Volumetric Data Analysis and Visualization with qim3d
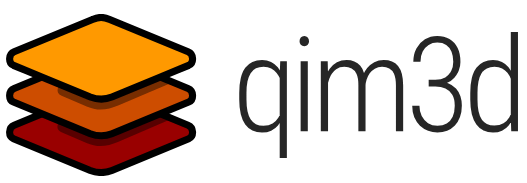
Felipe Delestro
Senior Research Software Specialist @ QIM Center
Danmarks Tekniske Universitet (DTU)
fima@dtu.dk
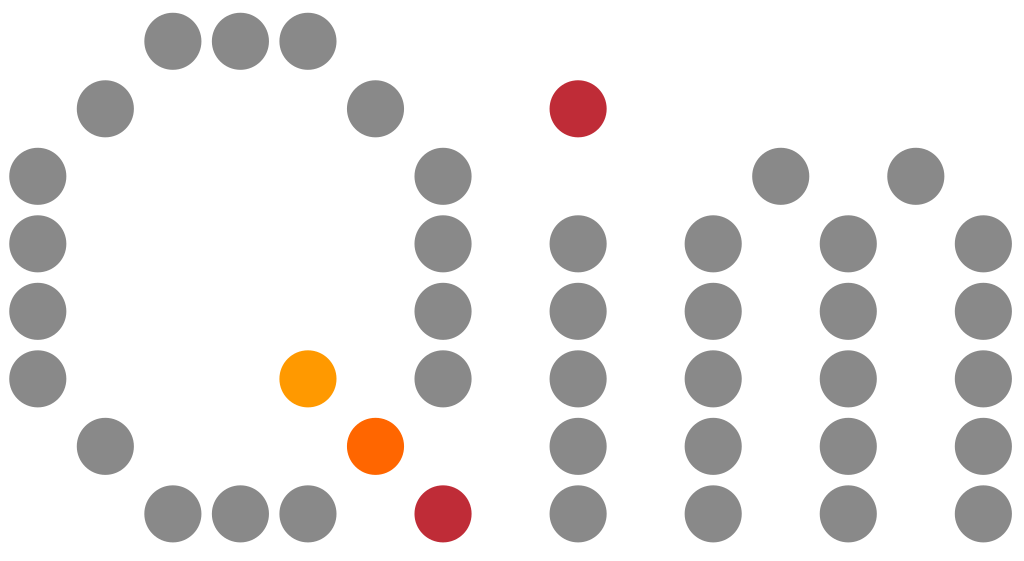
QIM Center
Center for Quantification of Imaging Data from MAX IV
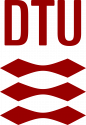
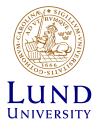
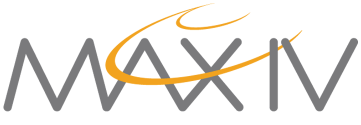
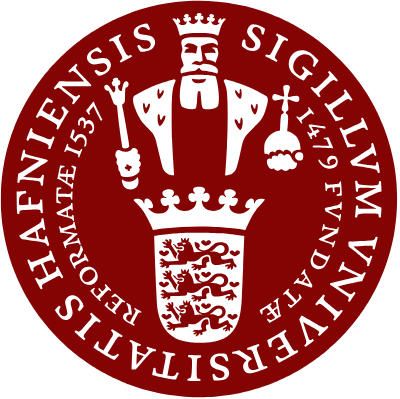
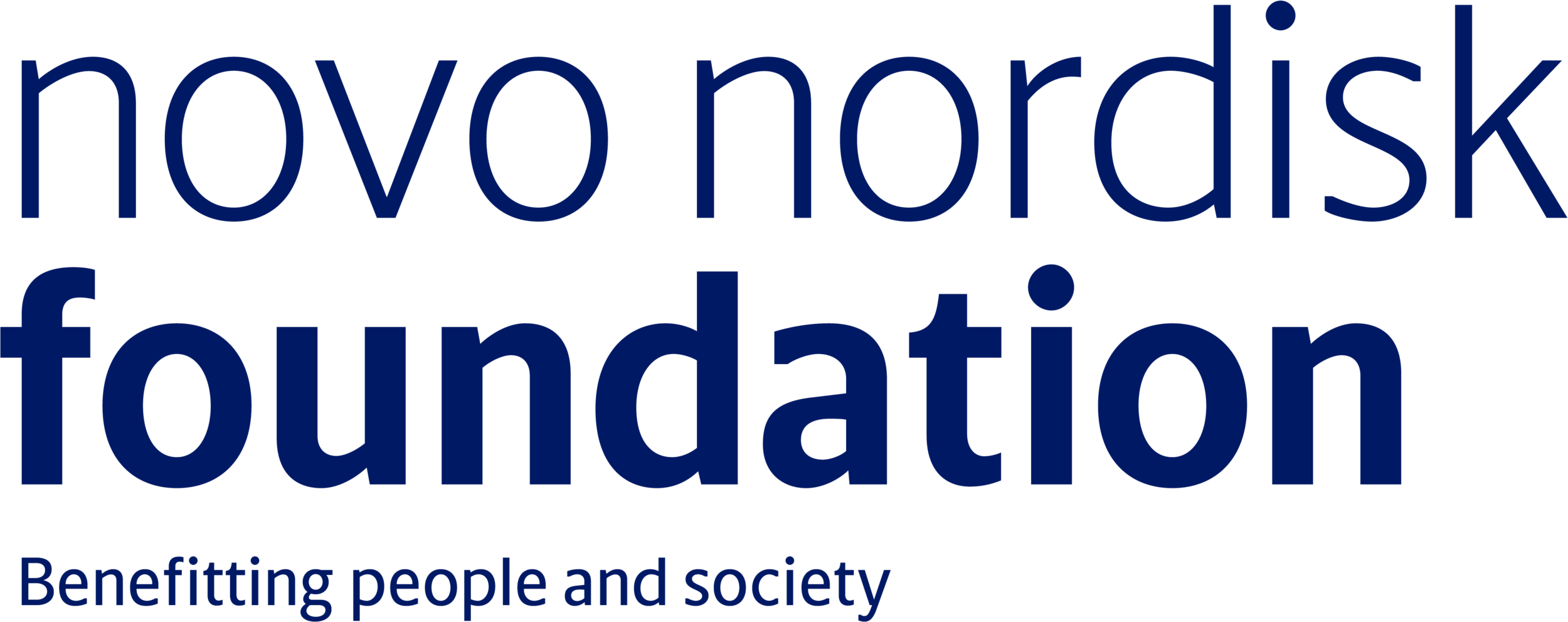
supported by
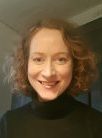
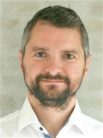
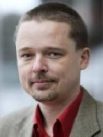
