Epidemiology of representations
an empirical approach
École Doctorale Cerveau-Cognition-Comportement (ED3C, ED n°158)
Centre d’Analyse et de Mathématique Sociales (CAMS, UMR 8557 EHESS-CNRS)
Sébastien Lerique
Pr. Russell Gray
Pr. Fiona Jordan
Dr. Márton Karsai
Pr. Jean-Pierre Nadal
Pr. Sharon Peperkamp
Pr. Camille Roth
Dr. Mónica Tamariz
Reviewer
Reviewer
Examiner
Supervisor
Examiner
Co-supervisor
Examiner
Thesis submitted for the degree of Ph.D. in Cognitive Science
27th October 2017
Cultural evolution
significant transformations
an epidemiology of representations
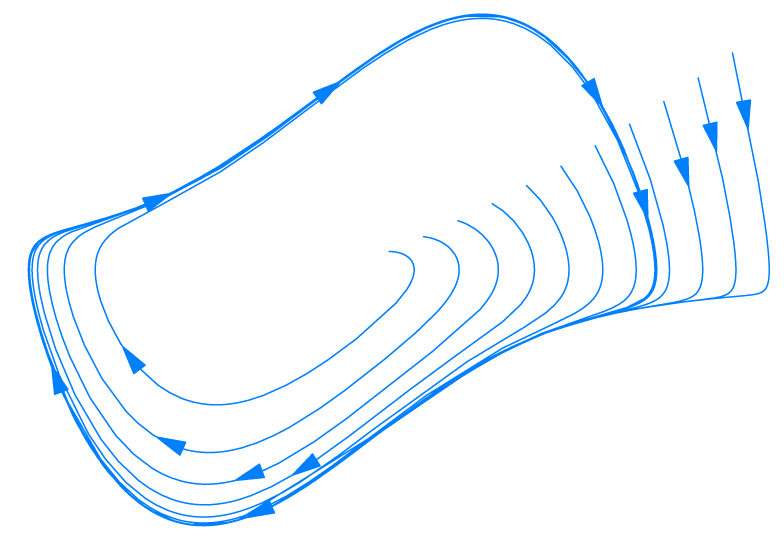
⟹
Evolutionary principles for culture
Parallel genetic and cultural change
Cultural Attraction Theory (Sperber 1996)
Standard evolutionary theory
Cognitive science
Anthropology
Standard Cultural Evolution
Cavalli-Sforza and Feldman (1981)
Boyd and Richerson (1985, 2005)
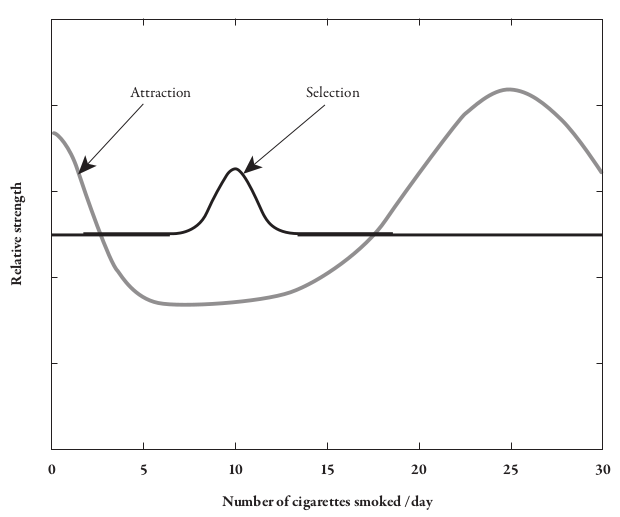
Claidière and Sperber (2007)
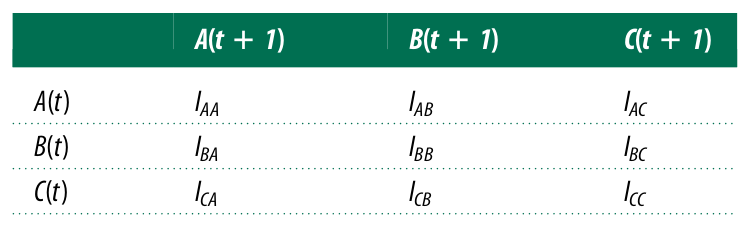
Claidière, Scott-Phillips, and Sperber (2014)
Transformations of linguistic representations
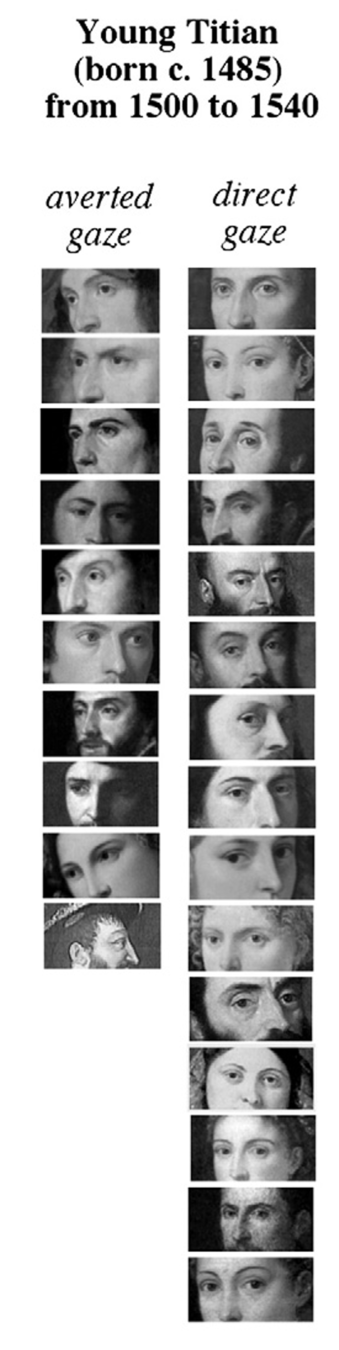
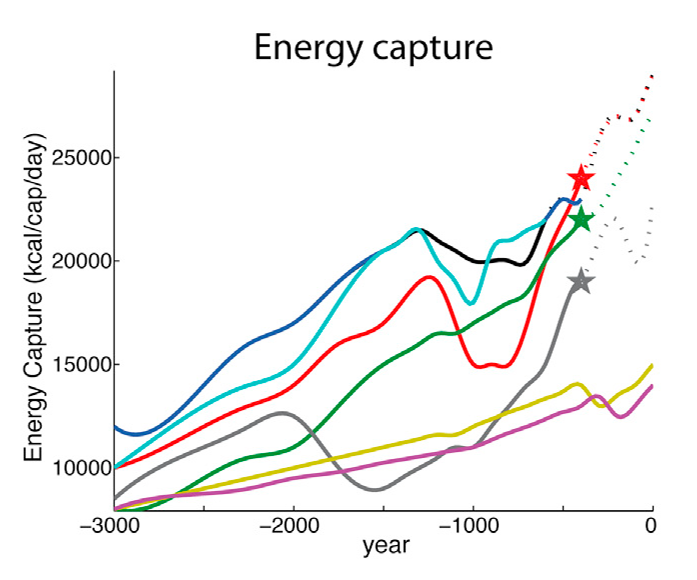
Morin (2013)
Baumard et al. (2015)
IN VIVO
Claidière et al. (2014)
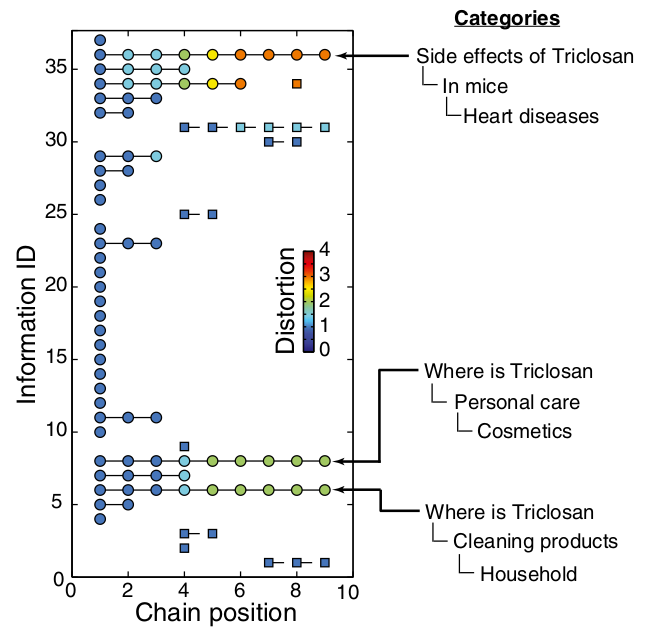
Moussaïd et al. (2015)
IN VITRO
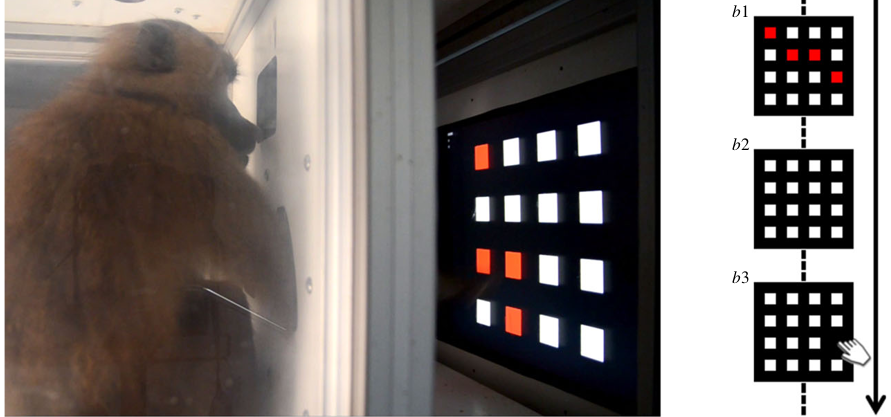
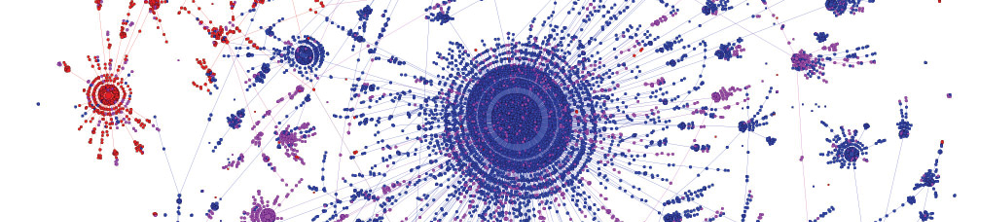
Adamic et al. (2016)
Historical data
Online data
Transmission chains
Empirical study of cultural evolution
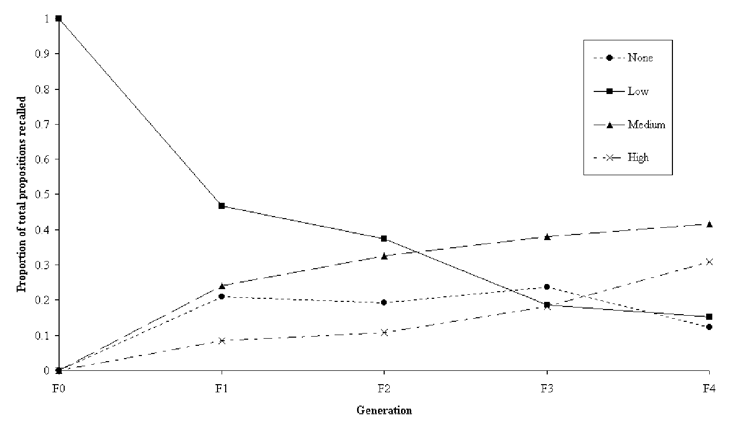
Mesoudi and Whiten (2004)
In vivo online data
Corpus of quotations from a large body of (8.5m) blog posts
August '08 to April '09 (Leskovec et al., 2009)
Groups (and dynamics) of sentences
Clusters | 71.6k | 45.7k |
Quotes | 310k | 128k |
Occurrences | 8.16m | 2.43m |
Raw
Cleaned
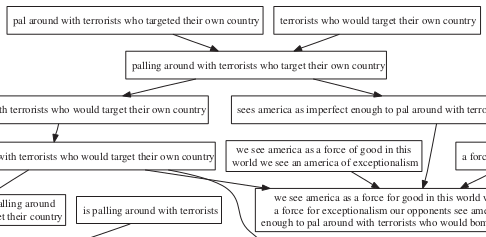
