follow the slides live at
https://slides.com/delestro/phd-defense/live
A multiple cell tracking method dedicated to the analysis of memory formation in vivo
Felipe Delestro
25th October 2018
PhD defense
under the supervision of Auguste Genovesio







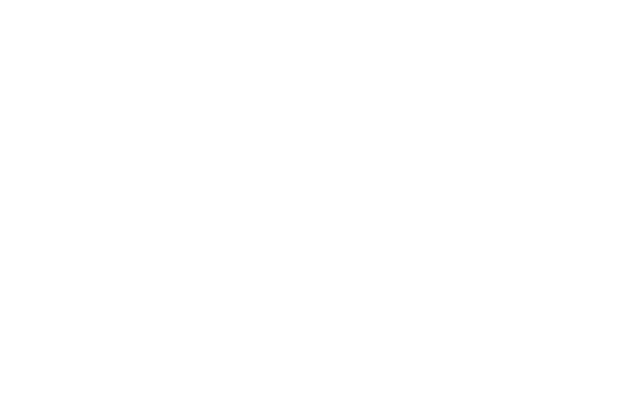



MELANIE PEDRAZZANI
LISA SCHEUNEMANN
Gènes et Dynamique des Systèmes de Mémoire
ESPCI

THOMAS
PREAT
Bio-Imagerie Computationnelle et Bioinformatique
IBENS
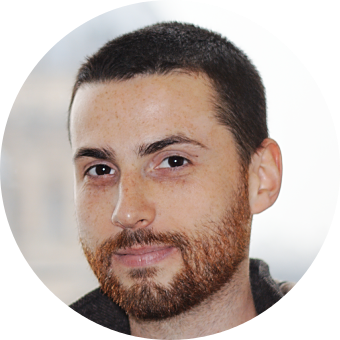

AUGUSTE
GENOVESIO
FELIPE
DELESTRO

If the brain were so simple we could understand it, we would be so simple we couldn't. Lyall Watson

Homo sapiens
5cm

Bandettini PA,
Twenty years of functional MRI: The science and the stories.
Neuroimage. 62(2):575–588. (2012)
Mus musculus
Homo sapiens
5cm

Moser M-B, Rowland DC, Moser EI.
Place Cells, Grid Cells, and Memory.
Cold Spring Harbor Perspectives in Biology. (2015)

Drosophila
melanogaster
1 mm
ODOR

Octan-3-ol (OCT)
ODOR
A. Pascual, & T. Préat,
Localization of long-term memory within the Drosophila mushroom body.
Science 294, 1115–1117 (2001).

Octan-3-ol (OCT)
Dolan DNA Learning Center
Cold Spring Harbor Laboratory
www.dnalc.org
Yu, D., Keene, A. C., Srivatsan, A., Waddell, S. & Davis, R. L.
Drosophila DPM neurons form a delayed and branch-specific memory trace after olfactory classical conditioning.
Cell 123, 945–957 (2005).

In quantitative terms, what are the changes in the brain after learning, at the single cell level ?


Honegger, K. S., Campbell, R. a a & Turner, G. C.
Cellular-resolution population imaging reveals robust sparse coding in the Drosophila mushroom body.
J. Neurosci. 31, 11772–85 (2011)
state of the art
- Analysis only for 2D slices
(incomplete view of the brain)
- Manual or assisted detection
(biased and low throughput)




Tugba, G.-O., Davis, R. L., Guven-ozkan, T. & Davis, R. L. Functional neuroanatomy of Drosophila olfactory memory formation. Learn. Mem. 21, 519–526 (2014)
Campbell, R. a a et al. Imaging a population code for odor identity in the Drosophila mushroom body. J. Neurosci. 33, 10568–81 (2013)
Lin, A. C., Bygrave, A. M., de Calignon, A., Lee, T. & Miesenböck, G. Sparse, decorrelated odor coding in the mushroom body enhances learned odor discrimination. Nat. Neurosci. 17, 559–68 (2014)
Aso, Y. et al. The neuronal architecture of the mushroom body provides a logic for associative learning. Elife 3, e04577 (2014)
Strategy
-
Record the full extent of the Mushroom body in 3D
By using of a confocal spinning disk we'll be able to perform fast and complete acquisitions
-
Automatically detect the neurons in 3D
An automated procedure guarantees an unbiased analysis
-
Analyse the difference between groups
A quantitative approach will allow us to infer how the memory trace is represented in the brain
mCherry & GCaMP
with a VT30559-GAL4 promoter
Expression is restricted to the kenyon cells (mushroom body neurons)

mCherry & GCaMP
with a VT30559-GAL4 promoter
Expression is restricted to the kenyon cells (mushroom body neurons)




