Big Data Cosmology meets AI
IAIFI Fellow
Carolina Cuesta-Lazaro

AstroAI Workshop - 20th June 2024
Video Credit: N-body simulation Francisco Villaescusa-Navarro

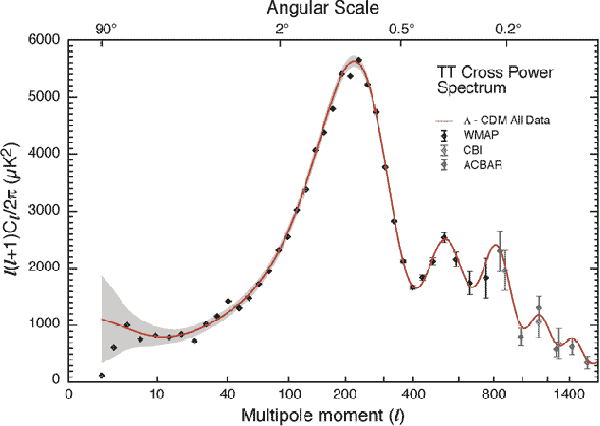
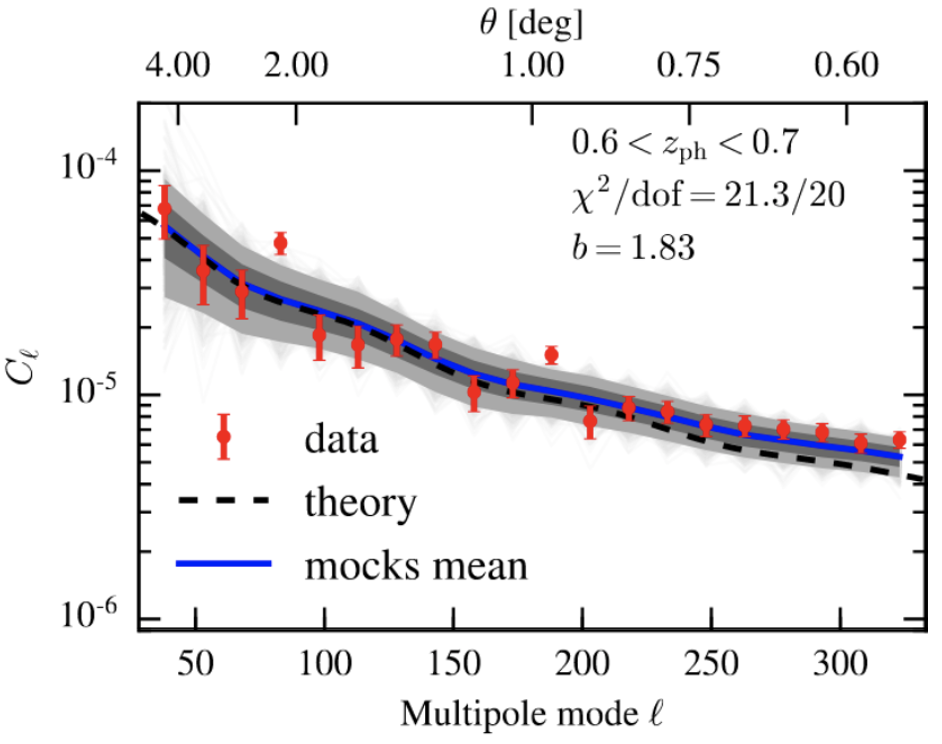
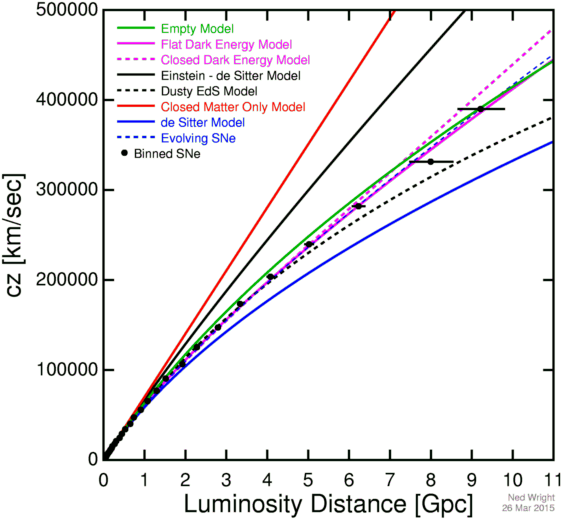
The era of Big Data Cosmology