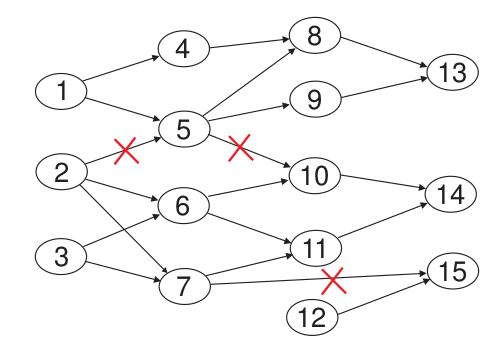
frequency
age of acquisition
#letters
#synonyms
clustering
orthographic neighbourhood density
Pakistani President Asif Ali Zardari:
– “we will not be scared of these cowards”
⭢ “we will not be afraid of these cowards”
US Senator McCain:
– “I admire Senator Obama and his accomplishments”
⭢ “I respect Senator Obama and his accomplishments”
Sentence reformulations
Word features
#phonemes
#syllables
phonological neighbourhood density
betweenness
degree
pagerank
frequency
age of acquisition
#letters
#synonyms
clustering
orthographic neighbourhood density
#phonemes
#syllables
phonological neighbourhood density
betweenness
degree
pagerank
And word list recall
Deese (1959), Roediger and McDermott (1995) paradigm
Zaromb et al. (2006)
Similar to sentence recall
Potter and Lombardi (1990)
Address the word frequency paradox
Mandler et al. (1982)
Psycholinguistics
Semantic network
Substitution model
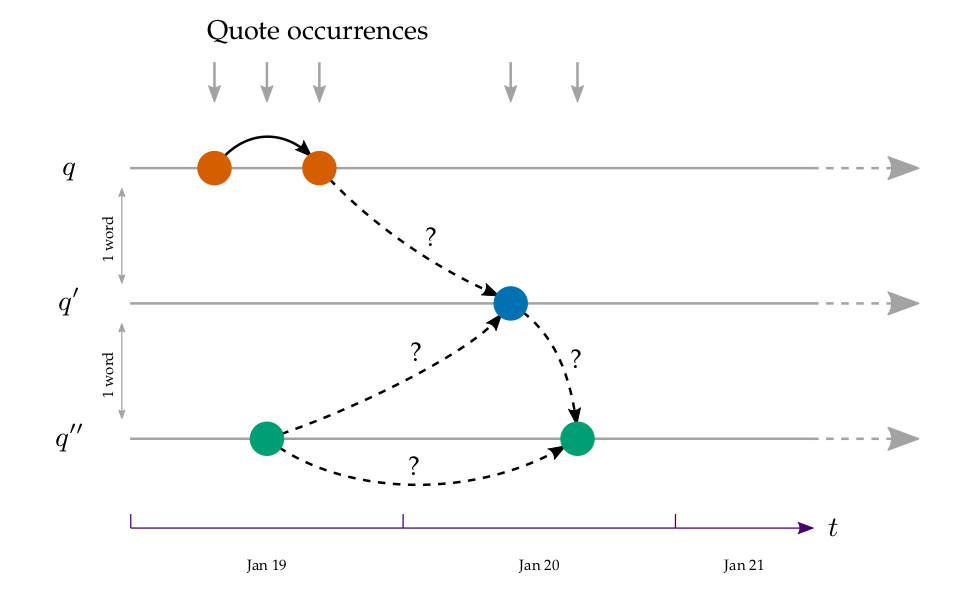
Time: continuous / discrete
Source: all / majority
Past: all / last bin
Destination: all / exclude past
Time: continuous / discrete
Source: all / majority
Past: all / last bin
Destination: all / exclude past
6177 substitutions
Susceptibility
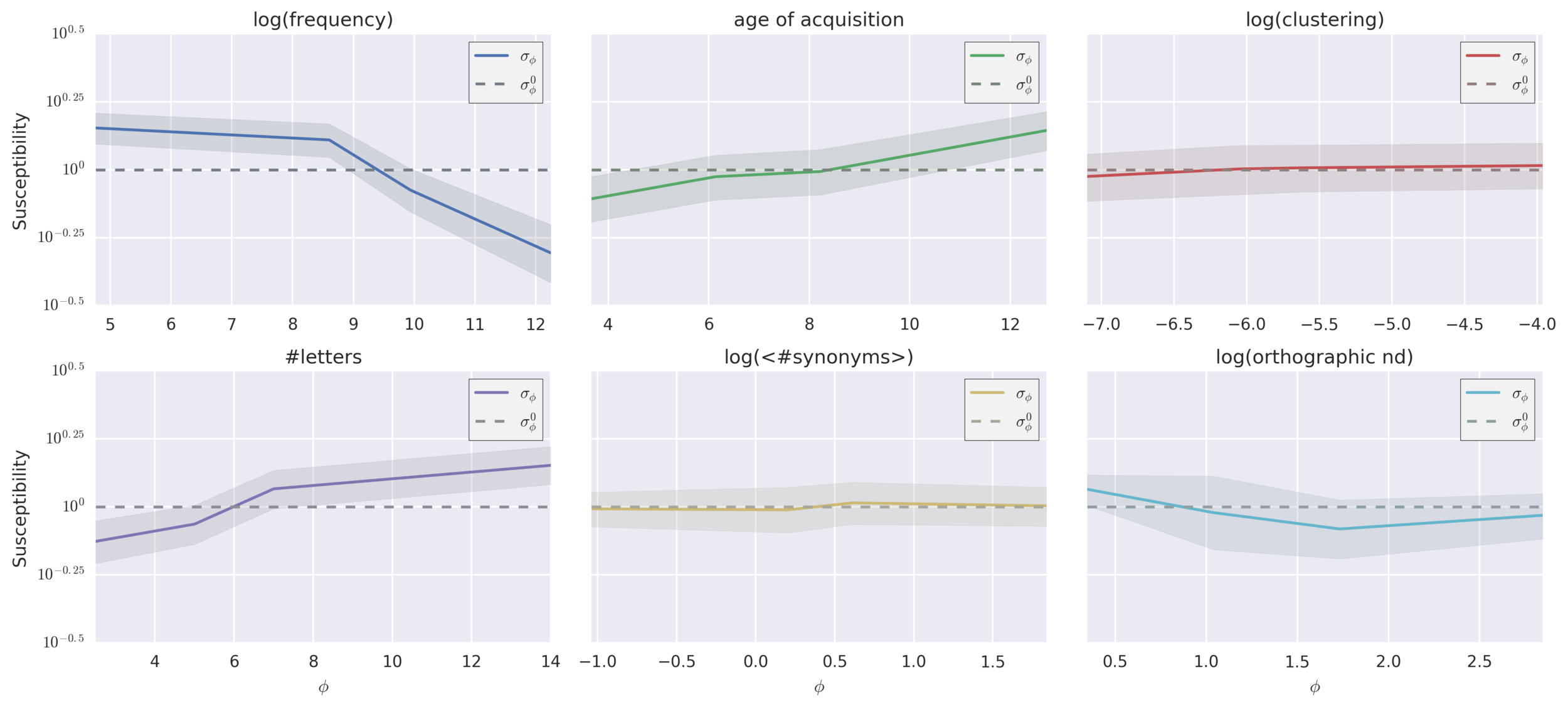
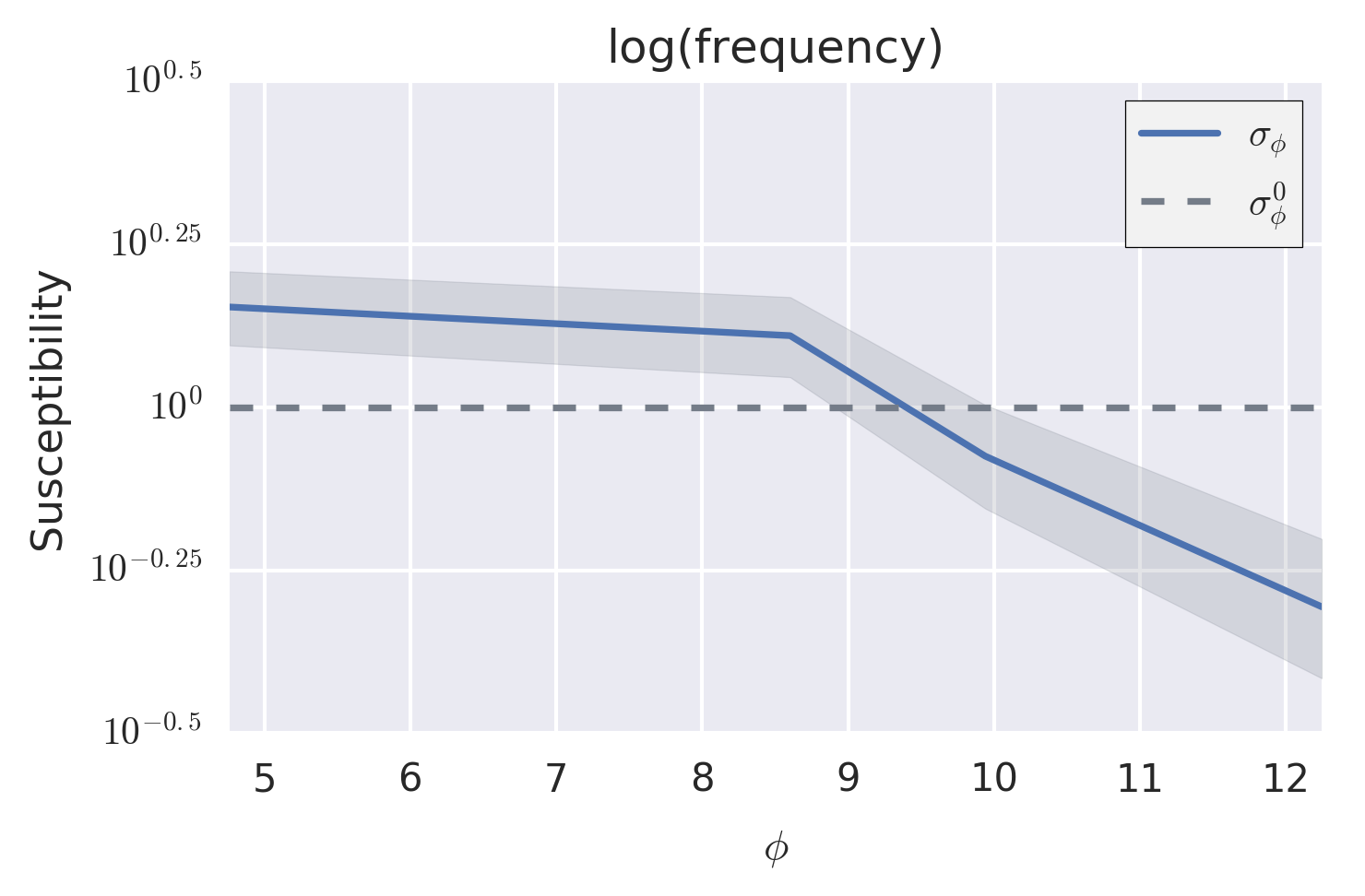
“This crisis did not develop overnight and it will not be solved overnight”
US President Bush
Replacement word
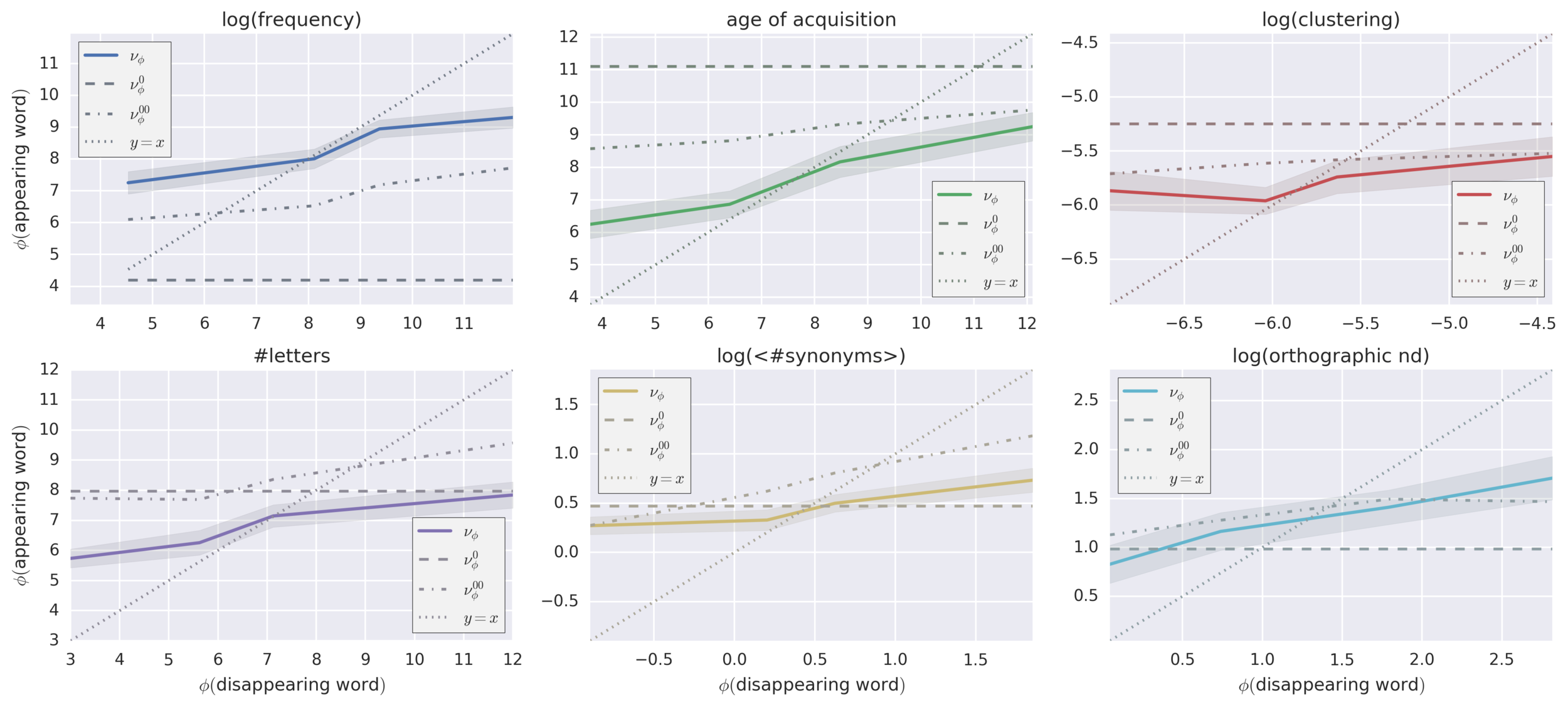
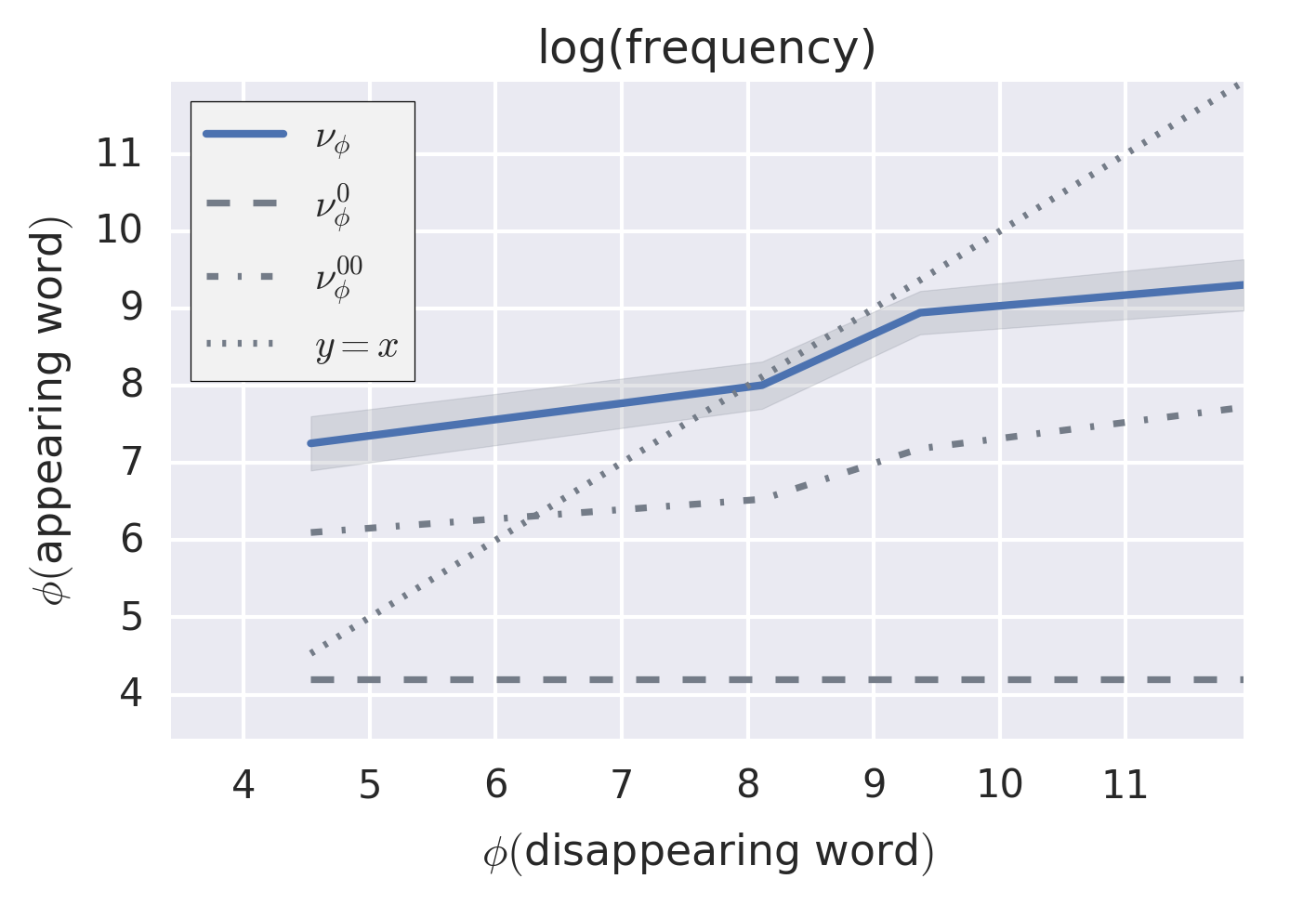
“This crisis did not develop overnight and it will not be solved overnight”
US President Bush
“This problem did not develop overnight and it will not be solved overnight”
Sentence context
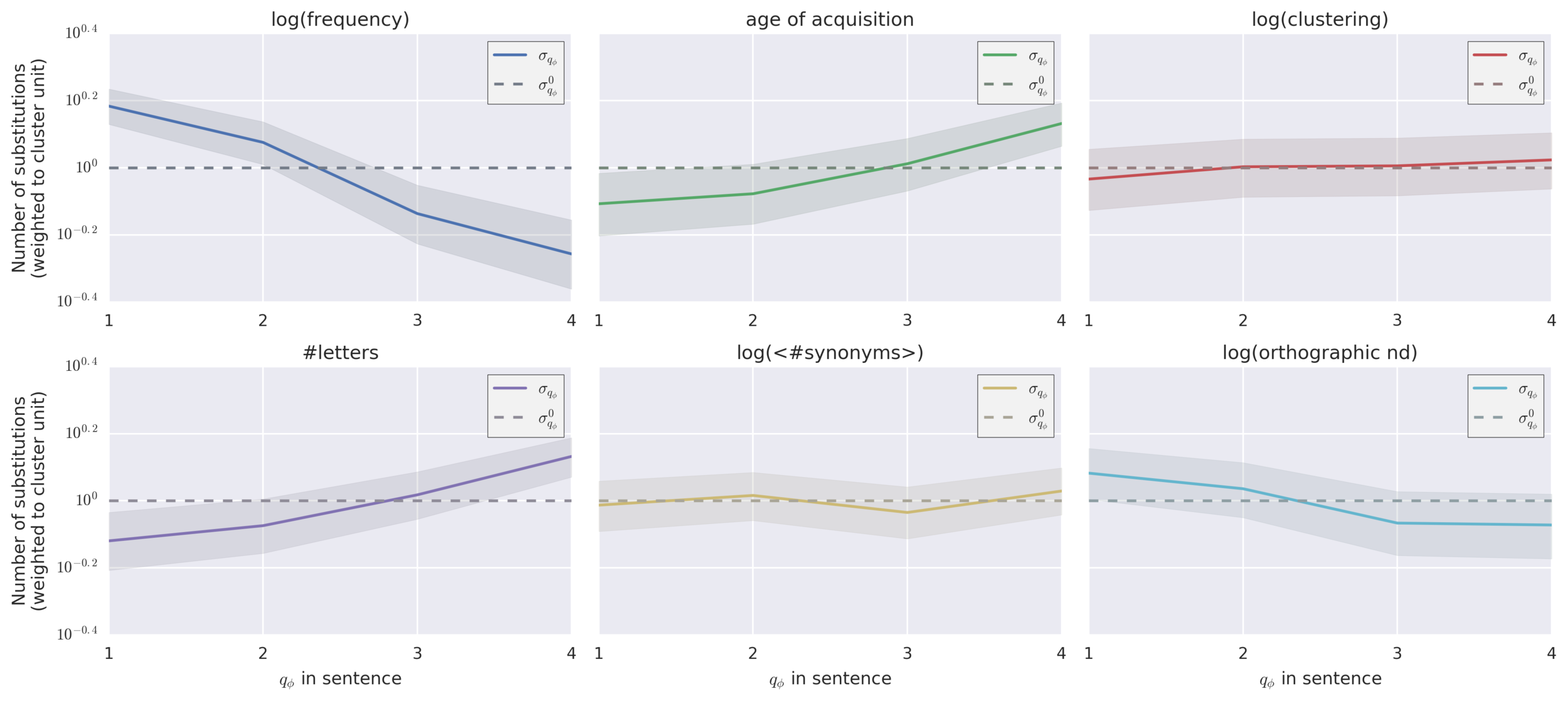
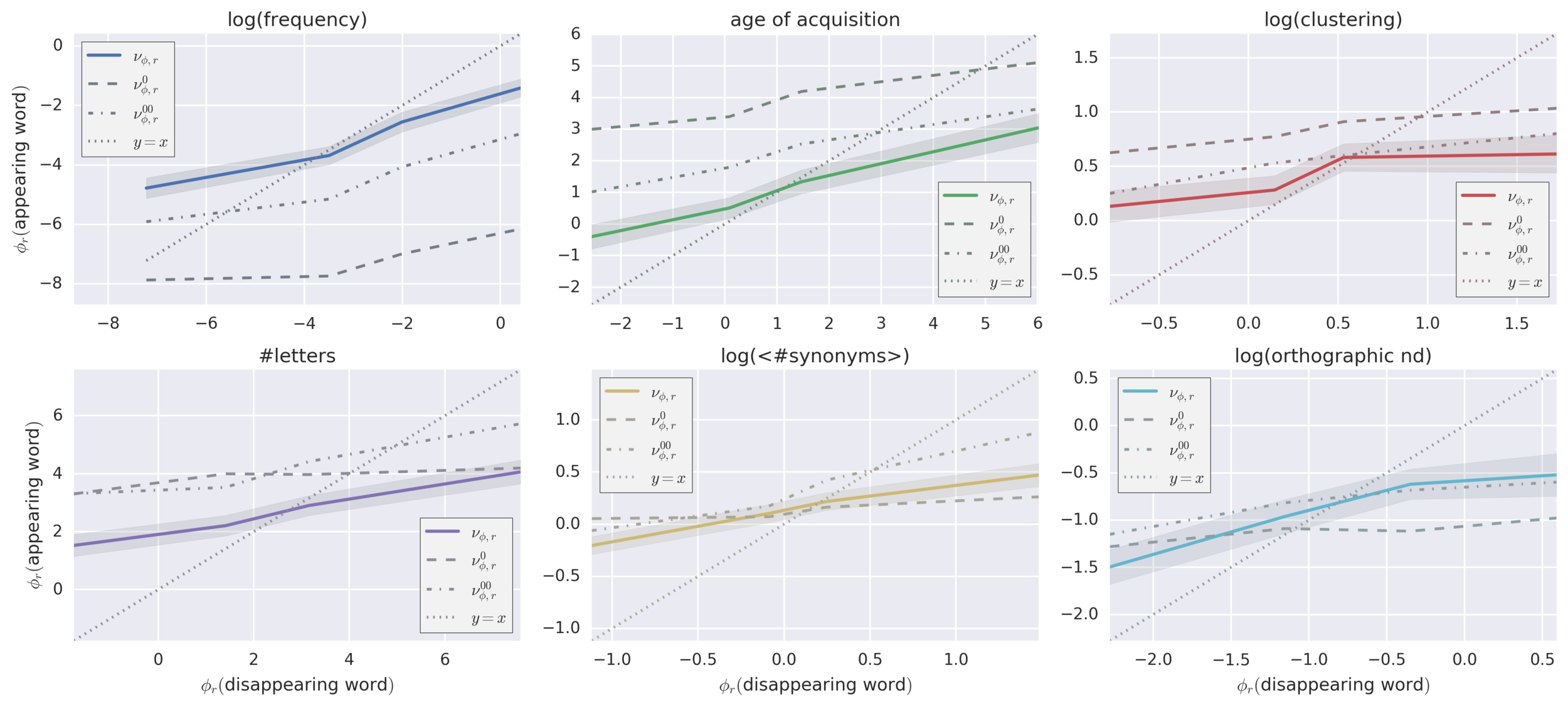
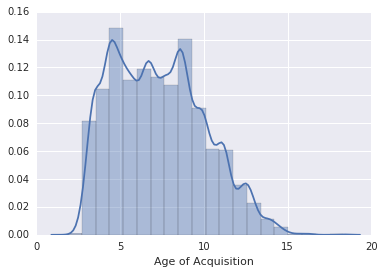
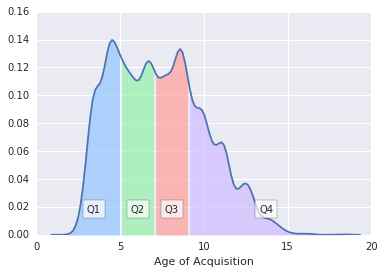
Susceptibility
Feature variation
