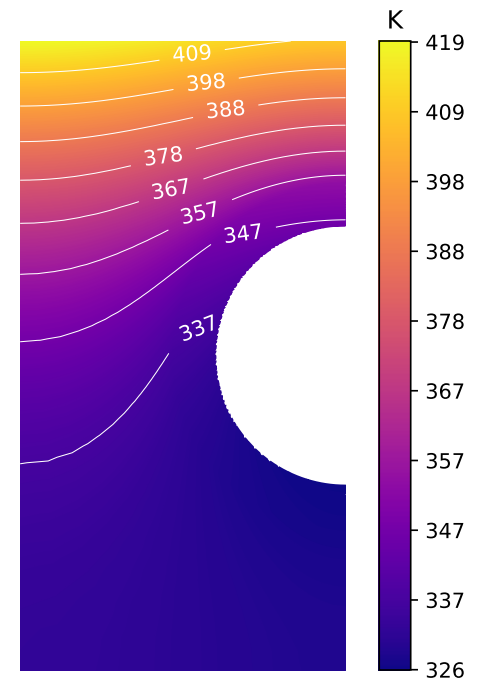
- High temperature gradient
- 2D temperature field
Monoblock thermal response
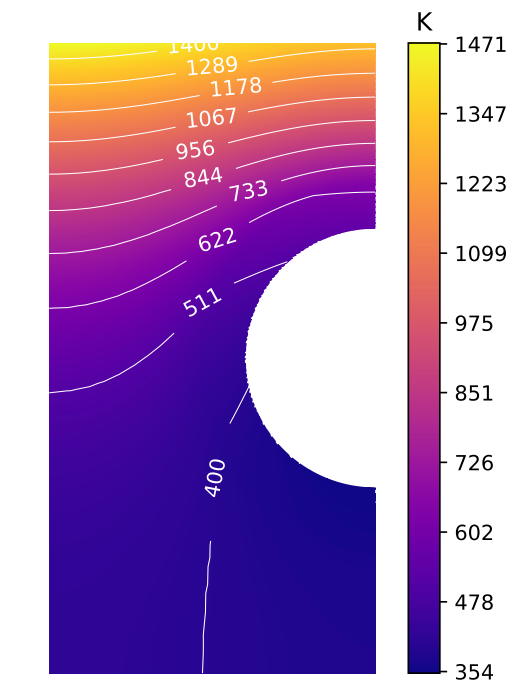
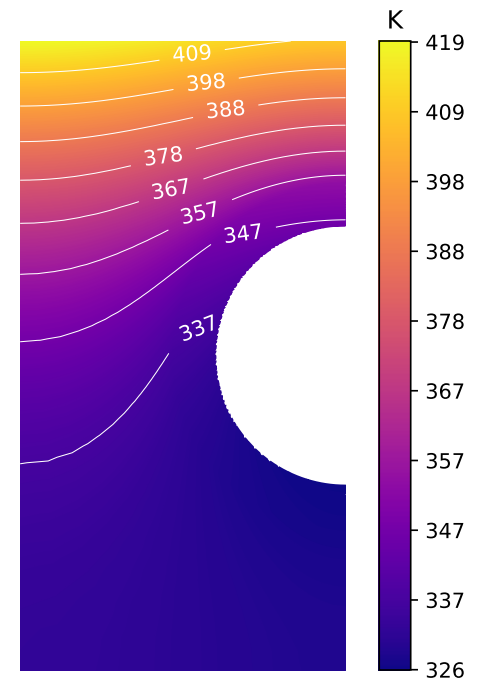
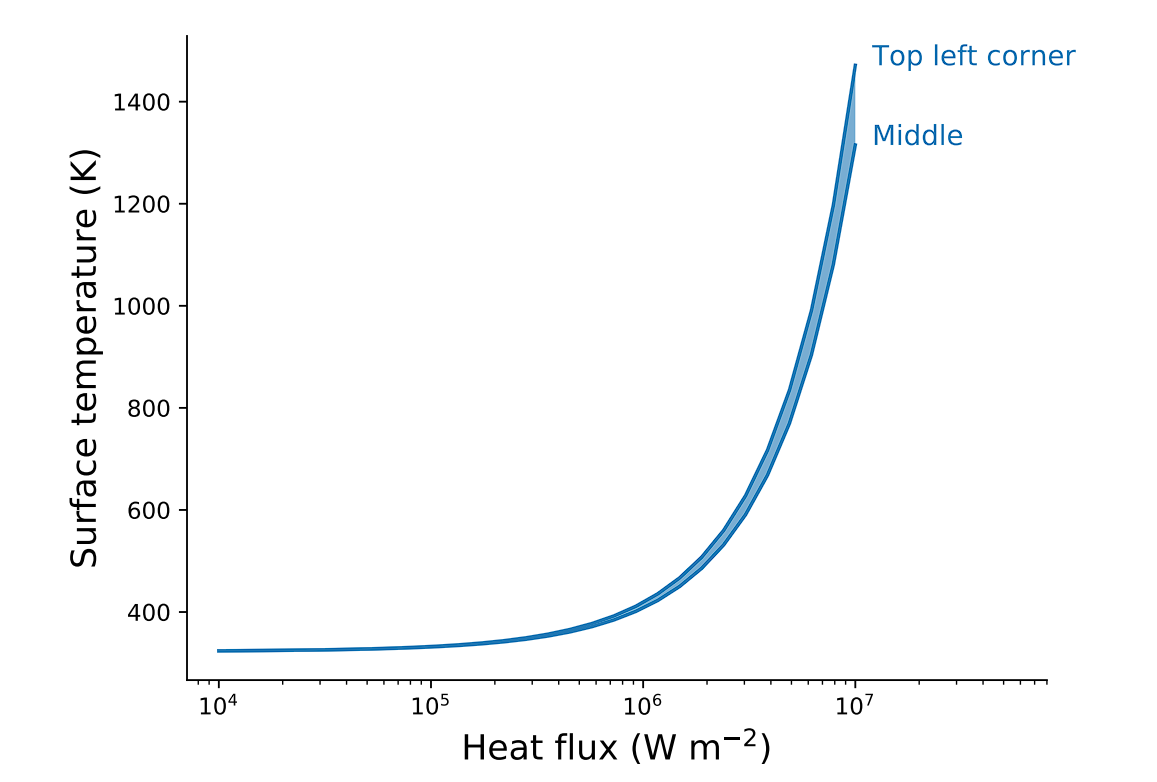
H concentration
At
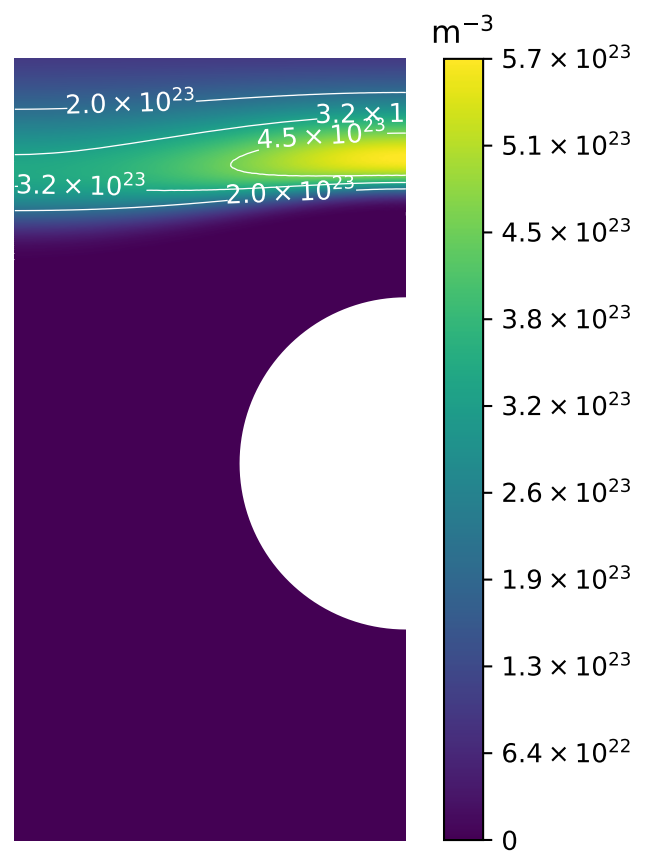
- Penetration front
- High retention zone in colder regions
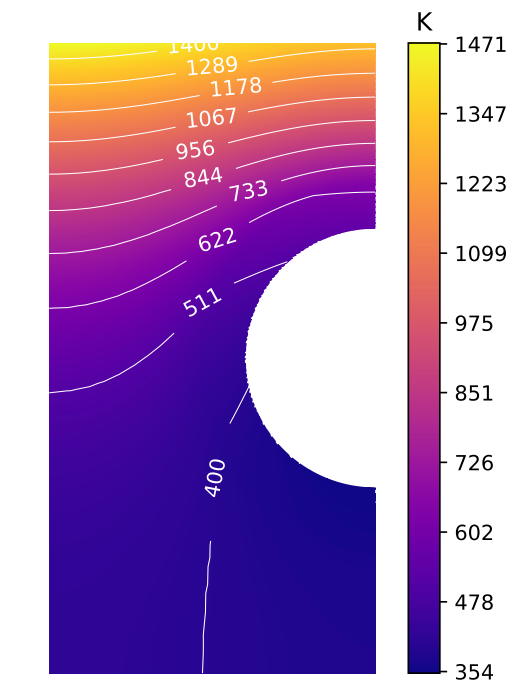
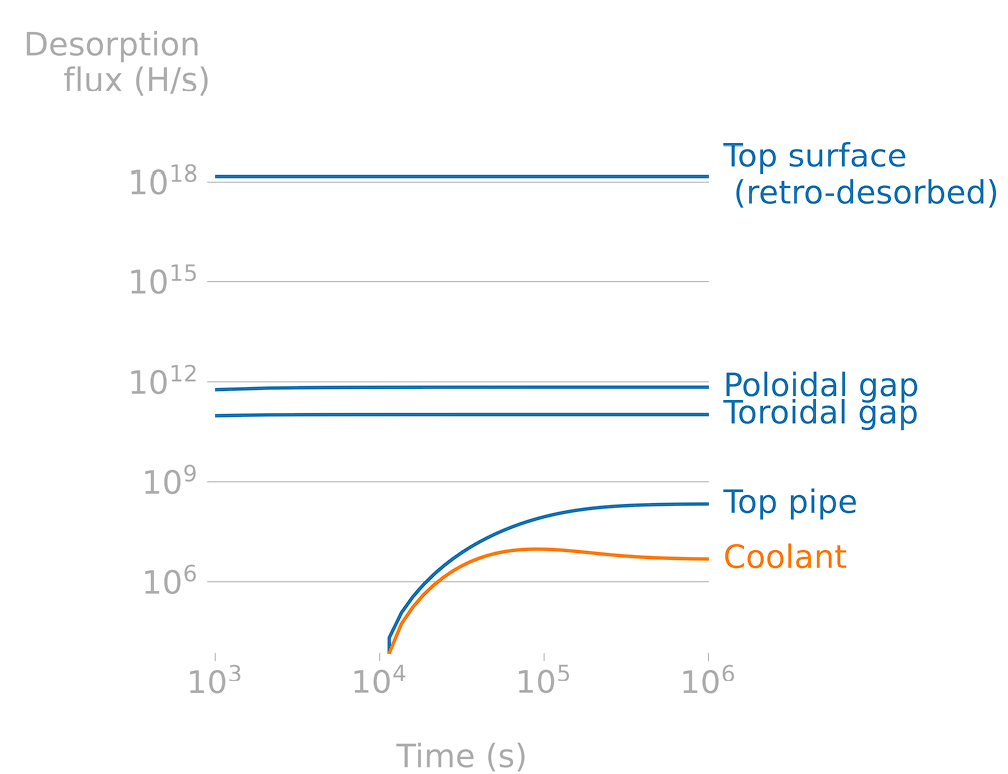
Permeation to coolant
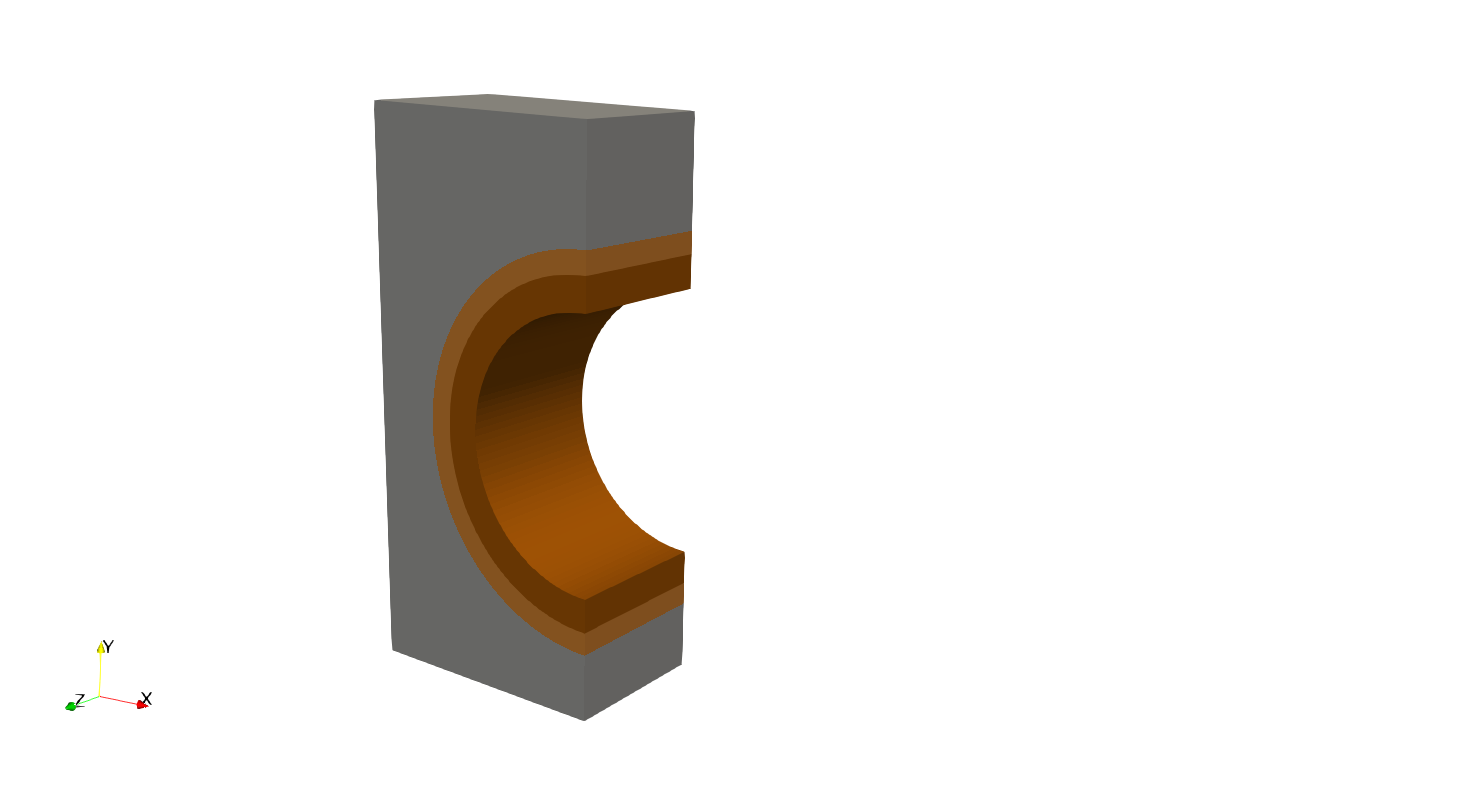
DEMO monoblock
Influence of non-instantaneous recombination
wo gap and wo recombination, independent of thickness (2D)
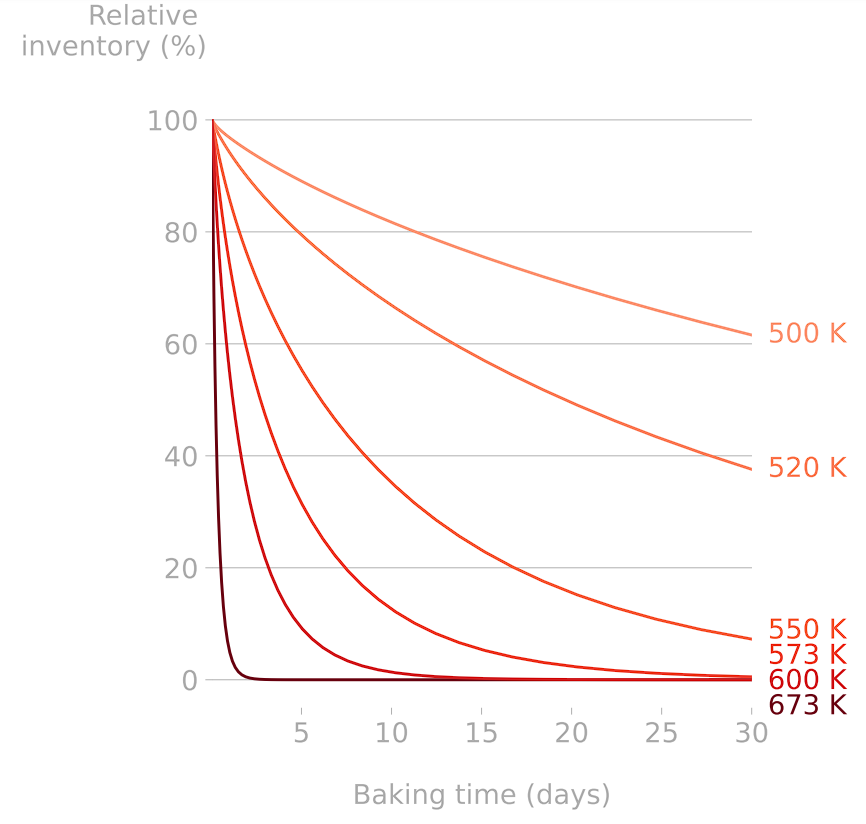
Monoblock baking
To be published
H concentration
At
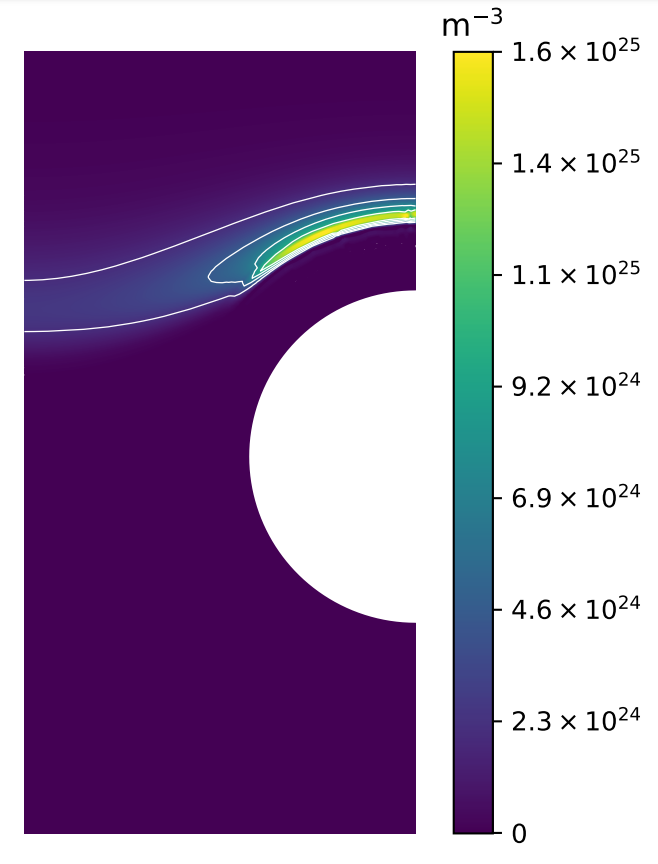
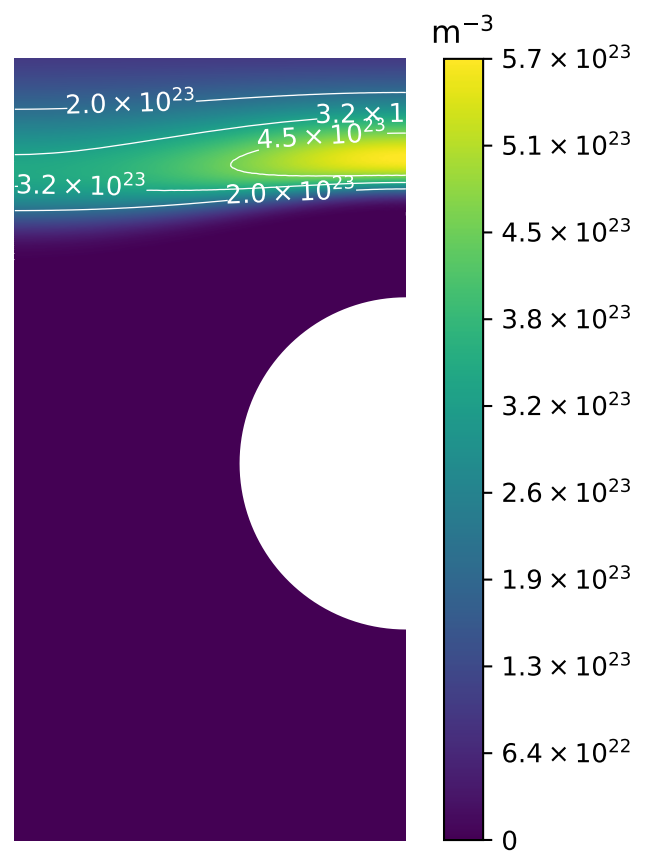
Parametric study
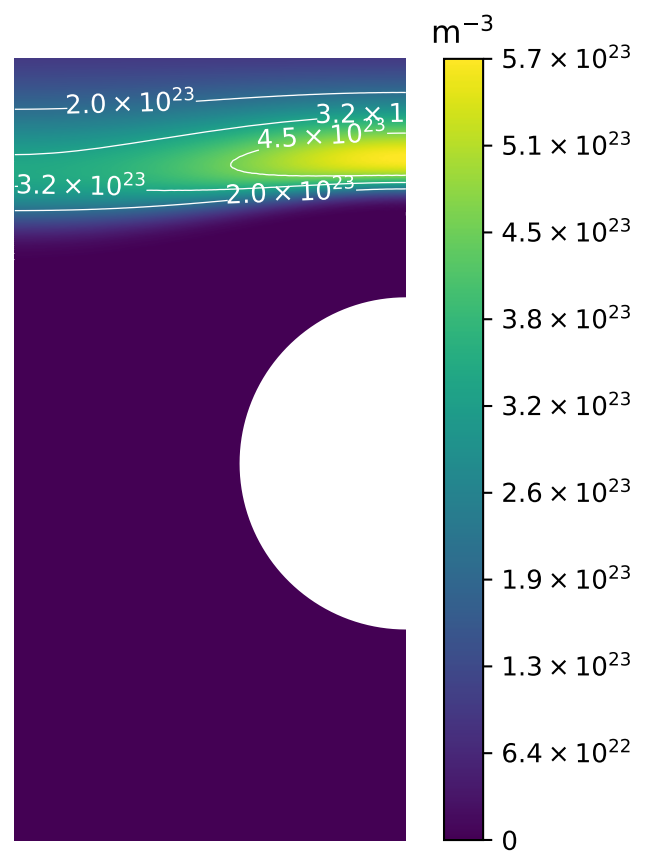
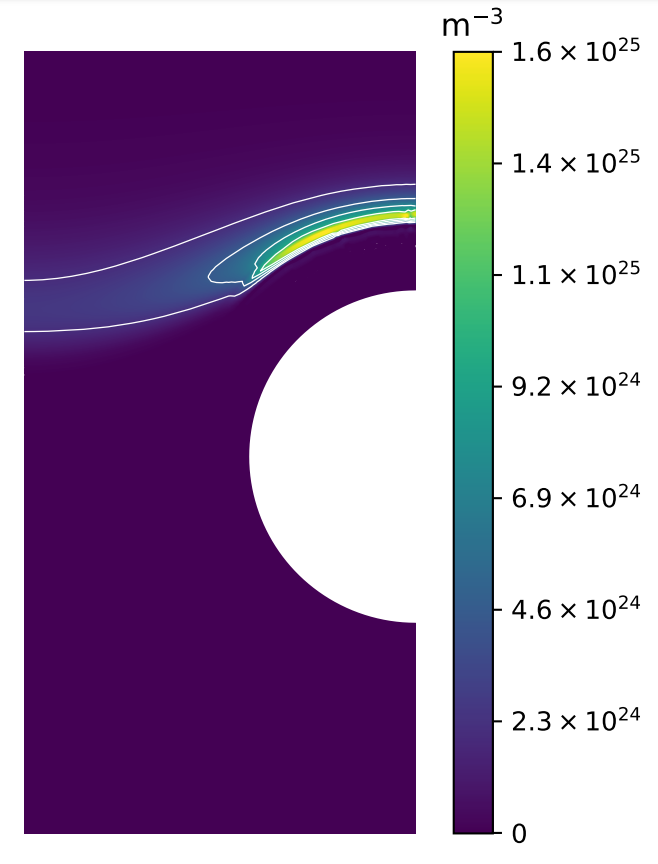
+
+
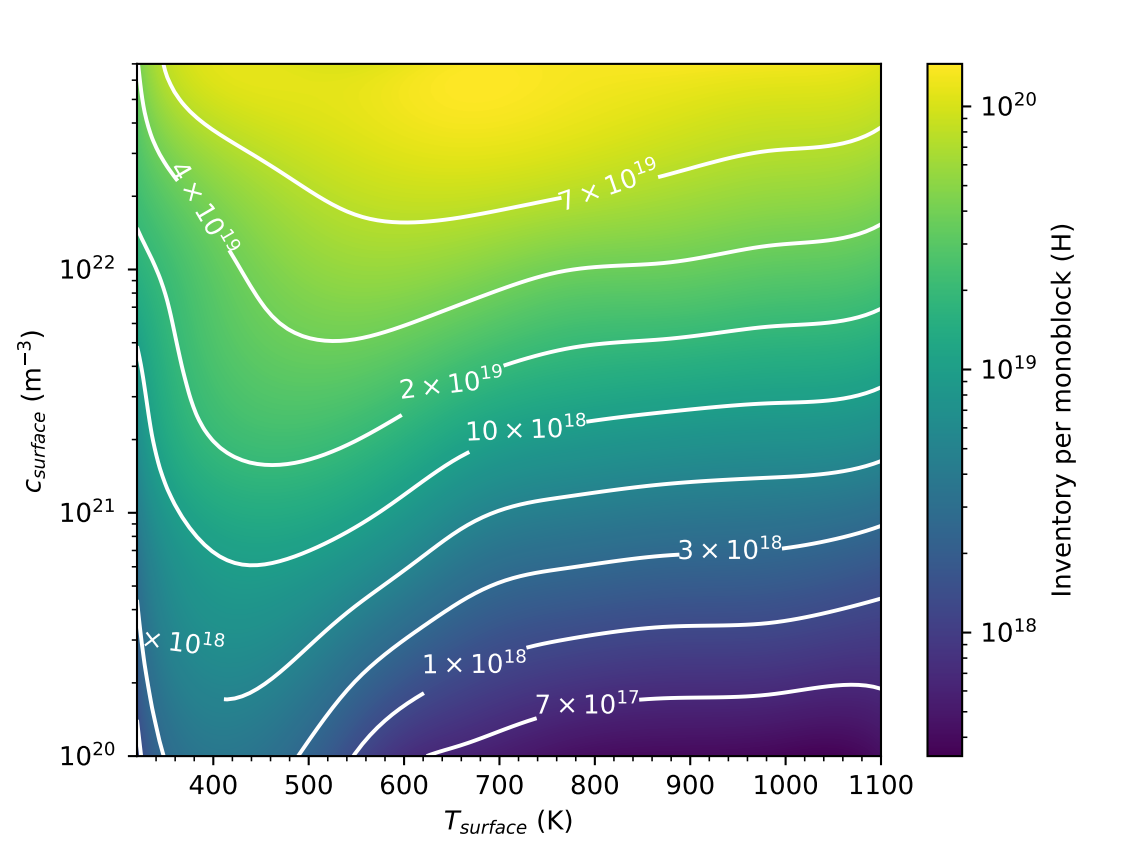
Tsurface (K)
csurface (m−3)