MELANIE PEDRAZZANI
LISA SCHEUNEMANN
Dimensions
X: 256 pixels (41.28µm)
Y: 512 pixels (82.56µm)
Z: 45 slices (67.50µm)
Acquisition time
20ms per slice
0.9 s per stack
135 s per acquisition
mCherry
GCaMP
Dimensions
X: 256 pixels (41.28µm)
Y: 512 pixels (82.56µm)
Z: 45 slices (67.50µm)
Acquisition time
20ms per slice
0.9 s per stack
135 s per acquisition
mCherry
GCaMP
About 200 recordings of unique flies where made for the standardisation of the protocol
Aditional 122 flies were used for the final analysis
(more than any other work)
This corresponds to about 4.6 hours of recorded signal, stored in more than 800 thousands of image slices
Data challenges
XY
YZ
Nuclei (mCherry) | Max projections

x
y
z
mCherry (nuclei)
Volumetric reconstruction


normal
artifact


normal
artifact


Max projection through time
Slices of problematic stack

Nuclei (mCherry)
Signal (GCaMP)

Natural brain movement
x
y
z
y
Data analysis
DETECTION
TRACKING
SIGNAL
DETECTION
TRACKING
SIGNAL
Find the position of the nuclei of neurons from the Mushroom body
Follow each one of the detected neurons through time
Measure the correspondent signal for the individual neurons
DETECTION
TRACKING
SIGNAL
Neuron detection
The goal is to find the positions of nuclei from the Mushroom body, so that the individual neurons can be identified
normal
artifact
normal
artifact


Toyoshima, Y. et al.
Accurate Automatic Detection of Densely Distributed Cell Nuclei in 3D Space.
PLoS Comput. Biol. 12, 1–20 (2016)


Štěpka, K. et al.
Performance and sensitivity evaluation of 3d spot detection methods in confocal microscopy.
Cytom. Part A (2015)
Estimating nuclei size
Using the Full Width at Half maximum, we can estimate the nuclei diameter

time frames
0
250
Detection validation
Synthetic images
A computer generate image, with similar characteristics to the original image, and known ground truth
Detection validation
synthetic
real
Synthetic images
A computer generate image, with similar characteristics to the original image, and known ground truth
Detection validation
Manual annotation
Using the CellCounter ImageJ plugin to manually mark the nuclei position in 3D
Detection validation
Real image
Synthetic image


time frames
0
250
DETECTION
TRACKING
SIGNAL
Neuron tracking
Once having the positions of every neuron, we need to link the detections trough time.


Chenouard, N. et al.
Objective comparison of particle tracking methods.
Nat. Methods 11, 281–289 (2014)
Non-rigid deformation
Natural movements of the brain cause deformations during the acquisition


top view
side view
Frame 1
Frame 2
Frame 1
Frame 2

Myronenko, A. & Song, X.
Point set registration: coherent point drift.
IEEE Trans. Pattern Anal. Mach. Intell. 32, 2262–75 (2010).
Ester, M., et al.
A Density-Based Algorithm for Discovering Clusters in Large Spatial Databases with Noise.
Proceedings of the 2nd International Conference on Knowledge Discovery and Data Mining, pp. 226-231. (1996)
DBSCAN
Original detections
Registered detections