“Senior general Than Shwe is foolish with power”
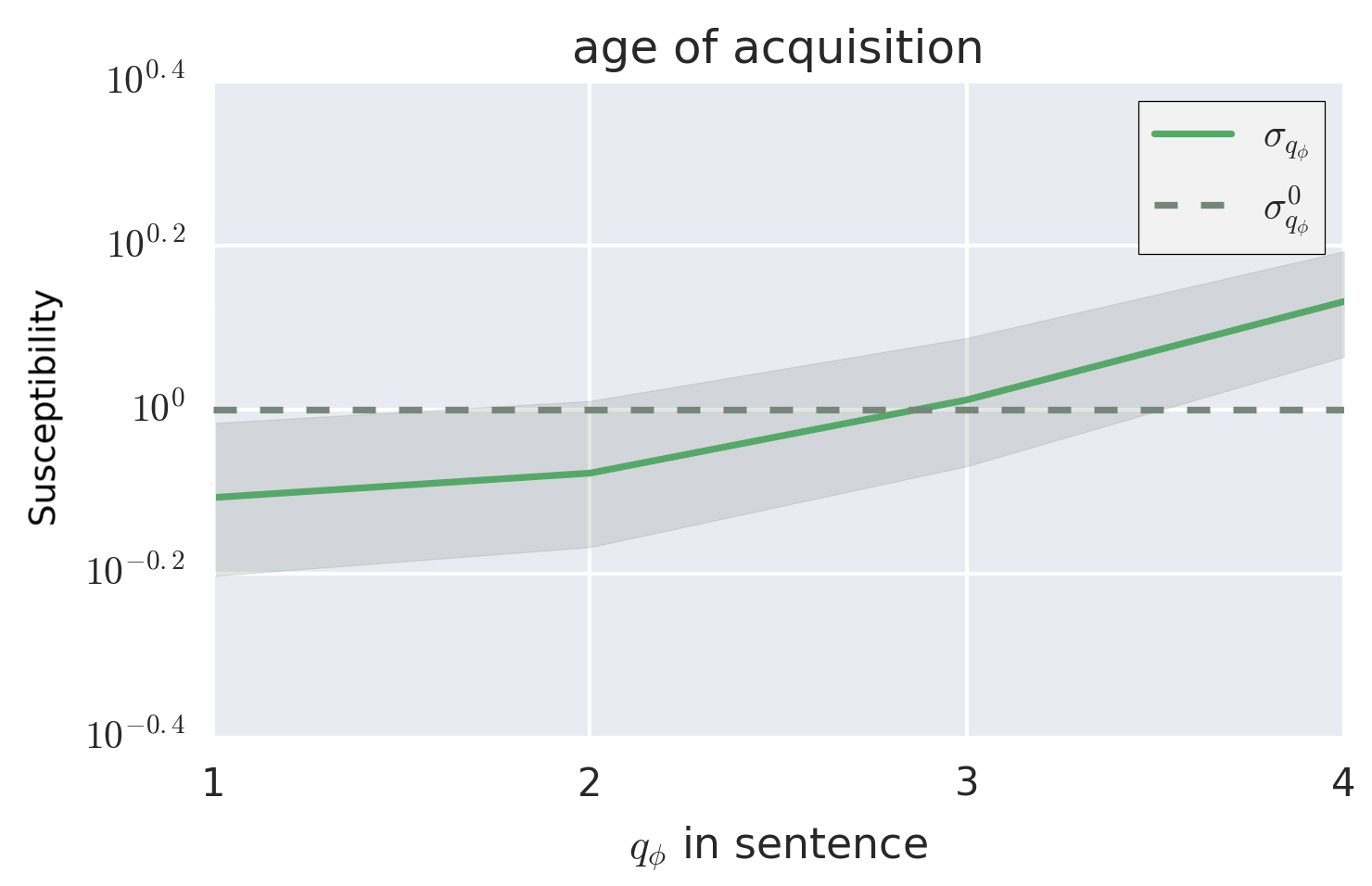
crazy
Stepping back
In vivo psycholinguistics experiment
Transformations → Substitutions
Attractors
Contractile behaviour
Towards words easier to recall
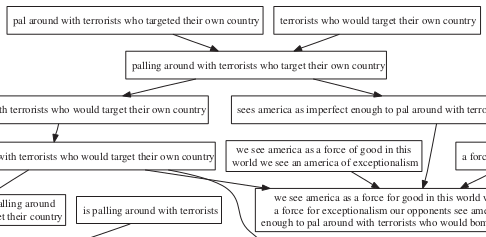
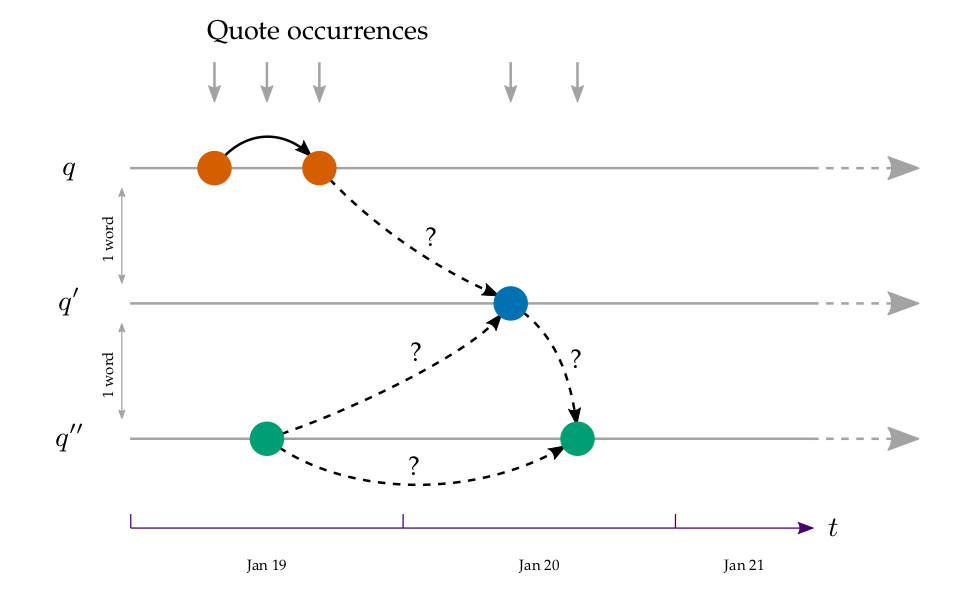
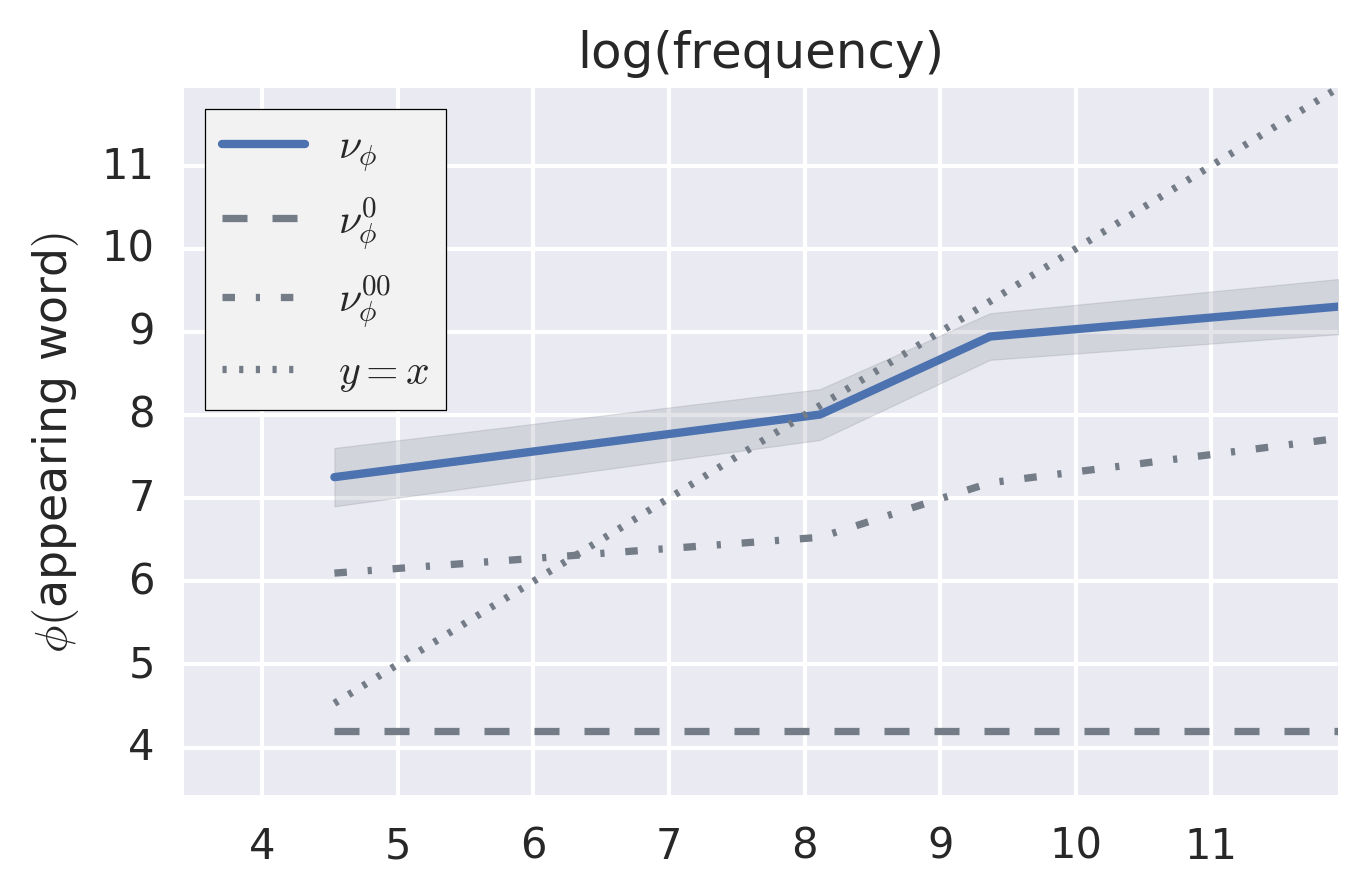
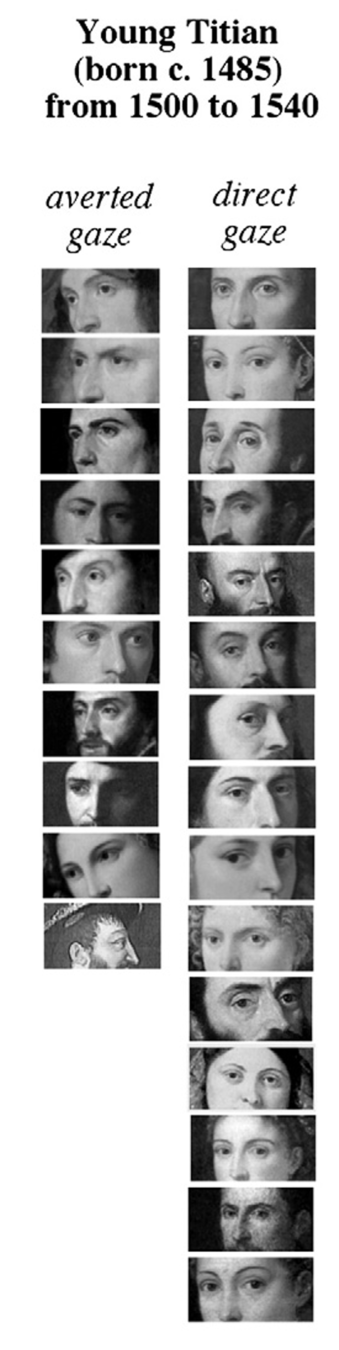
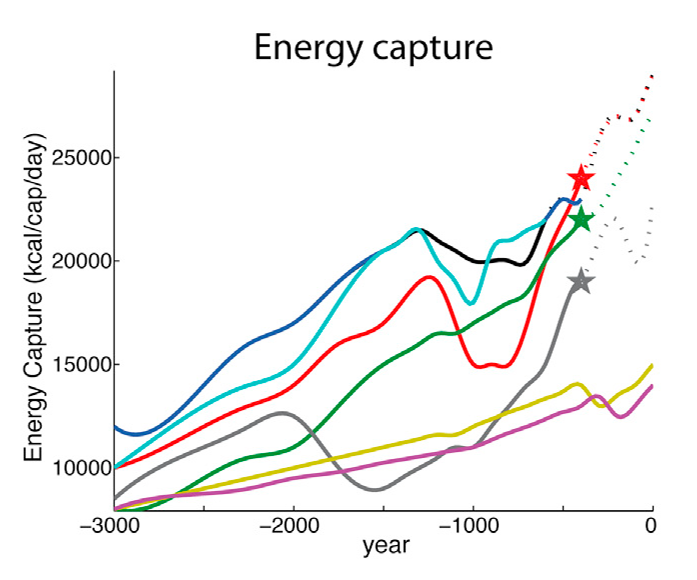
IN VIVO
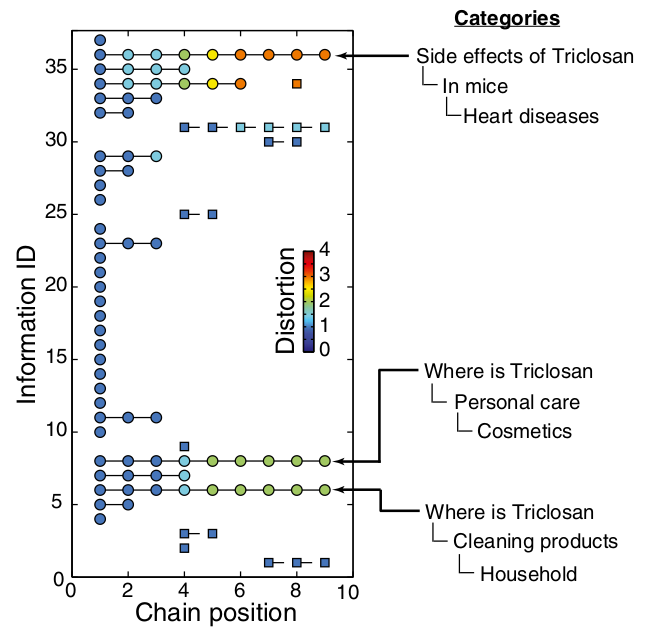
IN VITRO
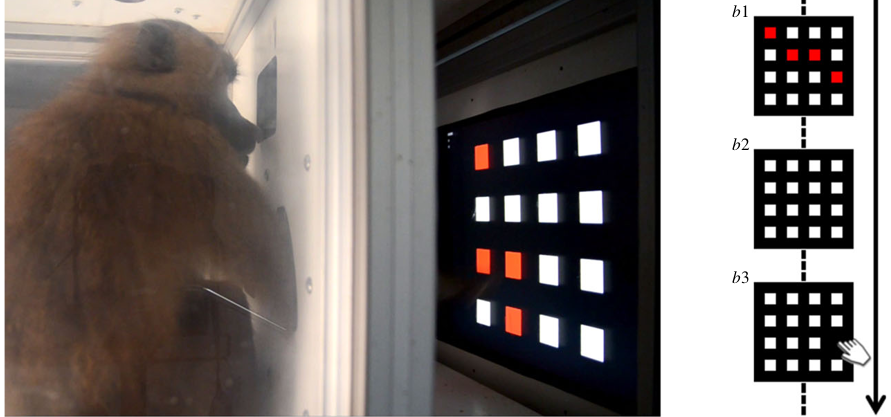
Historical data
Transmission chains
Long term evolution
Control over data generation
No complexity sacrifice
Low-level cognitive biases in the wild
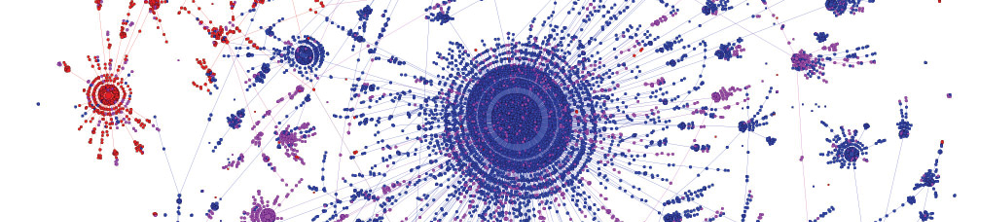
Online data
Empirical bind
Realistic content
Control over data-generation
Computational analysis
Already coded
Do-it-by-hand
Simple setting
Danescu-Niculescu-Mizil et al. (2012)
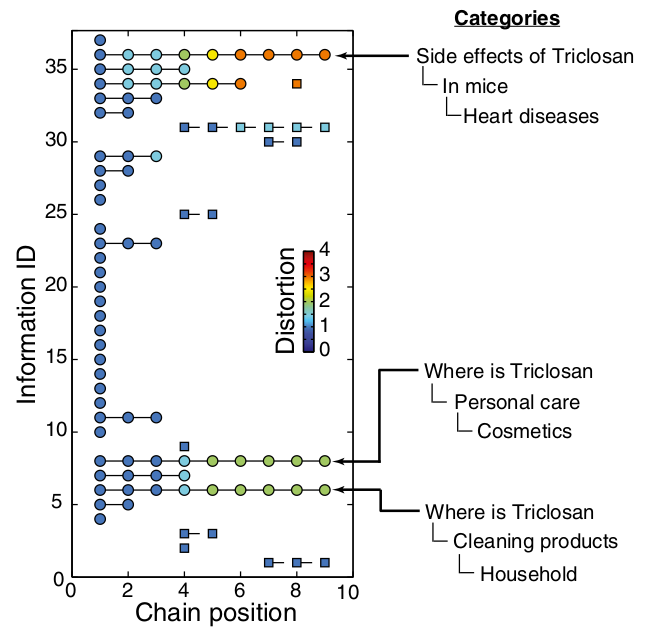
Moussaïd et al. (2015)
Lauf et al. (2013)
Cornish et al. (2013)
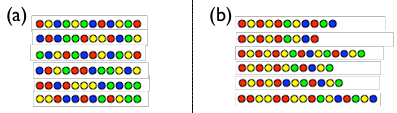
Claidière et al. (2014)
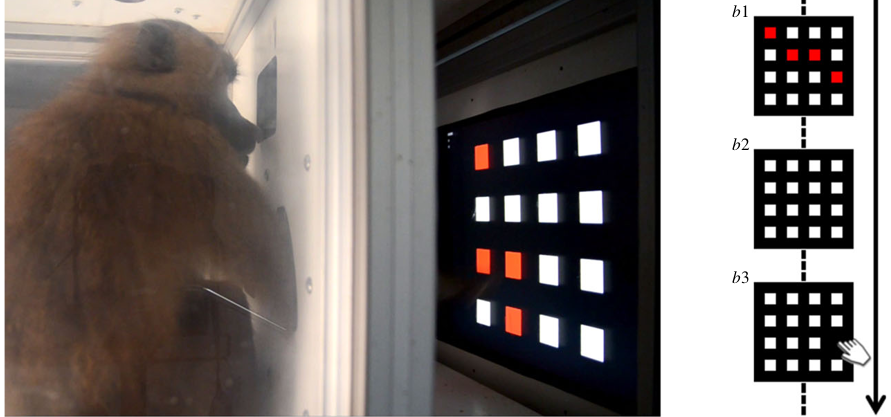
Web experiments
Sequence alignments
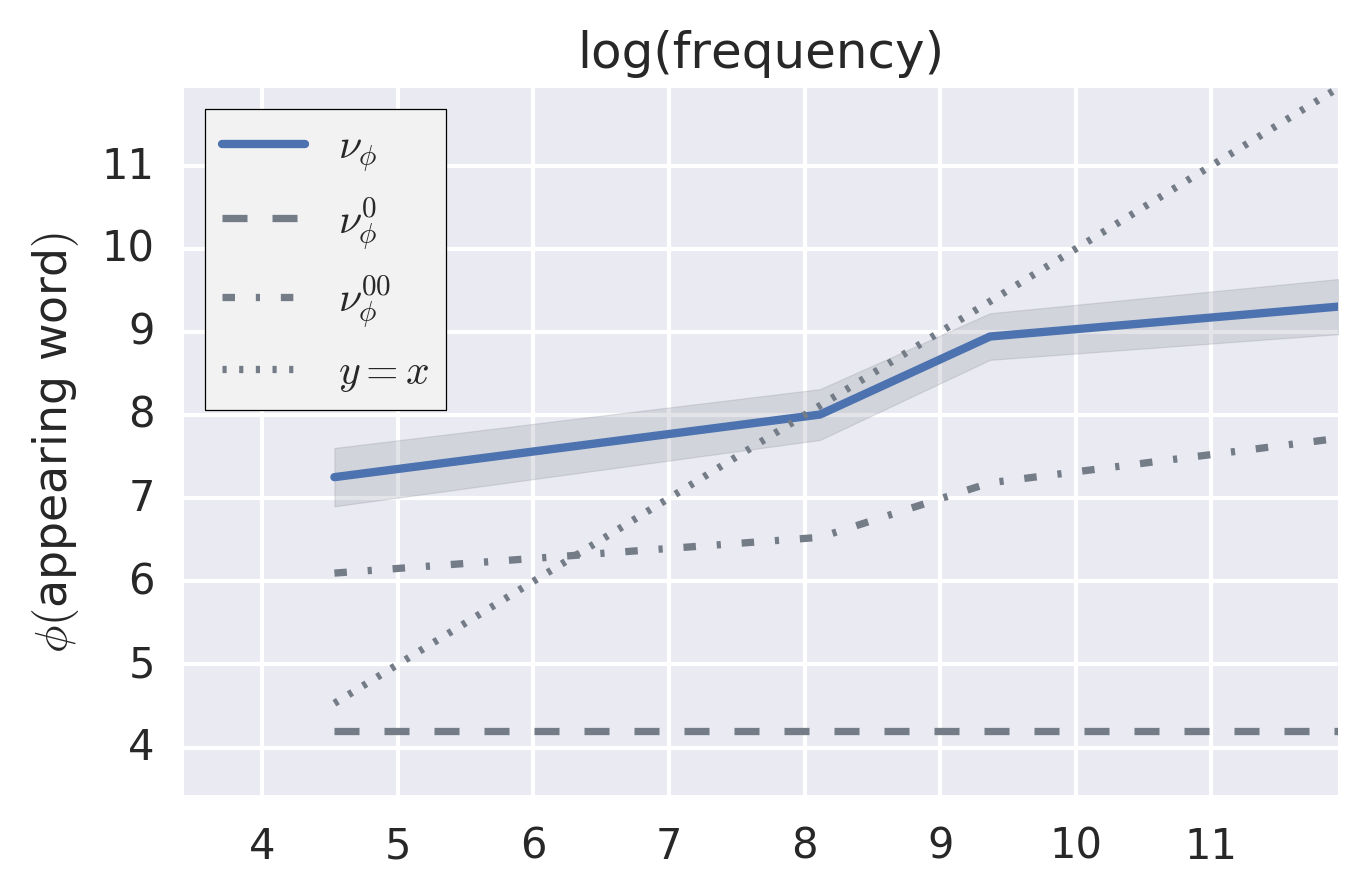
Case study 1
Requirements fulfilled
Control over experimental setting
Fast iterations
Scale
Exp. 1
MemeTracker, WikiSource,
12 Angry Men, Tales,
News stories
Exp. 2
Memorable/non-memorable quote pairs
(Danescu-Niculescu-Mizil et al., 2012)
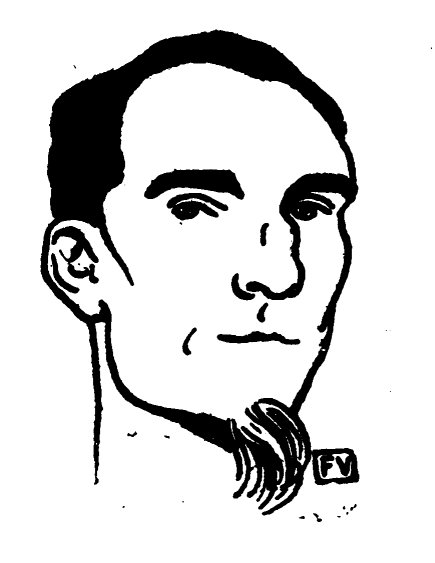
Exp. 3
Nouvelles en trois lignes
(Fénéon, 1906)
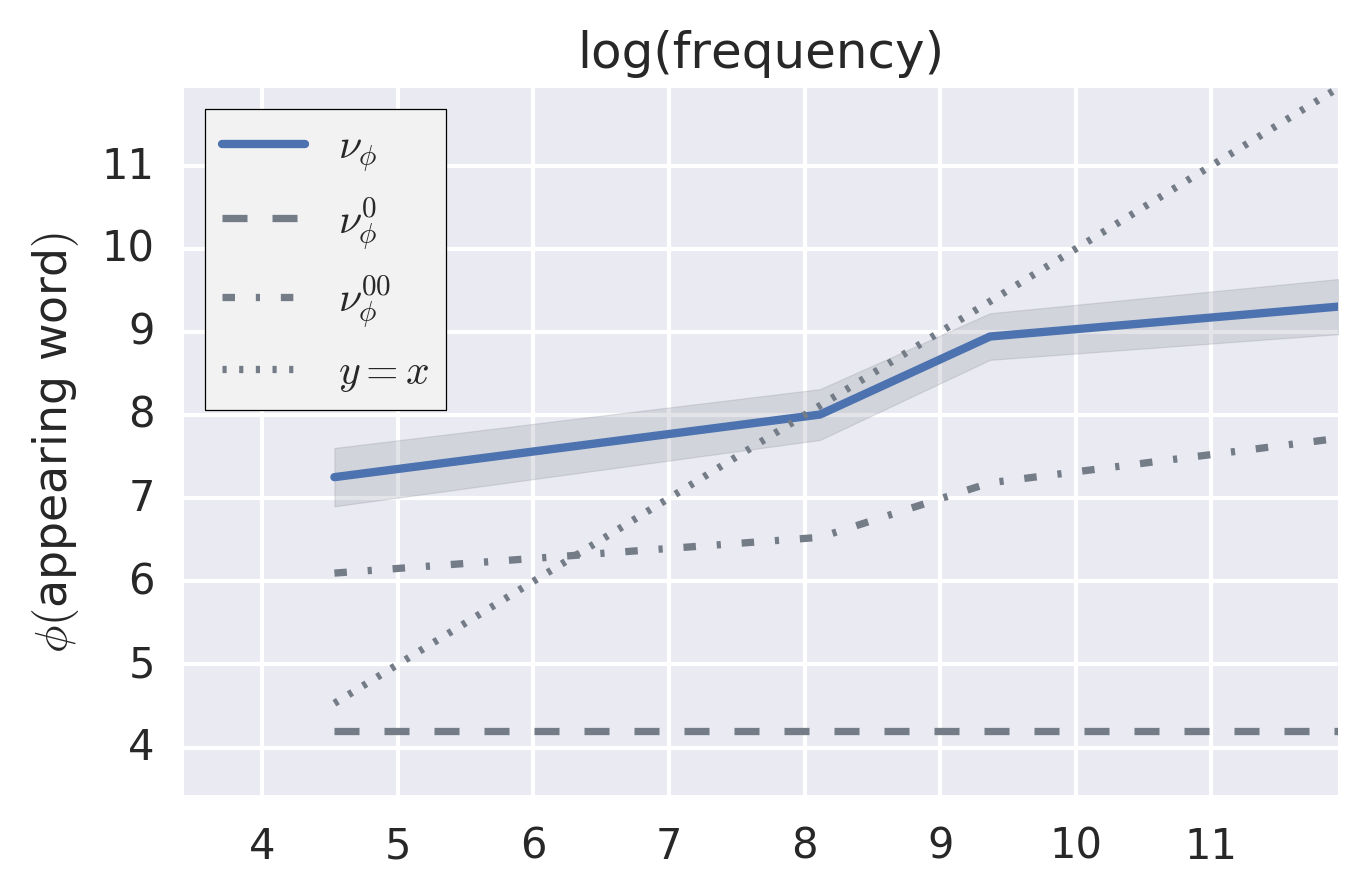
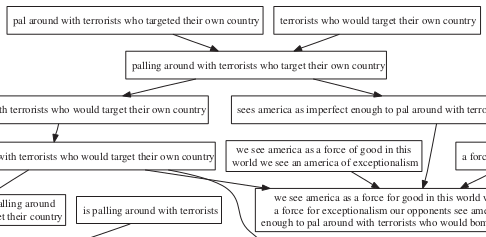
Web-based experiments
Experiment setup
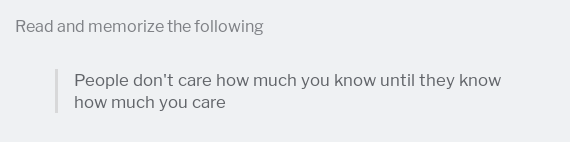

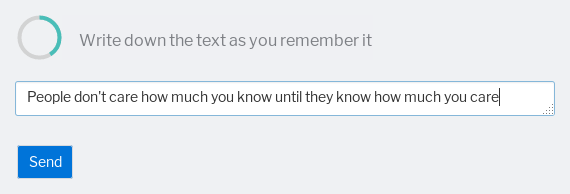
reading and writing time ∝ number of words
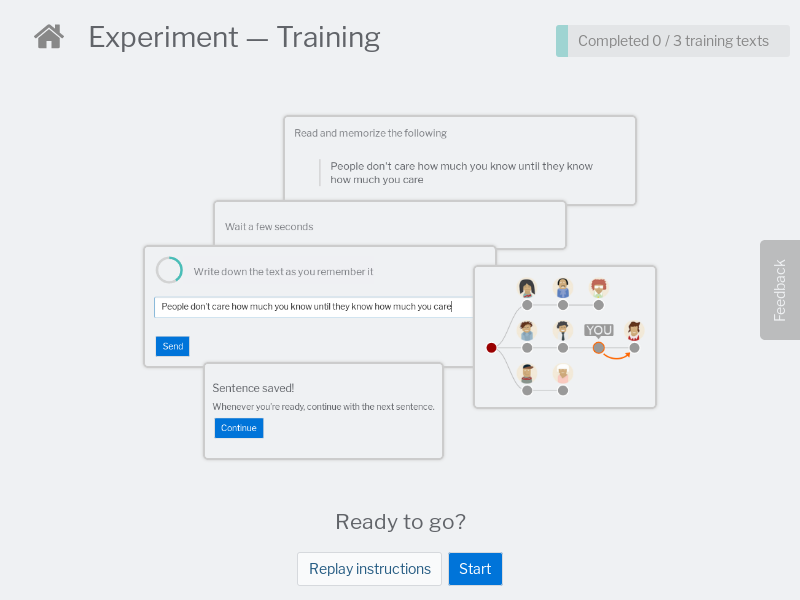
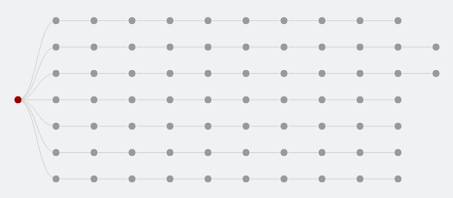