Parametric study
+
+
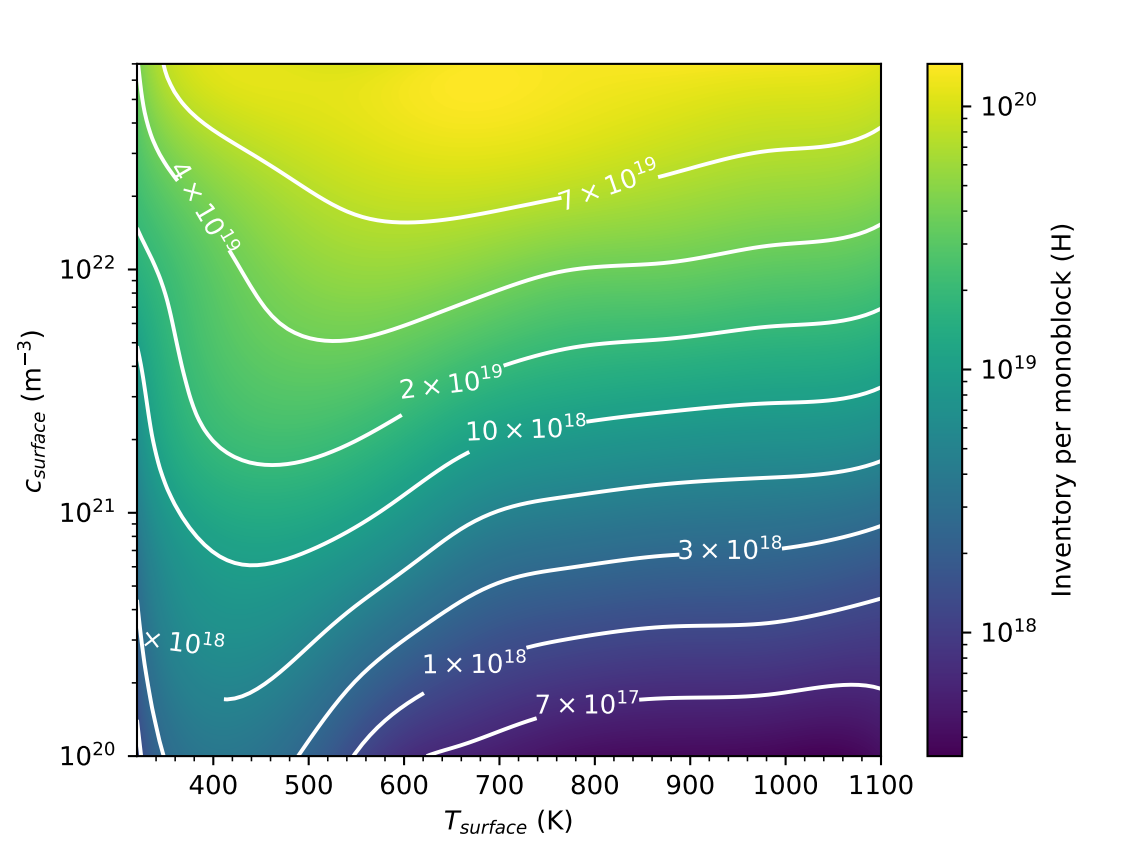
+
+
+
+
+
+
+
+
+
+
+
+
Tsurface (K)
csurface (m−3)
Parametric study
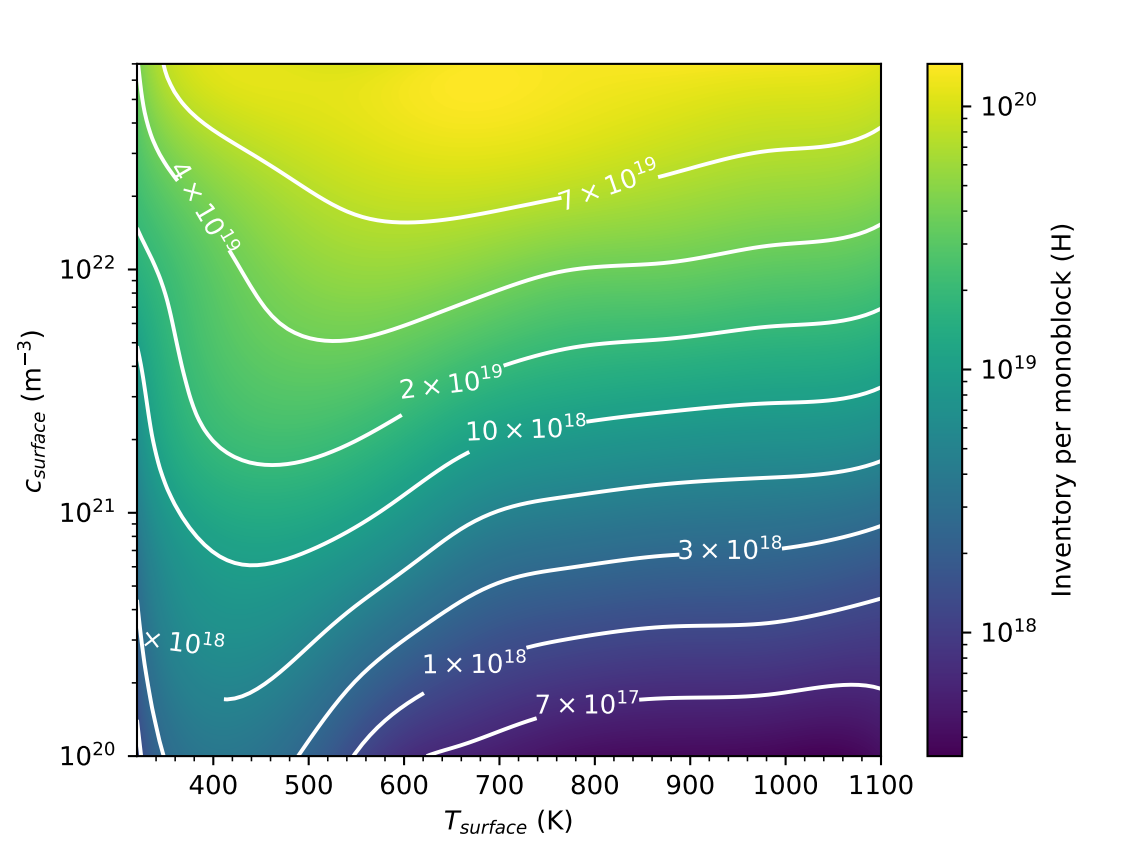
At
Gaussian Process Regression (GPR)
Tsurface (K)
csurface (m−3)
Gaussian process regression
https://juanitorduz.github.io/gaussian_process_reg/
Parametric study
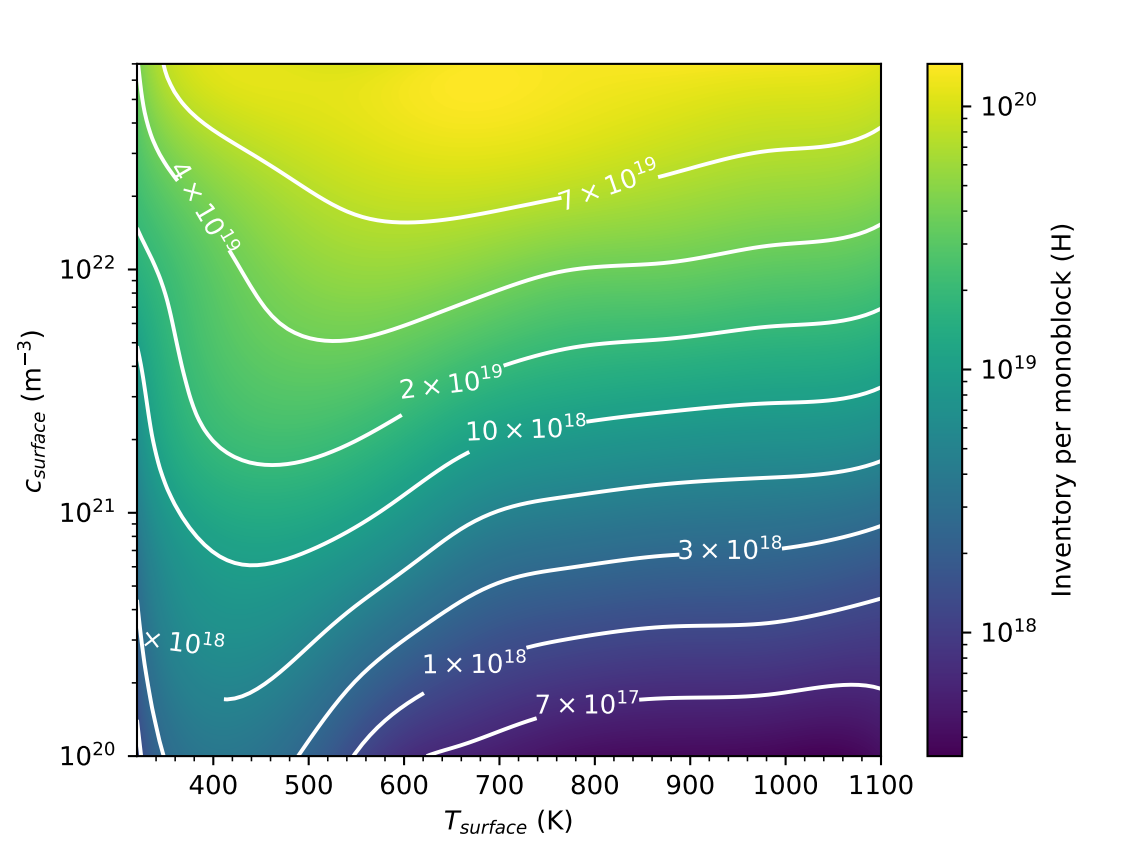
Monoblock behaviour law
At
Rapid assessment of monoblock inventories
csurface (m−3)
Tsurface (K)
+
+
+
+
+
+
+
+
+
+
A better behaviour law
Instantaneous recombination
✔️Non homogeneous surface temperature
✔️Non homogeneous surface concentration
❌Only works for instantaneous recombination
+
+
+
+
+
+
+
+
+
+
A better behaviour law
Non-instantaneous recombination
✔️Non homogeneous surface temperature
✔️Non homogeneous surface concentration
✔️Non-instantaneous recombination
❌3 independent variables
+
A better behaviour law
Automatic sampling
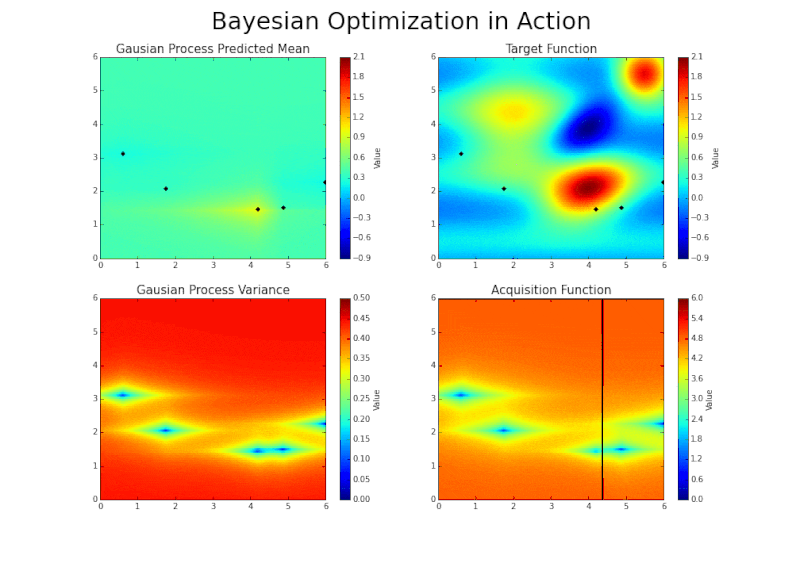
Scaling up to the divertor?
