Modelling the spread of Covid-19 in the UK
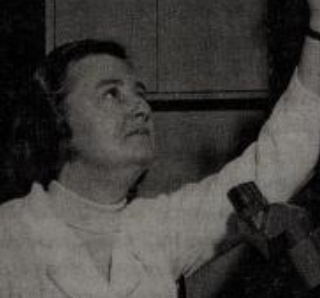
June Dalziel Almeida
JUNE
Joseph Bullock, Carolina Cuesta-Lazaro, Arnau Quera-Bofarull, Miguel Icaza-Lizaola, Aidan Sedgewick, Henry Truong, Aoife Curran, Edward Elliott, Richard Hayes, James Nightingale, Tristan Caulfield, Kevin Fong, Ian Vernon, Julian Williams, Richard Bower and Frank Krauss
Outline
- Motivation for new Covid-19 model
- Main model components:
- Virtual population
- What can residents do in JUNE?
- Modelling and mitigating infection
- Tuning the model
- How does JUNE differ from other models?
- Preliminary results
The questions JUNE would like to answer
- Understanding the geographical spread of the disease
- What measures need to be taken to end national lockdown situations safely, and how effective are local/regional lockdowns?
- What are the most effective measures to be taken to not overwhelm NHS capacity?
We need an accurate model of the UK's geography and demography to answer these questions!
Virtual population: a "digital twin"
- Use 2011 Census information
- What defines a JUNE "resident"?