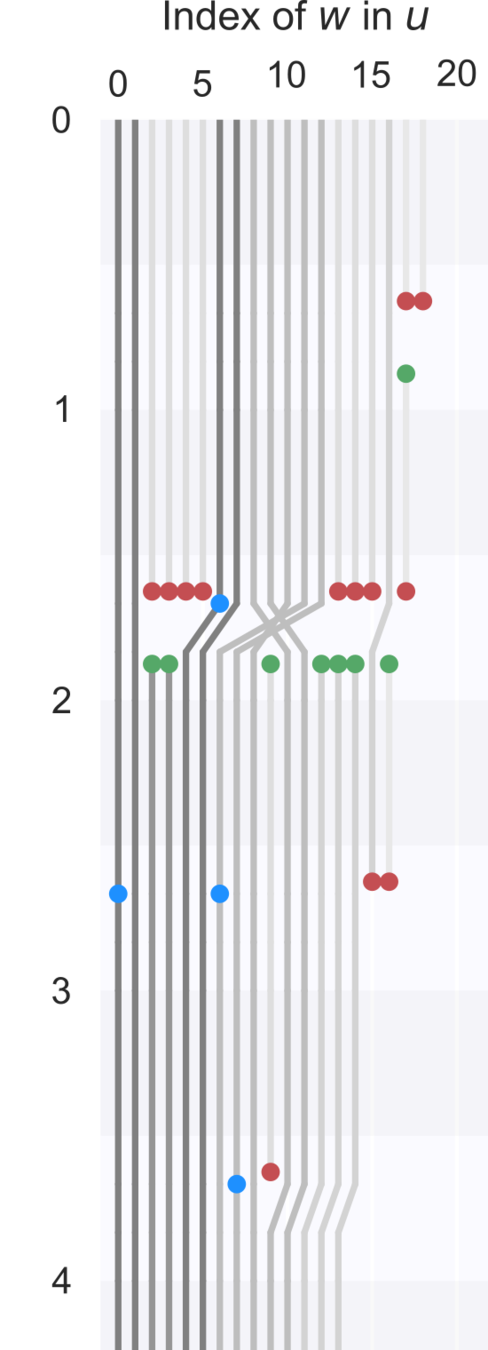
Transformation model
At Dover, the finale of the bailiffs' convention. Their duties, said a speaker, are "delicate, dangerous, and insufficiently compensated."
depth in branch
At Dover, the finale of the bailiffs convention,their duty said a speaker are delicate, dangerous and detailed
At Dover, at a Bailiffs convention. a speaker said that their duty was to patience, and determination
In Dover, at a Bailiffs convention, the speaker said that their duty was to patience.
In Dover, at a Bailiffs Convention, the speak said their duty was to patience
At Dover, the finale of the bailiffs' convention. Their duties, said a speaker, are "delicate, dangerous, and insufficiently compensated."
At Dover, the finale of the bailiffs convention,their duty said a speaker are delicate, dangerous and detailed
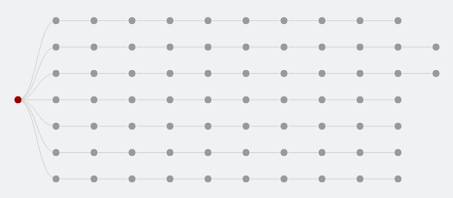
Sequence alignments
Needleman and Wunsch (1970)
AGAACT-
| ||
-G-AC-G
AGAACT
GACG
Finding her son, Alvin, 69, hanged, Mrs Hunt, of Brighton, was so depressed she could not cut him down
Finding her son Arthur 69 hanged Mrs Brown from Brighton was so upset she could not cut him down
Finding her son Alvin 69 hanged Mrs Hunt of - - Brighton, was so depressed she could not cut him down
Finding her son Arthur 69 hanged Mrs - - Brown from Brighton was so upset she could not cut him down
Apply to utterances using NLP
At Dover, the finale of the bailiffs convention, their duty said a speaker are delicate, dangerous and detailed
At Dover, at a Bailiffs convention. a speaker said that their duty was to patience, and determination
At Dover the finale of the - - bailiffs convention - - - - their duty At Dover - - - - at a Bailiffs convention a speaker said that their duty said a speaker are delicate dangerous - - - and detailed - - - - - - - was to patience and - determination