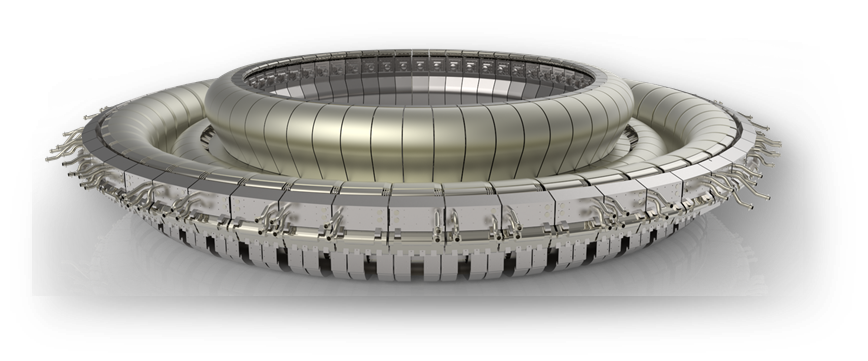
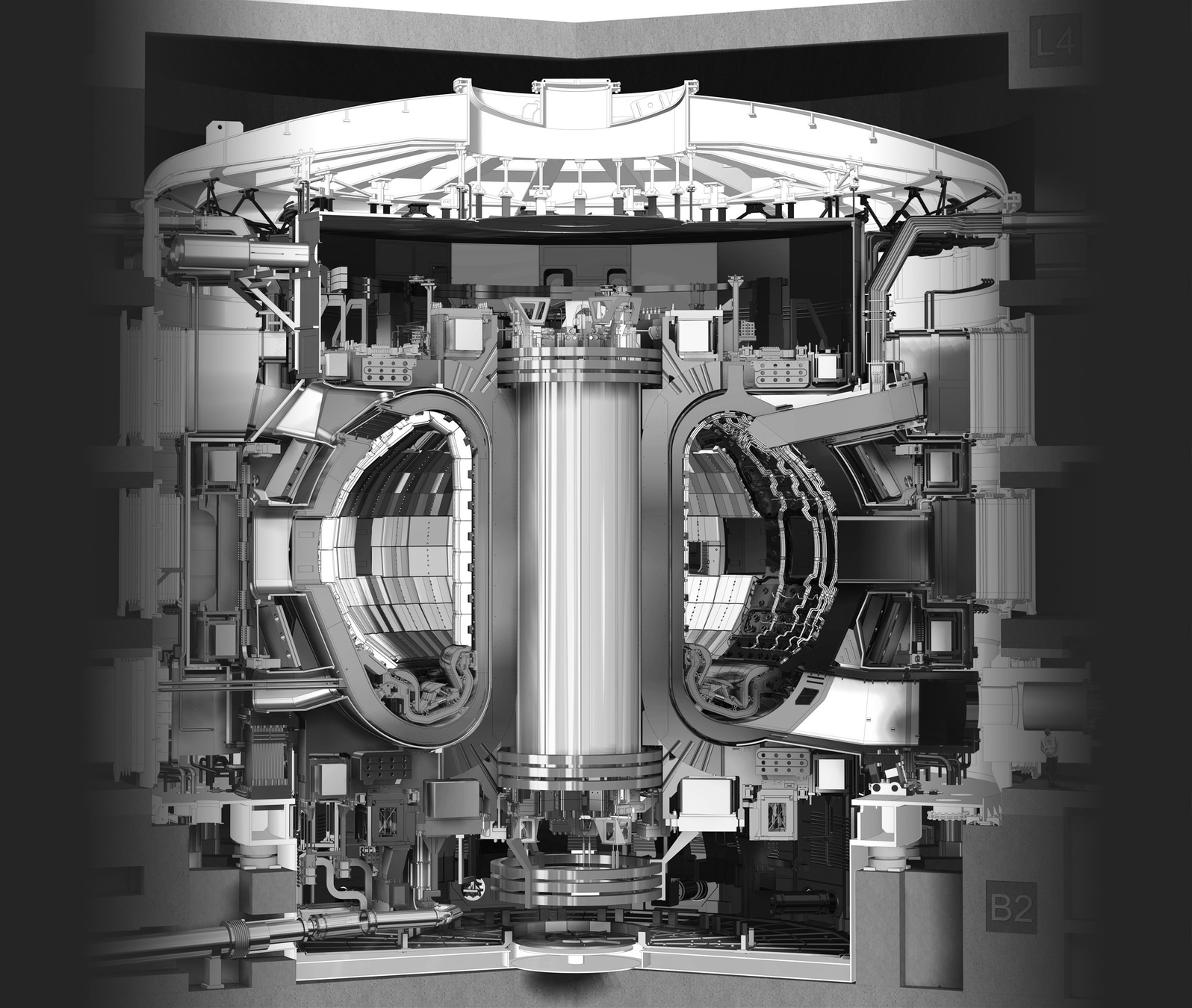
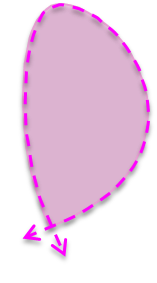
Inner Vertical Target
Inner Strike Point
Outer Strike Point
Outer Vertical Target
Dome
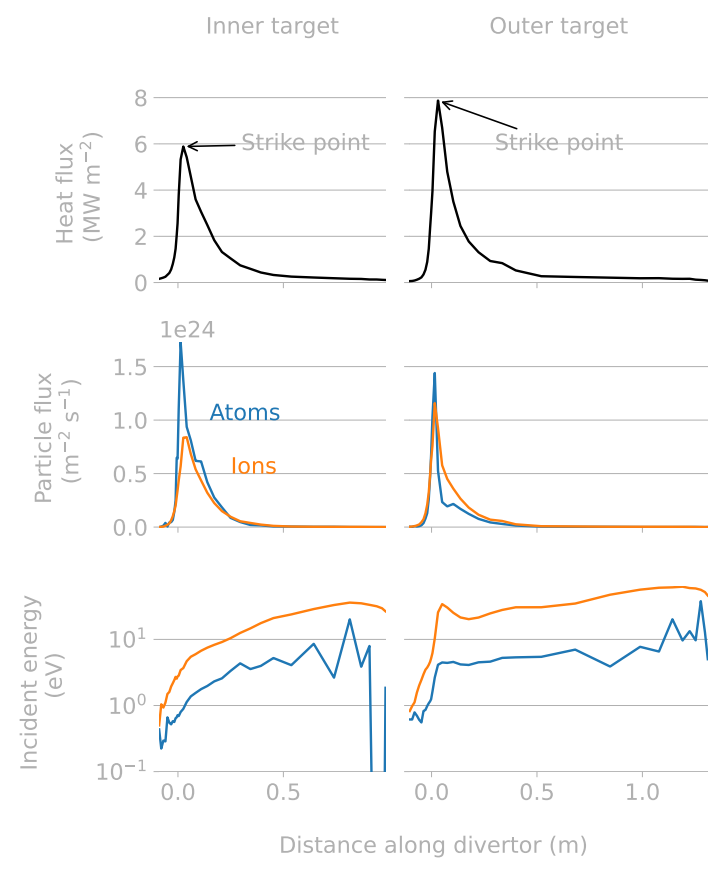
Converting plasma inputs...
Heat flux from SOLPS
Shot #2399
Plasma codes
Mass transport
Momentum
Ions energy Ti
Electrons energy Te
n: density
u: velocity
Surface concentration can be estimated
Implantation depth Rp
Depth
Concentration
φimp
φdiff
φdesorption
φimp=φdesorption+φdiff
When φdiff≪φdesorption→cmax=DφimpRp
cmax
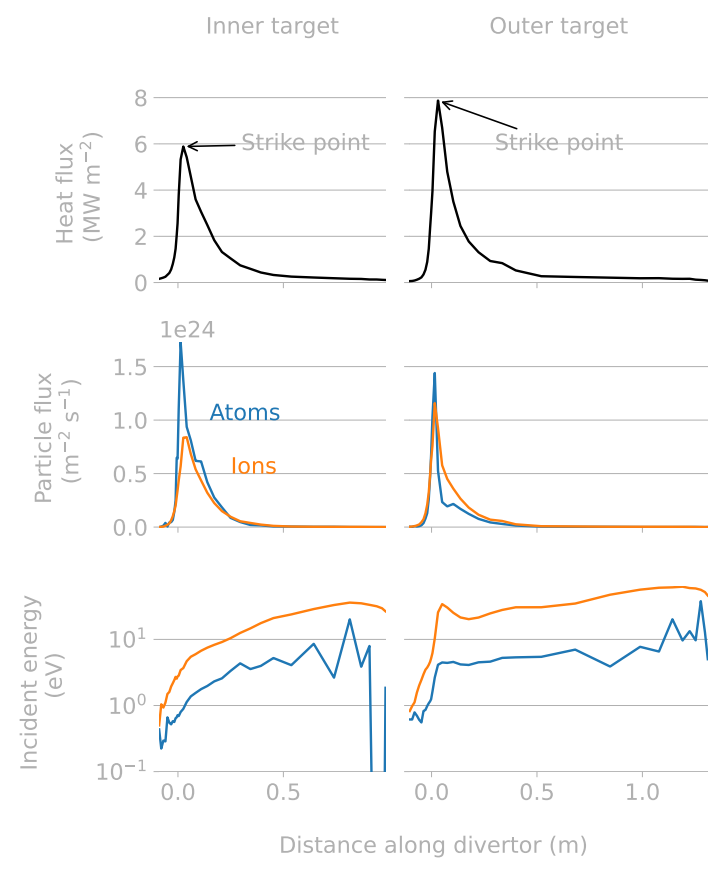
Converting plasma inputs...
Implantation range and reflection coeff. are obtained from SRIM
Use of SRIM is questionable here...