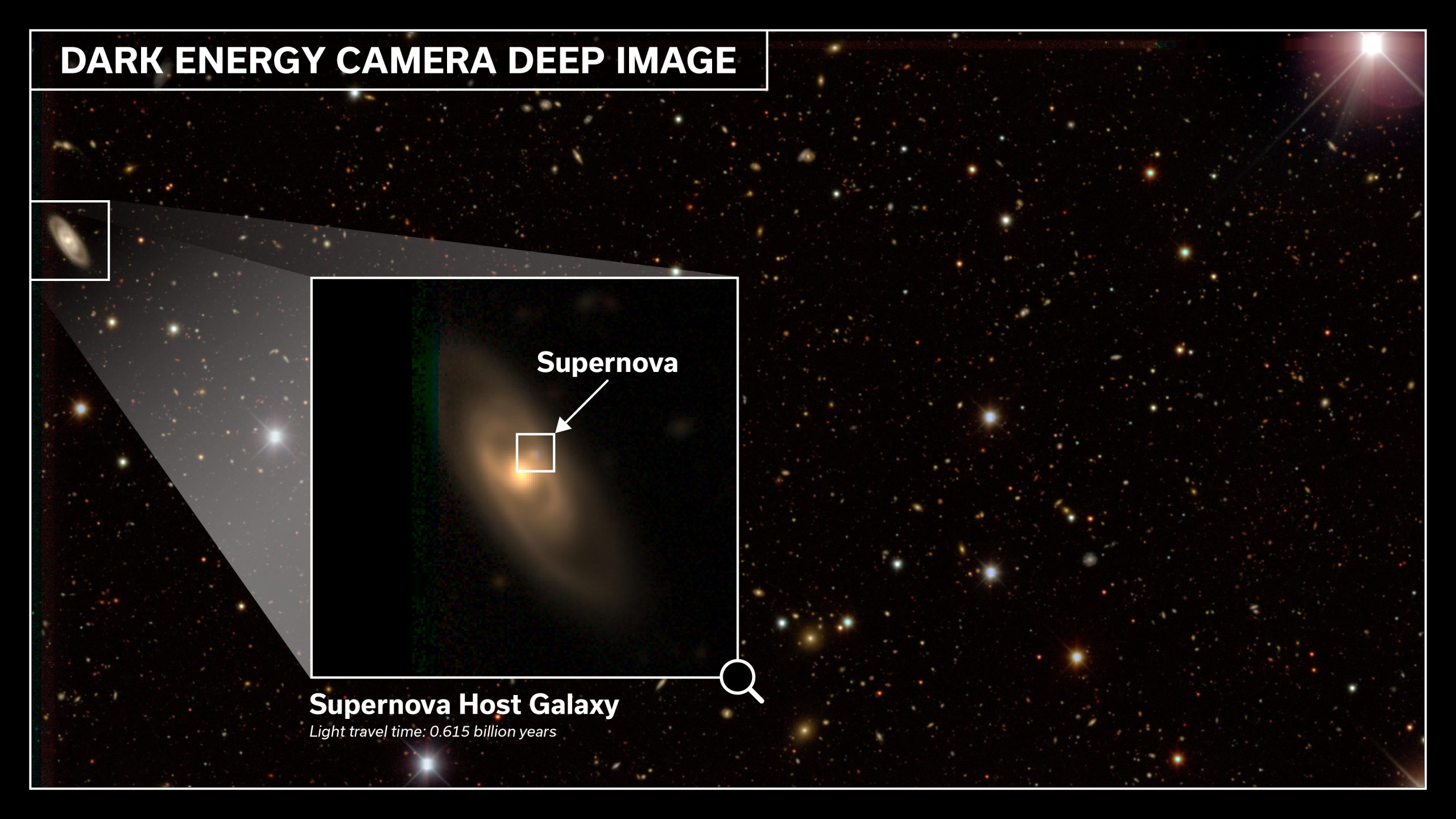
1-Dimensional
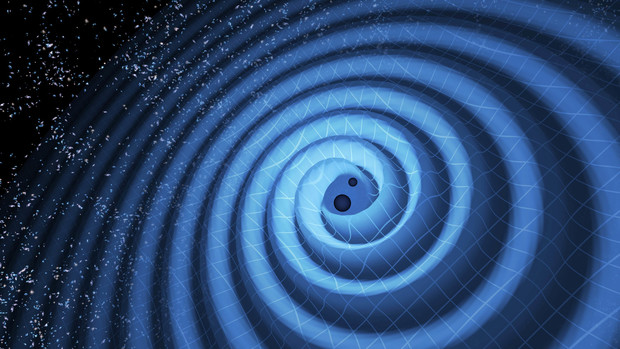