At Dover the finale of the - - bailiffs convention |-Exchange-1------| their duty
At Dover - - - - at a Bailiffs convention a speaker said that their duty
said a speaker are delicate dangerous - - - and detailed -
|-Exchange-1------------------------| was to patience and - determination
said a speaker are delicate dangerous |-E2----|
|E2| a speaker - - - said that
said -
said that
↪E1
↪E2
Extend to build recursive deep alignments
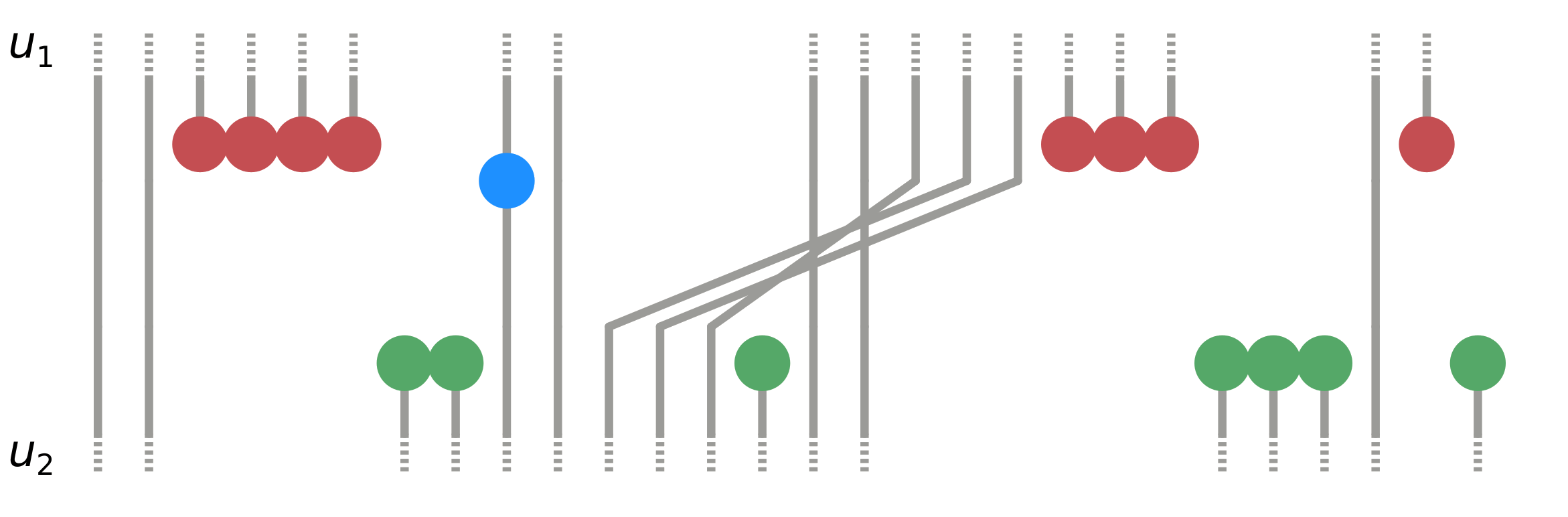
Insertion-deletion chunks
Bursts in branches
Detailed behaviours
Frequency
Frequency
Frequency
|chunk|
|chunk|
Deletion
Insertion
Replacement
Position in u
∣u∣w
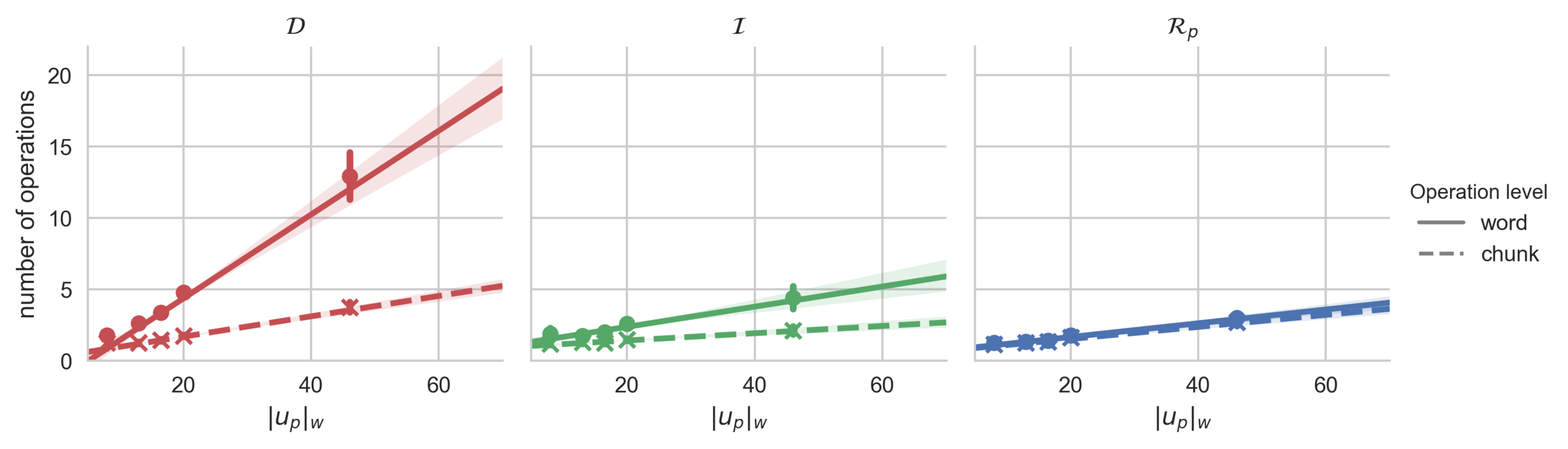
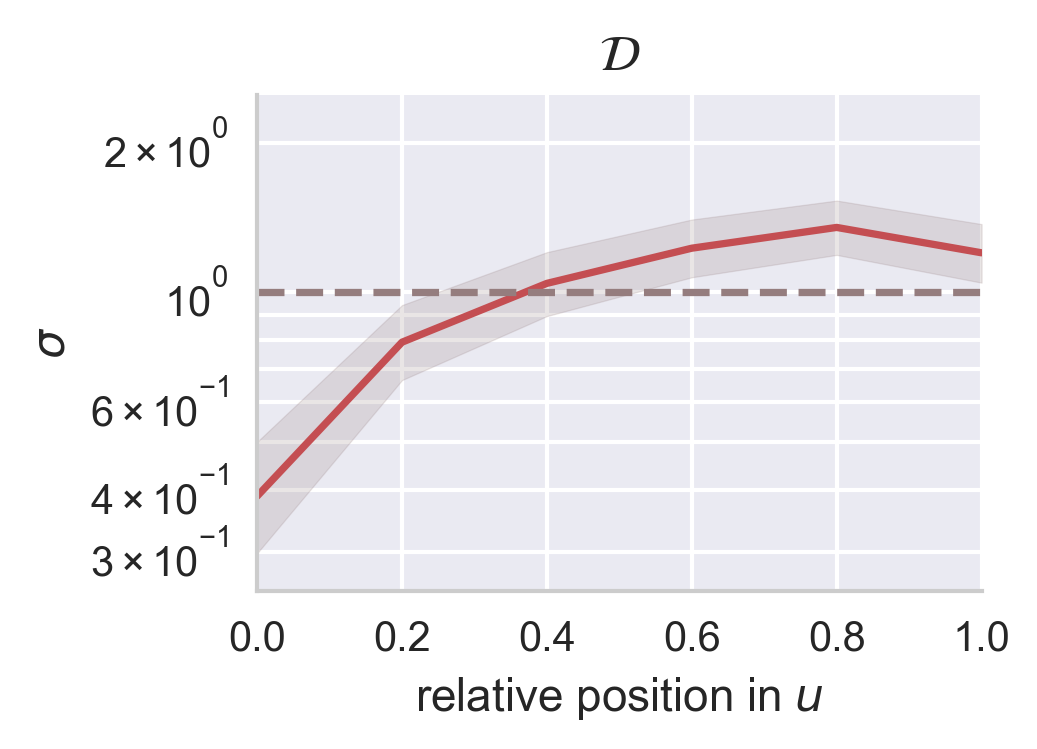
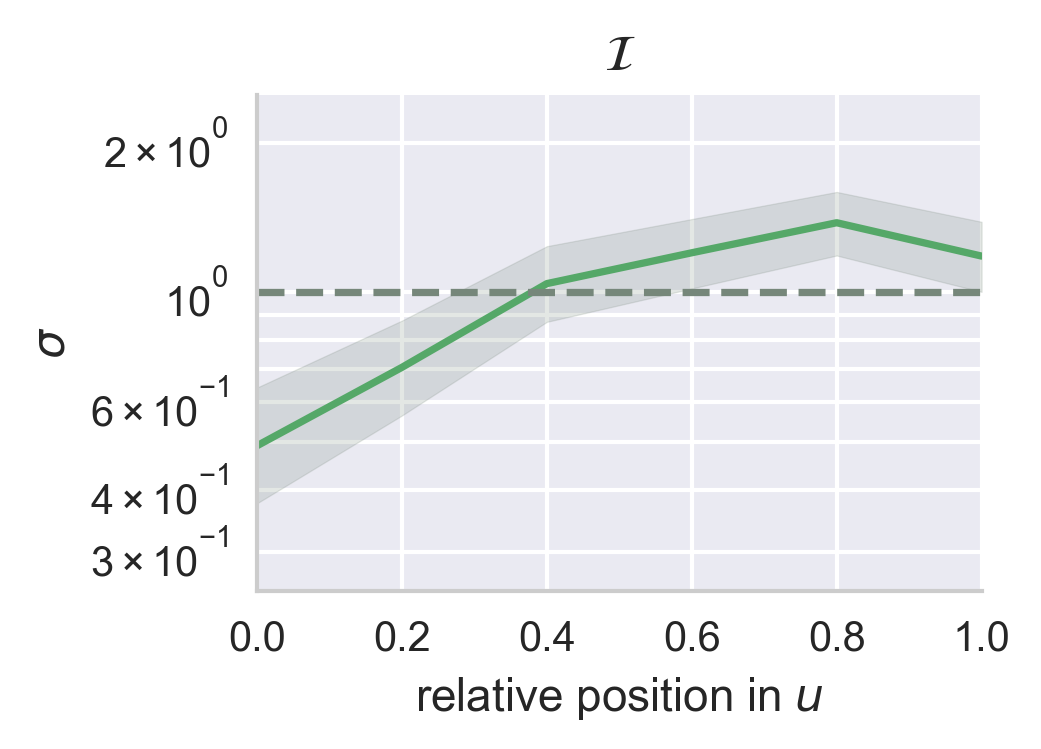
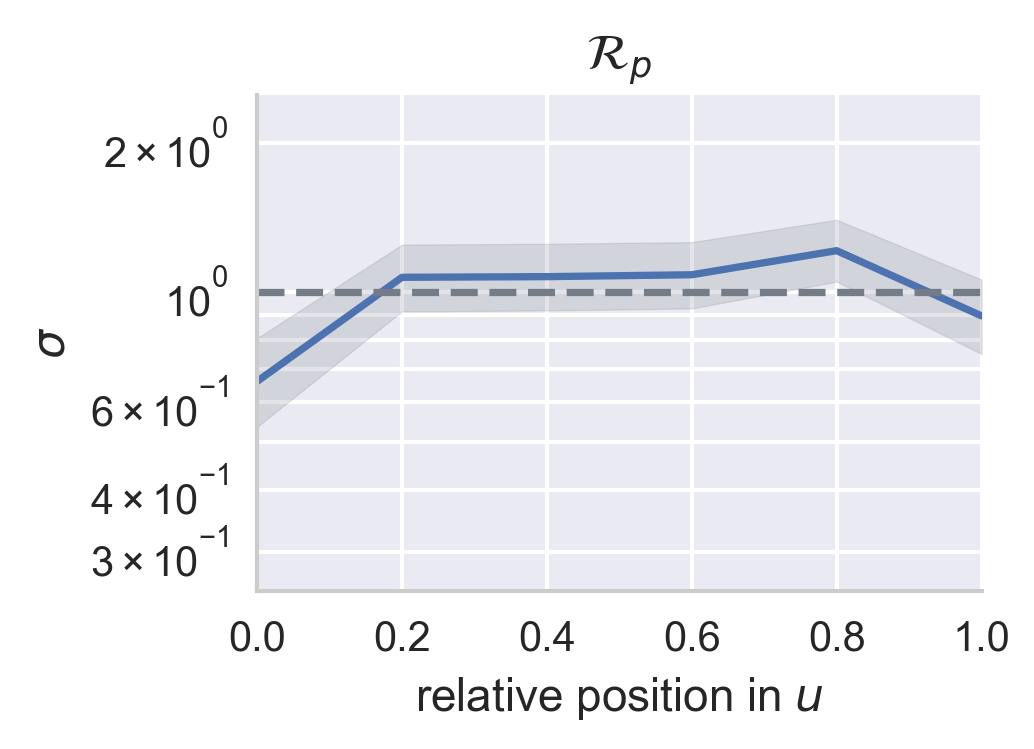
Number of operations vs. utterance length
Susceptibility vs. position in utterance
Deletions tend to gate other operations
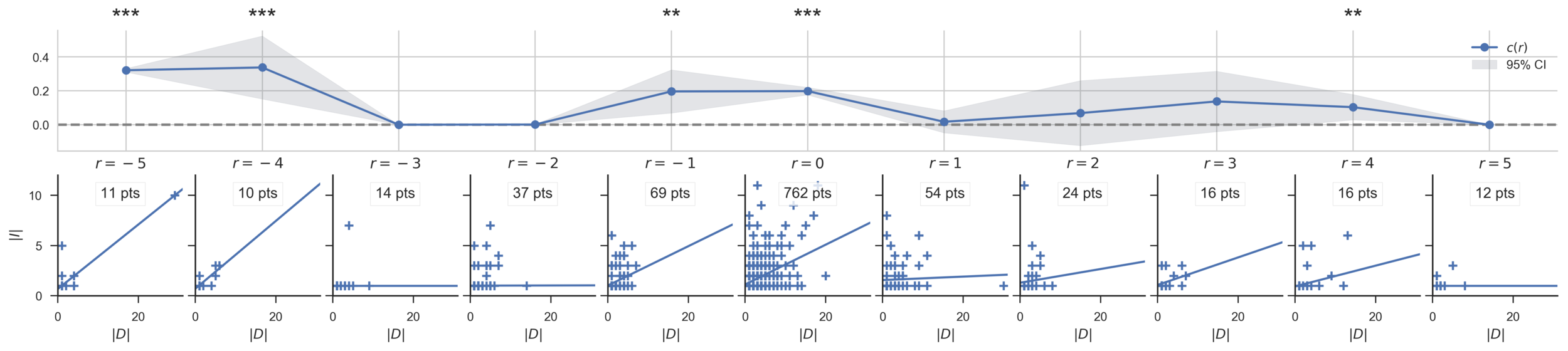
Insertions relate to preceding deletions
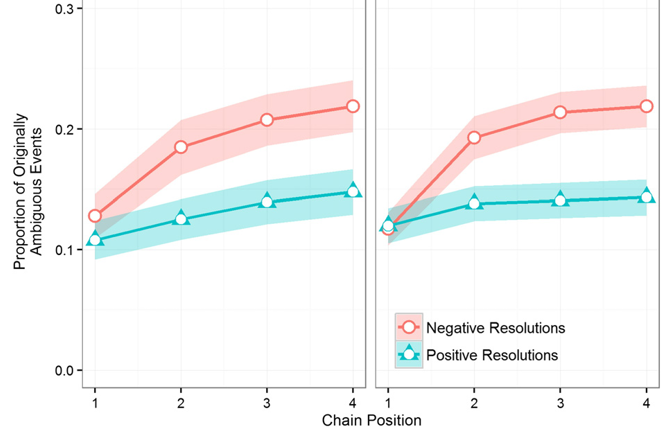
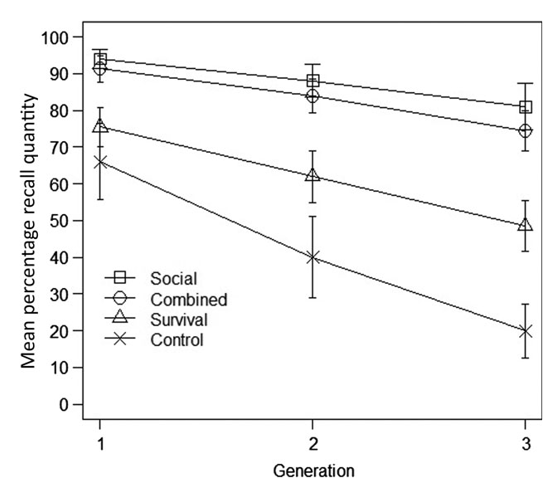
Stubbersfield et al. (2015)
Bebbington et al. (2017)
Links the low-level with contrasted outcomes
Substitutions in online quotations
Complete transformations in chains
IN VIVO
IN VITRO
Recover susceptibility and variation results
Extend to insertions and deletions
Measure lexical evolution in the long term
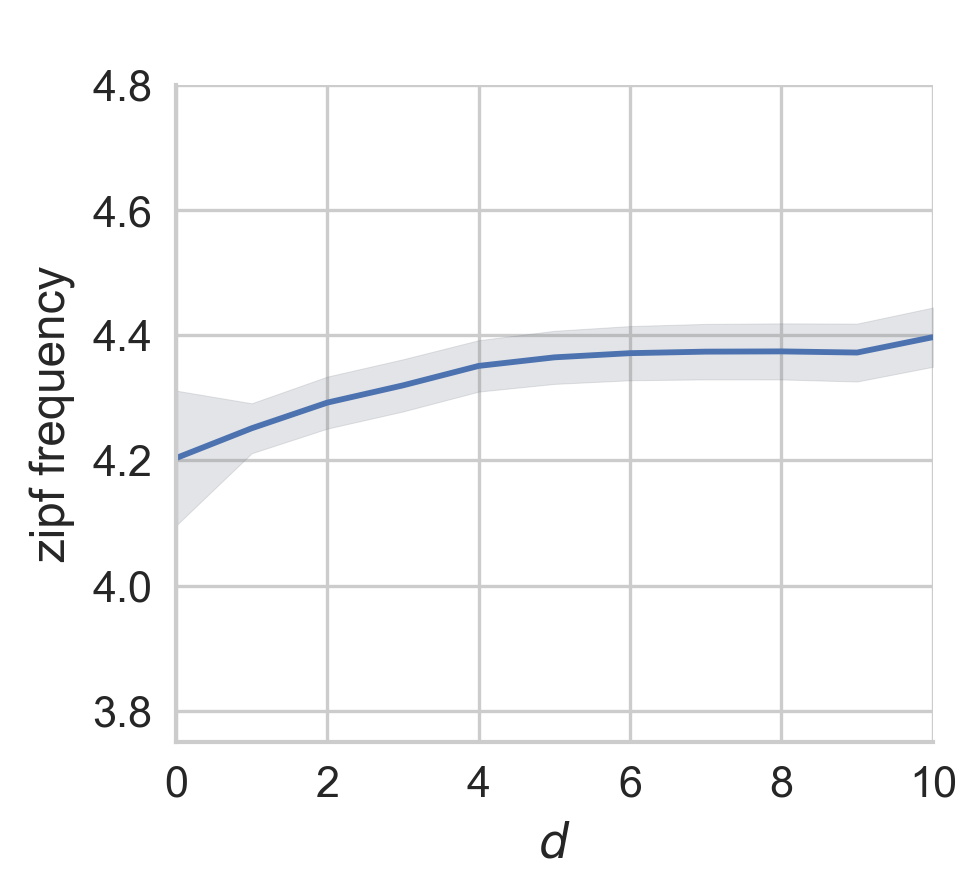
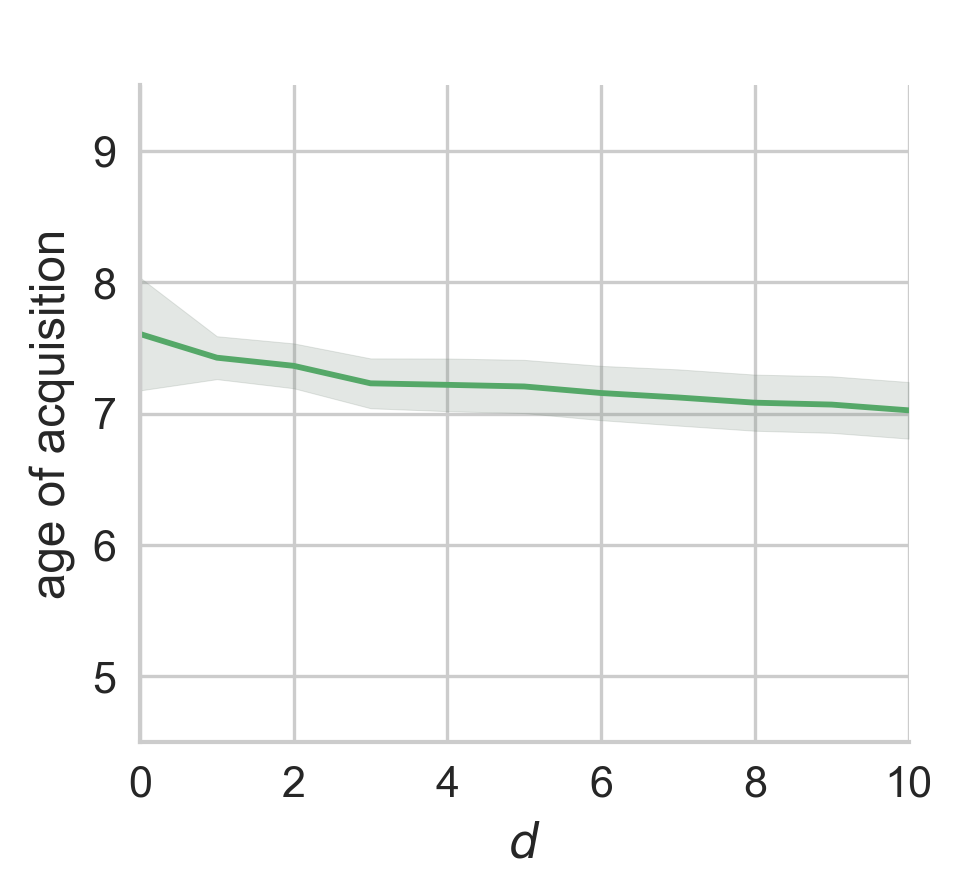
Recover online quotations
Realistic content
Control over data-generation
Computational analysis
Already coded
Do-it-by-hand
Simple setting
Empirical (un)bind
Quantitative analysis of complex meaning change
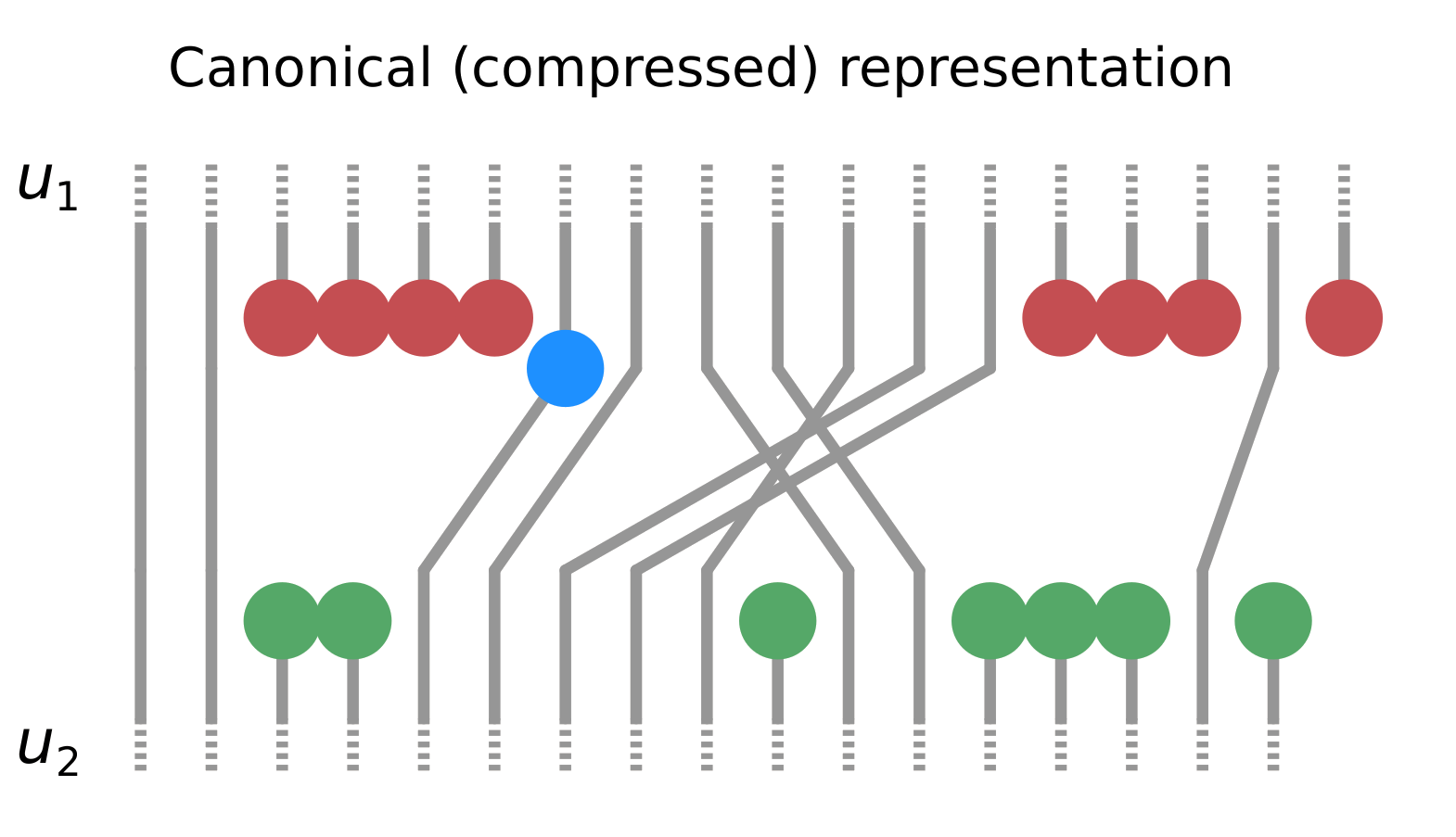
Structural changes from exchanges
Relating insertion and deletion chunks
Inner structure of transformations
Sequence alignments of semantic parses
Further work