The flux of He is ≈ 1 % of that of D
...to divertor inventory
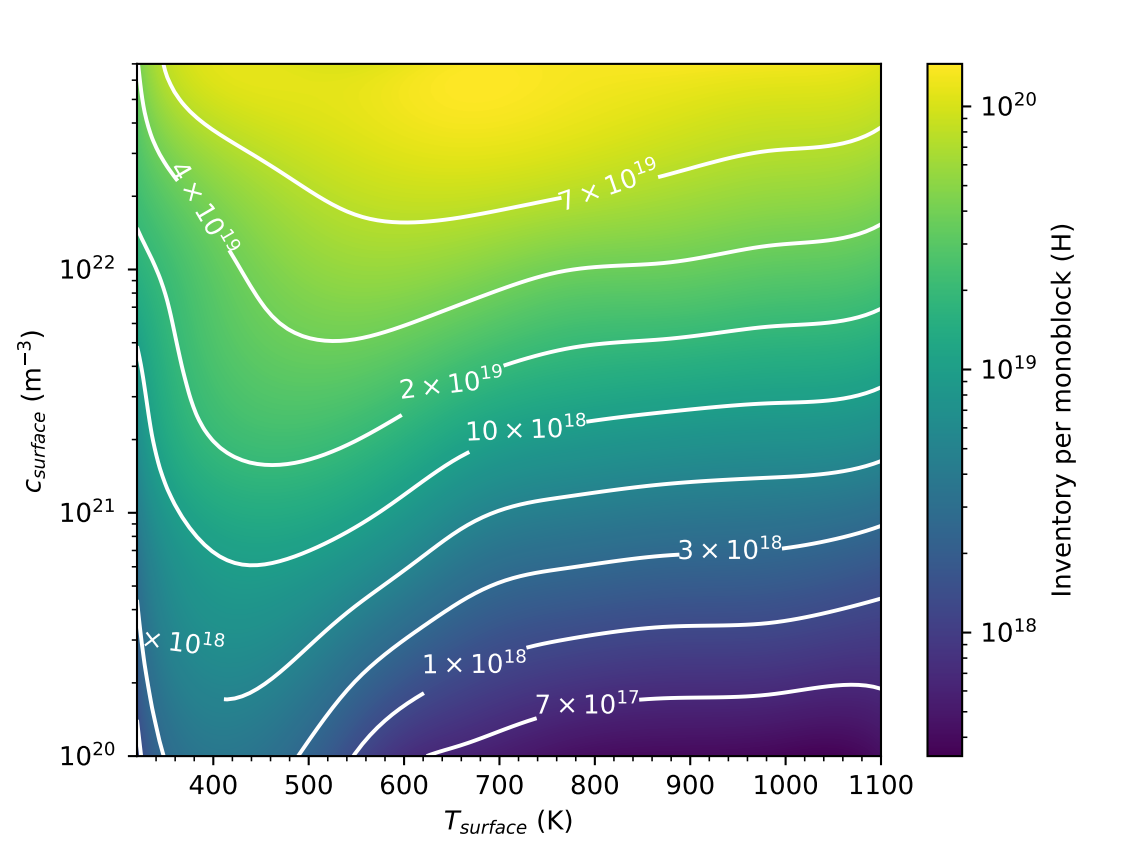
Monoblock inventory
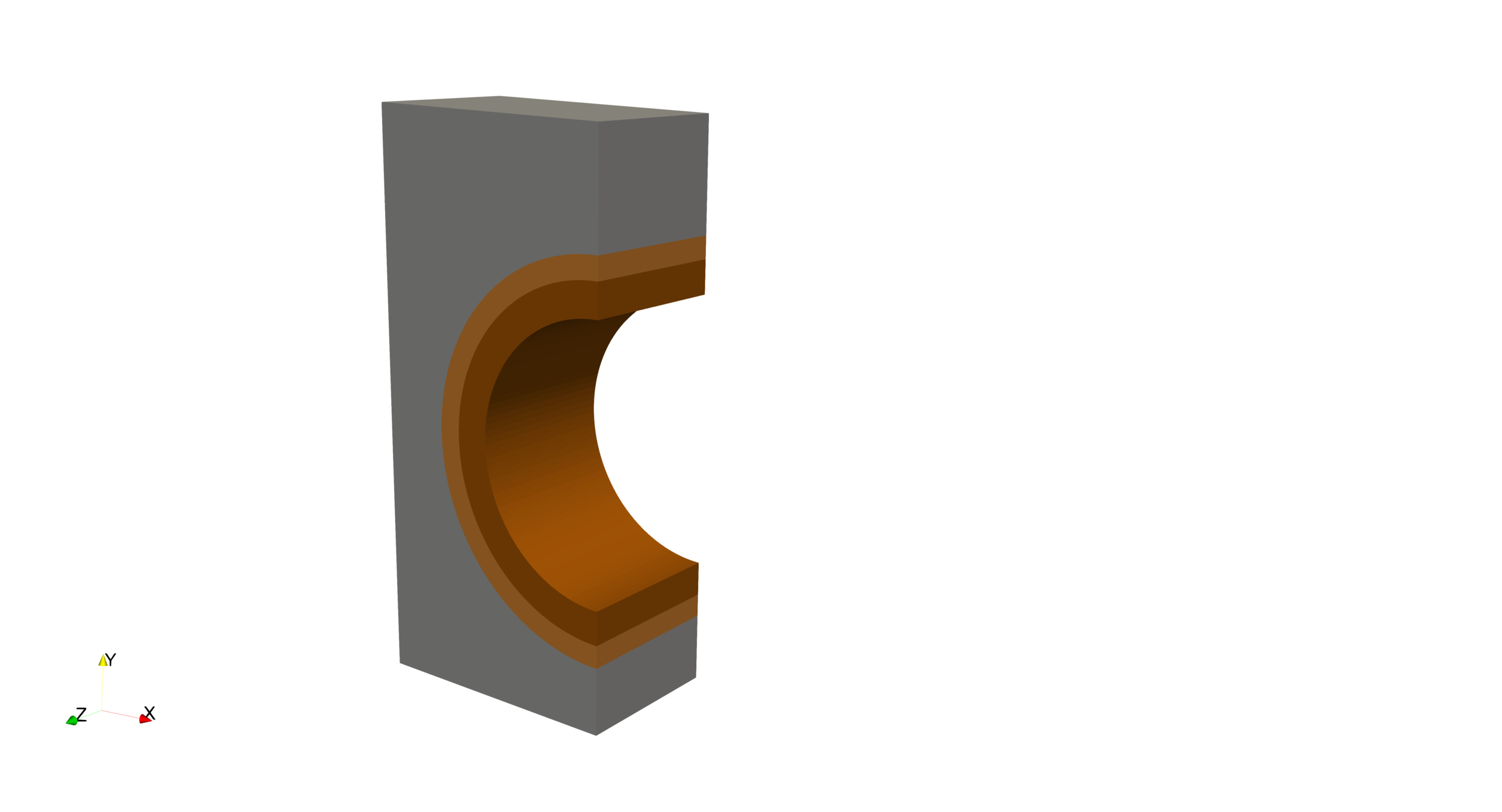
+
Divertor inventory
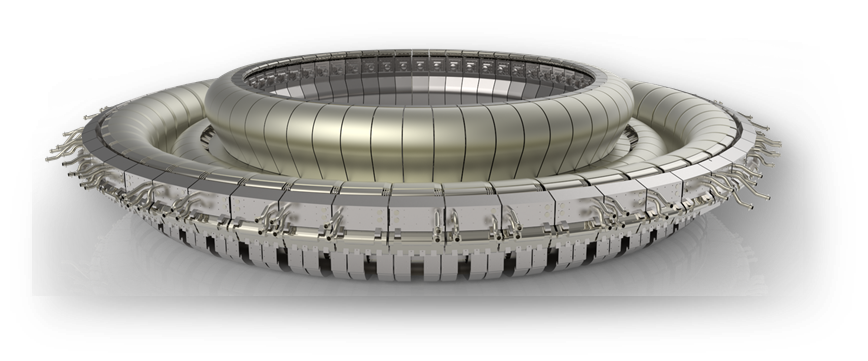
Exposure conditions can be obtained for several divertor pressures
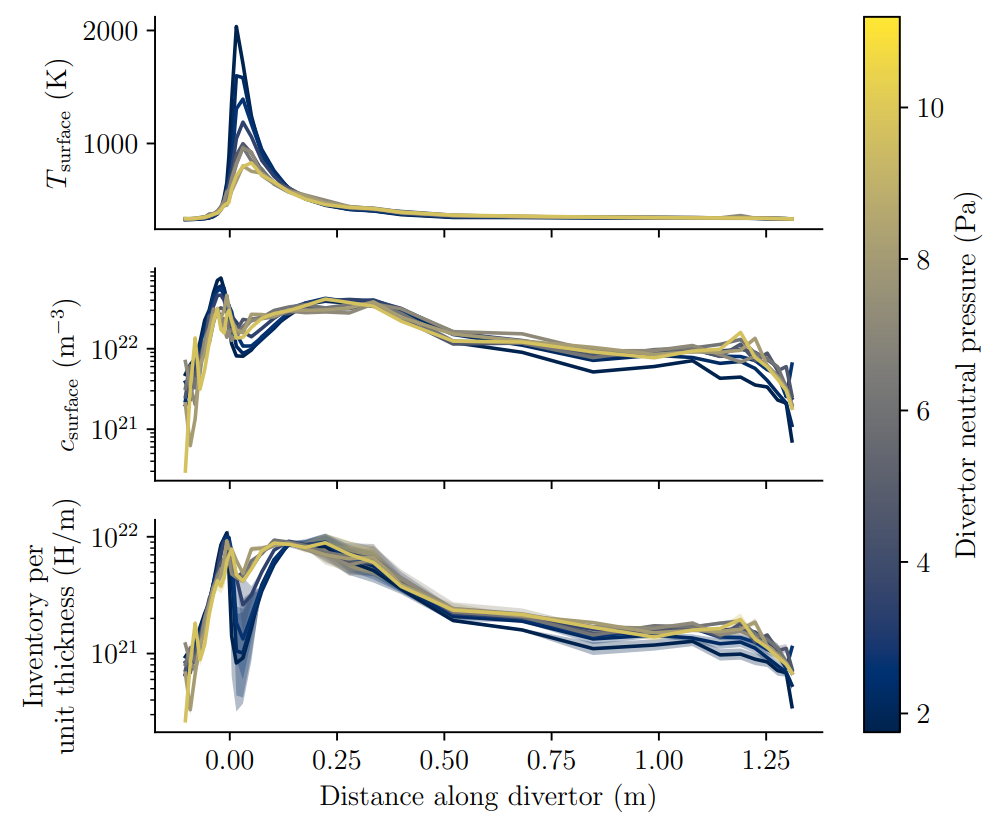
SOLPS runs: Pitts et al NME (2020)
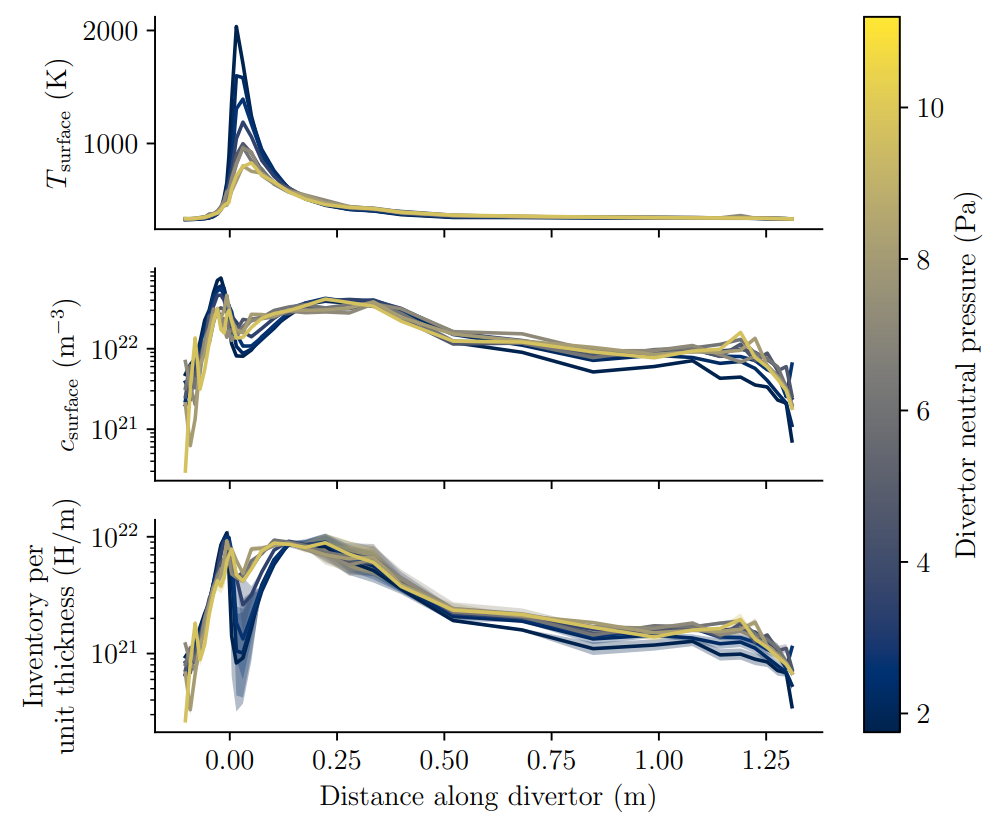
The inventory is estimated from the surrogate model
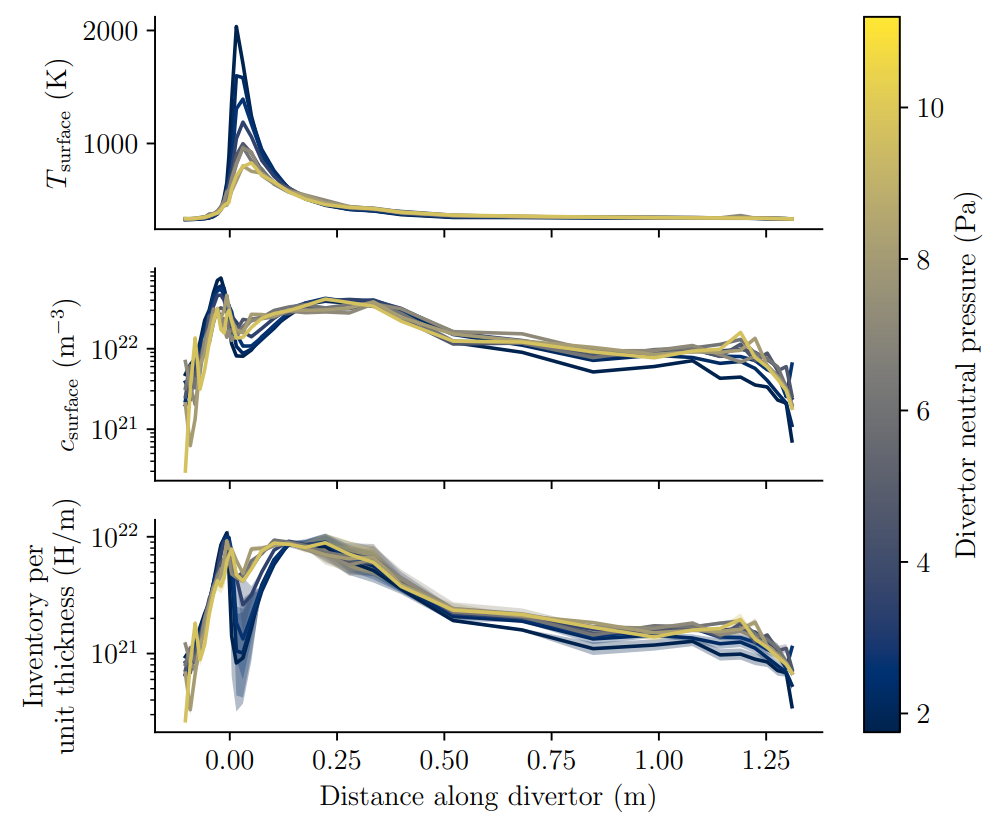
SOLPS runs: Pitts et al NME (2020)
- The strike point is not the maximum H inventory
- The total divertor inventory can be computed
invdivertor=Ncassettes (NPFU−IVT∫invIVT(x)dx+
NPFU−OVT∫invOVT(x)dx)
The inventory is estimated from the surrogate model
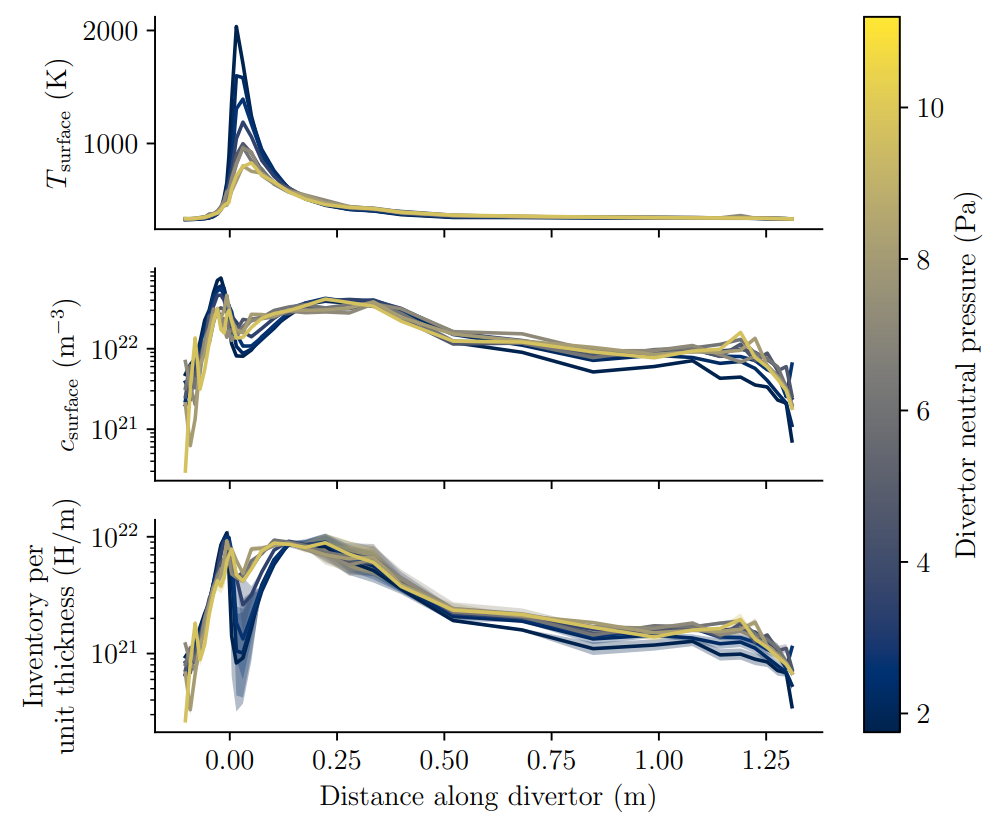
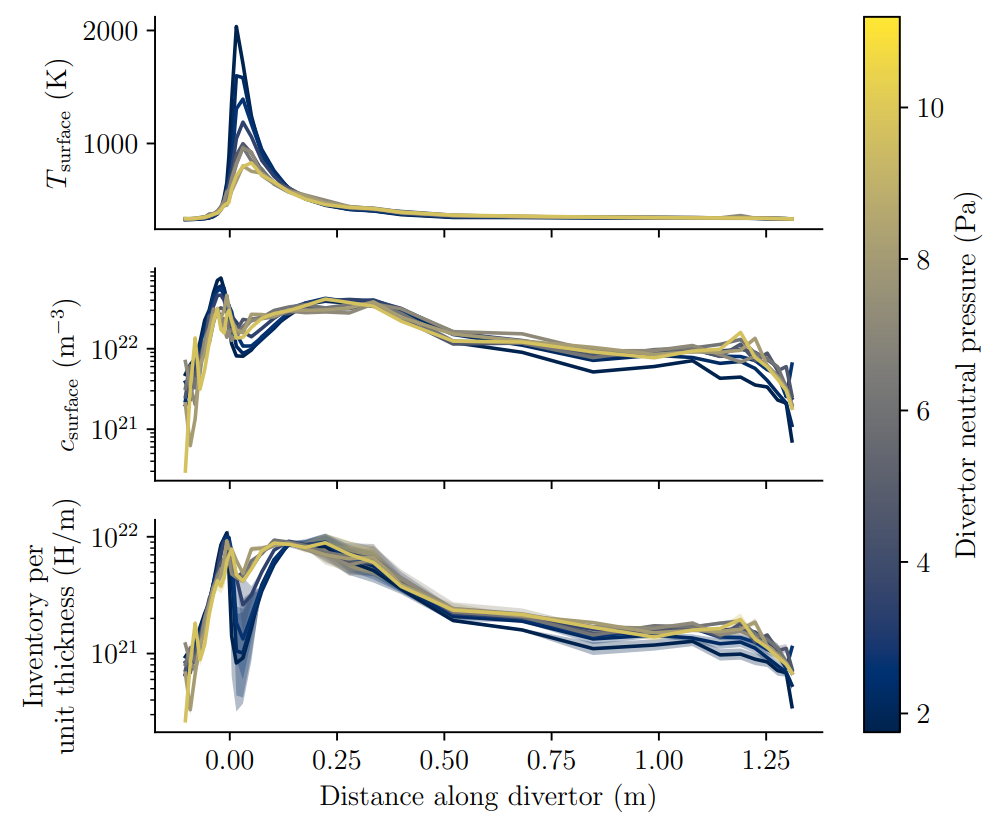
SOLPS runs: Pitts et al NME (2020)
- The strike point is not the maximum H inventory
- The total divertor inventory can be computed
invdivertor=54 (16∫invIVT(x)dx+
22∫invOVT(x)dx)
The inventory is estimated from the surrogate model
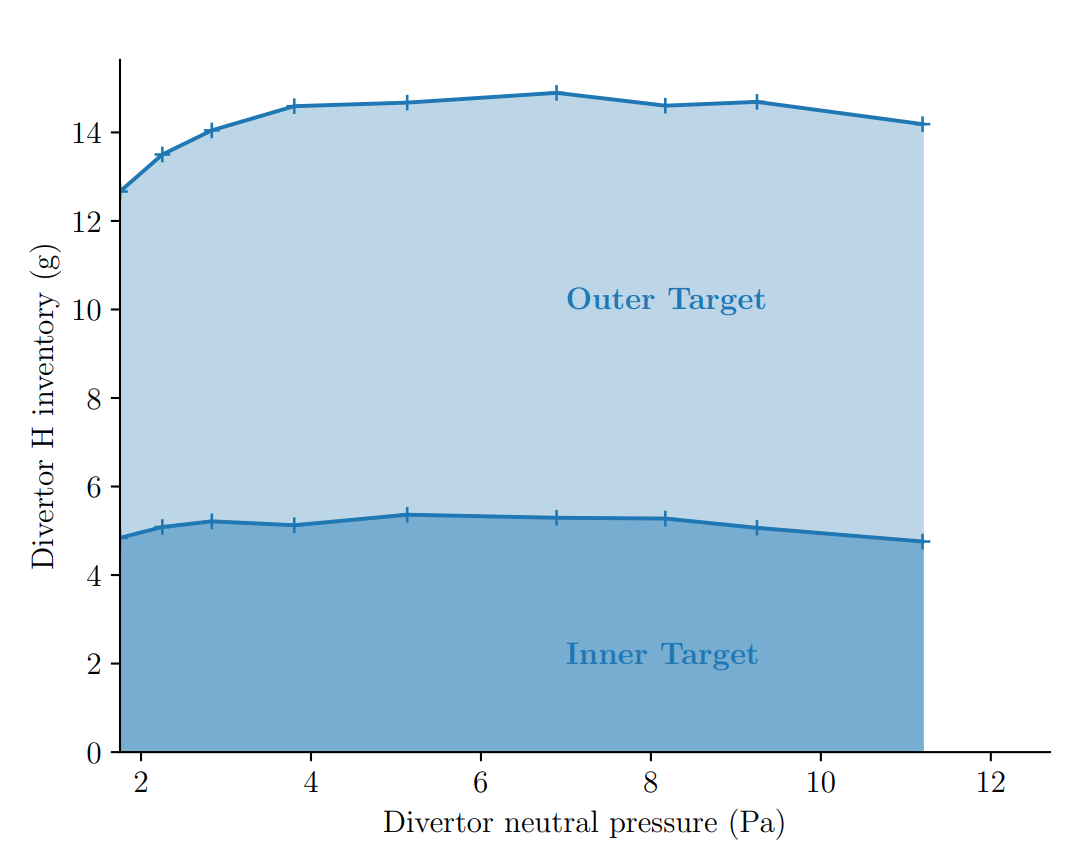
Divertor H inventory (g) at t=107s
Safety limit = 700 g of tritium
max≈ 2 % limit ✅
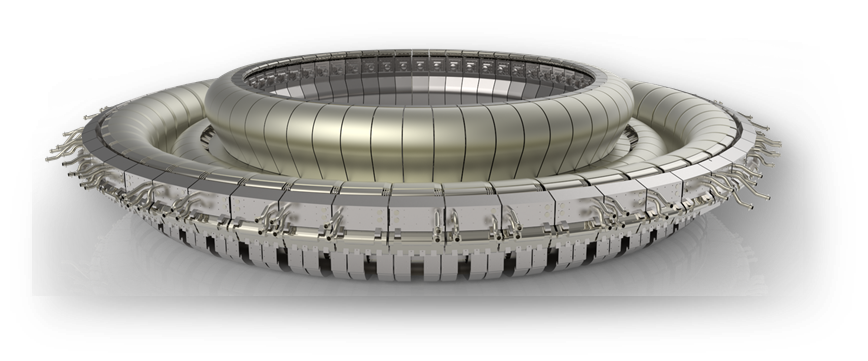
ITER divertor inventory
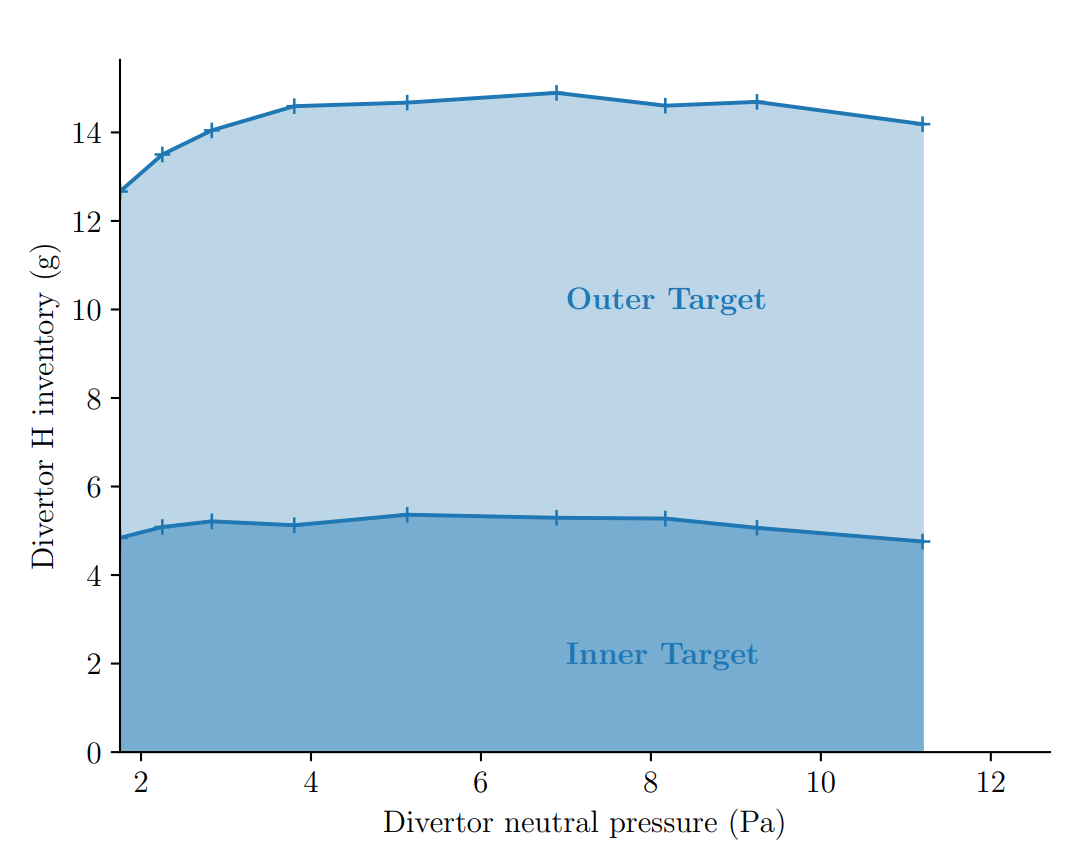
Safety limit = 700 g of tritium
max≈ 2 % limit ✅
Assuming 50% T
≈ 1% limit ✅
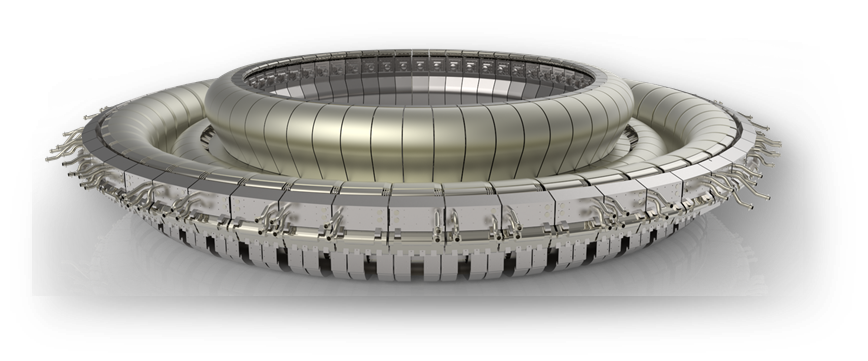
ITER divertor inventory
Divertor H inventory (g) at t=107s
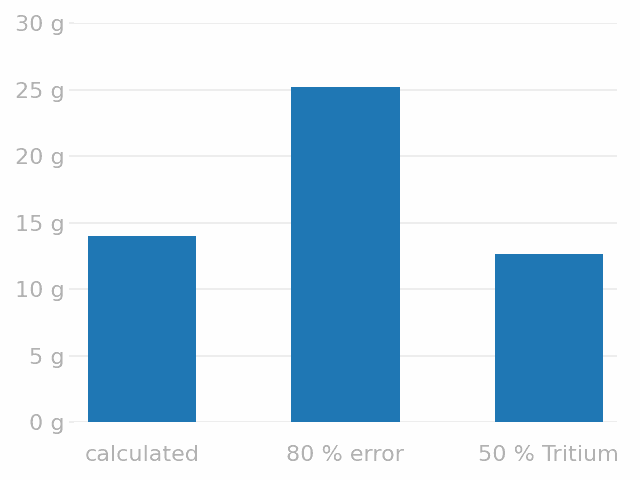
Neglecting trap 2
Influence of helium on hydrogen transport
Why should we care?
Why should we care?
Bubbles
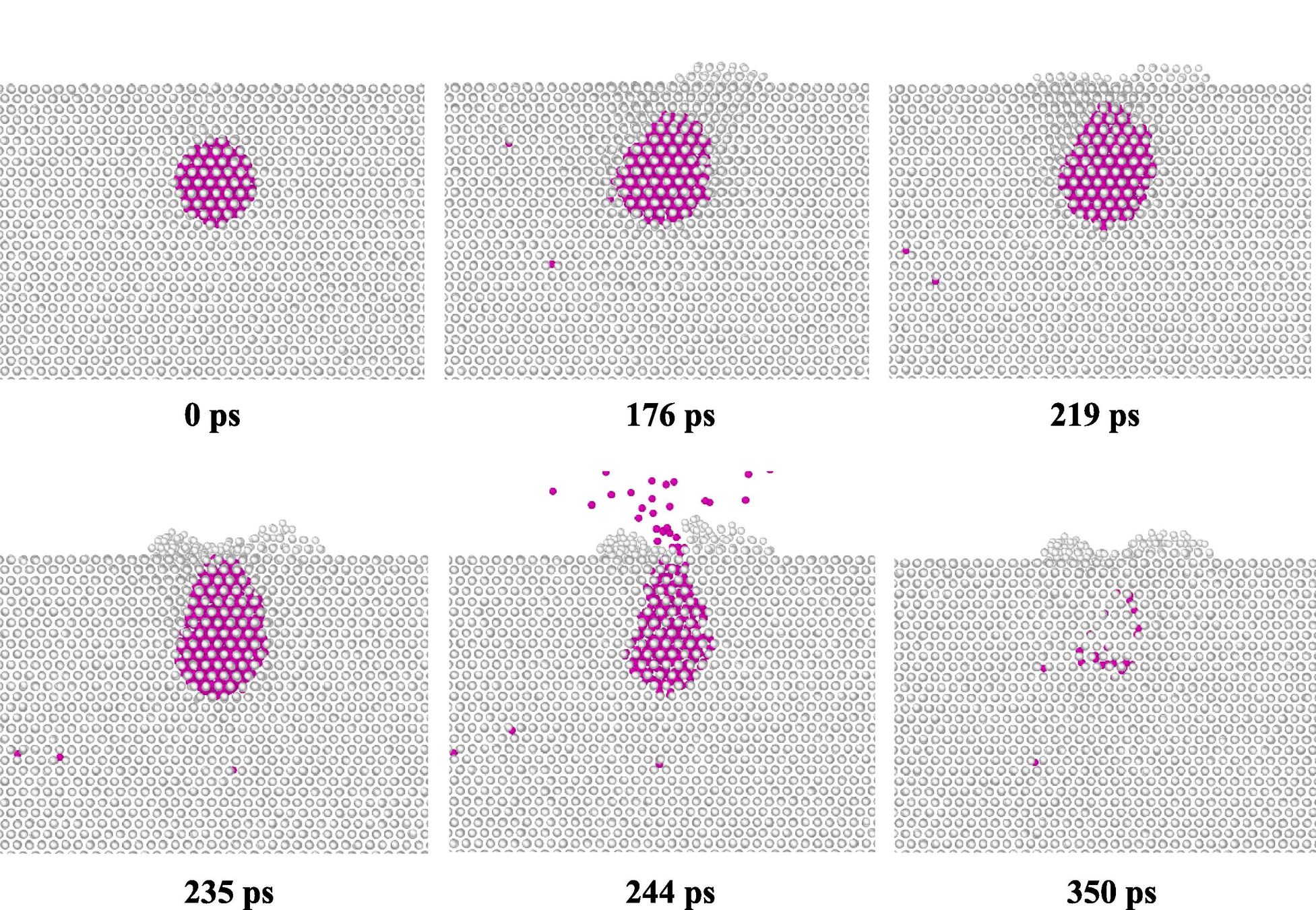