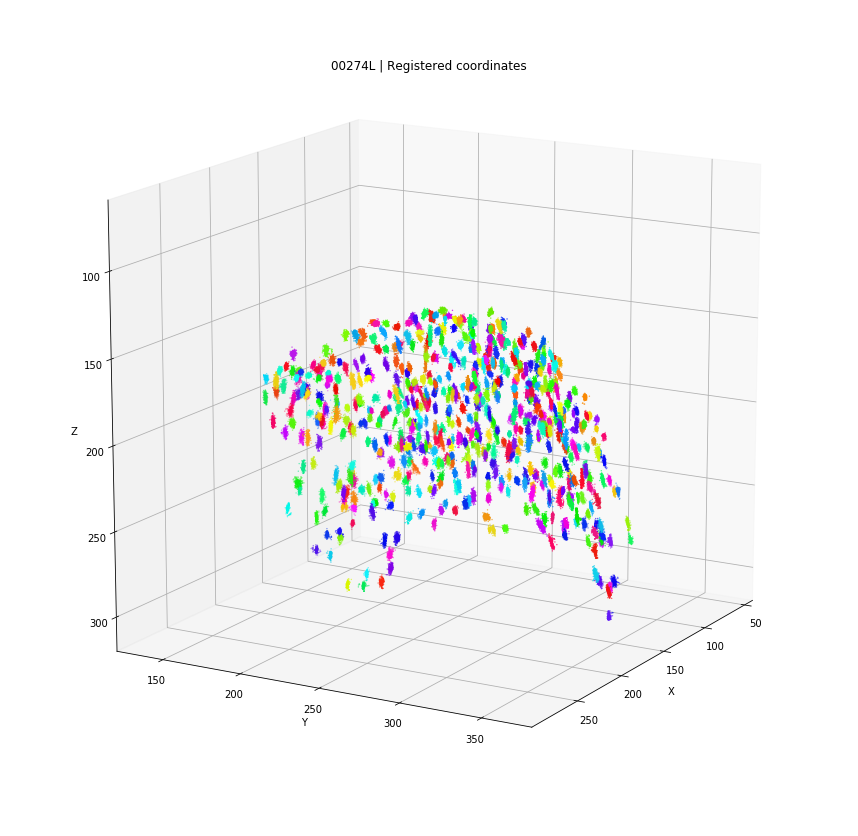
time frames
clusters

Clusters in original coordinates


time frames
0
400
Every detection projected into a single 3D space
Tracks for one of the detected clusters
cluster fix
using time information to fix merged clusters
cluster fix
using time information to fix merged clusters
cluster fix
using time information to fix merged clusters
cluster fix
using time information to fix merged clusters
cluster fix
using time information to fix merged clusters
original detections
tracked nuclei
DETECTION
TRACKING
SIGNAL
Measuring signal
The last step is to actual measure the activity signal from the neurons, while the fly receives a stimulus

intensity 2d histogram
Nuclei & Signal
Axial max projection




before stim
during stim




Memory traces
What are the quantitative changes in the brain to represent the memory trace ?
unpaired control
paired conditioning
Unpaired (no long-term memory)
Paired (long-term memory formed)
Neuron recruitment
Response intensity
Mann-Whitney two-sided test
p-value: 0.0014
t-test
p-value: 0.248
Contributions
Data acquisition
Detection & Tracking
Memory traces
Fine-tuning of a system capable of acquiring 3D+Time images of the Mushroom body, while the fly experiences an odor
Development of an open-source and fully automated detection and tracking algorithm, capable of handling the high density of neurons and movements of the brain
This unique set-up allowed us to quantify, for the first time, the recruitment of new neurons during long-term memory formation
Perspectives

Graph features
More subtle characteristics of the neuronal activation pattern could be revealed by the use of graphs
Training during acquisition
An update on the fly chamber could allow the electric stimulation during the imaging, showing live how the brain is reacting
Genetic modifications
The system here developed would allow us to verify how different levels of expression of specific genes change the neuron recruitment

Song, L. L., Liang, R., Li, D. D. & Al., E. A
Systematic Analysis of Human Disease-Associated Gene Sequences In Drosophila melanogaster.
Genome Res. 59, 1114–1125 (2001)
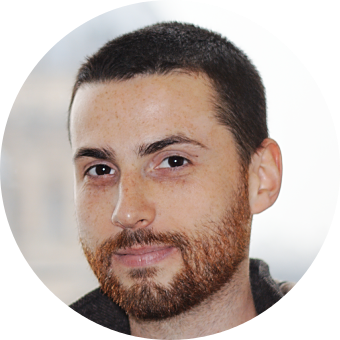