In vivo applications to more complete data sets (social networks)
Sentence processing ↔ Higher level evolution
Feedback loops: utterance distribution ↔ detailed transformations
Long-lived chains with recurring changes
Semantic parsing and NLP methods on the inner structure
Connect to the constitution of meaning in interaction and context
Openings
Semantics
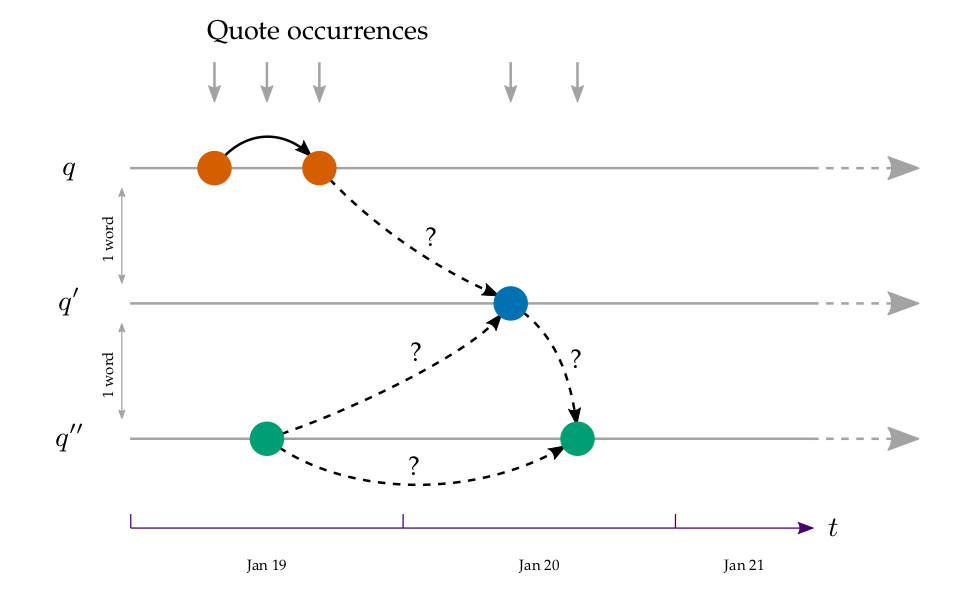
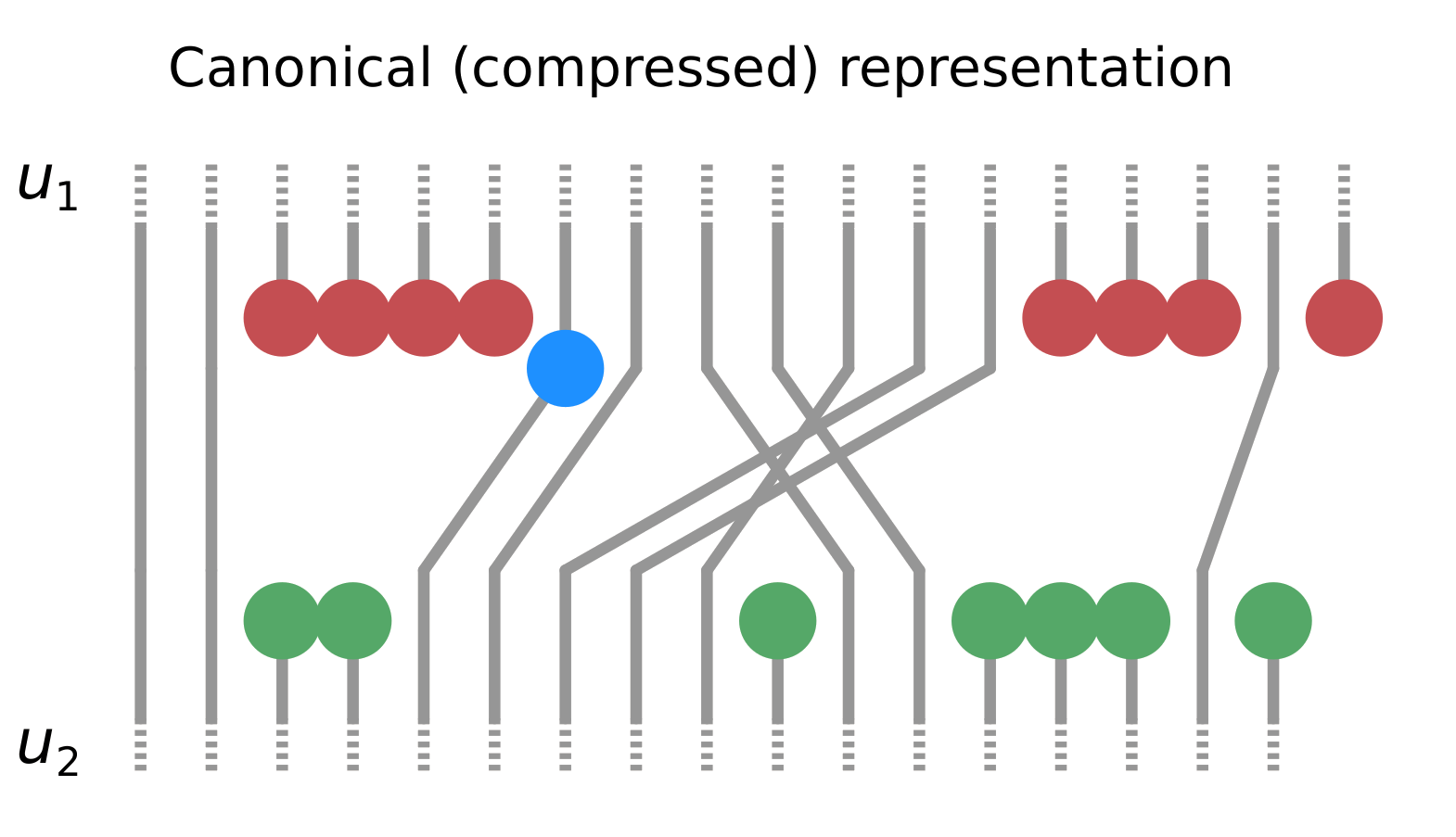
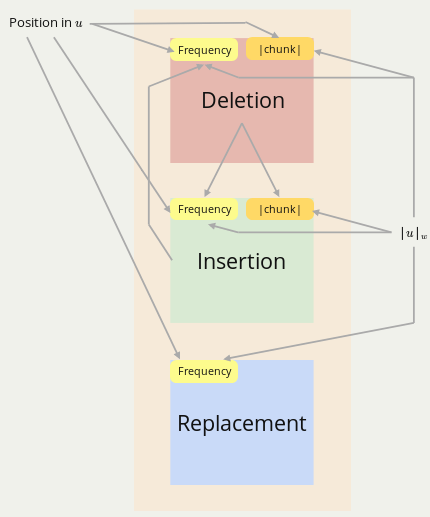
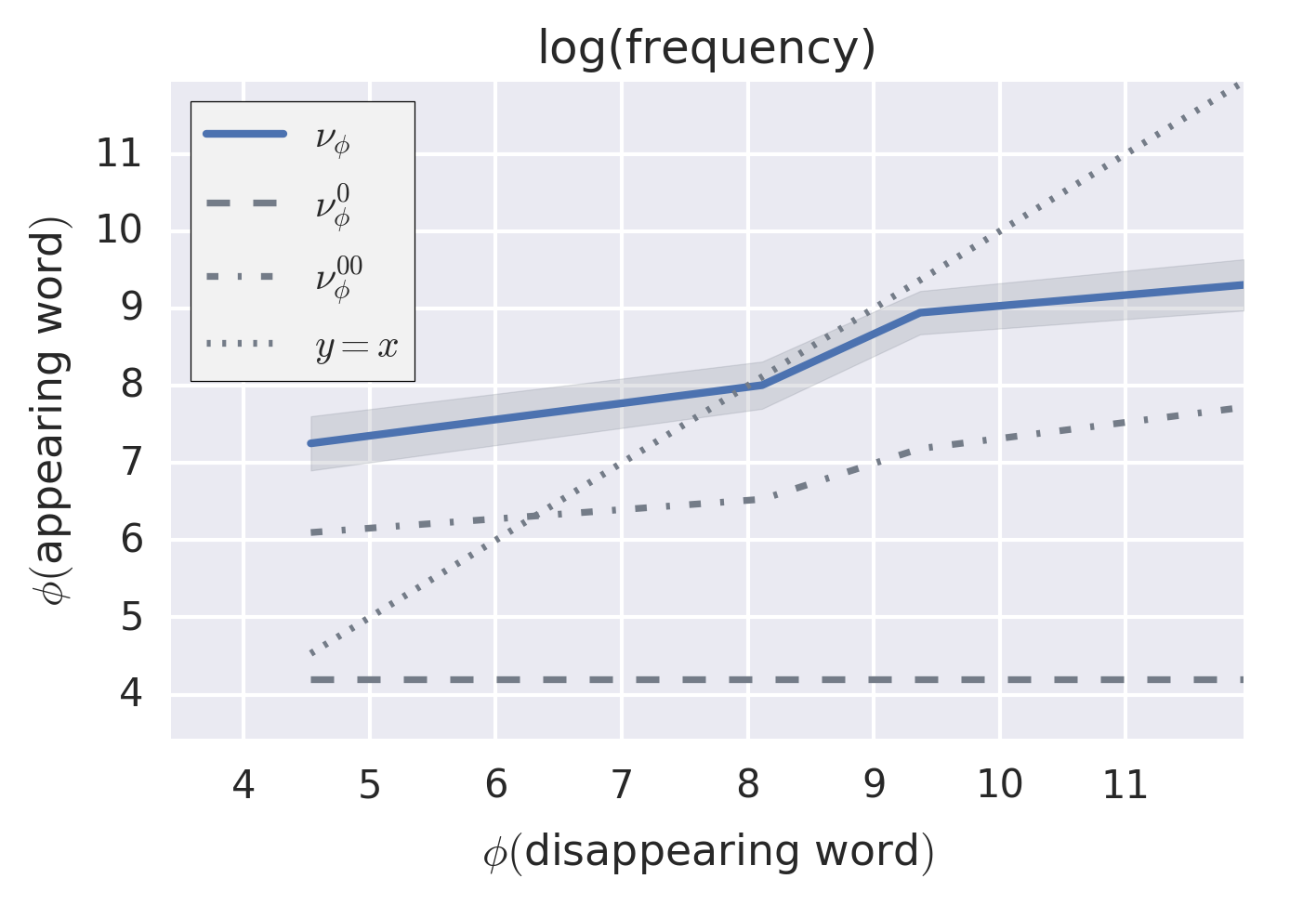
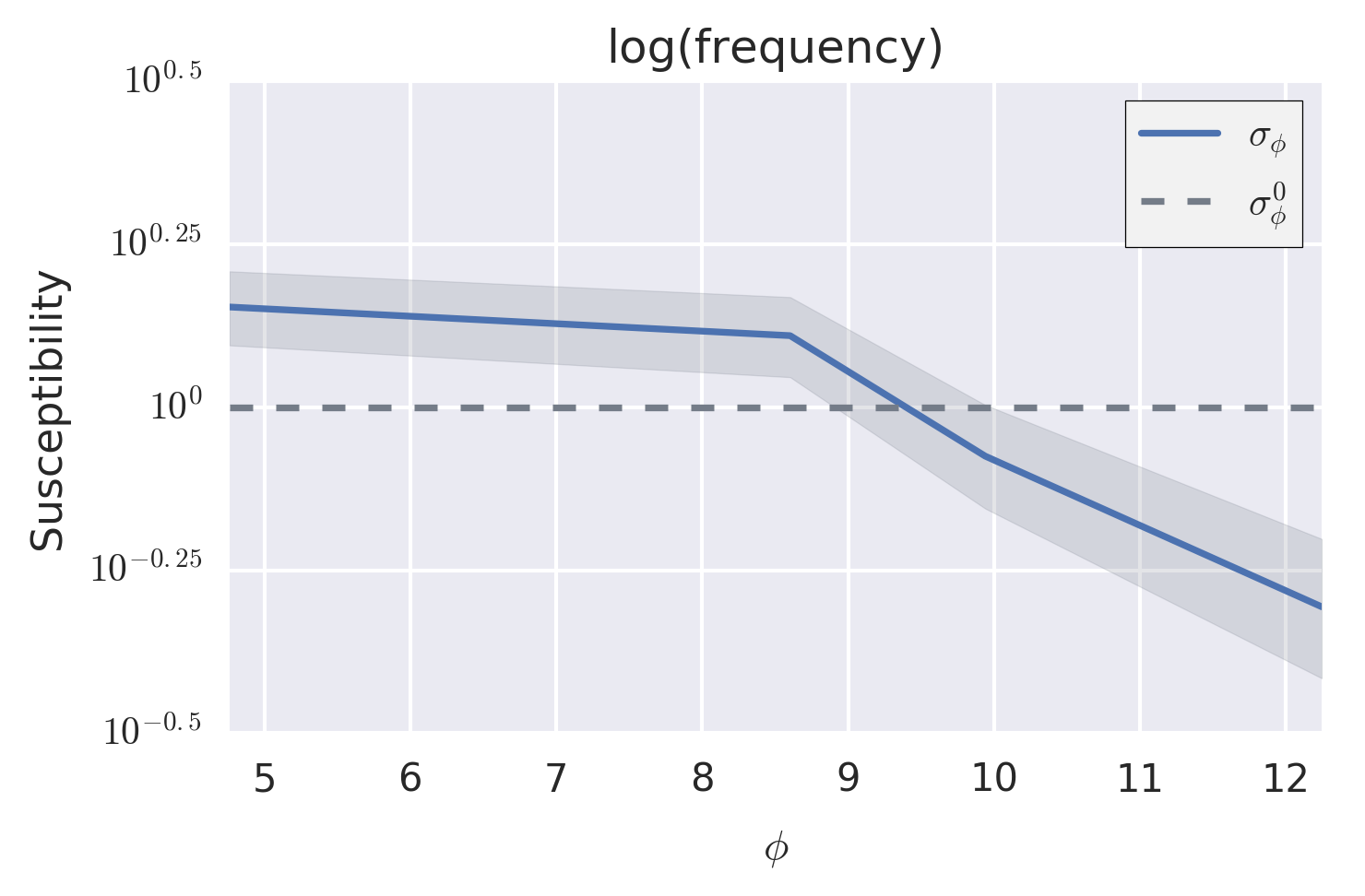
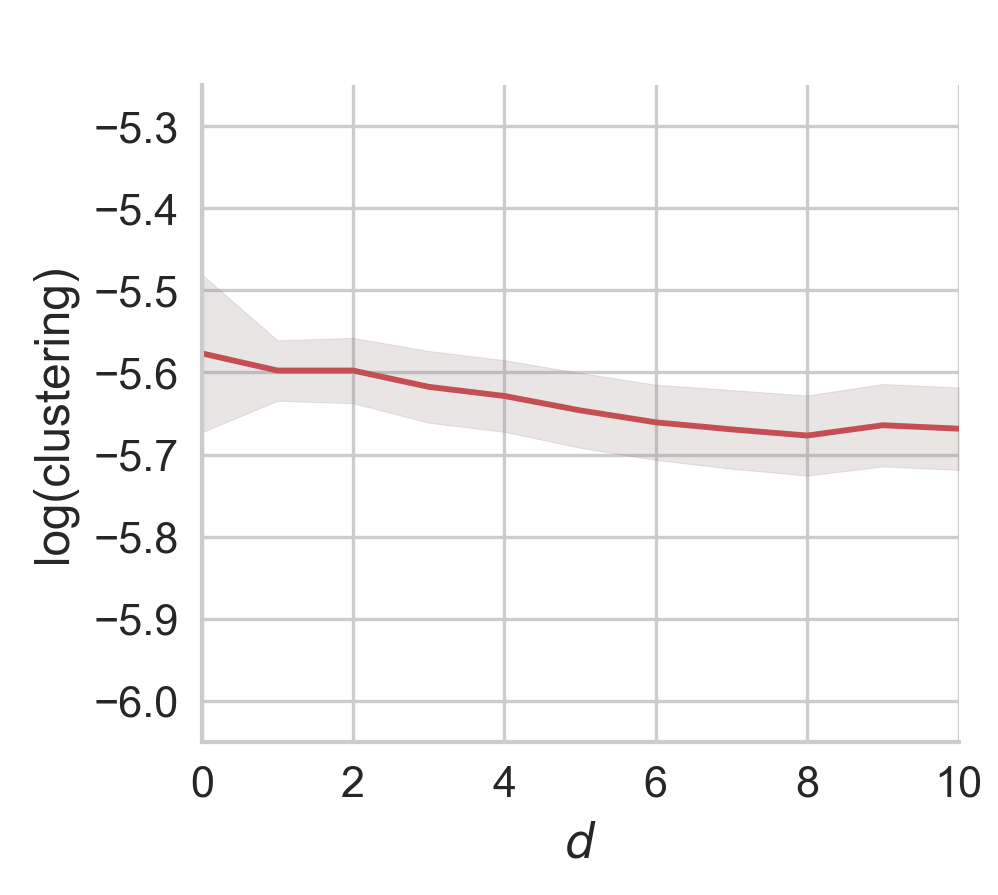
Thank you
Jury
Supervision
Support
Jean-Pierre Nadal & Camille Roth
Family & friends
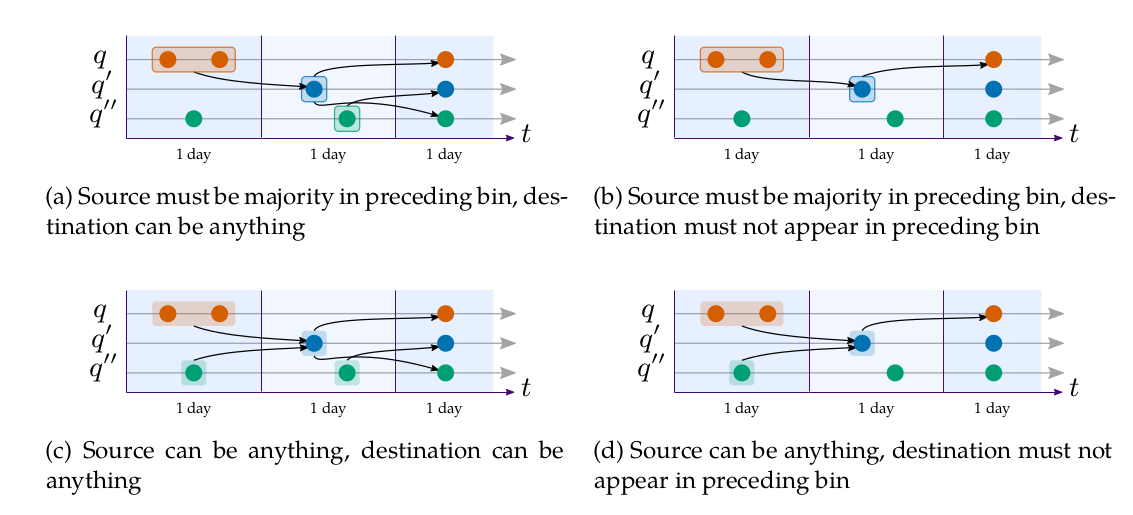
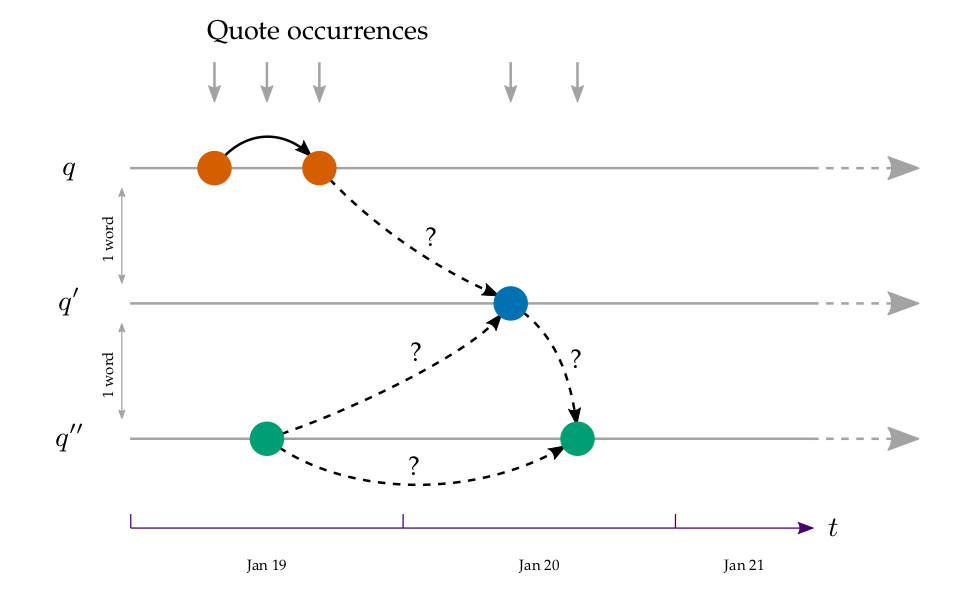
Substitution models
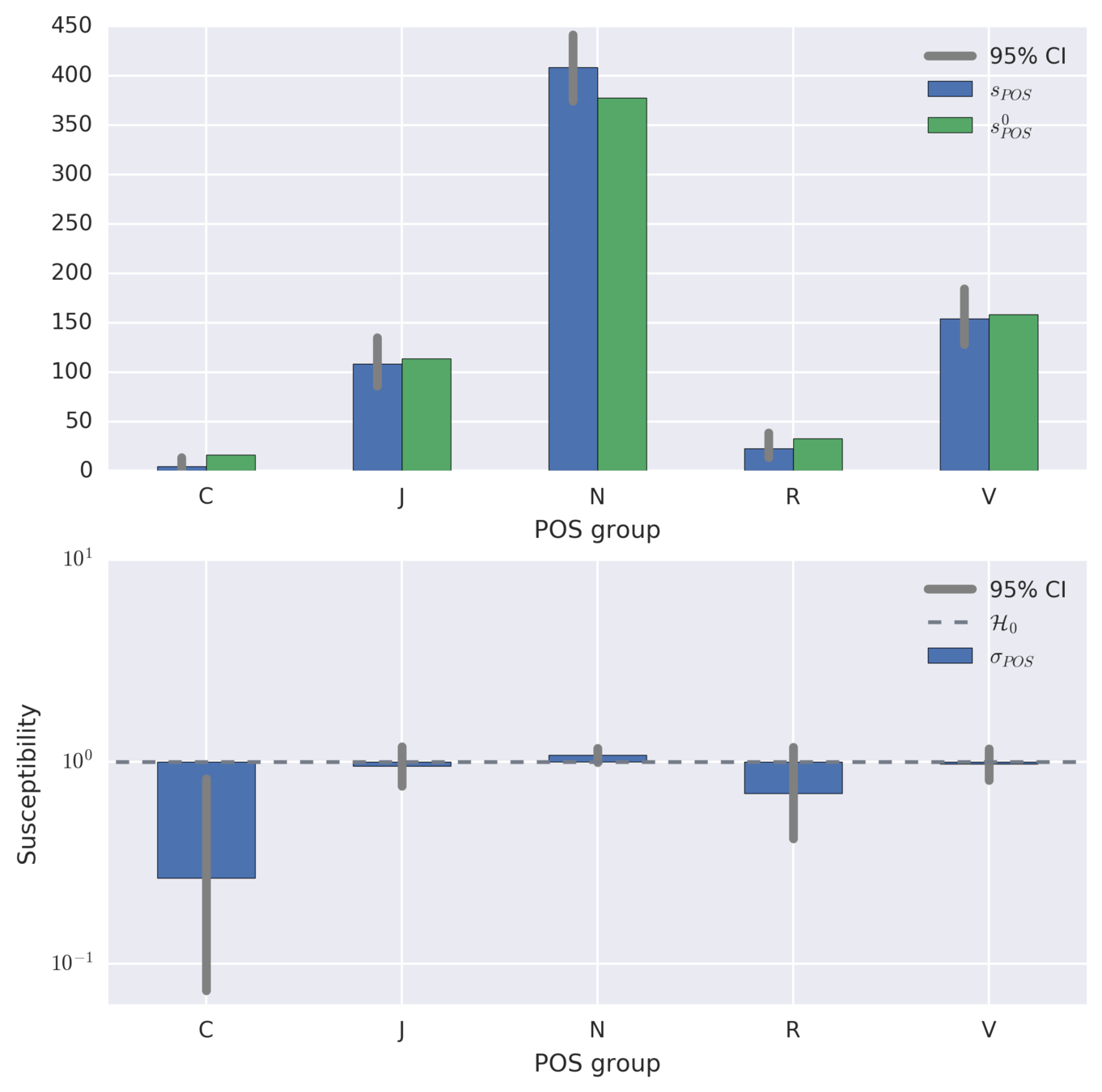
POS Susceptibility
Feature interactions
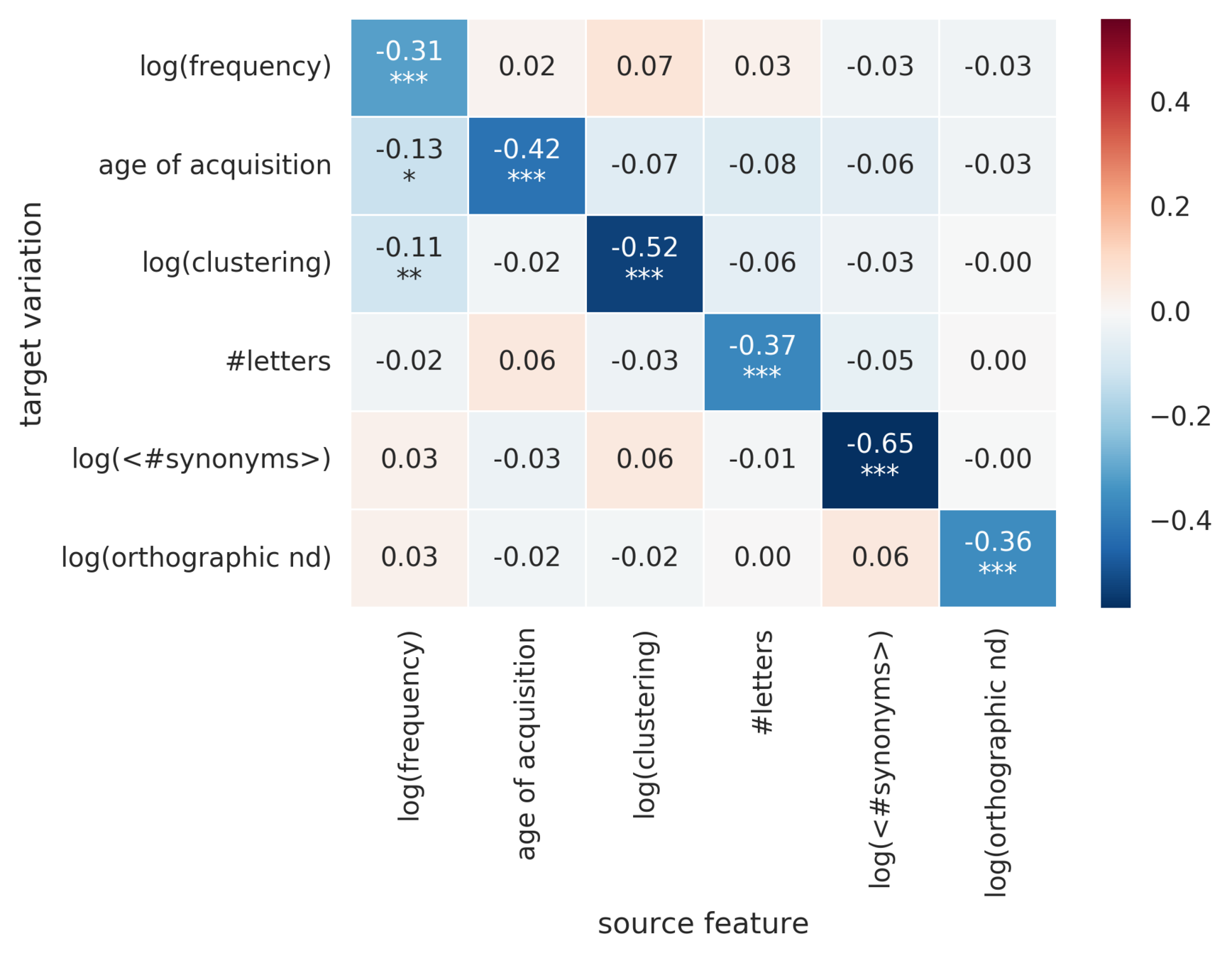
Burmese poet Saw Wai (Nov 2008):
– “Senior general Than Shwe is foolish with power”
⭢ “Senior general Than Shwe is crazy with power”
– "foolish": 8.94 y.o., 675 times, cc of .0082
⭢ "crazy": 5.22 y.o., 4100 times, cc of .0017
Live experiment
Data quality
#participants |
#root utterances |
tree size |
Duration |
Spam rate |
Usable reformulations |
53 | 49 | 2 x 70 |
54 | 50 | 25/batch |
48 | 49 | 70 |
64min | 43min | 37min/batch |
22.4% + 3.5% | 0.8% + 0.6% | 1% + 0.1% |
1980 | 2411 | 3506 |
Exp. 1 | Exp. 2 | Exp. 3 |
-
First large-scale launch
- Bugs and customer service
- Mistaken UI affordances
-
Extensive rewrite
- Automated tests
- Pilots evaluating the UI
- Pilots sampling root utterances
– “There is no hope for peace, it is a lost cause”
⭢ “There is no lose hope that rara ra to op”
– “My Government's overriding priority is to ensure the stability of the British economy”
⭢ “My governments overall liability is to sort out the... not sure.”