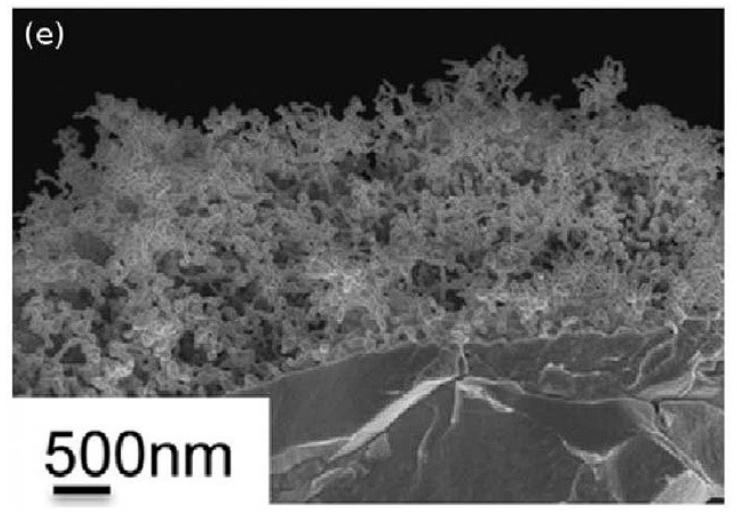
Tungsten fuzz
Thermo-mechanical properties
Tritium production
Hydrogen transport
Sources of helium
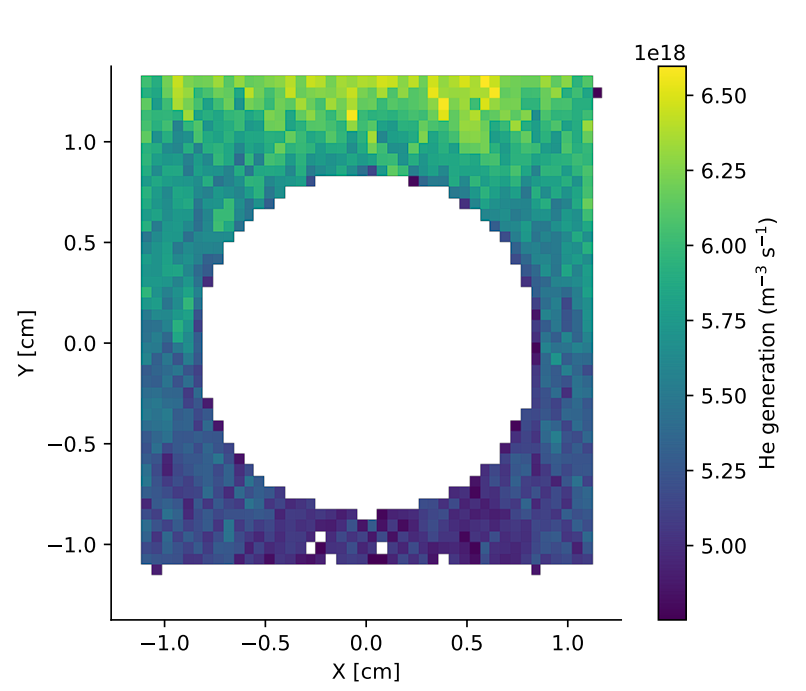
Neutronics monoblock simulations with OpenMC
500 MW of fusion power
≈1020n/s
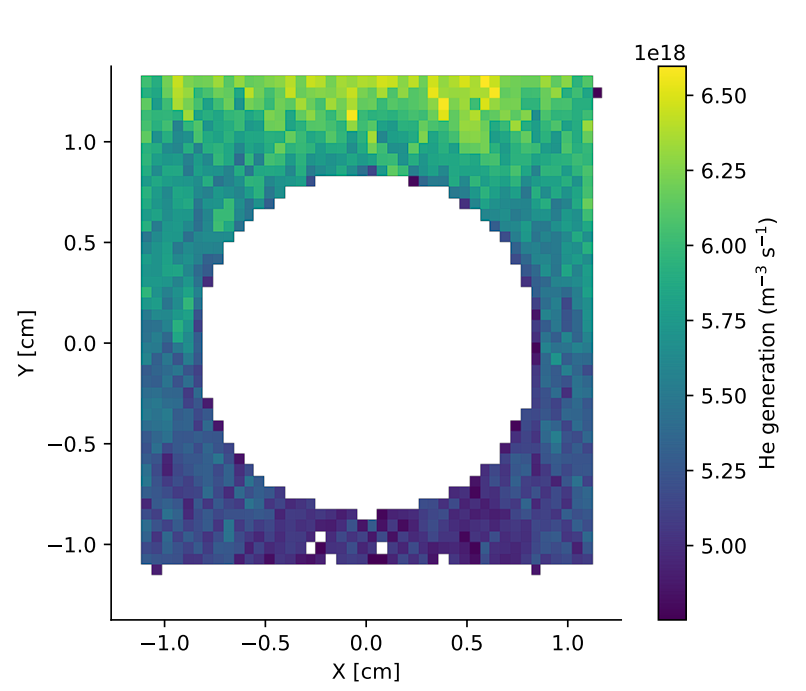
500 MW of fusion power
≈1020n/s
Energy released by 1 DT reaction:
17.58 MeV=2.81×10−12 J
Tally (n,Xa) reaction
DT neutron source energy spectrum
He generation distribution
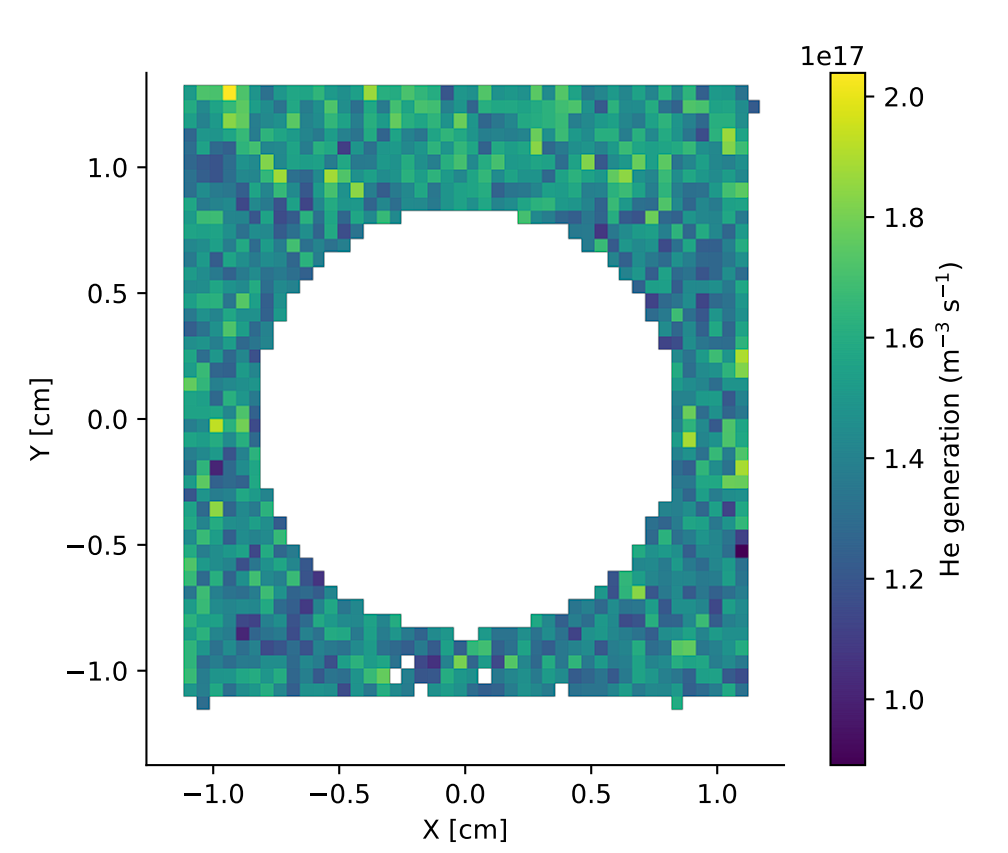
Standard deviation
Neutronic heating
Todo: assess influence of this on the thermals
Sources of helium
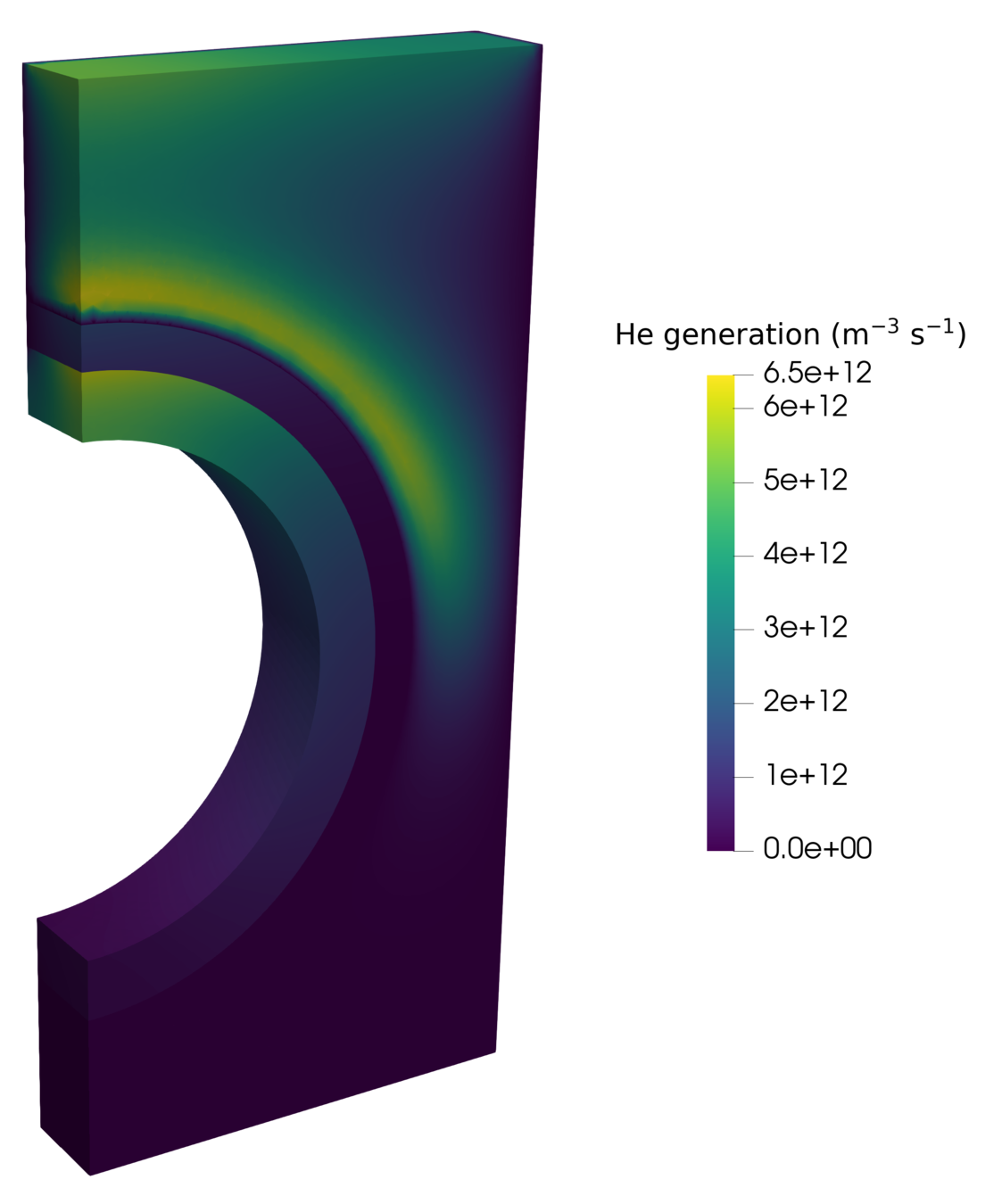
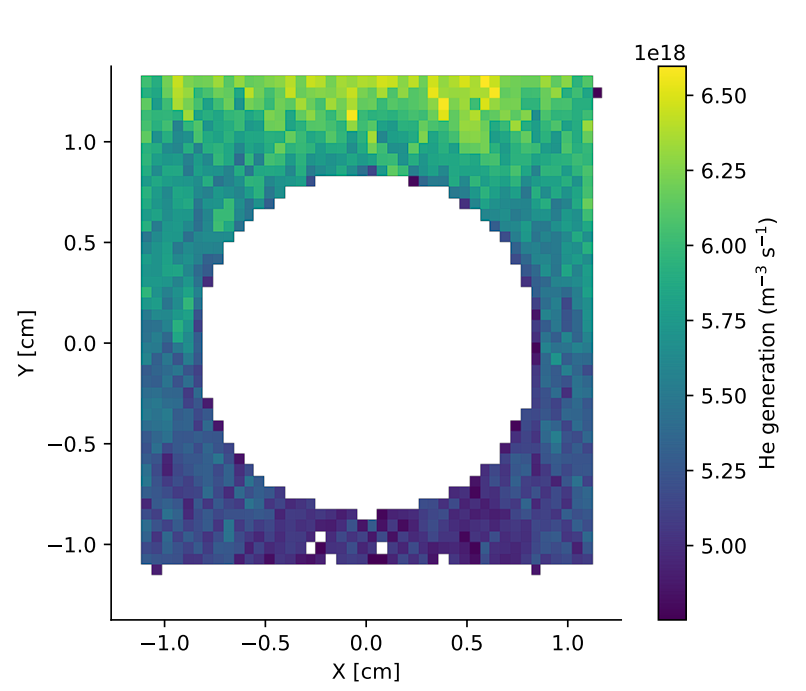
Tritium decay simulations with FESTIM
Neutronics monoblock simulations with OpenMC
Tritium decay
Decay constant λ=1.77×10−9 s−1
Decay source for Helium: λ∑ ci
Sources of helium
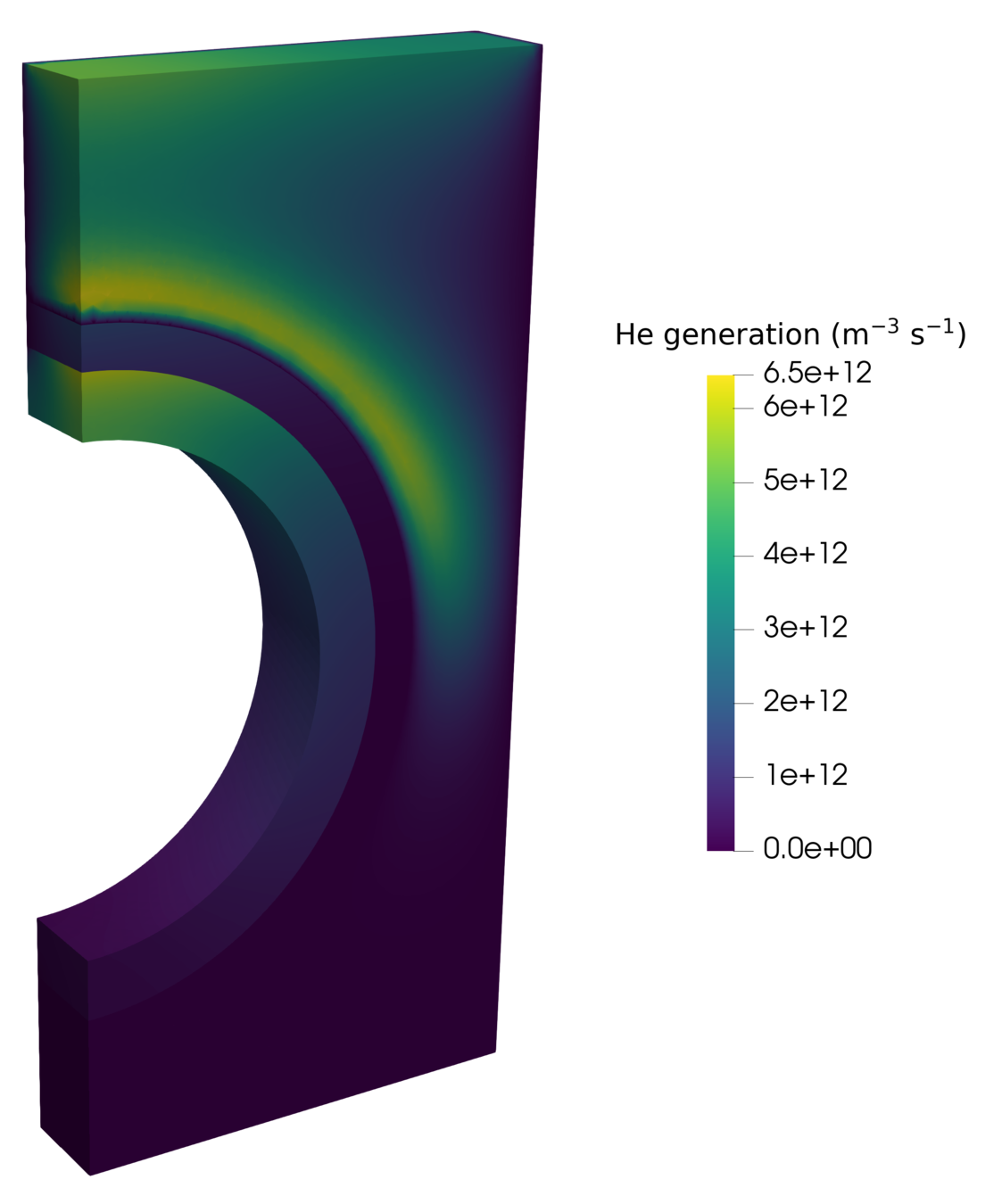
Tritium decay simulations with FESTIM
Neutronics monoblock simulations with OpenMC
→ Focus on direct implantation
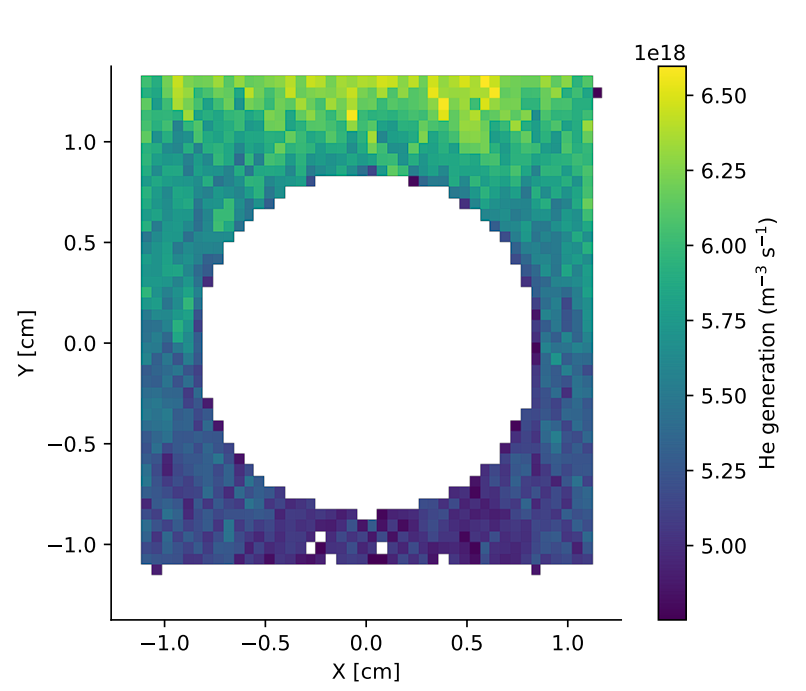
He1
He2
He4
He3
V1He7
V1He8
V1He9
V1He10
Our clustering scheme
Trap mutation
or
self-trapping
∂t∂c1=∇⋅(D1∇c1)+P1−2k1,1+c12−i=2∑6k1,i+c1ci−⟨kb+⟩c1cb
⋮
∂t∂c6=∇⋅(D6∇c6)−k1,6+c1c6+k1,5+c1c5
∂t∂cb=k1,6+c1c6
∂t∂⟨ib⟩cb=7k1,6+c1c6+⟨kb+⟩c1cb
Our clustering scheme
average clustering rate in bubbles
⟨kb+⟩=4πD1(r1+⟨rb⟩)
average bubble radius
⟨rb⟩=rHe0V1+(4π32a034⟨ib⟩)1/3−(4π32a03)1/3
Only 8 equations:
Let's consider the following reactions
Helium clustering (or emission)
Trap mutation
For each reaction
3-species model:
Rate constants:
N-species model:
Diffusion coefficients
Capture radii
Diffusion
