Redshift surveys in a nutshell
Learning summary statistics with machine learning
Carolina Cuesta-Lazaro
13th December 2021 - MPA

Collaborators: Cheng-Zong Ruan, Yosuke Kobayashi, Alexander Eggemeier, Pauline Zarrouk, Sownak Bose, Takahiro Nishimichi, Baojiu Li, Carlton Baugh











Fifth forces modify structure growth
GROWTH
- GRAVITY
- FIFTH FORCE
+ EXPANSION
Credit: Cartoon depicting Willem de Sitter as Lambda from Algemeen Handelsblad (1930).


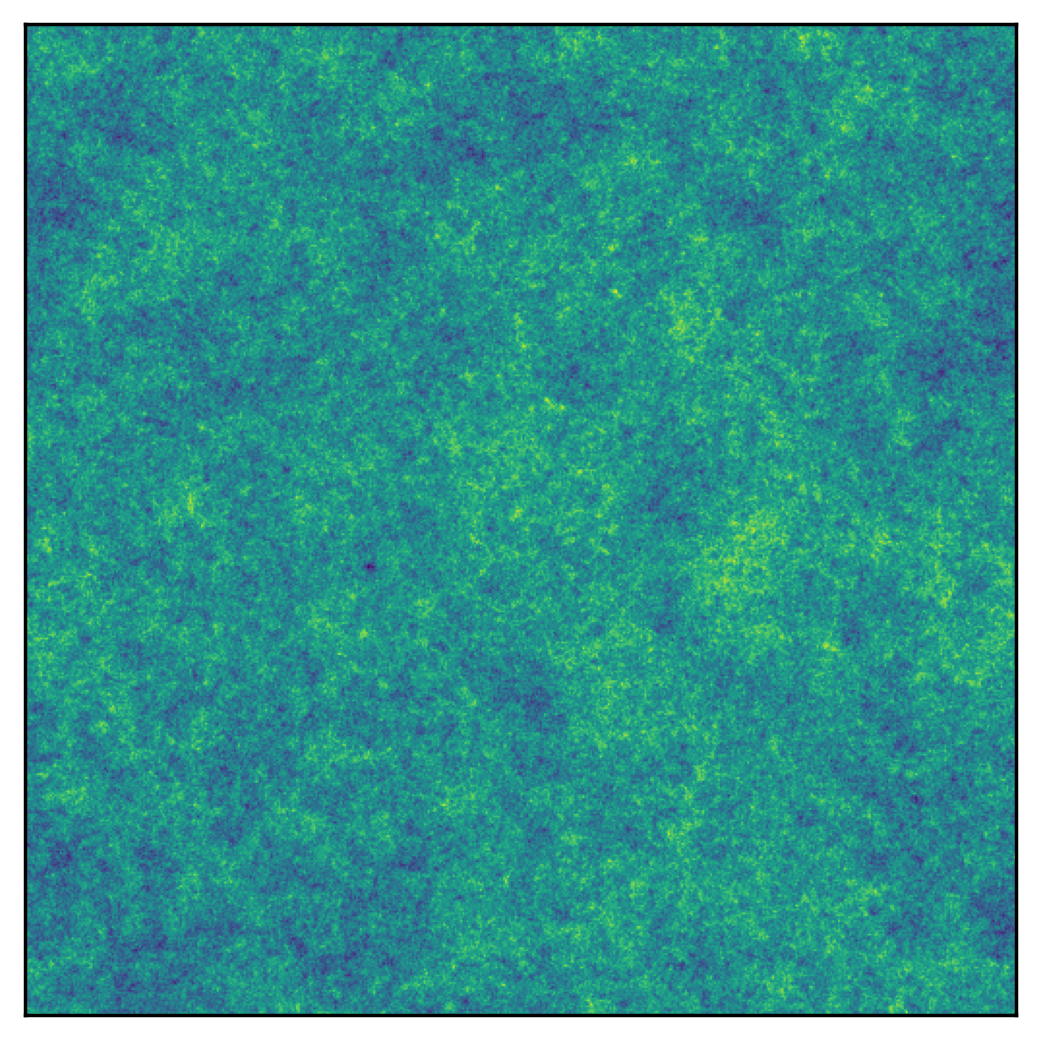











Cosmology =



Main Assumptions
1) Galaxies don't impact dark matter clustering
2) Number of galaxies depends on halo mass only -> Assembly bias?