Example data
Immediately after I become president I will confront this economic challenge head-on by taking all necessary steps
immediately after I become a president I will confront this economic challenge
Immediately after I become president, I will tackle this economic challenge head-on by taking all the necessary steps
This crisis did not develop overnight and it will not be solved overnight
the crisis did not developed overnight, and it will be not solved overnight
original
This, crisis, did, not, develop, overnight, and, it, will, not, be, solved, overnight
this, crisis, did, not, develop, overnight, and, it, will, not, be, solved, overnight
this, crisis, did, not, develop, overnight, and, it, will, not, be, solved, overnight
crisi, develop, overnight, solv, overnight
tokenize
lowercase & length > 2
stopwords
stem
The crisis didn't happen today won't be solved by midnight.
crisi, happen, today, solv, midnight
d = 0,6
Utterance-to-utterance distance
Aggregate trends
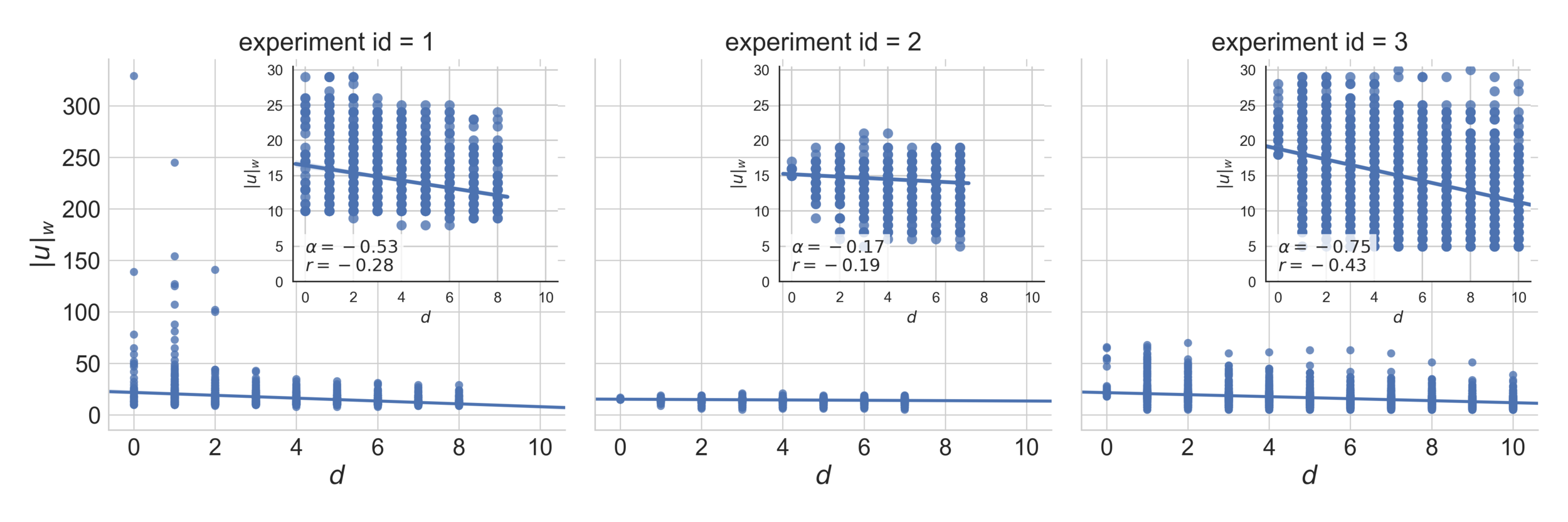
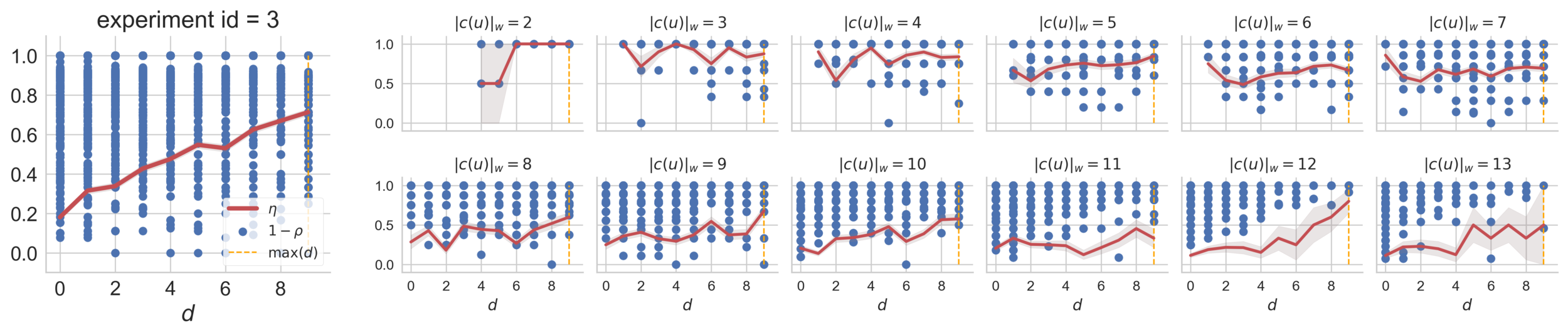
Size reduction
Transmissibility
Variability
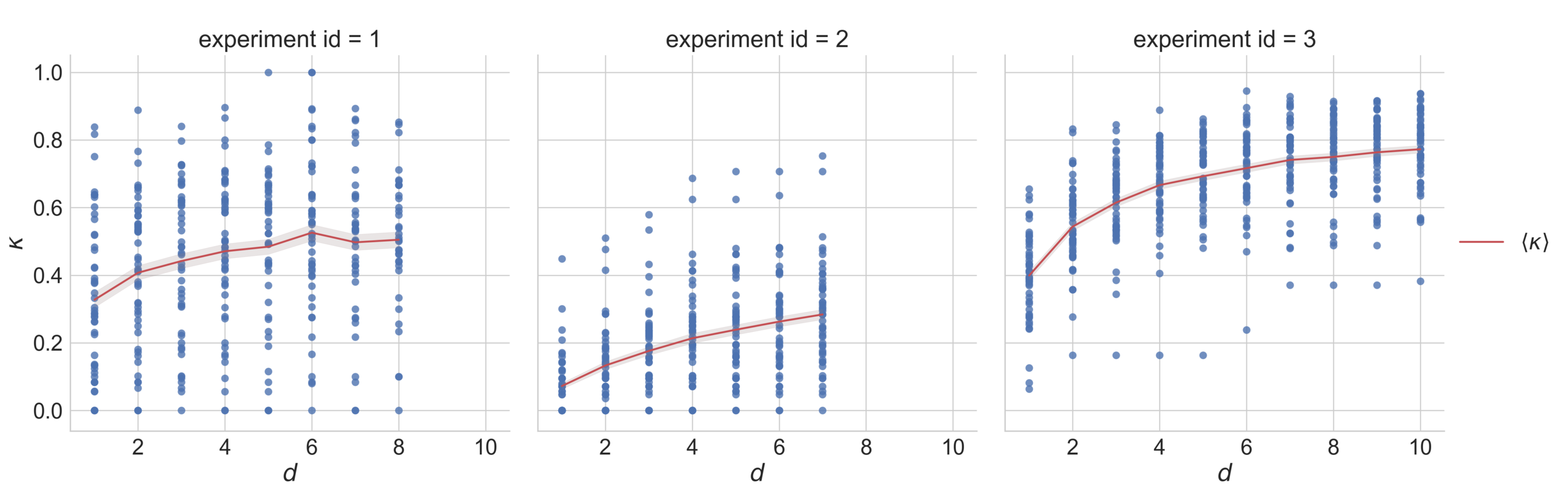
Sequence alignments
Needleman and Wunsch (1970)
AGAACT-
| ||
-G-AC-G
AGAACT
GACG
Finding her son, Alvin, 69, hanged, Mrs Hunt, of Brighton, was so depressed she could not cut him down
Finding her son Arthur 69 hanged Mrs Brown from Brighton was so upset she could not cut him down
Finding her son Alvin 69 hanged Mrs Hunt of - - Brighton, was so depressed she could not cut him down
Finding her son Arthur 69 hanged Mrs - - Brown from Brighton was so upset she could not cut him down
Gap open cost →θopen
Gap extend cost →θextend
Item match-mismatch
Applied to utterances
At Dover, the finale of the bailiffs convention, their duty said a speaker are delicate, dangerous and detailed
At Dover, at a Bailiffs convention. a speaker said that their duty was to patience, and determination
At Dover the finale of the - - bailiffs convention - - - - their duty
At Dover - - - - at a Bailiffs convention a speaker said that their duty