Production
Reaction
Binding energy
Derivation of the forward reaction rate
ni: local concentration
ci: mean concentration
ri,j=ri+rj: coordinate where the reaction happens (capture radii)
ci
j
in spherical coordinates
2 diffusive species from superpostion principle:
ki,j+=4π ri,j (Di+Dj)
Issue #1: Too many equations
∂t∂c1=∇⋅(D1∇c1)+P1−2k1,1+c12−i=2∑∞k1,i+c1ci
⋮
∂t∂ci=∇⋅(Di∇ci)−k1,i+c1ci+k1,i−1+c1ci−1
⋮
∂t∂cN= −k1,N+c1cN +k1,N−1+c1cN−1
∂t∂cN+1= −k1,N+1+c1cN+1 +k1,N+c1cN
∂t∂cN+2= −k1,N+2+c1cN+2 +k1,N+1+c1cN+1
⋮
💪
∂t∂c1=∇⋅(D1∇c1)+P1−2k1,1+c12−i=2∑∞k1,i+c1ci
⋮
∂t∂ci=∇⋅(Di∇ci)−k1,i+c1ci+k1,i−1+c1ci−1
⋮
∂t∂cN+1= −k1,N+1+c1cN+1 +k1,N+c1cN
∂t∂cN+2= −k1,N+2+c1cN+2 +k1,N+1+c1cN+1
∂t∂cN+3= −k1,N+3+c1cN+3 +k1,N+2+c1cN+2
⋮
💪
Issue #1: Too many equations
∂t∂c1=∇⋅(D1∇c1)+P1−2k1,1+c12−i=2∑∞k1,i+c1ci
⋮
∂t∂ci=∇⋅(Di∇ci)−k1,i+c1ci+k1,i−1+c1ci−1
⋮
i=N+1∑∞∂t∂ci=k1,N+c1cN
💪
Issue #1: Too many equations
∂t∂c1=∇⋅(D1∇c1)+P1−2k1,1+c12−i=2∑∞k1,i+c1ci
⋮
∂t∂ci=∇⋅(Di∇ci)−k1,i+c1ci+k1,i−1+c1ci−1
⋮
∂t∂cb=k1,N+c1cN
cb=i=N+1∑∞ci : bubble concentration
💪
Issue #1: Too many equations
∂t∂c1=∇⋅(D1∇c1)+P1−2k1,1+c12−i=2∑Nk1,i+c1ci−i=N+1∑∞ki,1+cic1
⋮
∂t∂ci=∇⋅(Di∇ci)−k1,i+c1ci+k1,i−1+c1ci−1
⋮
∂t∂cb=k1,N+c1cN
cb=i=N+1∑∞ci : bubble concentration
💪
Issue #1: Too many equations
∂t∂c1=∇⋅(D1∇c1)+P1−2k1,1+c12−i=2∑Nk1,i+c1ci−⟨kb+⟩c1cb
⋮
∂t∂ci=∇⋅(Di∇ci)−k1,i+c1ci+k1,i−1+c1ci−1
⋮
∂t∂cb=k1,N+c1cN
cb=i=N+1∑∞ci : bubble concentration
⟨kb+⟩=(i=N+1∑∞ki,1+ci)/cb : average clustering rate in bubbles
💪
Issue #1: Too many equations
cb=i=N+1∑∞ci : bubble concentration
⟨kb+⟩=(i=N+1∑∞ki,1+ci)/cb
=(i=N+1∑∞4πD1(r1+ri)ci)/cb
💪
average clustering rate in bubbles
Issue #2: what's the value of ⟨kb+⟩?