- age (27)
- sex (f)
- ethnic group (Caribbean)
- deprivation index 2 (1-10)
- household composition
- work sector / subsector
(healthcare/doctor) - mode of transport (public)
- area of residence
- area of work
(From Nomis census service)
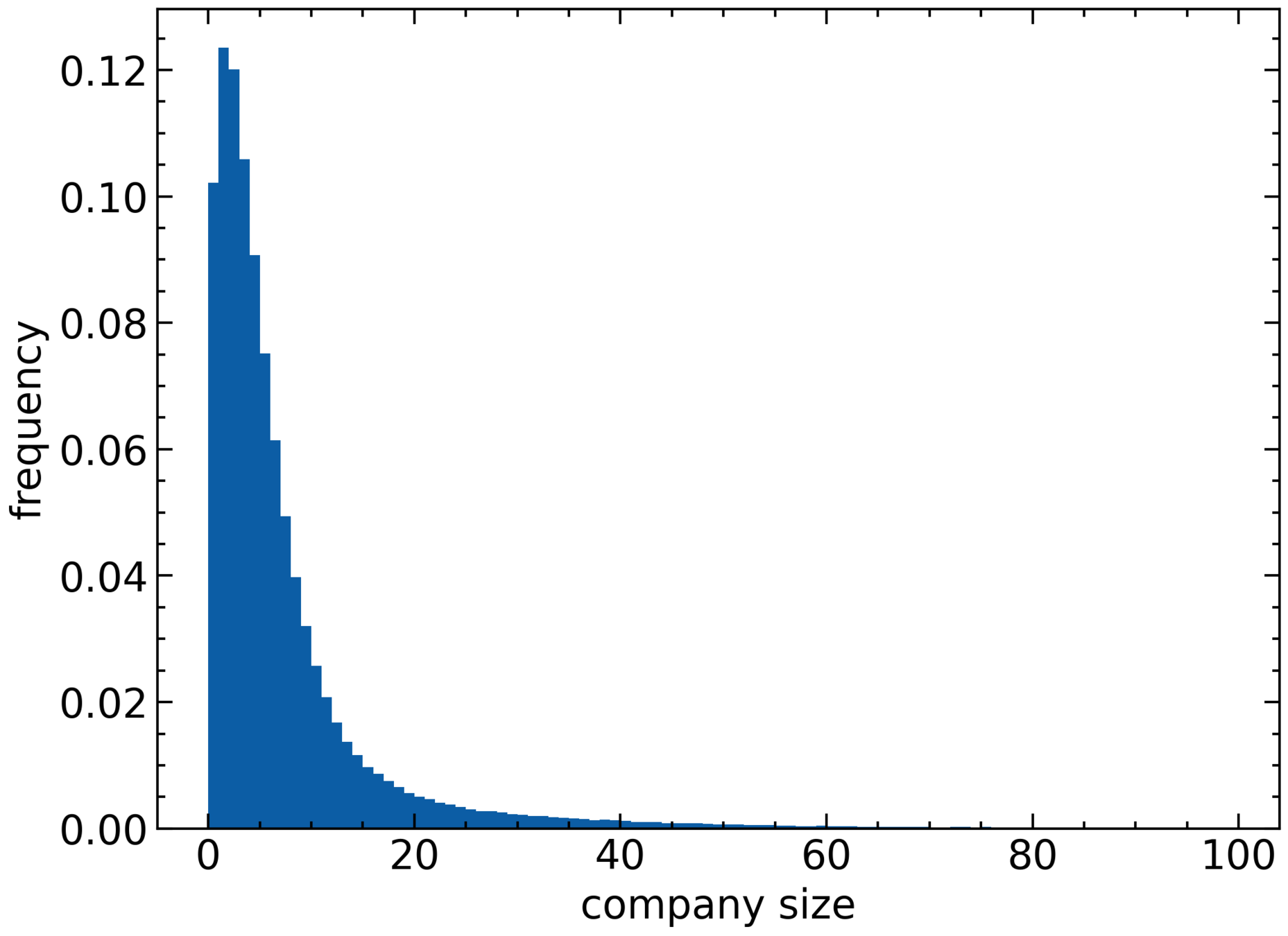
London
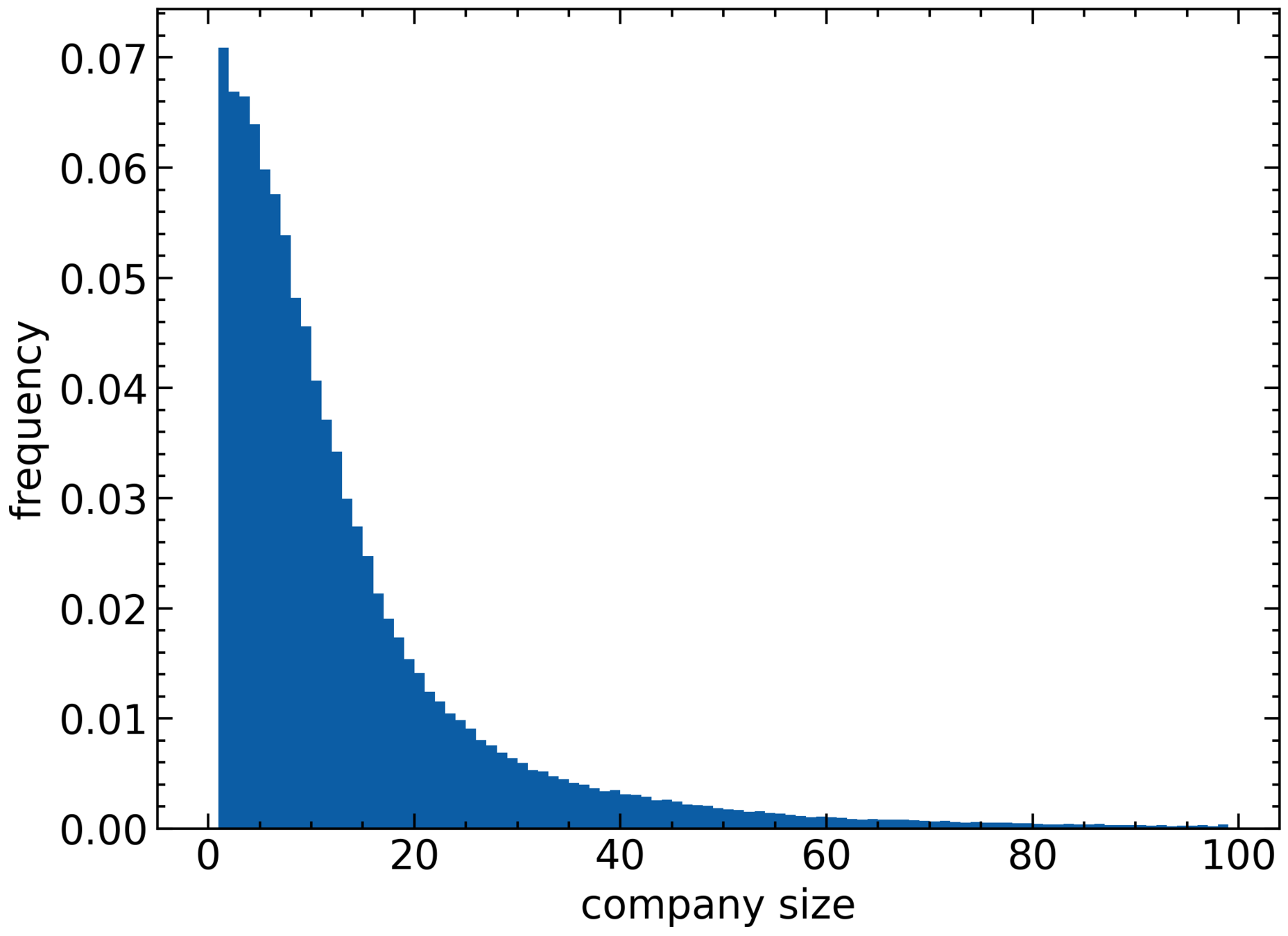
North East
June's Demography
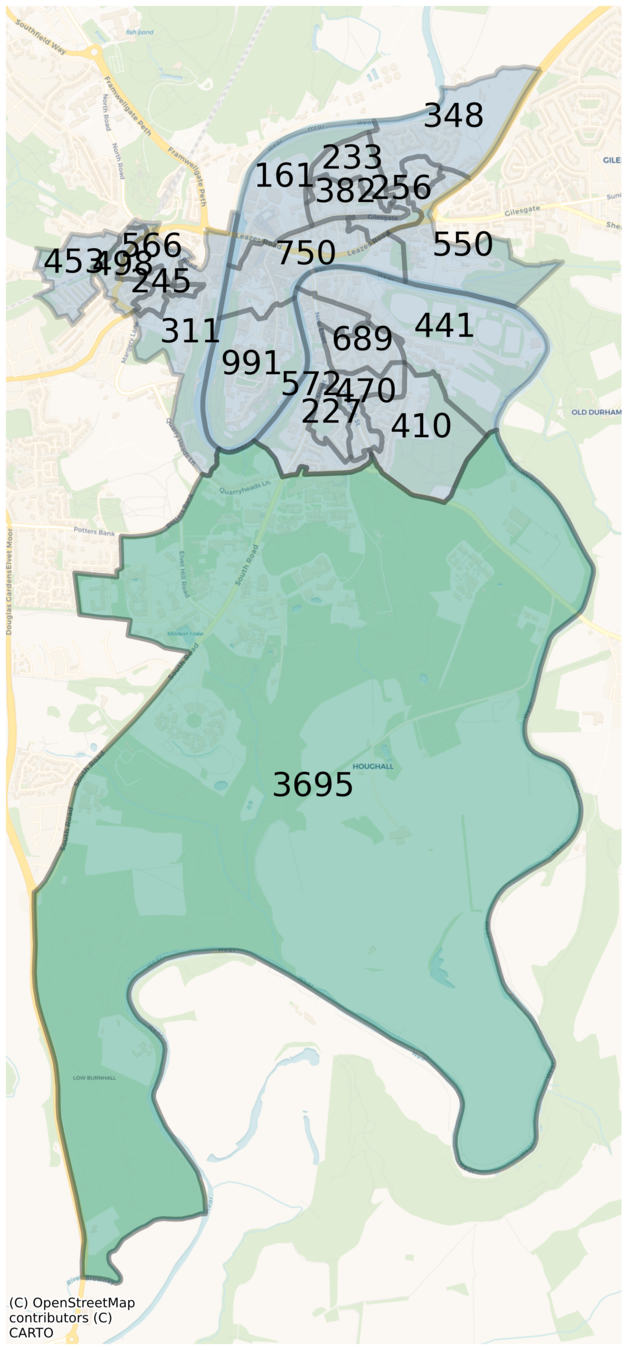
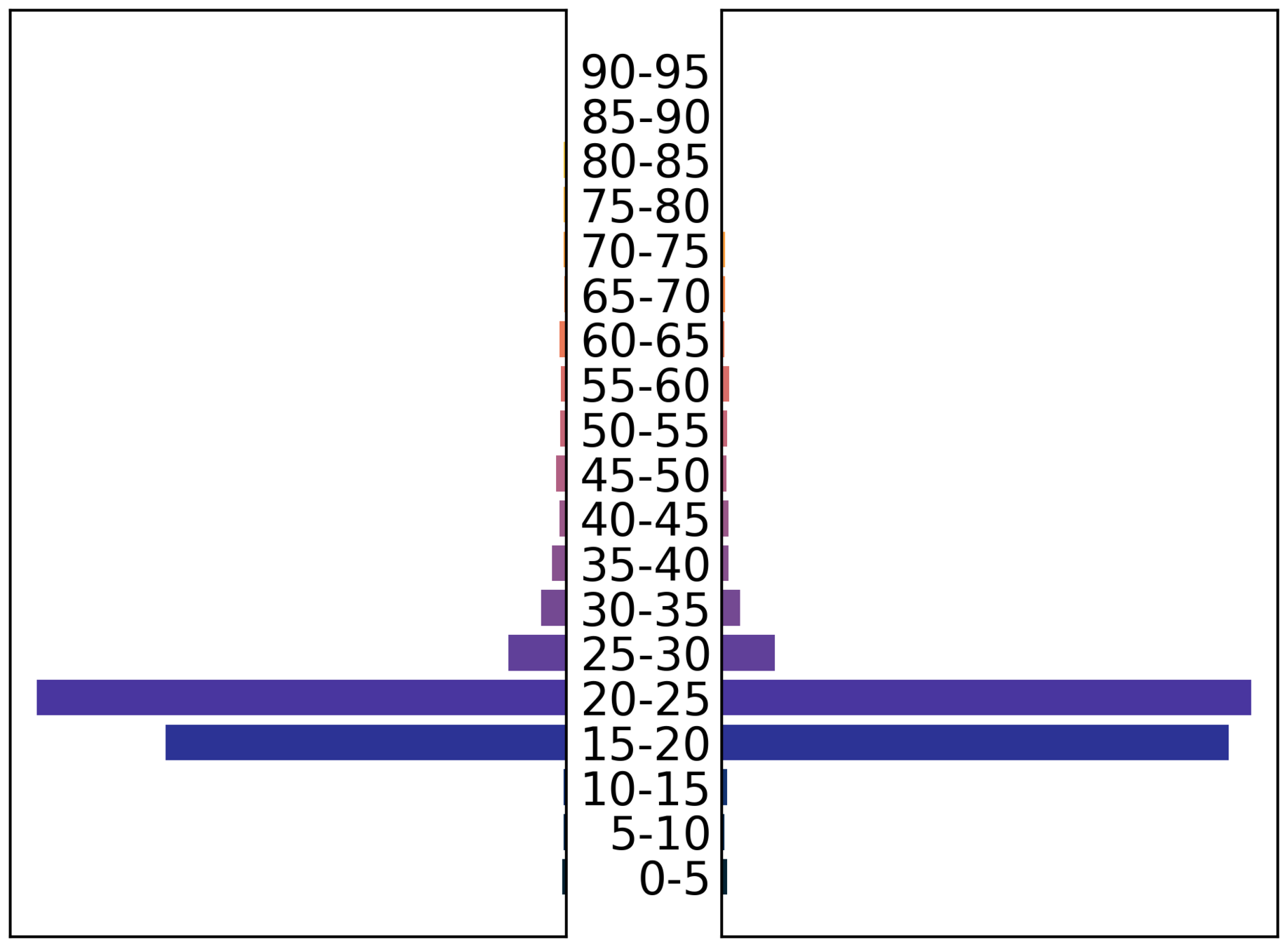
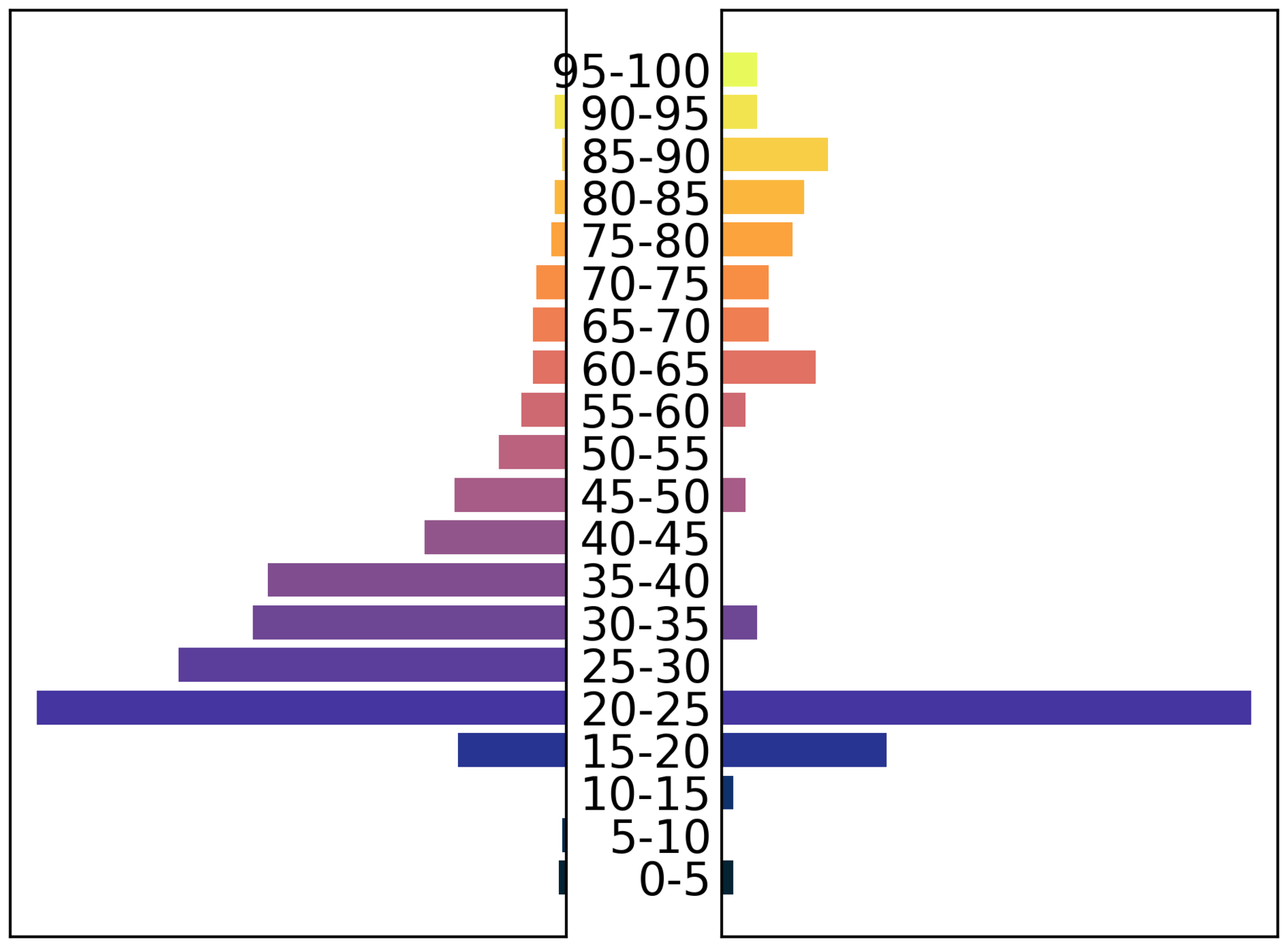
male
female
male
female
What can residents in JUNE do?