said a speaker are delicate dangerous - - - and detailed -
- - - - - - was to patience and - determination
At Dover the finale of the - - bailiffs convention |-Exchange-1------| their duty
At Dover - - - - at a Bailiffs convention a speaker said that their duty
said a speaker are delicate dangerous - - - and detailed -
|-Exchange-1------------------------| was to patience and - determination
said a speaker are delicate dangerous |-E2----|
|E2| a speaker - - - said that
said -
said that
↪E1
↪E2
Deep alignments
Alignment optimisation
θopen
θextend
θmismatch
θexchange by hand
All transformations
Hand-coded training set size?
Train the θ∗ on hand-coded alignments
Simulate the training process: imagine we know the optimal θ
1. Sample θ0∈[−1,0]3 to generate artificial alignments for all transformations
2. From those, sample n training alignments
3. Brute-force θ^1,...,θ^m estimators of θ0
4. Evaluate the number of errors per transformation on the test set
Test set
10x
10x
⟹ 100-200 hand-coded alignments yield ≤ 1 error/transformation
Transformation model


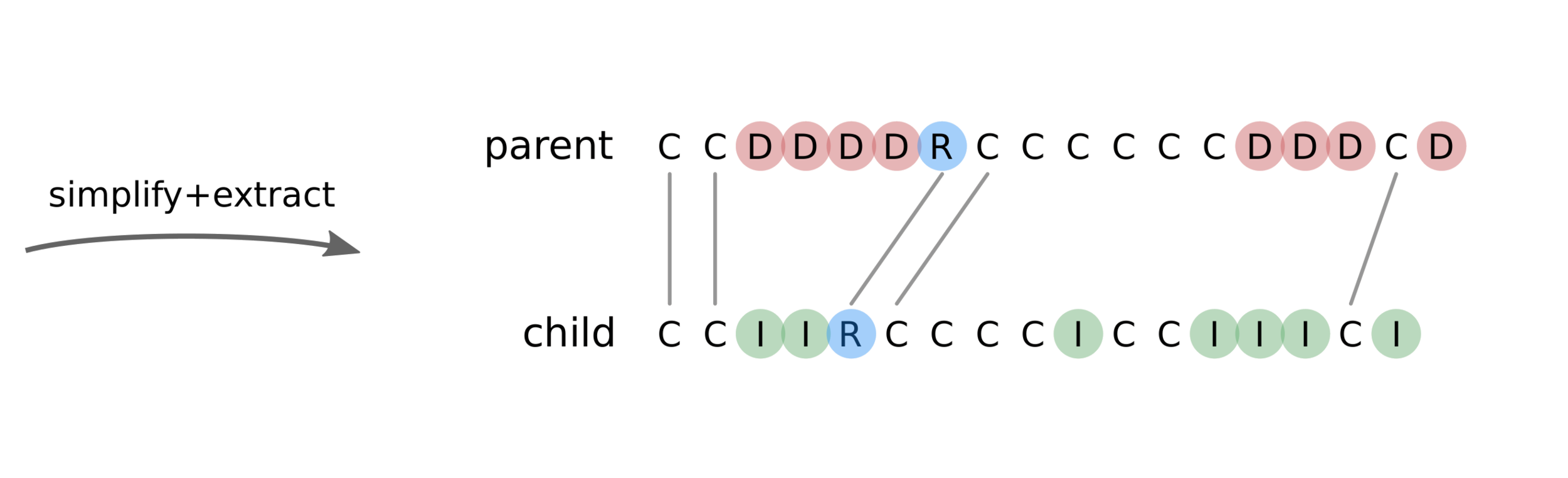
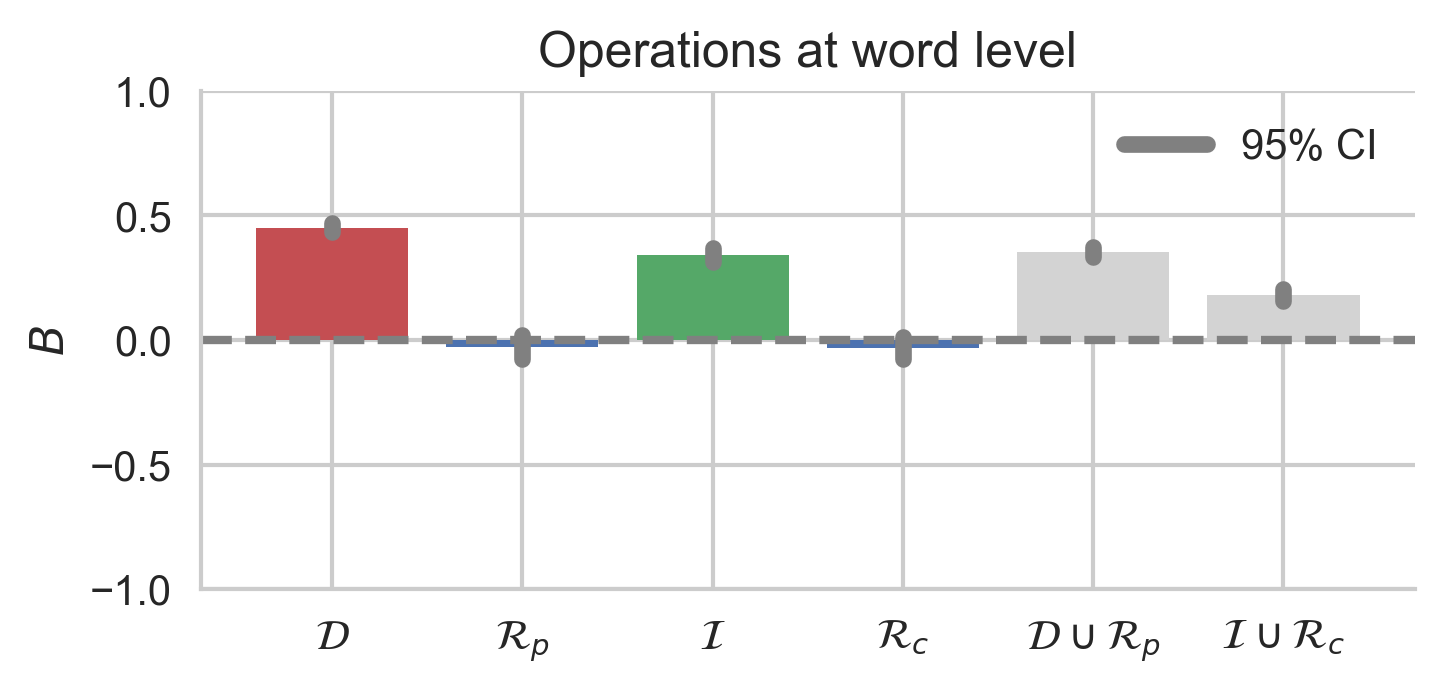
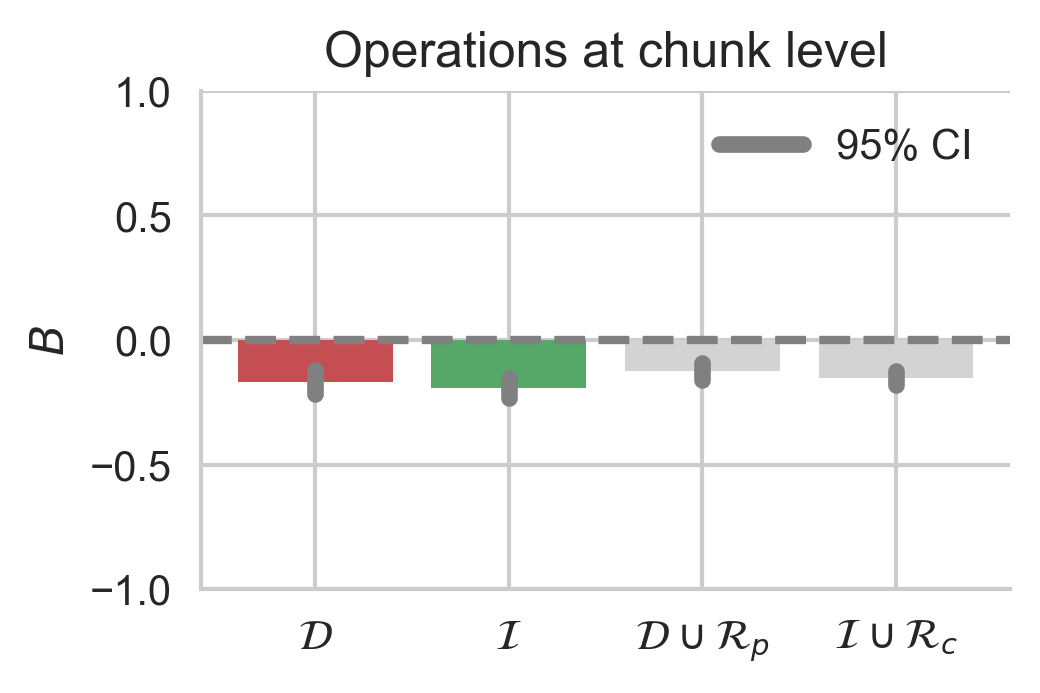
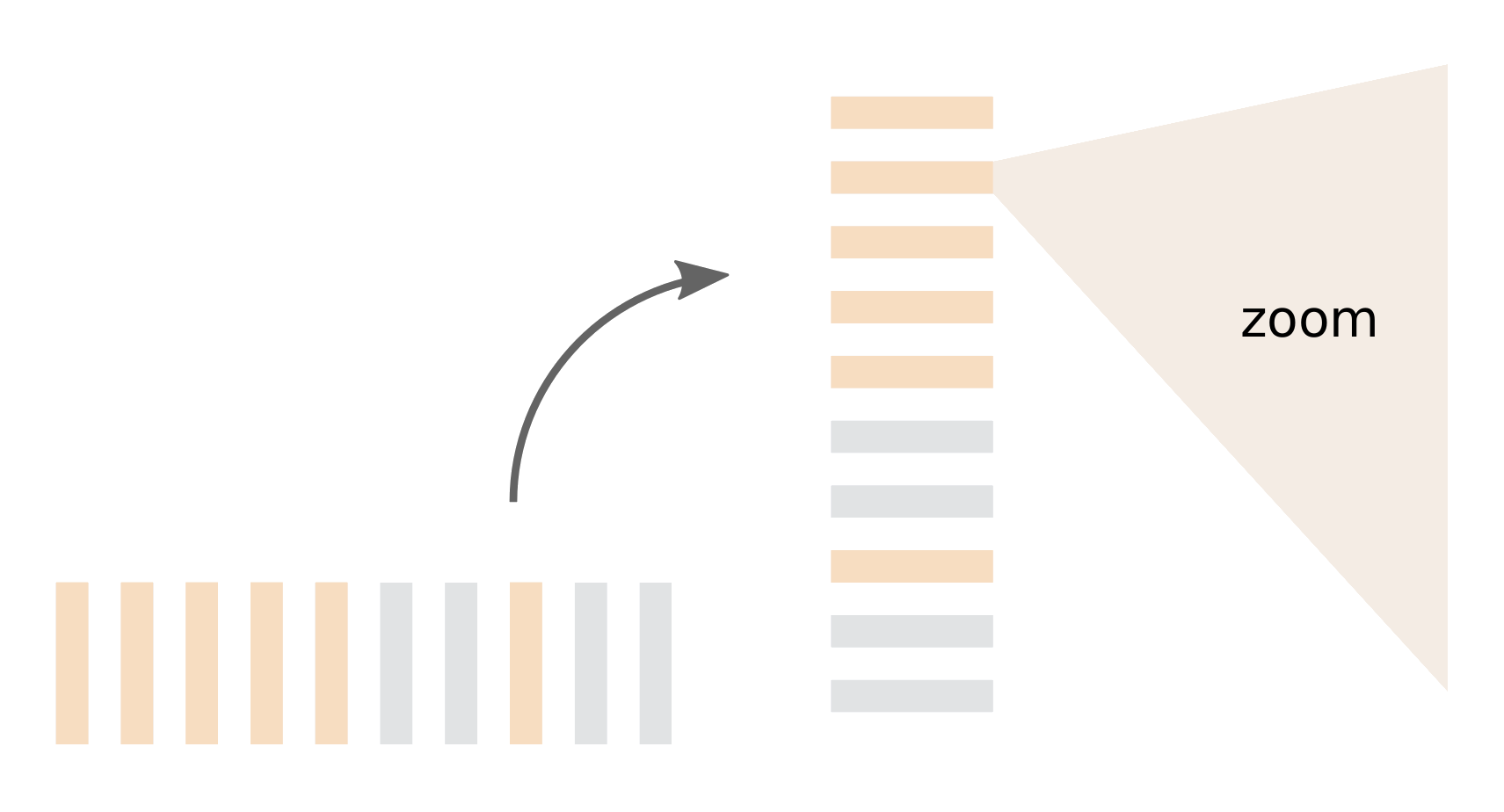
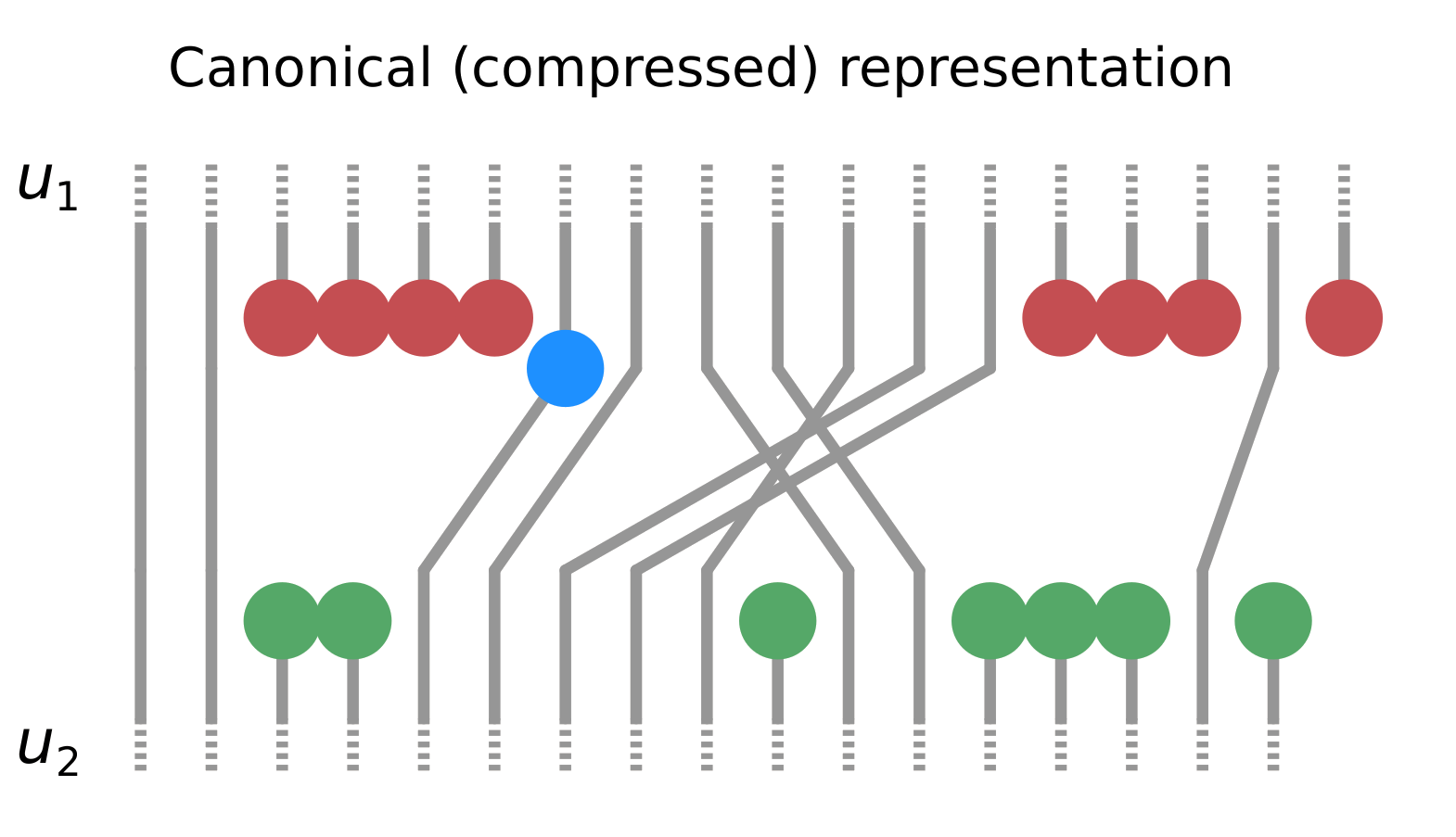
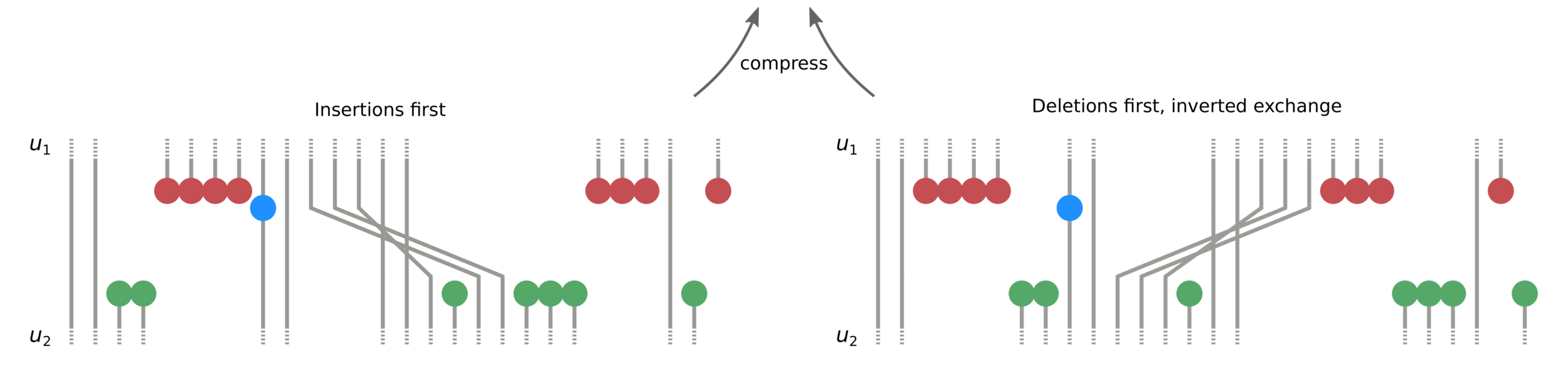
Lexical evolution (1)
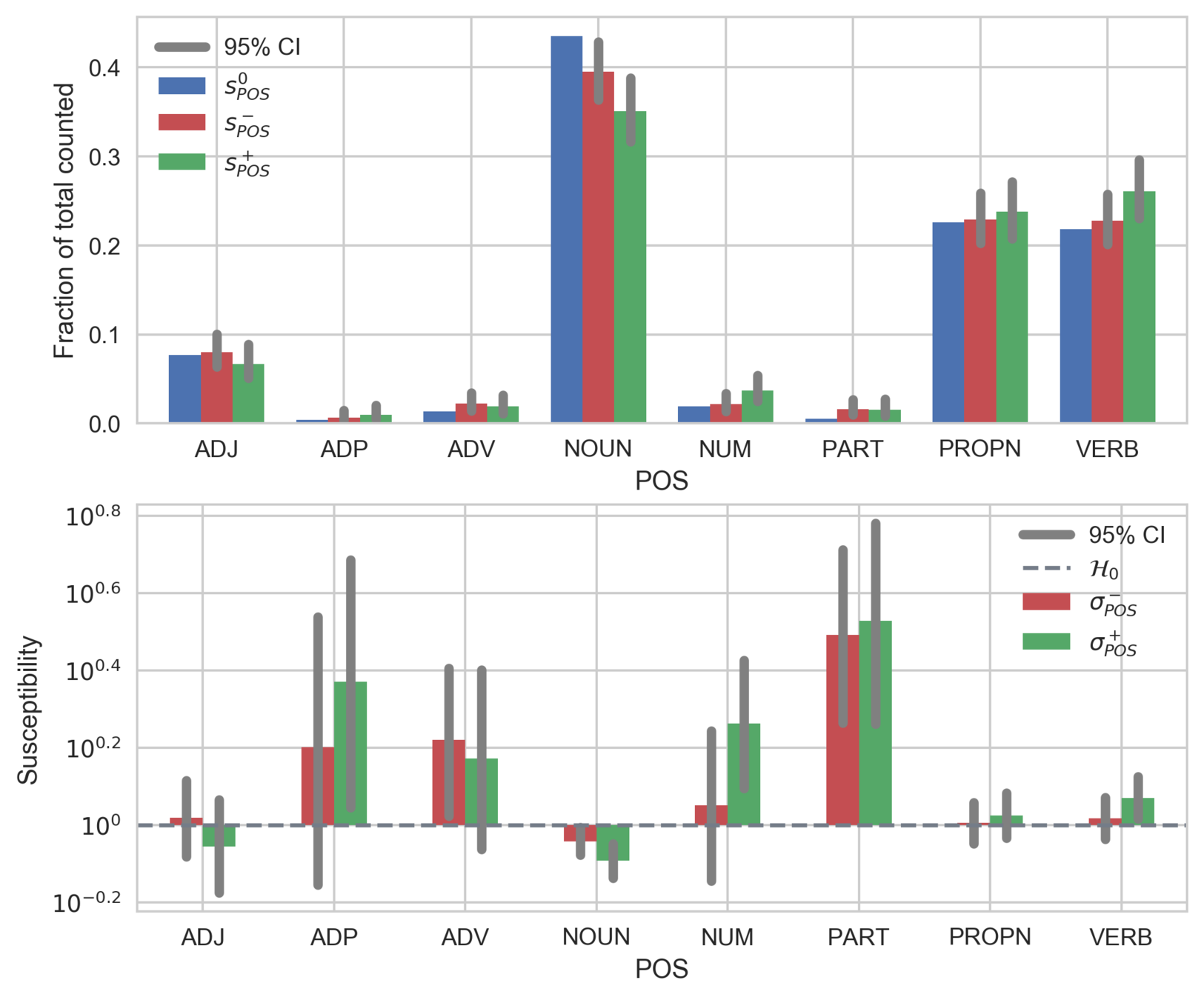



Step-wise
Susceptibility
Feature variation νϕ
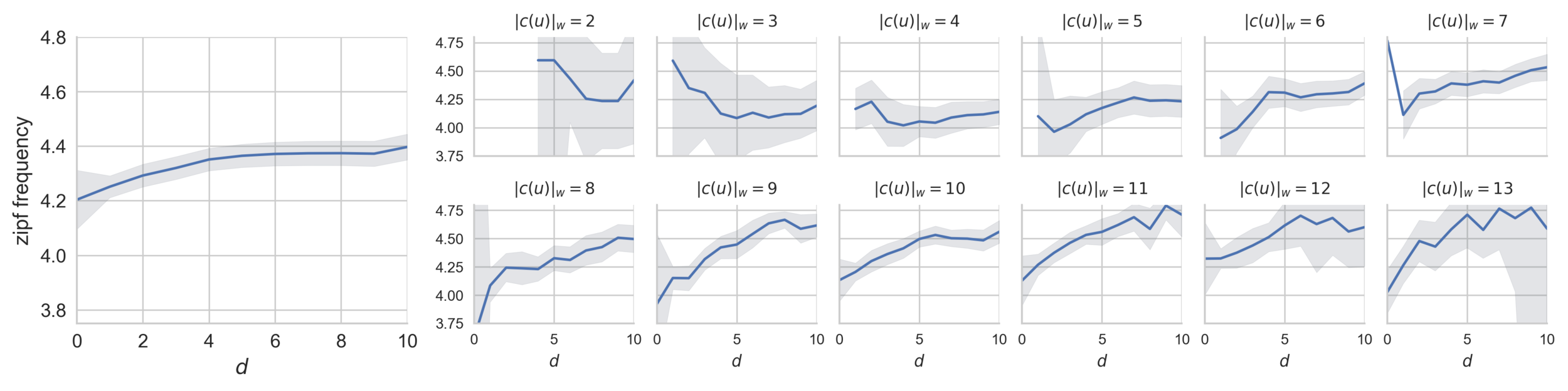
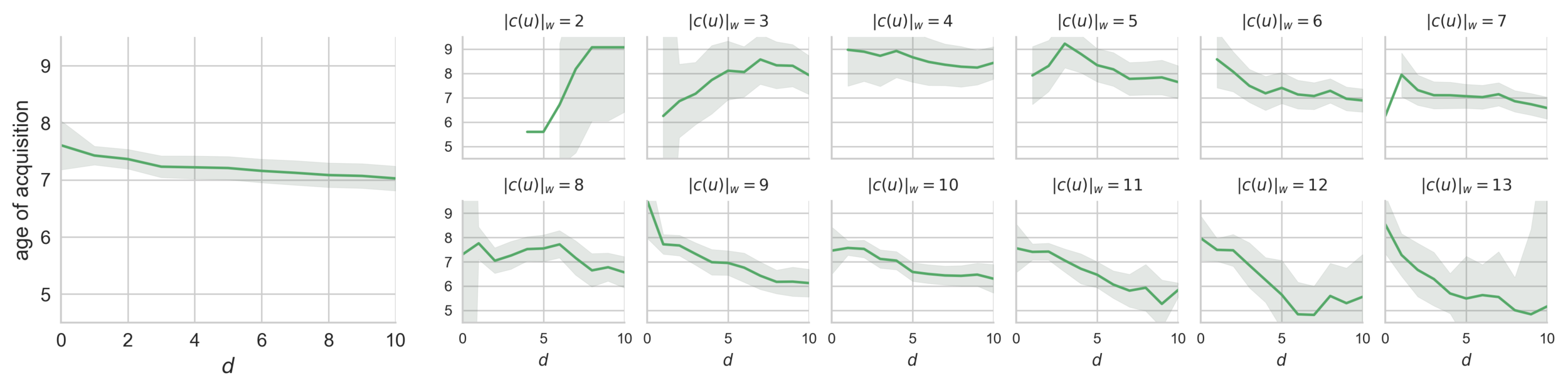
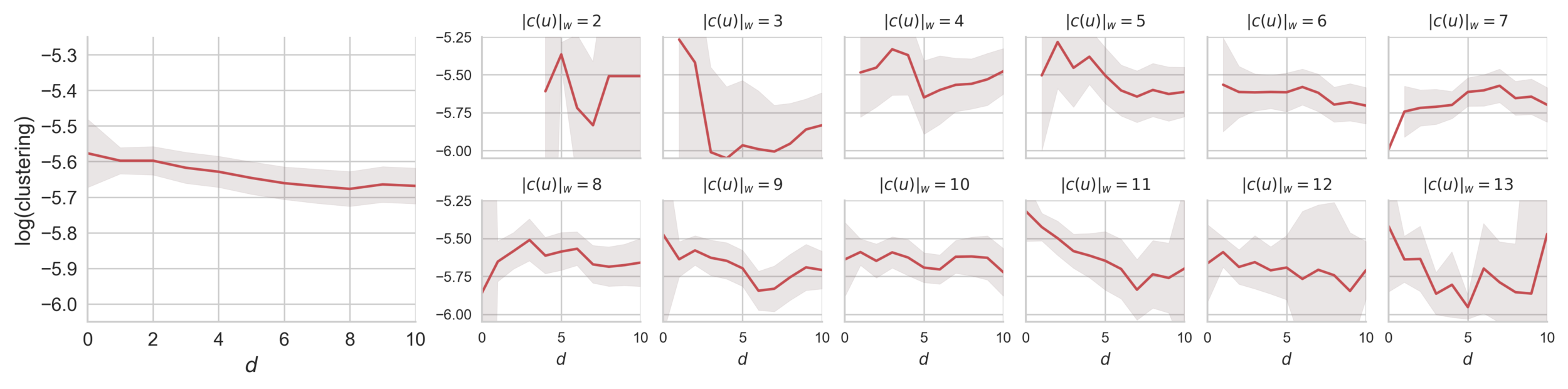
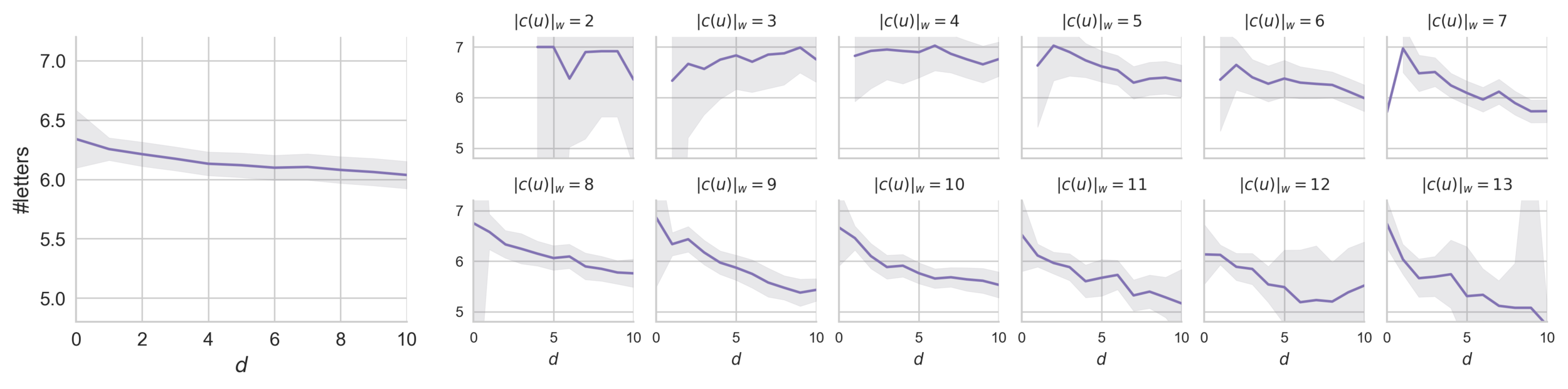
Lexical evolution (2)
Along the branches
Challenges with meaning
Can you think of anything else, Barbara, they might have told me about that party?
I've spoken to the other children who were there that day.
S
B
Abuser
The Devil's Advocate (1997)
?

Strong pragmatics (Scott-Phillips, 2017)
Access to context
Theory of the constitution of meaning
Challenges
PhD thesis defence
By Sébastien Lerique
PhD thesis defence
- 1,238