2015
2016
Jul Aug Sep Oct Nov Dec Jan Feb Mar Apr May Jun Jul Aug Sep Oct Nov Dec Jan Feb Mar Apr May Jun Jul Aug Sep Oct Nov Dec Jan Feb Mar Apr May Jun Jul Aug Sep Oct Nov Dec Jan Feb Mar Apr May Jun Jul Aug Sep Oct
2014
AUGUSTE GENOVESIO
FELIPE DELESTRO
MINHUI WU
THOMAS PREAT
PAUL TCHENIO
MELANIE PEDRAZZANI
YANN DROMARD
LISA SCHEUNEMANN
2017
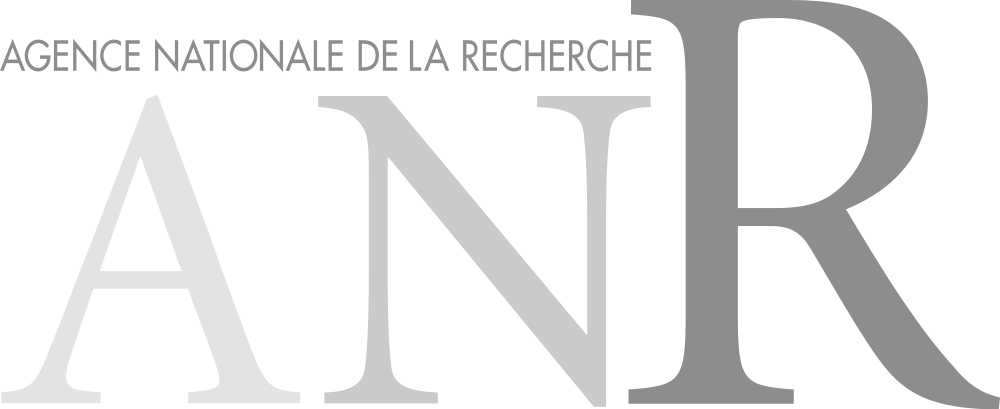







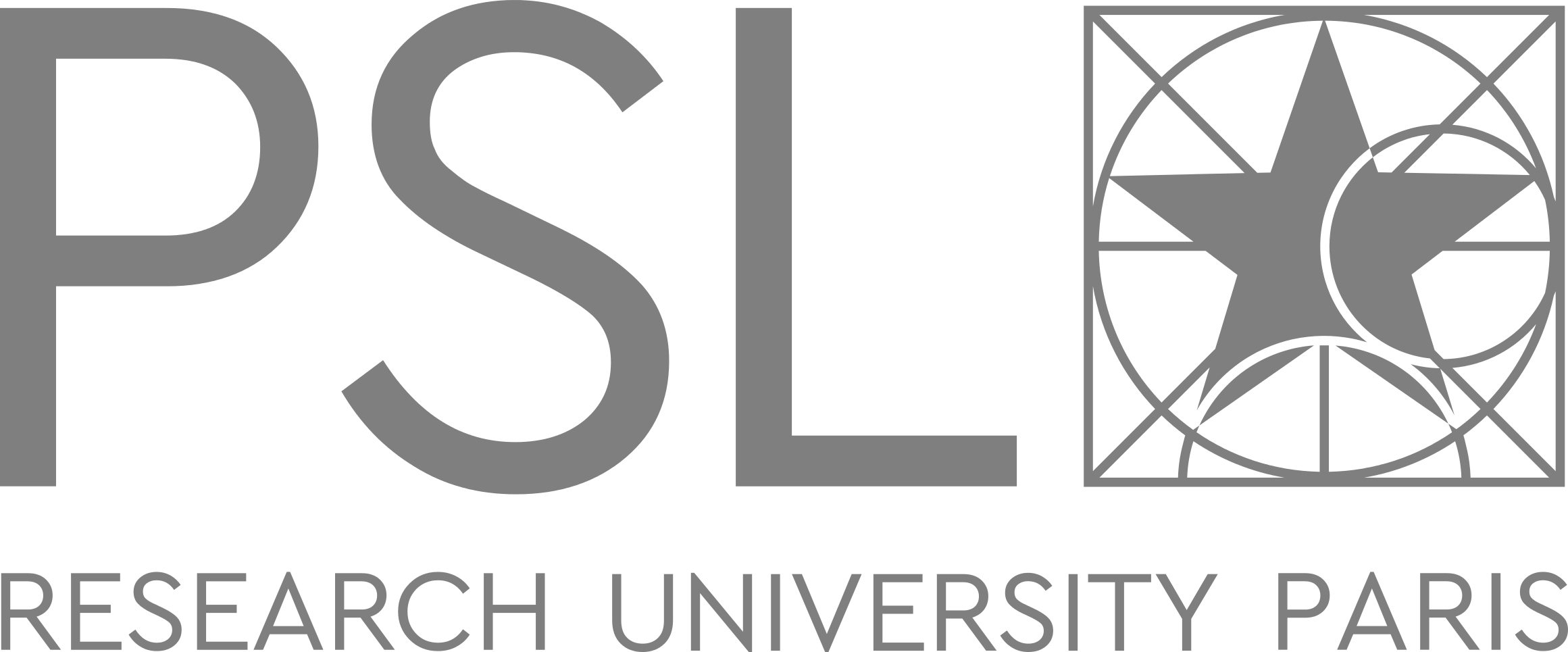
2018
Computational Bioimaging and Bioinformatics
Auguste Genovesio
Elise Laruelle
France Rose
Nikita Menezes
Tiphaine Champetier
Toni Paternina
Solène Weill
Mathieu Bahin
Amira Kramdi
Ouardia Ait Mohamed
Maxime Corbé
Guillaume Delevoye
Fatemeh Habibolahi
Benoit Noël
Delase Amesefe
Alice Othmani
Ayfer-Marie Montibus
Asm Shivuddin
Perrine Lacour
Sreetama Basu
Charles Bernard
Jennifer Salazar
Alexis Renault
Leila Bastianelli
Minhui Wu
Elton Rexhepaj
Quentin Viautour
Charles Wang
Yingbo Li