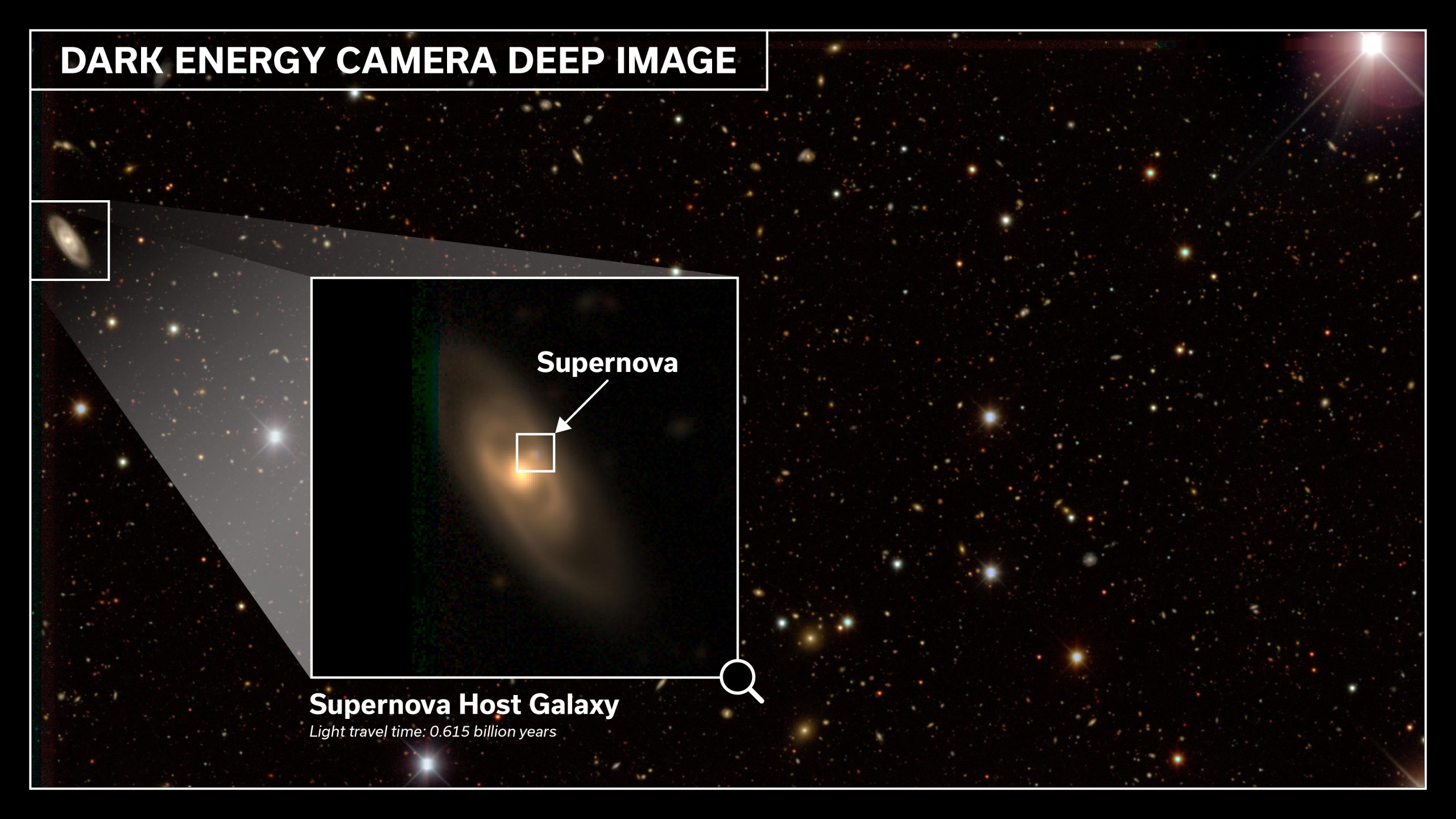
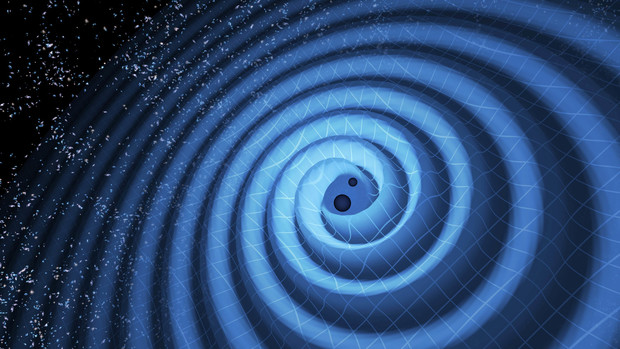