1) We don't know the Initial Conditions
2) Data is very high dimensional
3) Impact of unknowns (baryonic physics)
4) N-body sims extremely slow to run!
Cosmology =
Galaxy =

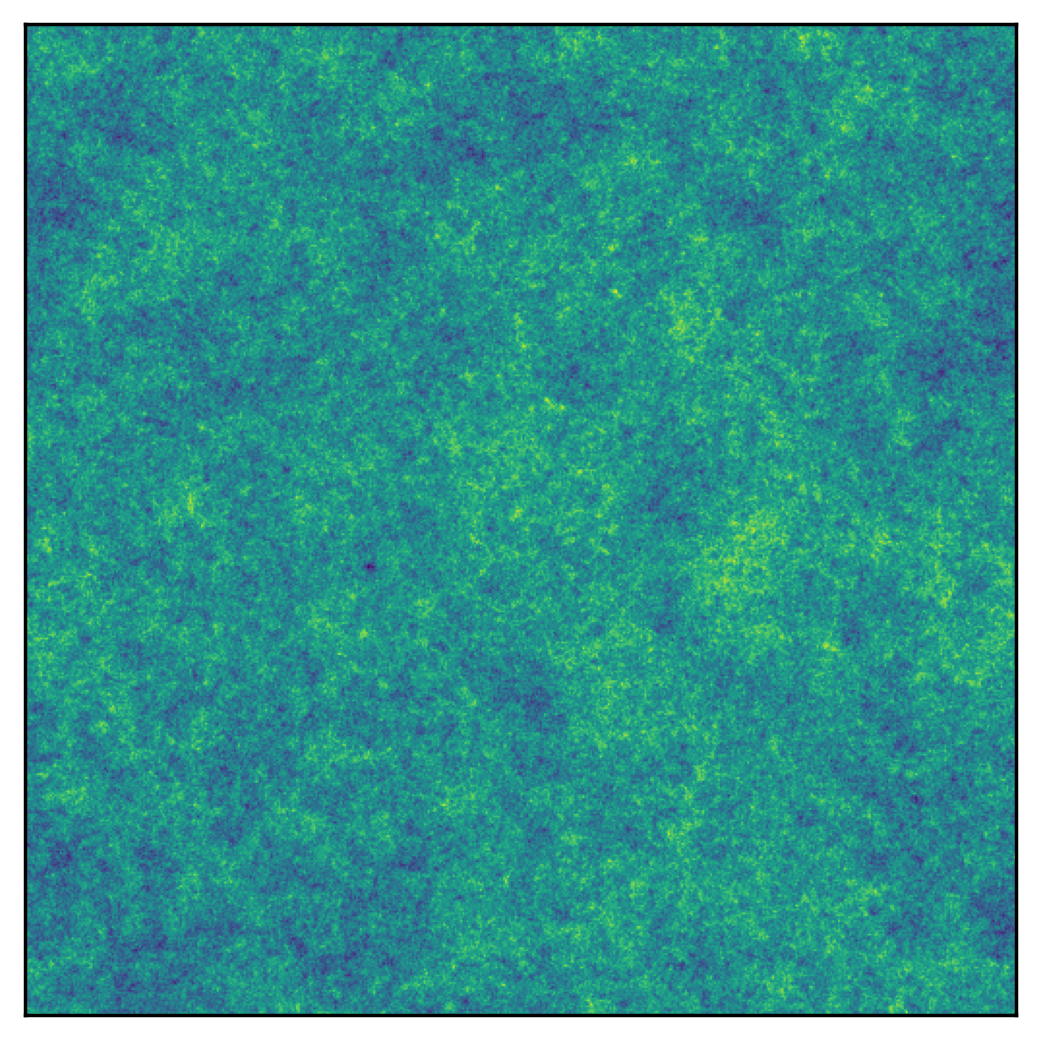





















Summarise the data


1. Modelling Redshift Space Distortions
The Streaming Model
PAIRWISE VELOCITY
DISTRIBUTION




Probability of finding a pair of galaxies at distance r


INFALL
OUTFLOW
On large scales,
slowly varying function of

INFALL
OUTFLOW

Two representative MG models f(R) and nDGP:
- The background expansion is the same as LCDM
- One parameter to describe deviations from LCDM
(same large scale real space clustering)

How do these vary with cosmological parameters on small scales?
Described by four parameters
2. Simulation-based models
Cosmology =

Neural Network Emulator
1) Very fast -> MCMC
2) Halo-Galaxy mapping modelled very accurately
3) Allows for flexible implementations of Halo-Galaxy connection
4) Modelling RSD through the Streaming Model simplifies the functions the emulator needs to learn
Galaxy =



WORK IN PROGRESS
3. Complementary summary statistics












Simplify the model by separating different environments

Voids
Clusters

Assumed density splits identified in real space
How much information is still missing??
Input
x
Neural network
f
Representation
(Summary statistic)
r = f(x)
Output
o = g(r)



Invariance to known unknowns
Increased interpretability through structured inputs
Modelling cross-correlations
Conclusions
- Redshift Space Distortions allow us to constrain gravity models
- We need to account for the non-Gaussian real to redshift space mapping to correctly model deviations from GR
- Can we learn the optimal summary statistic through Machine Learning?
- Current constrains can be improved by extending models to smaller pair separations through N-body simulations
- How limited are we by our Halo-Galaxy connection assumptions?
MPA
By carol cuesta
MPA
- 346