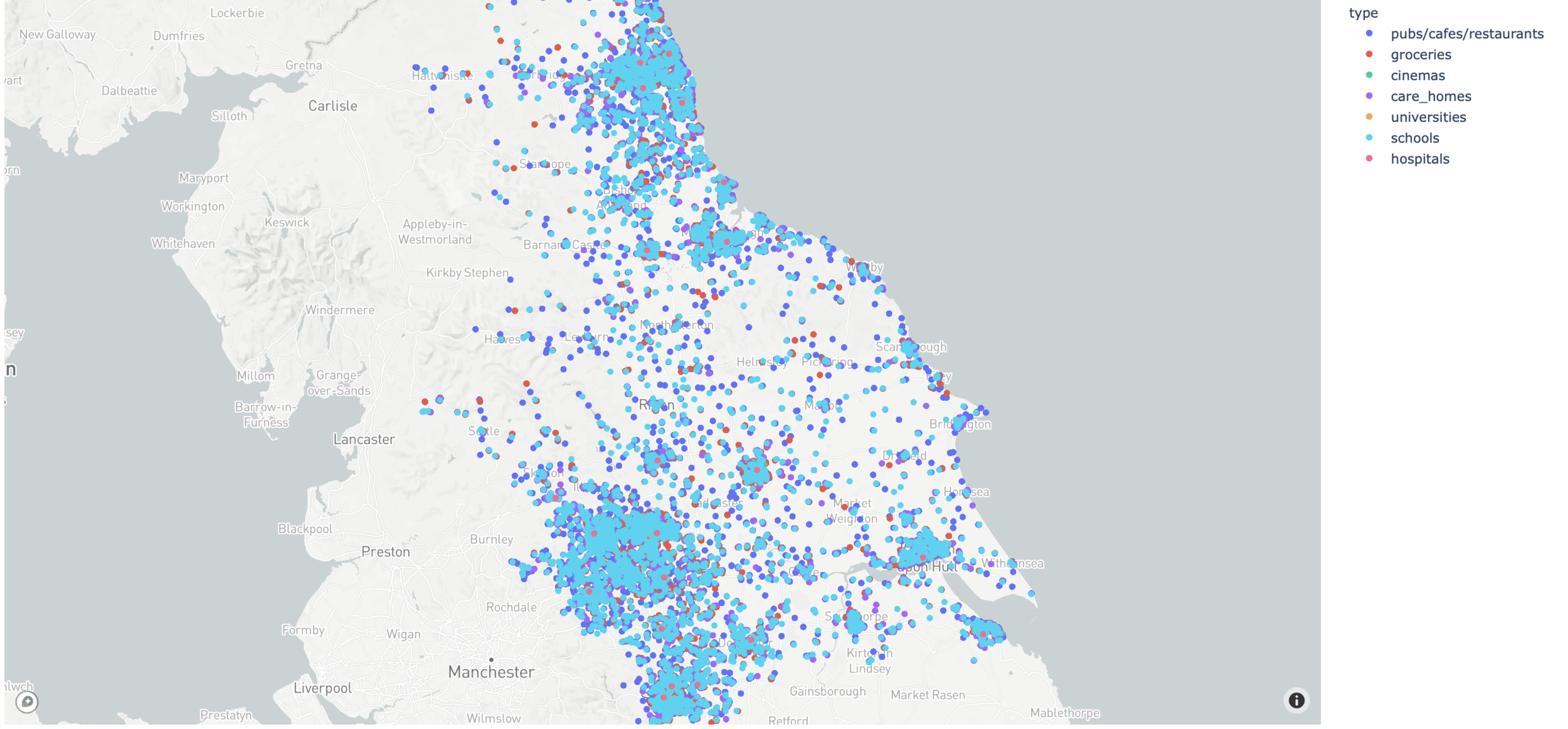
- Residence
- Care Home
- Household
- Primary activity
- Company
- Hospital
- School
- Care Home
- University
- Travel
- Commute
- National Travel
- Leisure
- Shopping
- Pubs/restaurants
- Cinema
- Residence visits
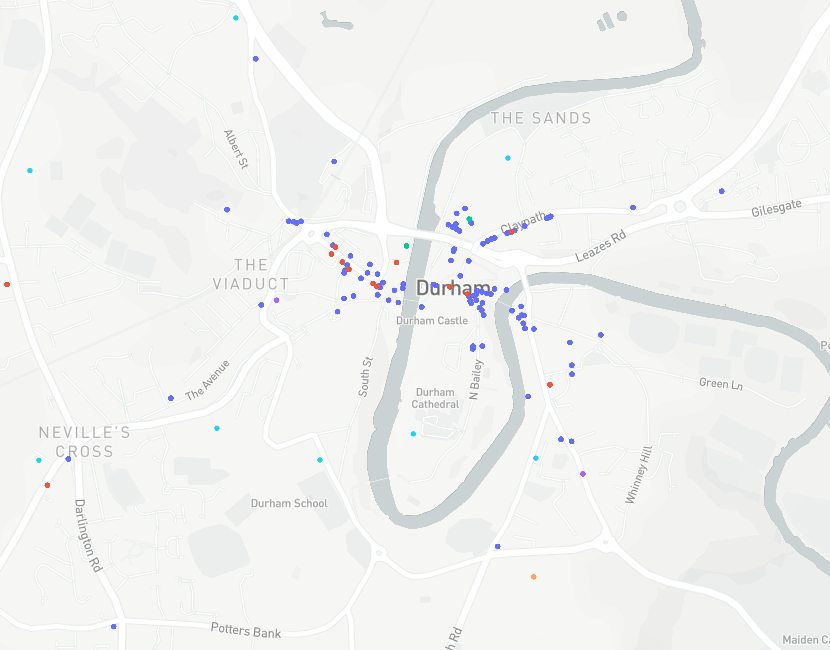
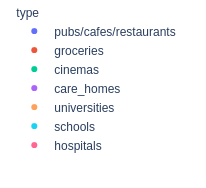
Modelling and mitigation infection
- Model the spread of the infection as related to:
- strength of interaction in a given venue
- proportion of infected venue occupants, and how infectious they are
- number of contacts experienced in a given venue, and length of time
- 'Policy' interventions to reduce the spread of the infection
- Close schools, except for children of key workers
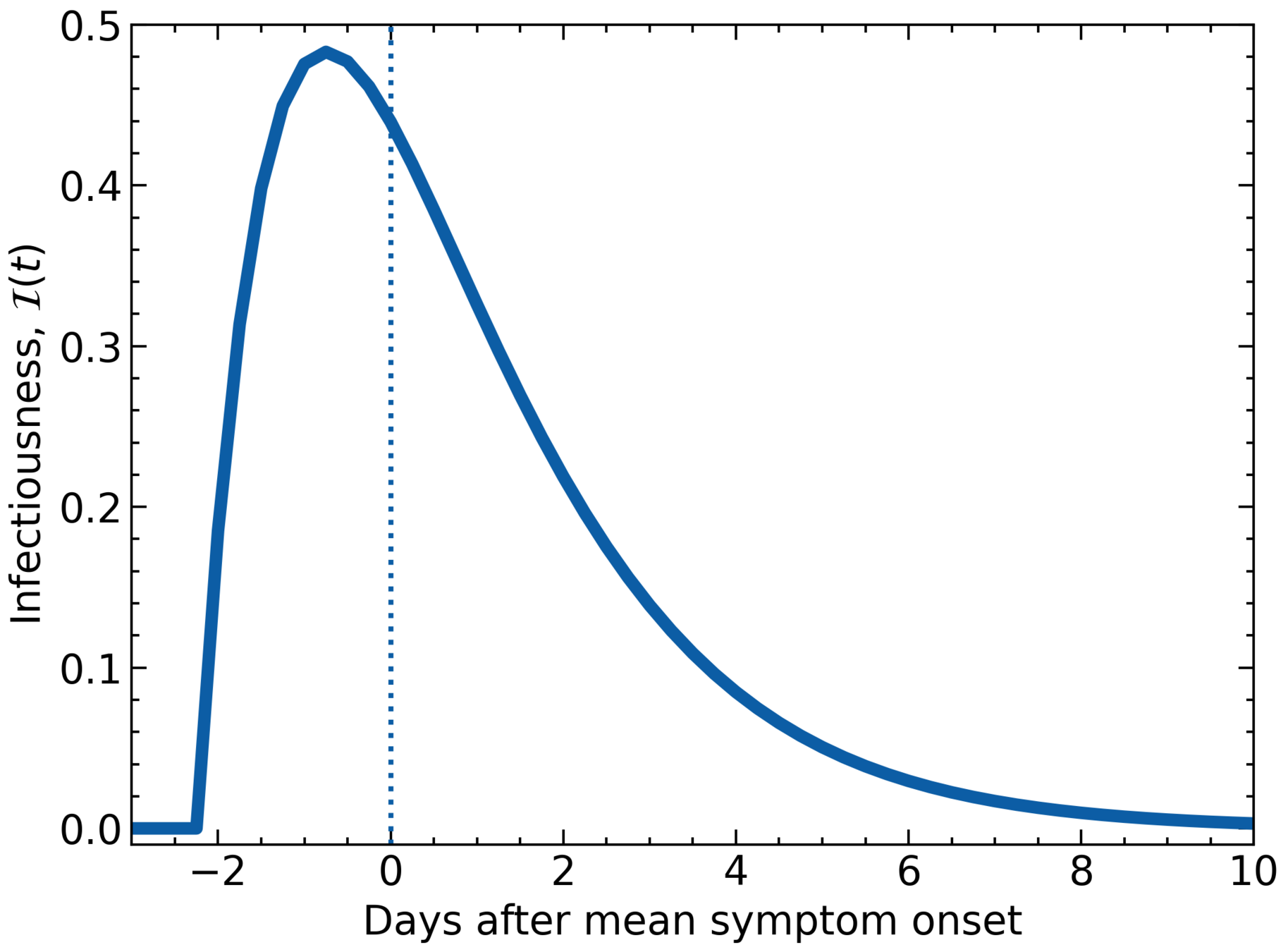
Tuning the model
- Tunable parameters?