Supplementary slides

Projections, detections & signal

SMAX comparison

standardization with naïve flies
Detection on naive flies
Volumes from
groups
Automated fly filter
Naïve
Paired
Unpaired
AIR
OCT
AIR
AIR
OCT
AIR
MCH
AIR
MCH
AIR
Paired
control
Unpaired
control
OCT
OCT
OCT
OCT
OCT
MCH groups


3D detections paper
MB details
Frame 1
Frame 2
Frame 3
Frame 1
Frame 2
Frame 3
DBSCAN
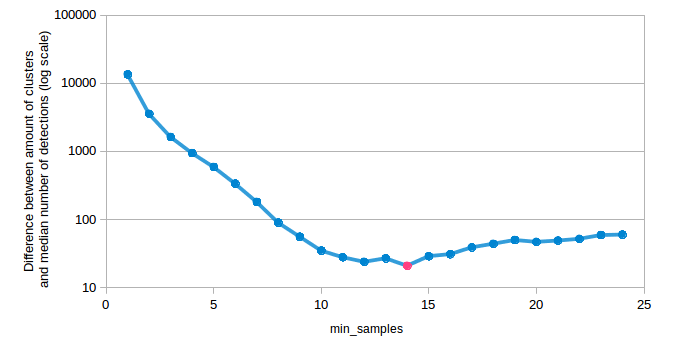
DBSCAN parameter scan
Detection removal without split

ICY Multiple hypothesis tracking



Variables
- Expected track length
- Minimum probability of existence for confirmation
- Probability of existence threshold
- Expected XY displacement
- Expected Z displacement
- Expected new objects per frame
- Expected number of objects in first frame
- Depth of track trees
- Gate factor for association