Issue #2: what's the value of ⟨kb+⟩?
cb=i=N+1∑∞ci : bubble concentration
⟨rb⟩=(i=N+1∑∞rici)/cb : average bubble radius
⟨kb+⟩=(i=N+1∑∞ki,1+ci)/cb
=(i=N+1∑∞4πD1(r1+ri)ci)/cb
=4πD1(r1+⟨rb⟩)
💪
average clustering rate in bubbles
ri=rHe0V1+(4π32a03nV,i)1/3−(4π32a03)1/3
💪
cb=i=N+1∑∞ci : bubble concentration
⟨kb+⟩=4πD1(r1+⟨rb⟩) : average clustering rate in bubbles
⟨rb⟩=(i=N+1∑∞rici)/cb : average bubble radius
Issue #3: what's the value of ⟨rb⟩?
ri=rHe0V1+(4π32a034i)1/3−(4π32a03)1/3
nV,i=i/4 : 4 He per vacancy
💪
cb=i=N+1∑∞ci : bubble concentration
⟨kb+⟩=4πD1(r1+⟨rb⟩) : average clustering rate in bubbles
⟨rb⟩=(i=N+1∑∞rici)/cb : average bubble radius
Issue #3: what's the value of ⟨rb⟩?
cb=i=N+1∑∞ci : bubble concentration
⟨kb+⟩=4πD1(r1+⟨rb⟩) : average clustering rate in bubbles
⟨rb⟩=(i=N+1∑∞rici)/cb : average bubble radius
⟨ib⟩=(i=N+1∑∞ici)/cb : average He content in bubbles
nV,i=i/4 : 4 He per vacancy
💪
⟨rb⟩=rHe0V1+(4π32a034⟨ib⟩)1/3−(4π32a03)1/3
ri=rHe0V1+(4π32a034i)1/3−(4π32a03)1/3
Issue #3: what's the value of ⟨rb⟩?
⟨rb⟩=rHe0V1+(4π32a034⟨ib⟩)1/3−(4π32a03)1/3
cbi=N+1∑∞i1/3ci ≈(i=N+1∑∞ici /cb)1/3=⟨ib⟩1/3
When ci has a narrow gaussian distribution (ie. σ/μ≪1 )
Sum approximation
⟨ib⟩=(i=N+1∑∞ici)/cb
⟨ib⟩cb= i=N+1∑∞ici
∂t∂⟨ib⟩cb=i=N+1∑∞i∂t∂ci
💪
∂t∂⟨ib⟩cb=(N+1)k1,N+c1cN+⟨kb+⟩c1cb
↓ trust me
cb=i=N+1∑∞ci : bubble concentration
⟨kb+⟩=4πD1(r1+⟨rb⟩) : average clustering rate in bubbles
⟨ib⟩=(i=N+1∑∞ici)/cb : average He content in bubbles
Issue #4: what's the value of ⟨ib⟩?
∂t∂c1=∇⋅(D1∇c1)+P1−2k1,1+c12−i=2∑N−1k1,i+c1ci−⟨kb+⟩c1cb
⋮
∂t∂ci=∇⋅(Di∇ci)−k1,i+c1ci+k1,i−1+c1ci−1
⋮
∂t∂cb=k1,N+c1cN
💪
∂t∂⟨ib⟩cb=(N+1)k1,N+c1cN+⟨kb+⟩c1cb
cb=i=N+1∑∞ci : bubble concentration
⟨kb+⟩=4πD1(r1+⟨rb⟩) : average clustering rate in bubbles
⟨ib⟩=(i=N+1∑∞ici)/cb : average He content in bubbles
Issue #4: what's the value of ⟨ib⟩?
Assumption time!
- Only pure He clusters are mobile
- Trap-mutation from 7 He
- Grouping starts at i>6
- He/V ratio: 4
- No He emission from bubbles
- Pre-existing vacancies are neglected
- We don't solve for W self-interstitials
-
Diffusion coefficients from Faney et al. Nucl. Fusion (2015)
-
Dissociation energies from Becquart et al. J. Nucl. Mater. (2010)
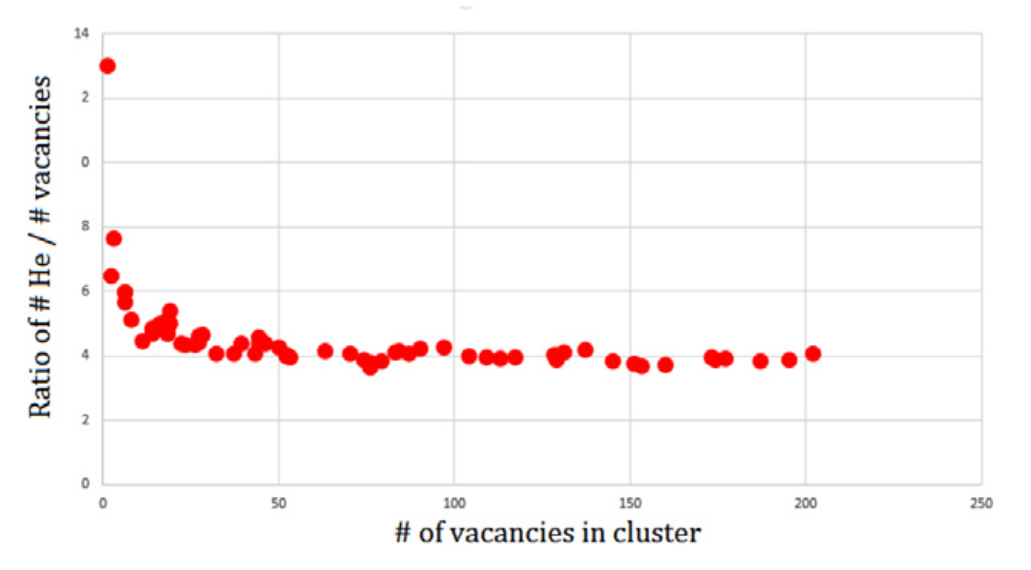
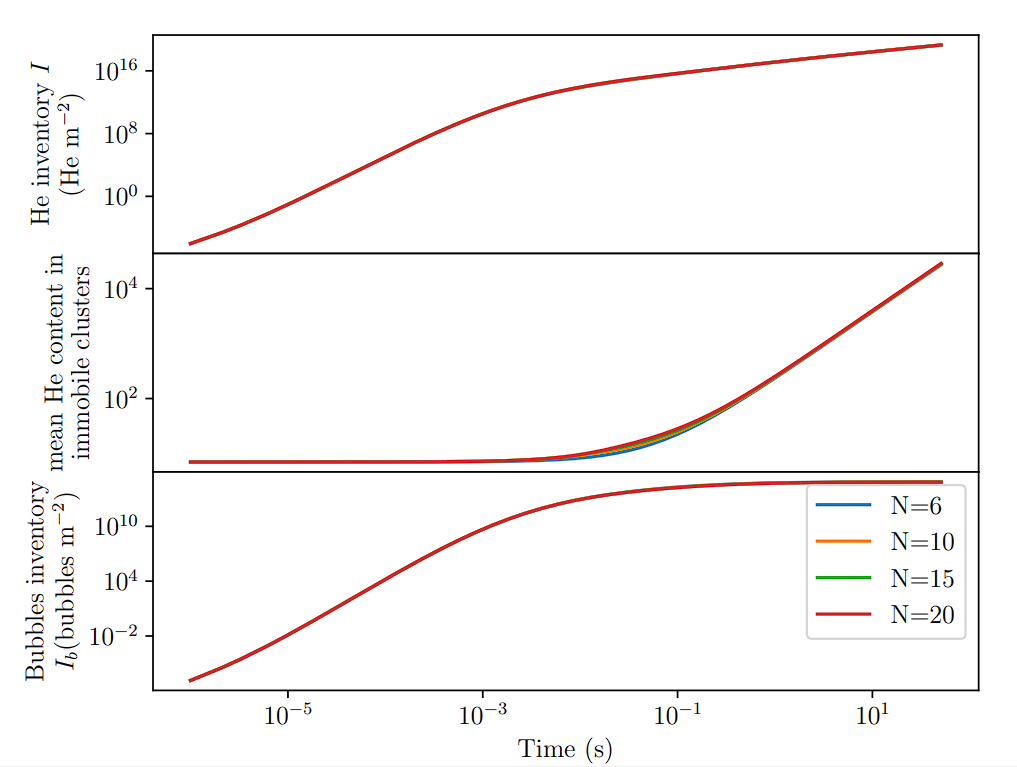
Varying the grouping threshold
Results on a half-slab case
- Helium first diffuses rapidly into the bulk
- Mobile helium is consumed to form bubbles
ci=0
W
He implantation
Mobile helium
Bubbles
Retention
m−3
Half-slab case
- "Semi-infinite" (0.6 mm)
-
Helium source
- 100 eV
-
Gaussian distribution
- μ=1.5nm σ=1.5nm
- Flux 1022m−2s−1
- Temperature 1000 K
ci=0
0.6 mm
Code comparison
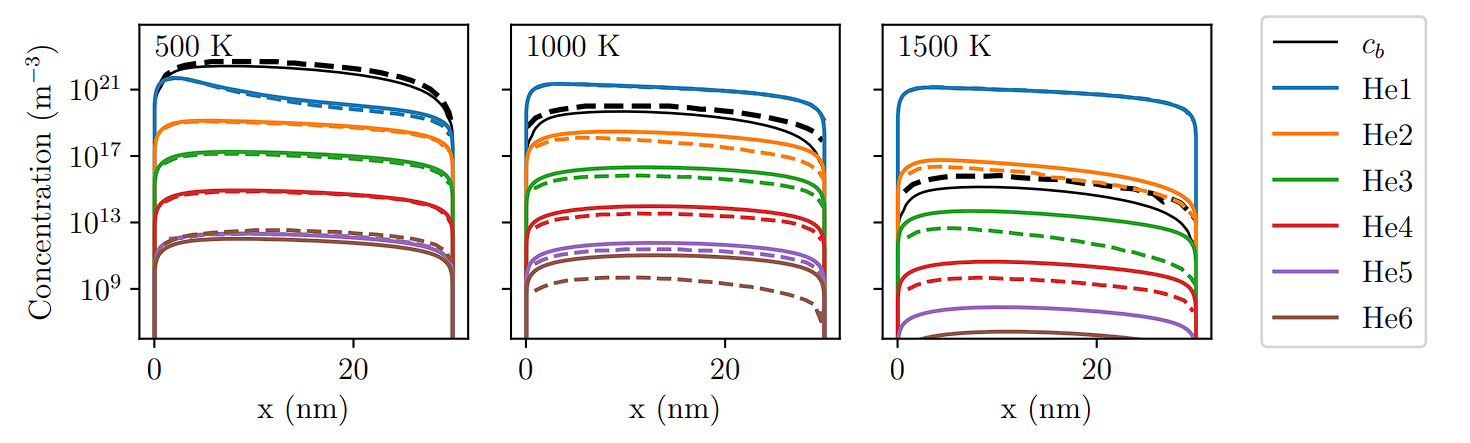
Faney et al. Nucl. Fusion 2014
- 100 eV He
- Flux 1022m−2s−1
- Fluence 5×1025 m−2
30 nm
ci=0
ci=0
Discrepancies at high T due to different sets of dissociation energies
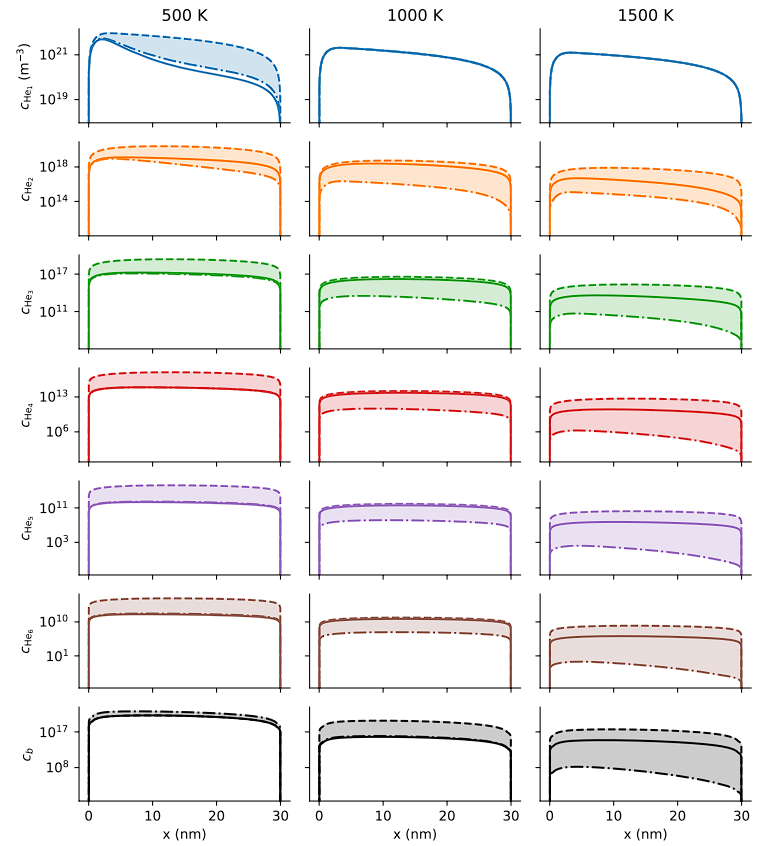
Dissociation energy sensitivity
Solid: +0
Dashed: + 0.5 eV
Dash-point: - 0.5 eV
Varying flux and temperature
cHe1ideal=D1(T)φimpRp
Varying temperature and particle flux
Parametric study
1 h
∫cb⟨ib⟩dx
He inventory in bubbles
Parametric study
1 h
⟨ib⟩ˉ=∫cbdx∫cb⟨ib⟩dx=totalbubblesinventory
Mean helium content in bubbles
Parametric study
∫cbdx
Total bubbles
1 h efef
Two regimes can be identified
Nucleation
🡺Self trapping
🡺cb increases
Growth
🡺⟨ib⟩ increases
🡺⟨kb+⟩ increases
⟨ib⟩ is low
⟨kb+⟩ is low
When cb is large enough
Two regimes can be identified
∂t∂cb=k1,N+c1cN
∂t∂⟨ib⟩cb=(N+1)k1,N+c1cN+⟨kb+⟩c1cb
⟨ib⟩≈7⇔⟨kb+⟩≈0
⇔∂t∂⟨ib⟩cb≈(N+1)k1,N+c1cN
⇔⟨ib⟩∂t∂cb+cb∂t∂⟨ib⟩≈(N+1)k1,N+c1cN
⇔∂t∂⟨ib⟩∝N+1−⟨ib⟩≈0
cb≫cN
⇔cN≈0
⇔∂t∂cb≈0
⇔∂t∂⟨ib⟩cb≈⟨kb+⟩c1cb
⇔∂t∂⟨ib⟩≈⟨kb+⟩c1
Nucleation regime
Growth regime
N cannot be lower than 6
Regime where intermediate clusters don't matter anymore
The bubble growth model was compared with experiments
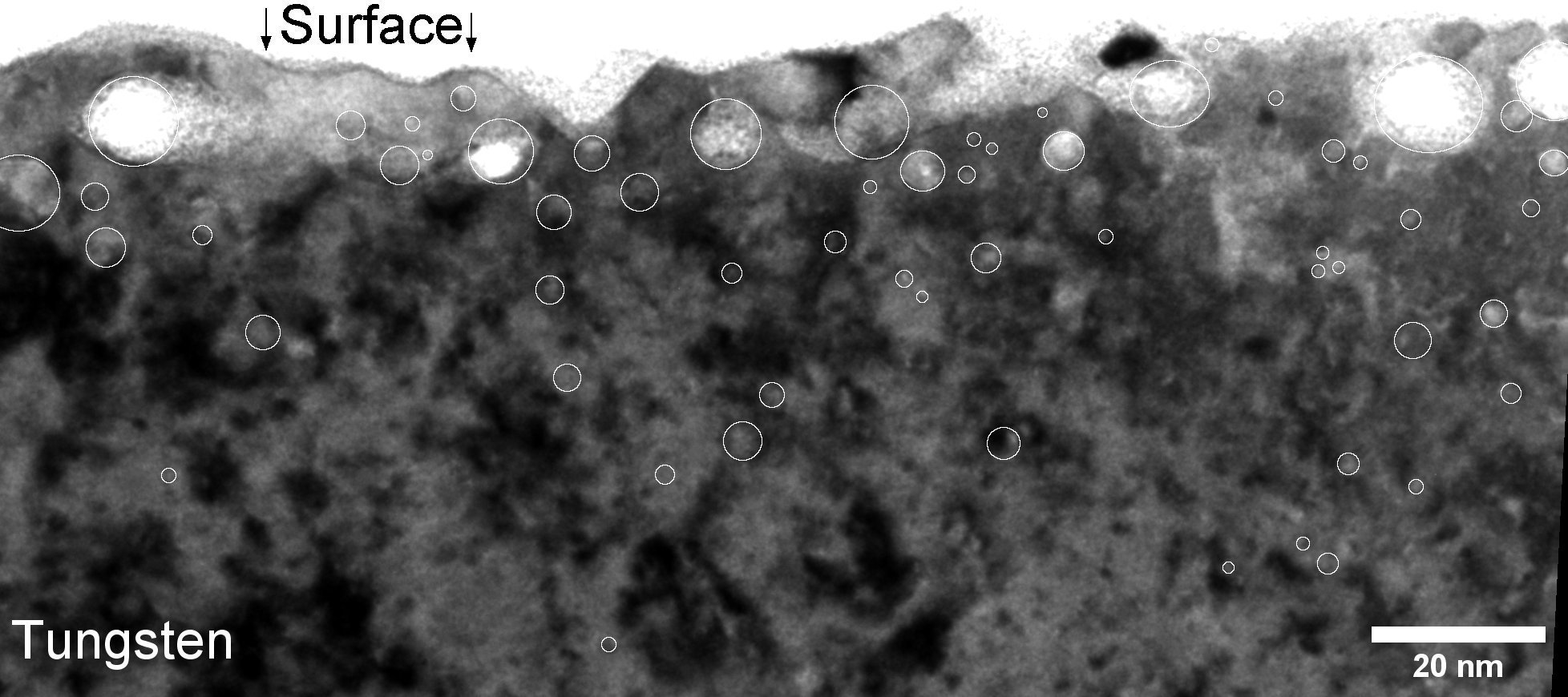
Mykola Ialovega's PhD research
75 eV He at 2.3×1022 m−2 s−1 and 1053 K for 13 s
How to couple this to H transport?