Machine Learning
Secondary anisotropies
Galaxy formation
Intrinsic alignments
Dust
xAstrophysics



DESI, DESI-II, Spec-S5
Euclid
LSST
Simons Observatory
CMB-S4
Ligo
Einstein
LSST
Early Universe Inflation

Late Universe

Energy and matter content
Evolution
Dark matter
Dark energy
Hubble Constant
Baryons
Neutrino masses
Non-Gaussianity
Tilt power spectrum
Hubble tension
Beyond the Standard Model
Multifield Inflation
The Universe's forward model
Cosmological (field level) Inference for Galaxy Surveys
DESI

Point clouds and equivariance


Hybrid ML - Physics Simulators
Learning missing physics
Self-supervised representations
Anomaly detection / OOD
A forward model samples the likelihood
Parameters
Observable
DESI

Forward Model
Dark matter
Dark energy
Inflation

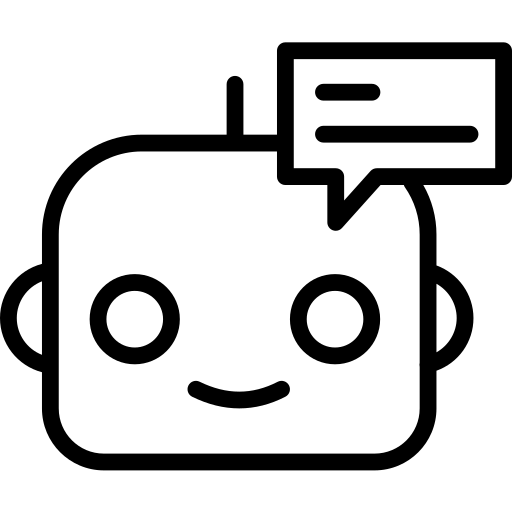
A 2D animation of a folk music band composed of anthropomorphic autumn leaves, each playing traditional bluegrass instruments, amidst a rustic forest setting dappled with the soft light of a harvest moon
Image credit: DALL·E 3
1024x1024


"A point cloud approach to generative modeling for galaxy surveys at the field level"
Cuesta-Lazaro and Mishra-Sharma
arXiv:2311.17141
Base Distribution
Target Distribution
- Sample
- Evaluate

Siddharth Mishra-Sharma

Fixed Initial Conditions
Varying Cosmology

Trained on only 5000 positions!


Nayantara Mudur
"Diffusion-HMC: Parameter Inference with Diffusion Model driven Hamiltonian Monte Carlo"
Mudur, Cuesta-Lazaro and Finkbeiner
in prep


CNN
Diffusion
Increasing Noise
"Diffusion-HMC: Parameter Inference with Diffusion Model driven Hamiltonian Monte Carlo"
Mudur, Cuesta-Lazaro and Finkbeiner
Making homogeneous and isotropic Universes
Base Distribution
Denoiser
Invariant
Equivariant
All learnable functions
All learnable functions constrained by your data
All Equivariant functions
More data efficient!

Equivariant models
Non-equivariant
SEGNN: arXiv:2110.02905
NequIP: arXiv:2101.03164

1 to Many:

https://arxiv.org/abs/2210.02747
https://arxiv.org/abs/2302.00482
Stochastic Interpolants: Bridging arbitrary densities


"Stochastic Interpolants: A Unifying Framework for Flows and Diffusions"
Albergo, Boffi, Vanden-Eijnden
arXiv:2303.08797
Flow ODE

Continuity Equation
Regress the velocity field
Unknown!

Boundary Conditions


Conclusions

1. There is a lot of information in galaxy surveys that ML methods can access
2. We can tackle high dimensional inference problems so far unatainable
3. Our ability to simulate will limit the amount of information we can extract
Hybrid simulators, forward models, robustness, unsupervised problems
Dark matter density reconstruction, Initial Conditions, let's get creative!
Field level inference


cuestalz@mit.edu

Cross correlation

Final
<Reconstructed,True>
Scale (k)
Scale (k)
Small
Large
Small
Large
Initial
<Reconstructed,True>
Comparison to Hamiltonian Monte Carlo for Particle Mesh
AstroAI Workshop2024
By carol cuesta
AstroAI Workshop2024
- 145