Will ML shape the future of cosmology?
A journey through obstacles and potential approaches to overcoming them
Carolina Cuesta-Lazaro

IAIFI Fellow
The golden days of Cosmology:
A five parameter Universe
Initial Conditions
(Inflation)
Dynamics
Dark energy
Dark matter
Ordinary matter
Amplitude initial density field
Scale dependence
t = 400,000 years

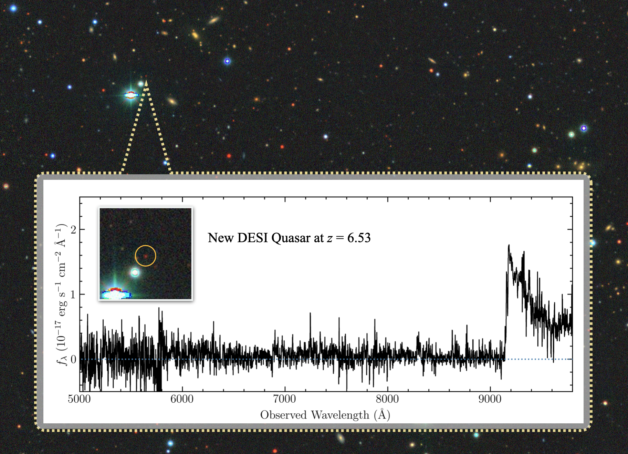
DESI: Dark Energy Spectroscopic Instrument
~35 Million spectra!
Text
(Image Credit: Jinyi Yang, Steward Observatory/University of Arizona)
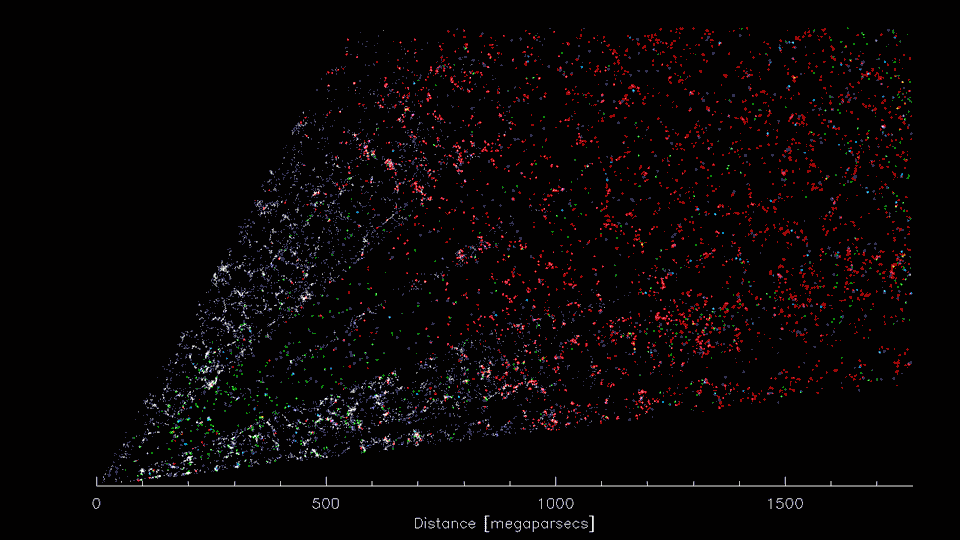
(Image Credit: D. Schlegel/Berkeley Lab using data from DESI)
Neutrino mass hierarchy
Primordial Non-gaussianity
Galaxy formation
Large scale modifications of gravity
using growth to detect the existence of fifth forces
LSS might provide the most accurate measurement
to probe the physics of inflation (single/multi field, particle content)
not only a nuisance to margnilize over!











1
Observe galaxies
4
Pick your favourite analytical likelihood (Gaussian!)
5
Compute ~1Million times to get posterior
Constrain cosmology 101
Missing Information!
Perturbation Theory inaccurate / hard to compute
Is it always true?