- ~10 interaction strengths
- Infection seeding strength
- Proportion of asymptomatic cases
- Policy "interaction strength" reduction
- ...
- Very expensive model to evaluate!
- Use emulators to estimate the behaviour model output between known data points
How does JUNE differ from other models?
- Granularity - demographic based on individual census OAs
- Data outputs
- Precise interventions to quantify the effectiveness of different policies
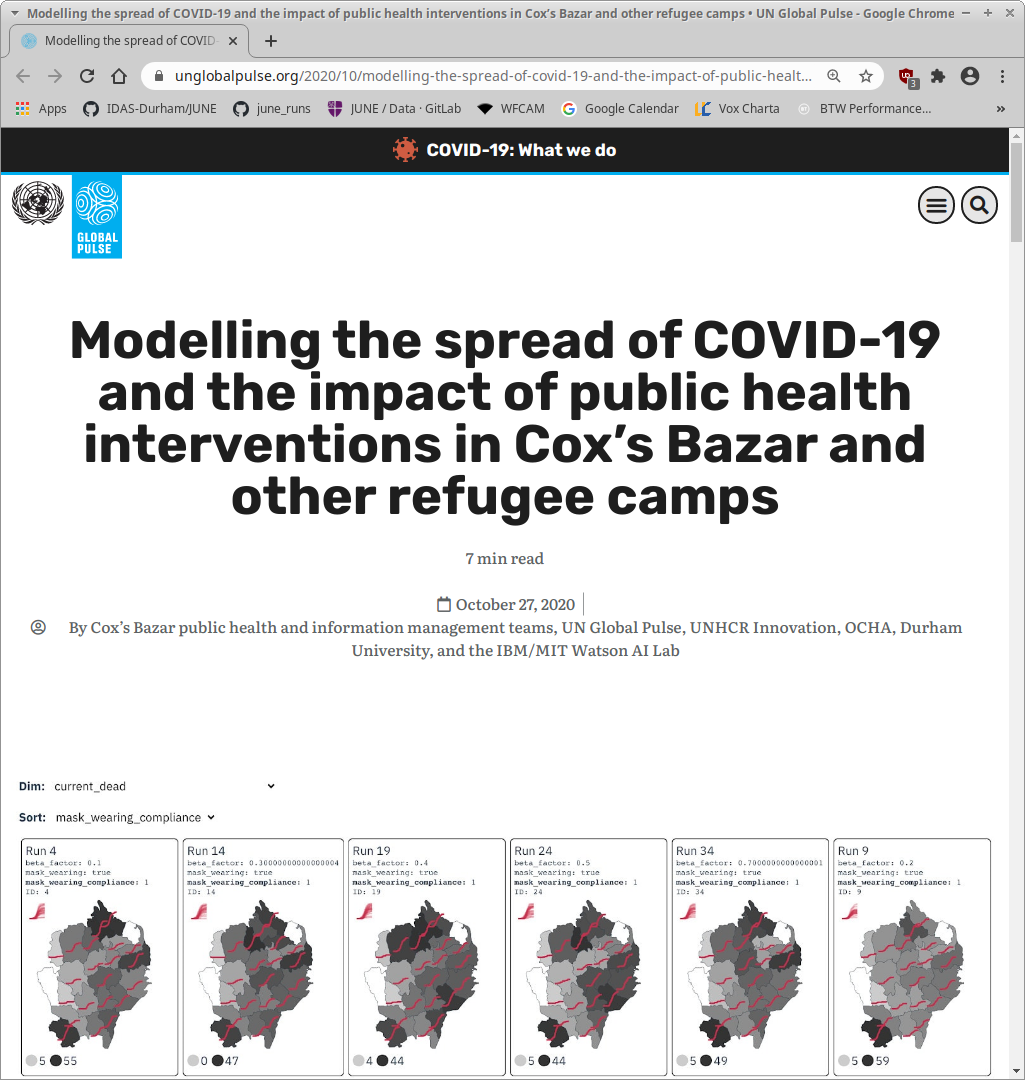
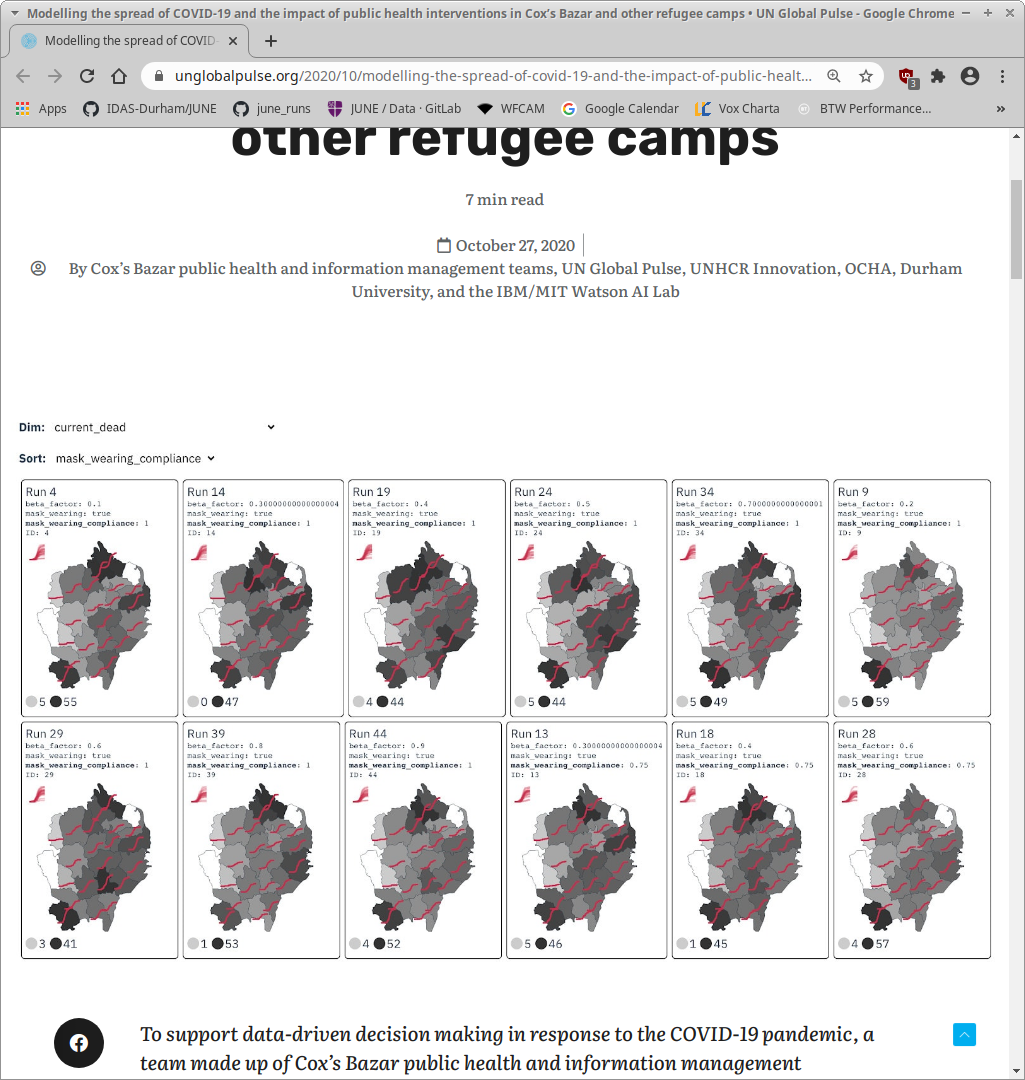
Results: matching the first peak
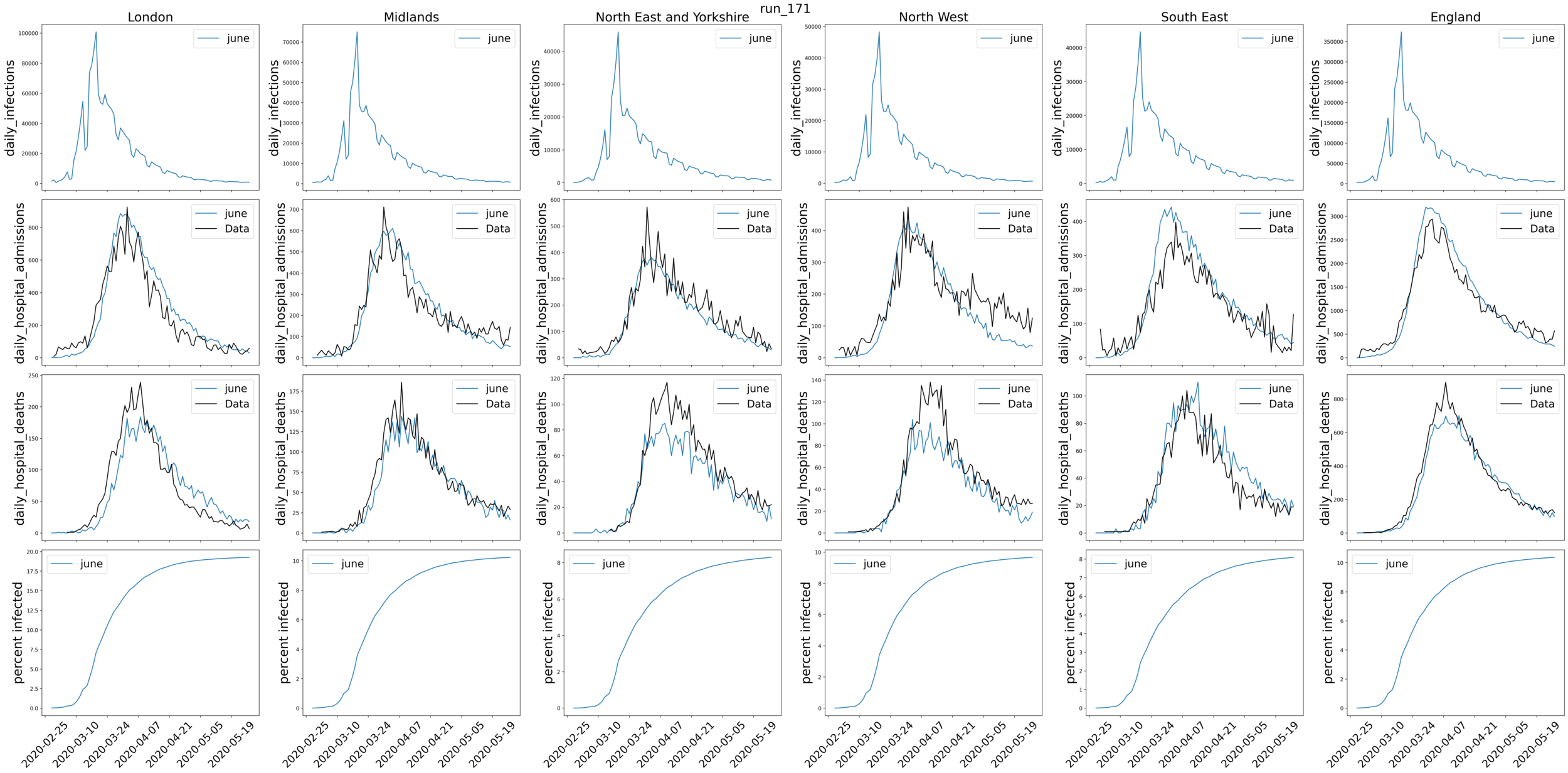
Results: Isolated regions do not capture the infection spread
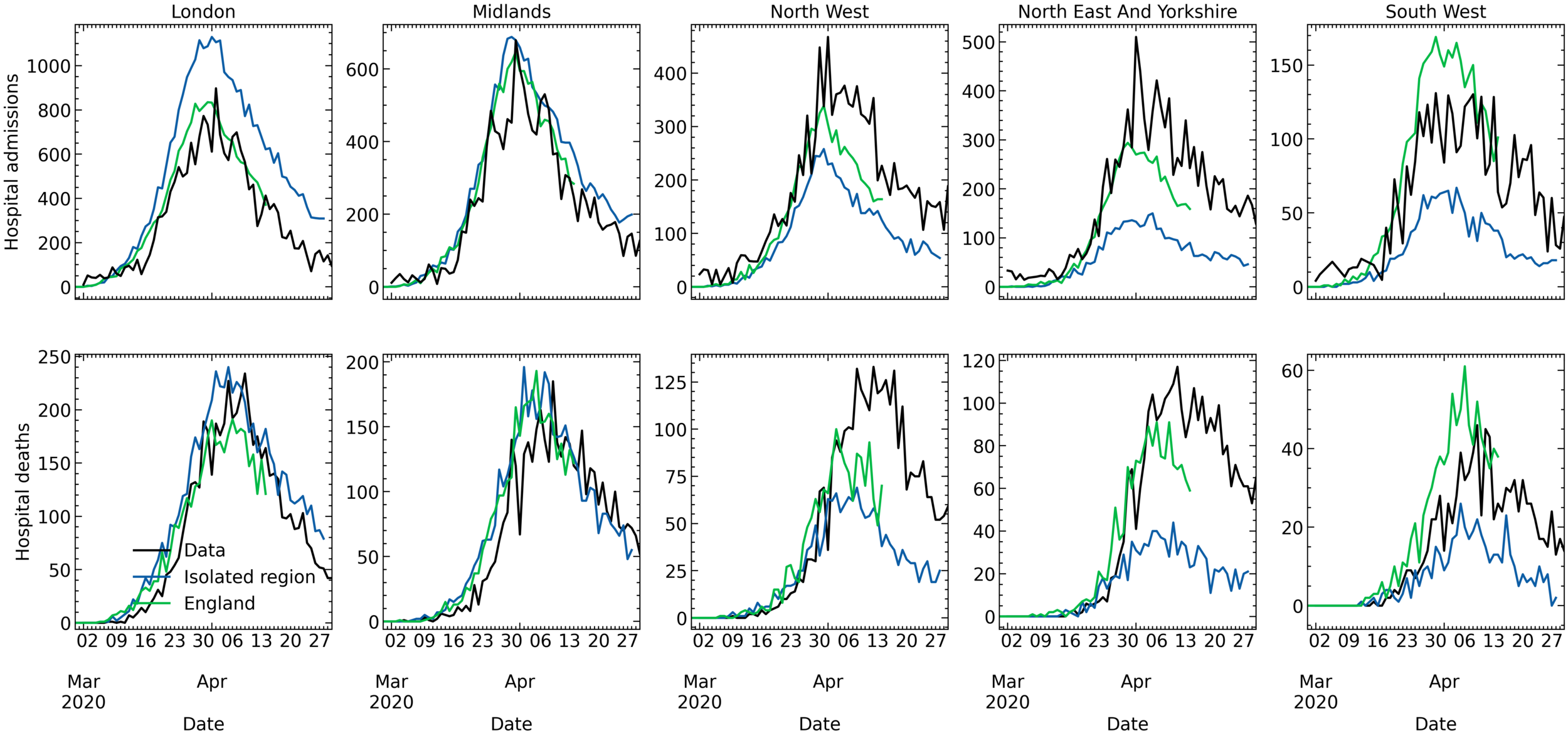
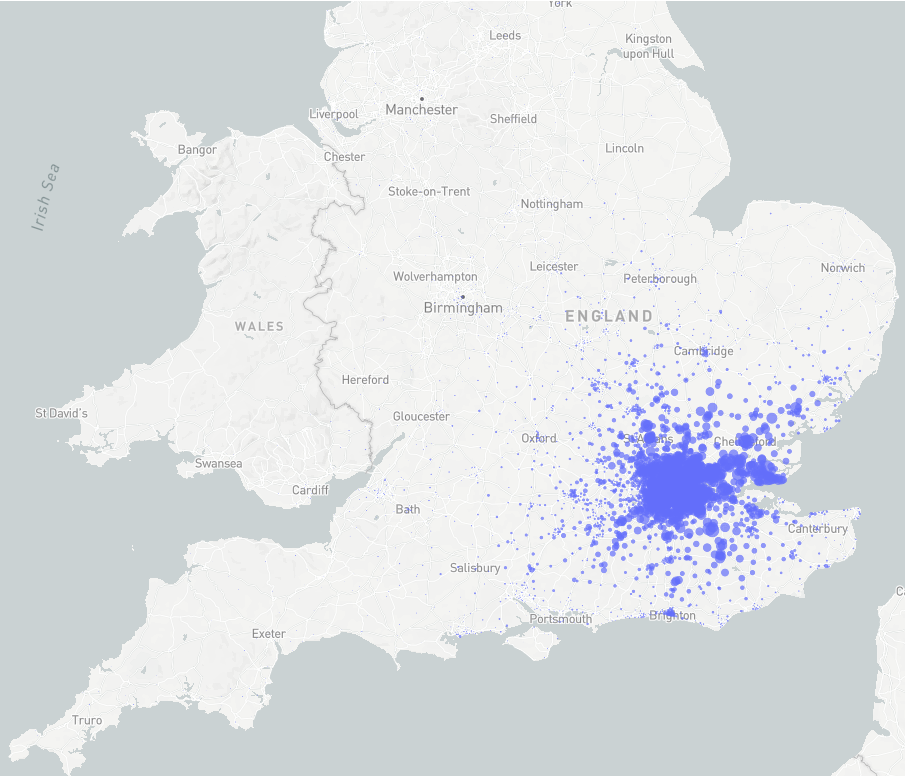