real
synthetic
Synthetic movie

Detection tracks with duplicates

Starting cluster stage...
Estimation of eps for DBSCAN:
Calculating distances between pairs: [65 & 178] [44 & 57] [254 & 2] [52 & 13] [88 & 110] [221 & 104] [163 & 138] [239 & 166] [234 & 133] [382 & 393] [381 & 247] [1 & 301] [335 & 102] [88 & 273] [83 & 11] [68 & 313] [321 & 58] [214 & 186] [351 & 350] [207 & 127] [232 & 394]
Done after 21 iterations, normal distribution with pvalue of 0.0341911133526
Global mean distance: 7.80852894987
Estimated eps value: 3.90426447493
Estimation of min_samples for DBSCAN:
Target: 726.615 clusters
6873 clusters for min_samples of 1 Difference from target: 6146.385
1946 clusters for min_samples of 2 Difference from target: 1219.385
892 clusters for min_samples of 3 Difference from target: 165.385
540 clusters for min_samples of 4 Difference from target: 186.615
419 clusters for min_samples of 5 Difference from target: 307.615
353 clusters for min_samples of 6 Difference from target: 373.615
278 clusters for min_samples of 7 Difference from target: 448.615
256 clusters for min_samples of 8 Difference from target: 470.615
227 clusters for min_samples of 9 Difference from target: 499.615
219 clusters for min_samples of 10 Difference from target: 507.615
215 clusters for min_samples of 11 Difference from target: 511.615
209 clusters for min_samples of 12 Difference from target: 517.615
216 clusters for min_samples of 13 Difference from target: 510.615
Best value for min_samples: 3
Initializing final DBSCAN...
eps: 3.90426447493
min_samples: 3
Number of clusters: 892
DBSCAN parameter estimation
Checking and fixing clusters...
Number of labels with duplicates: 70 (7%)
Resolving duplicates . . . . . .
62 cases with mode 0 (88%)
7 cases with mode 1 (10%)
0 cases with mode 2 (0%)
0 cases with mode 3 (0%)
0 cases with mode 4 (0%)
.
.
.
0 cases with mode 705 (0%)
0 cases with mode 706 (0%)
0 cases with mode 707 (0%)
1 cases with mode 708 (1%)
Remaining duplicates: 699
Number of labels with duplicates: 699 (43%)
Resolving duplicates . . . . . . . . . . . . . . . . . . . . . . . . . . . . . . . . . . . . . . . . . . . . . . . . . . . . . . . . . . . . . . .
197 cases with mode 0 (28%)
433 cases with mode 1 (61%)
69 cases with mode 2 (9%)
Remaining duplicates: 126
Number of labels with duplicates: 126 (7%)
Resolving duplicates . . . . . . . . . . .
49 cases with mode 0 (38%)
77 cases with mode 1 (61%)
Remaining duplicates: 0
Iterative cluster fix
CPD parameters



Chained registration

Axial drift through time
Axial drift


Rigid Vs Non-rigid registration
Only affine
Affine + CPD
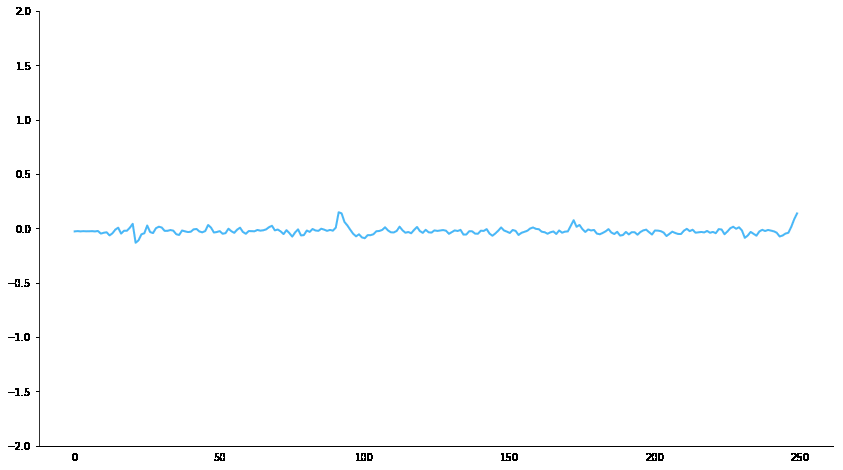

Signal filtering
Responsive neuron
Baseline neuron


3D + Time
Center crop





Voronoi maximum size
(relative to nuclei diameter)
x1
x2
x5
x10
no limit


Similarity matrix
Similarity matrix


Axial precision & Interpolation


sigma detections
0 0.000000 61006
1 7.507692 25
2 12.061538 24
3 14.400000 24
4 15.261538 24
5 15.384615 24
6 15.507692 24
7 16.369231 26
8 18.707692 23
9 23.261538 28
10 30.769231 11
11 41.969231 1
Sigma and object size



nuclei
signal
detection
z

intensity 2d histogram
nuclei
signal
Signal colocalization
Signal quality

Signal (GCaMP)
Signal quality

Signal quality

Signal quality

Signal quality


Data managment

Raster image registration

Raster image registration


PSF distortion
Synthetic images

Synthetic images

Synthetic images

Synthetic images

Synthetic images

Synthetic images

Synthetic images

Synthetic images

Synthetic images

Voxel dimensions
Copy of PhD defense
By Felipe Delestro
Copy of PhD defense
- 520