3
Work on your analytical theory

2
Count pairs as a function of distance













Enrique Paillas
Waterloo
arXiv:2209.04310
How much information do we lose?
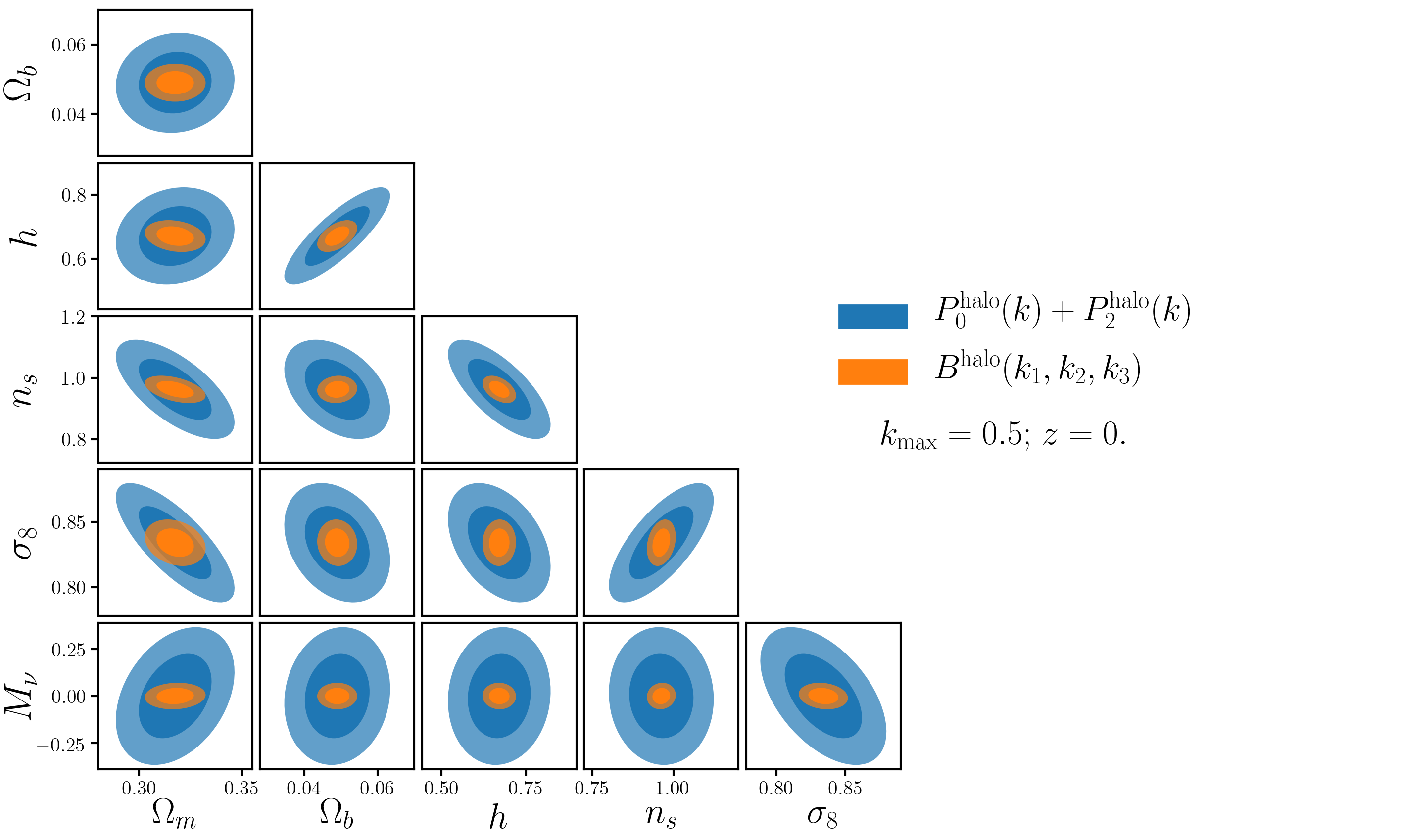
Bispectrum

Wavelet Scattering Transform
arXiv:1909.11107
arXiv:2204.13717
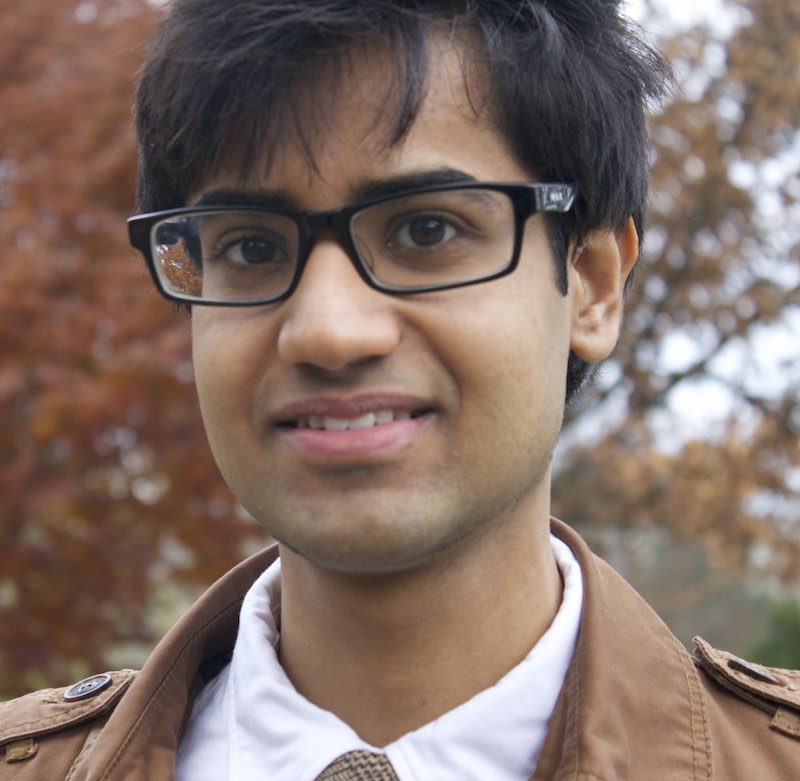
Siddharth Mishra-Sharma
Should we be using CNNs?