Ialovega's experiment
He exposure
D exposure
Thermo-desorption
Repeat 5 times
W
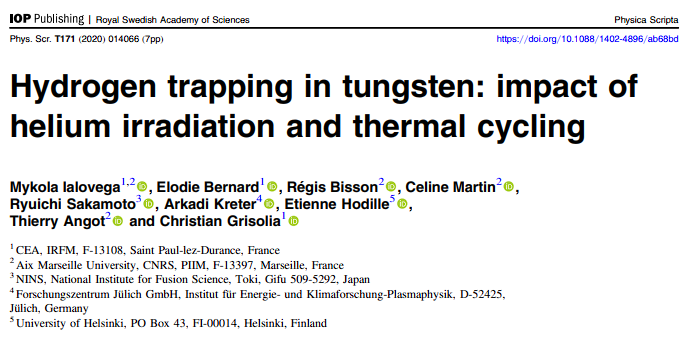
Experimental conditions
Sample: 100 μm W
Pre-damage: 75 eV He at 2.3×1022 m−2 s−1 and 1053 K for 13 s
Initial cleaning TDS after He implantation up to 870 K
D exposure: 250 eV at room temperature
Flux: 1.7×1016 m−2 s−1
Fluence: 4.5×1019 m−2
TDS temperature ramp: 1 K/s
Ialovega's experiment
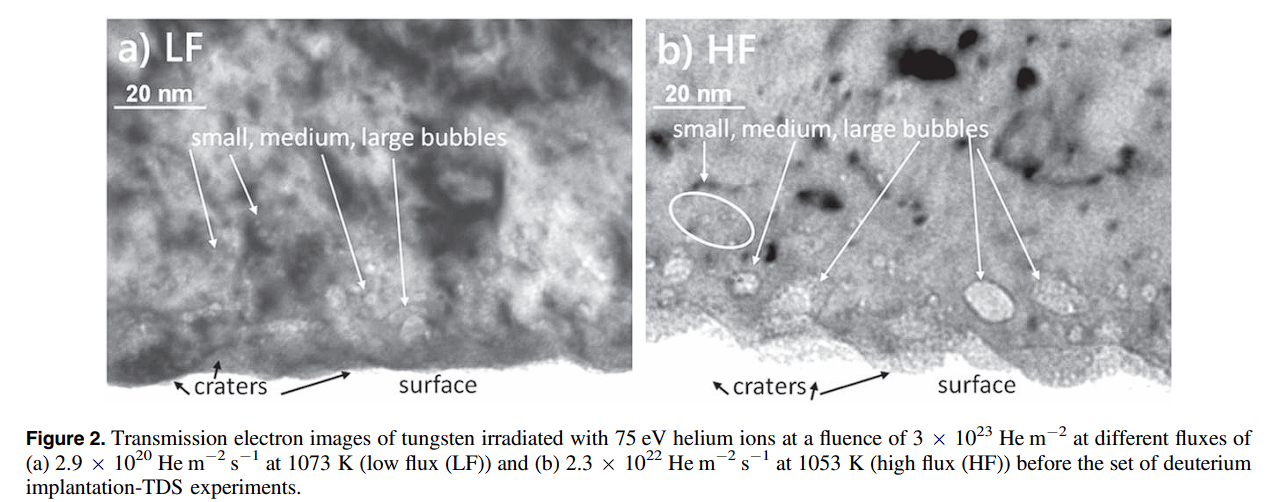
Ialovega's experiment
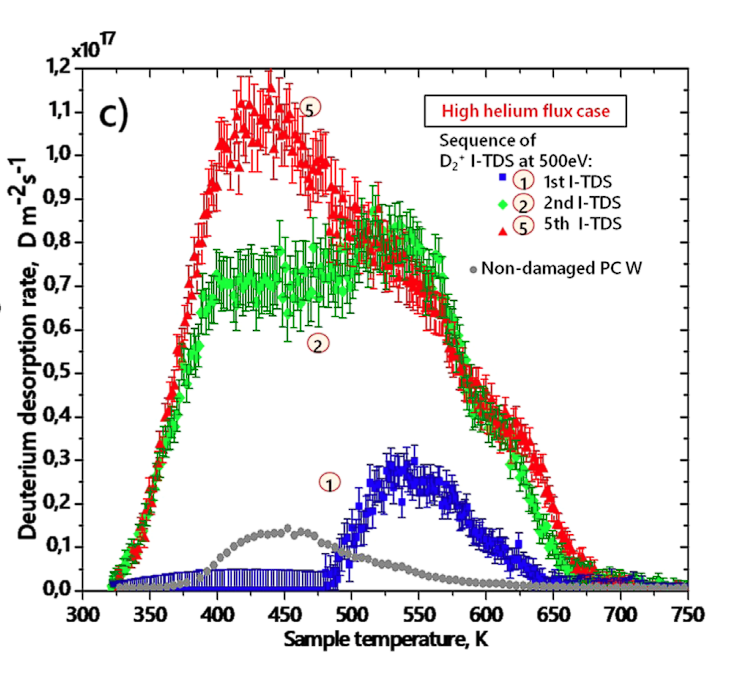
Lack of control experiment
❌No initial TDS
❌No cycling
❌Not performed at the time of the experiment
→ Can only be compared qualitatively!
Ialovega's experiment
Ialovega's experiment
Ialovega's experiment
Correlation He release/D release
Hypotheses
- Helium doesn't desorb from bubbles
- Pre-existing defects exist (suggested by further analysis)
- Helium can saturate traps for Deuterium
surface coverage of trapping sites (tuning parameter)
bubbles radius
bubbles area
The He model was weakly coupled to FESTIM
The model reproduced the experiment
Bubble-induced trap
- 4 traps including a bubble-induced trap
- TDS 1: density of available pre-existing traps is zero
- TDS 2-5: density of pre-existing traps ≈2×10−3 at.fr.
- f is unchanged 3×1018 m−2
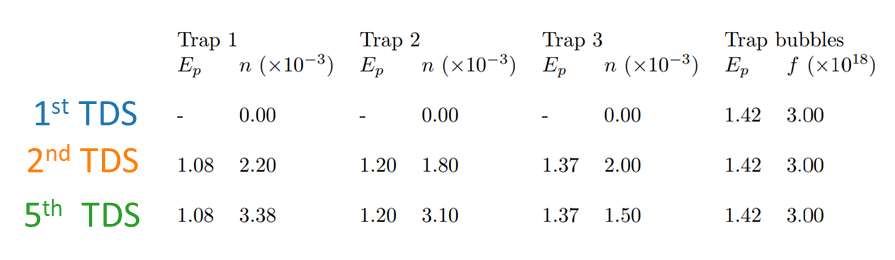
Initial state
Pre-existing defects
He implantation
He implantation
1st D implantation
1st D implantation
1st TDS
He is removed from pre-existing defects
D is desorbed from bubbles
2nd D implantation
2nd D implantation
2nd D implantation
2nd TDS
D desorption from secondary defects
Repeat...
Impact on divertor inventory?
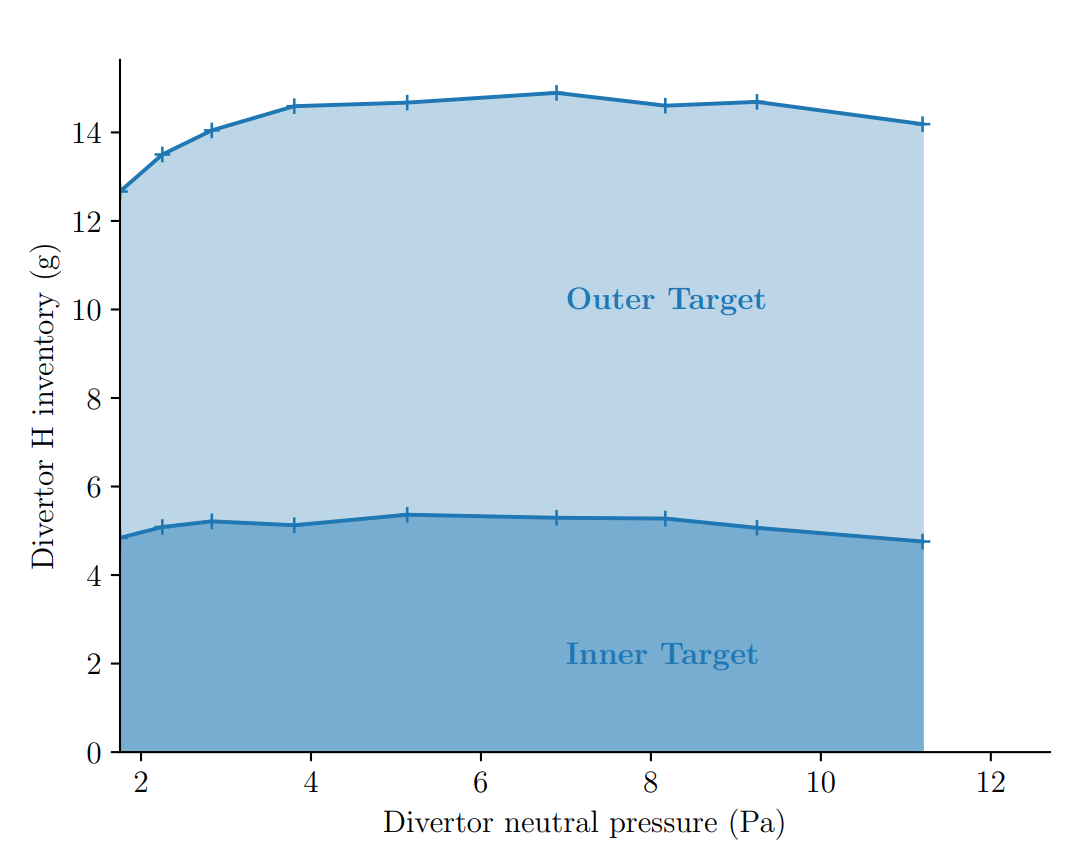
Divertor H inventory (g) at t=107s
Increased trapping (bubbles)
Reduced trapping
(trap saturation)
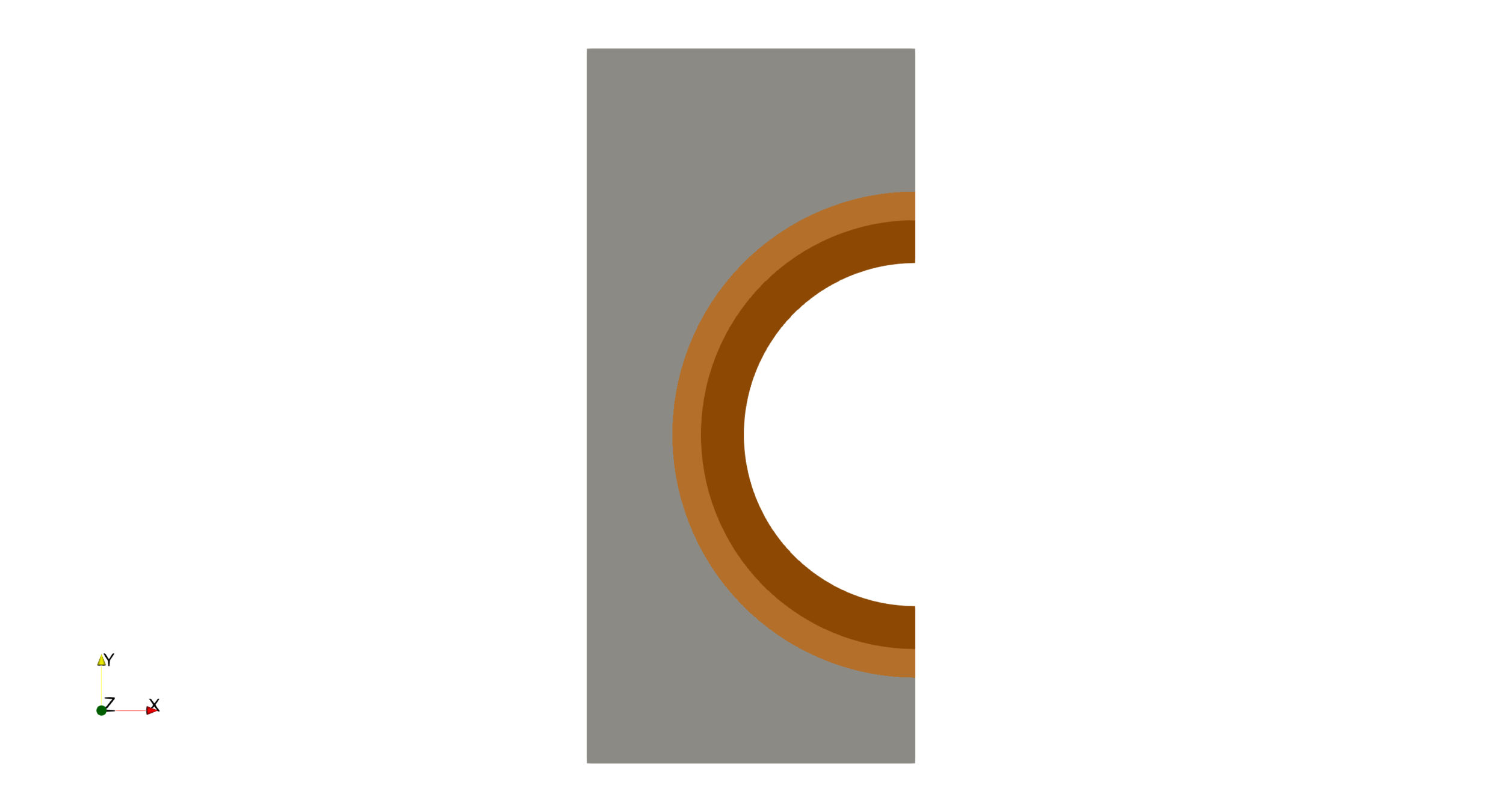
Divertor inventory could be even lower
Main conclusions
- FESTIM was developed to assess T inventory in ITER plasma facing components and is now used for other applications (breeding blankets, experimental work...).
- Under conservative assumptions, the T inventory in the ITER divertor is below 1% of the in-vessel safety limit after 25 000 pulses.
- Results suggest that the presence of helium could reduce this inventory further by saturating traps.
Where to go from here?
- Validate the monoblock model with experimental data
- Retention in Be co-deposited layers will domitate the inventory
- Confirm/infirm our interpretation of the He-H interactions
- Investigate other in-vessel components like breeding blankets
Adding bubble bursting
Thank you for your attention
Any questions?
Hydrogen transport in tokamaks
By Remi Delaporte-Mathurin
Hydrogen transport in tokamaks
This is the presentation I gave at my PhD defense on the 17th of October 2022
- 407