City of London workers' usual residence
Results: prevalence trends by demography
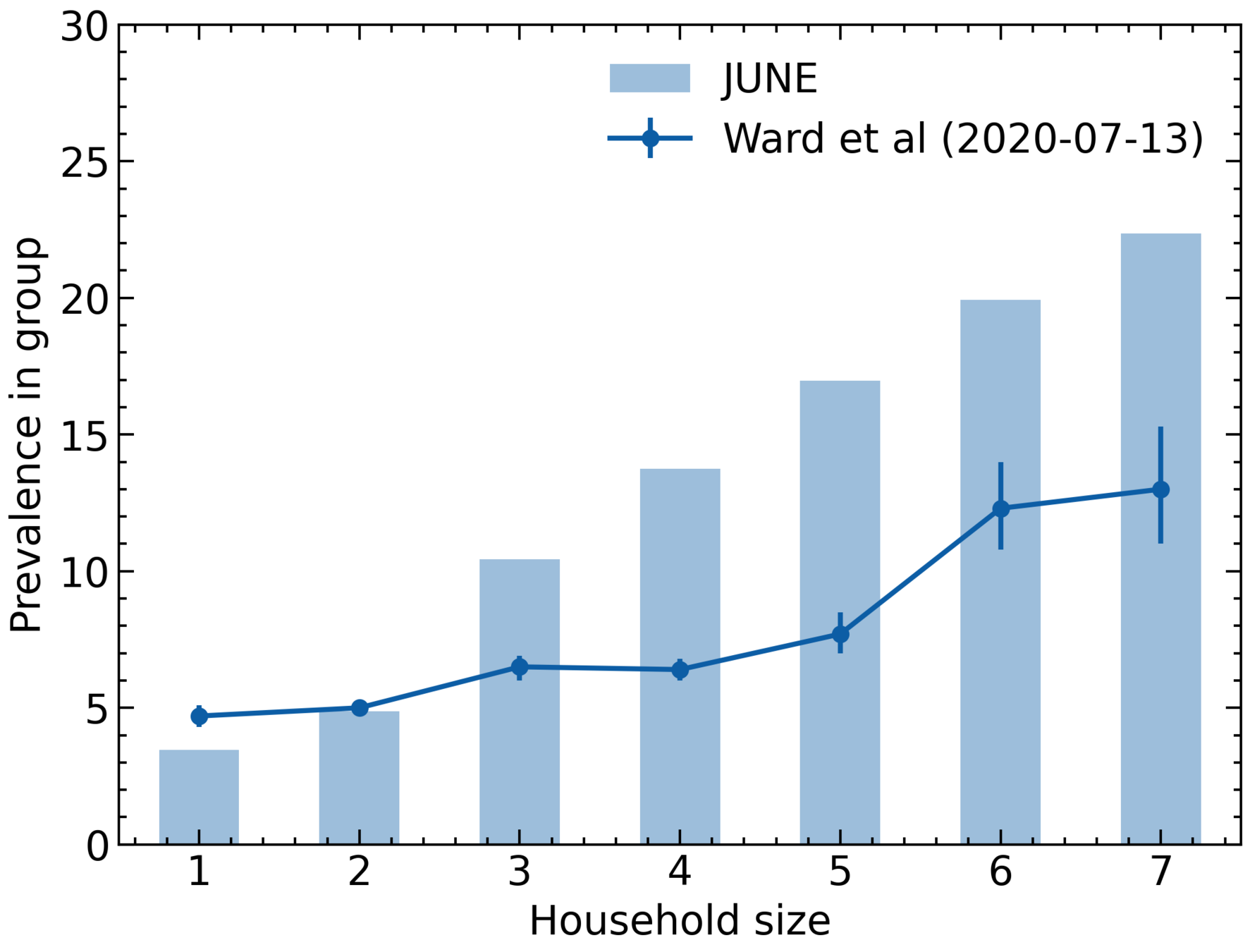
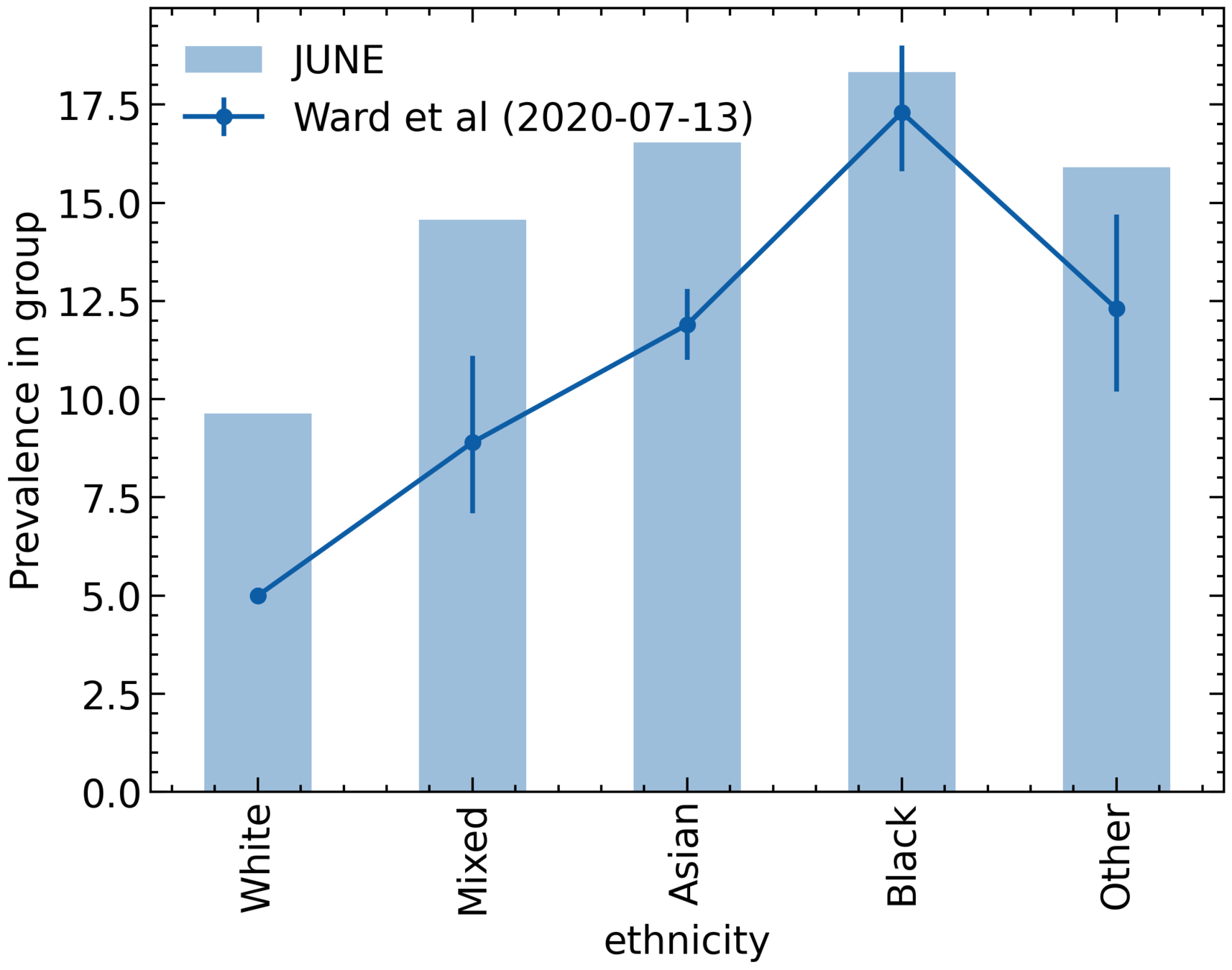
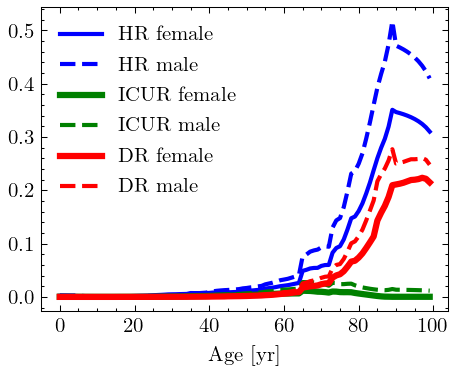
Rates


Infection probability
Intensive care
Asypmtomatic
Mild
Severe
Exposed
Hospitalised
Dead at home
Dead in hospital
Dead in ICU
Recovered
Modelling the spread of Covid-19 in the UK June Dalziel Almeida JUNE Joseph Bullock, Carolina Cuesta-Lazaro, Arnau Quera-Bofarull, Miguel Icaza-Lizaola, Aidan Sedgewick, Henry Truong, Aoife Curran, Edward Elliott, Richard Hayes, James Nightingale, Tristan Caulfield, Kevin Fong, Ian Vernon, Julian Williams, Richard Bower and Frank Krauss
JUNE201102
By aidansedgewick
JUNE201102
- 482