Galaxy positions
+ Magnitudes, velocities ...

Dark matter density field




Diffusion on sets
Reverse diffusion: Denoise your data
Forward diffusion: Add noise (fixed)


Set
Set
Neural network

Credit: Siddharth Mishra-Sharma



arXiv:2107.00630
Gaussian,
fully known
Also Gaussian,
but learned mean
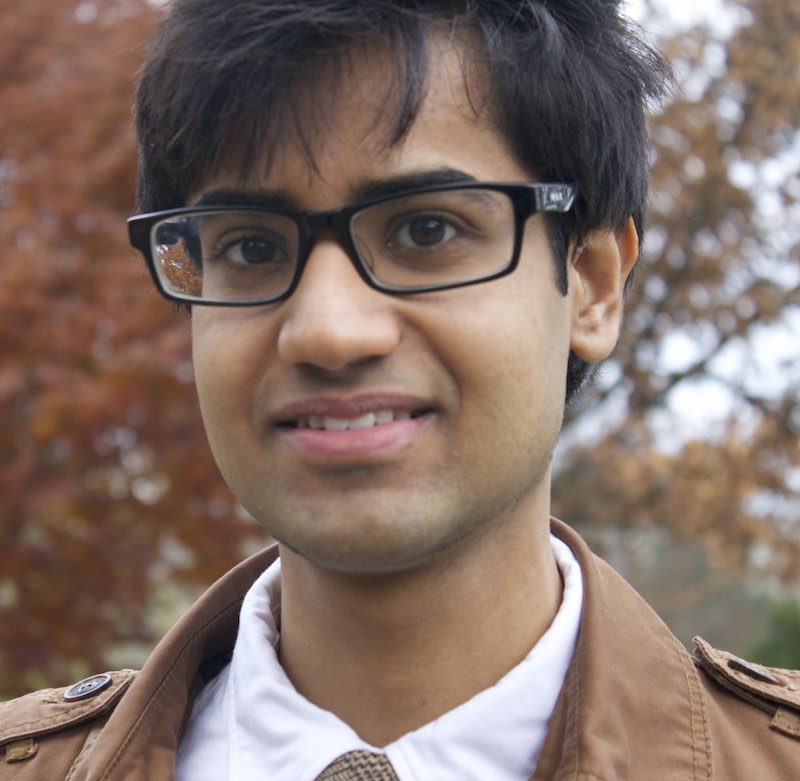
Mario Geiger
Siddharth Mishra-Sharma
Making homogeneous and isotropic universes
Invariant
to rotations and translations
Equivariant
Invariant
Invariant

+ Galaxy formation
+ Observational systematics (Cut-sky, Fiber collisions)
+ Lightcone, Redshift Space Distortions....




Forward Model
N-body simulations
Observations
SIMBIG arXiv:2211.00723

We can simulate the observable Universe, we just need hydrodynamical simulations
What are subresolution models?

Super massive black hole seeding in dark matter halos
BH feedback impacts galactic scales
Black holes can also growth through mergers



Effective models of astrophysical processes needed due to limited numerical resolutions or limited physical models
they can even teleport!
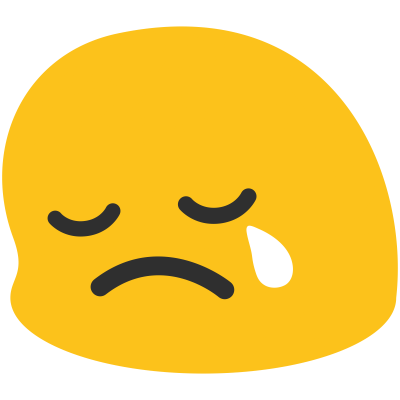

We can't model galaxy formation, how do we make our models robust?
Robust Summarisation
Summariser (neural net)
Increase the evidence of the observations
Sims misspecified


What ML can do for cosmology
- ML to accelerate non-linear predictions and density estimation
- Can ML extract **all** the information that there is at the field-level in the non-linear regime?
- Compare data and simulations, point us to the missing pieces?
cuestalz@mit.edu

Introducing homogeneity and isotropy
Redshift Space Distortions break isotropy

(Credit: E(3)-equivariant graph neural networks for data-efficient and accurate interatomic potentials)
Latent Generative Models: Normalising flows

No assumptions on the likelihood (likelihoods rarely Gaussian!)
No expensive MCMC chains needed to estimate posterior
(Image Credit: Phillip Lippe)

arXiv:2202.05282
Normalising flows as generative models
arXiv:2202.05282

- Fast theory models
- Do all these summaries combined get all info?
- Optimal information extraction
- Density estimation
- Improving current simulations?
- How can we deal with model mispecification?
ML to the rescue for
Invariance vs Equivariance
Invariance
Scalar interactions
Equivariance
What can we do with vectors?
Tensor products
LSS vs CMB

(Image Credit: Julian Bautista at Aix-Marseille University)
deck
By carol cuesta
deck
- 248