Rebecca
Engberg
Center Manager
Anders Bjorholm Dahl
Head of the QIM Center
Jon
Sporring
Deputy Head of the QIM Center
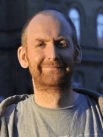
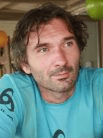
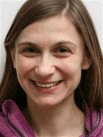
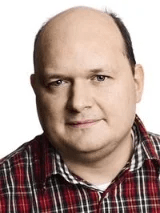
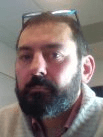
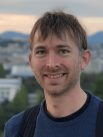
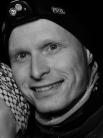
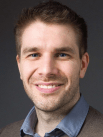
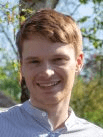
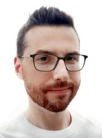
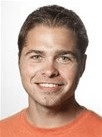
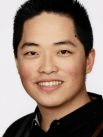
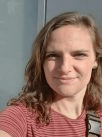
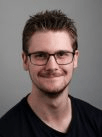
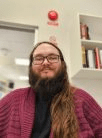
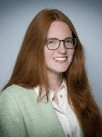
Volumetric data
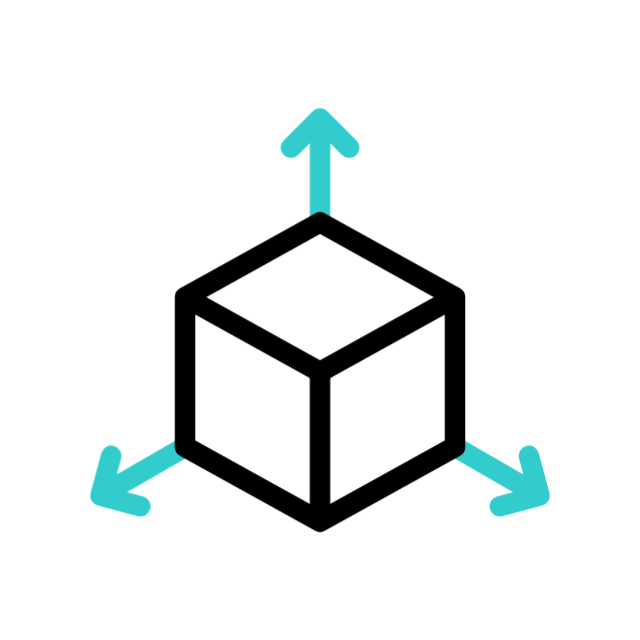
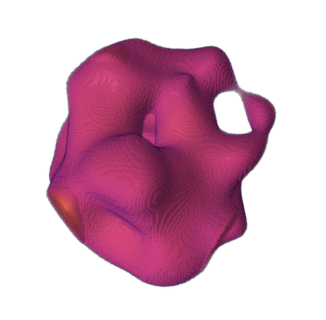
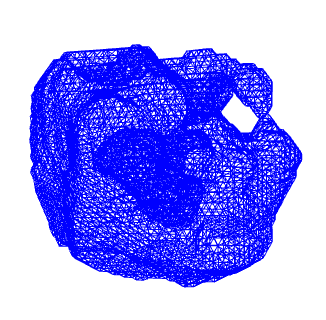
≠
volumetric rendering
data is composed of voxels (3D pixels)
mesh rendering
data is made of vertices and faces
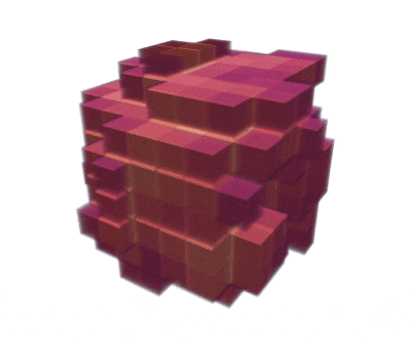
10x10x10
voxels
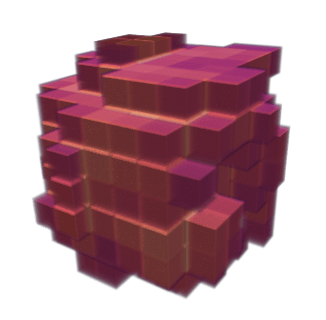
Z slices
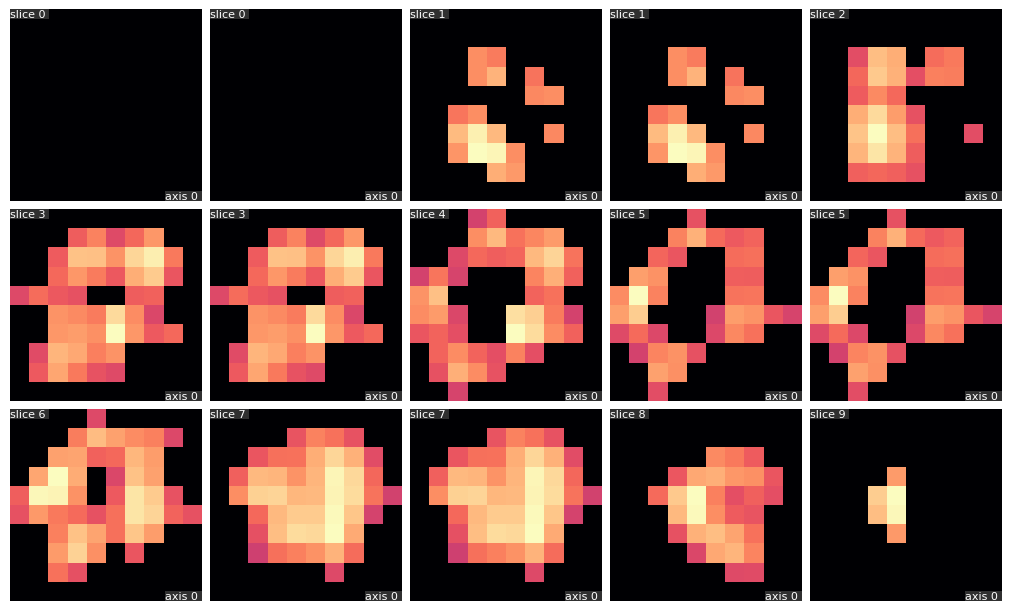
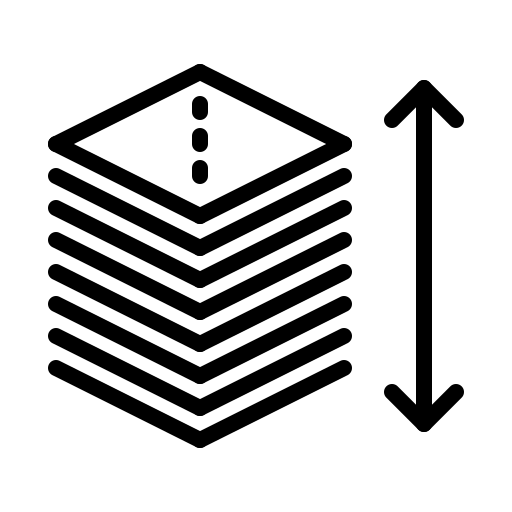
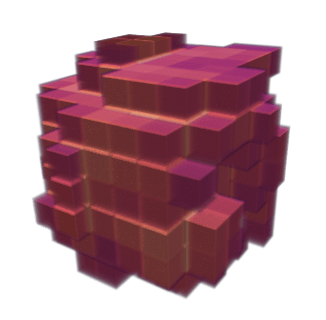
X slices
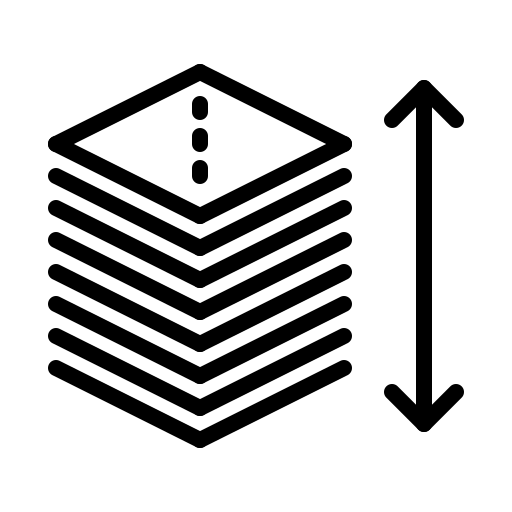
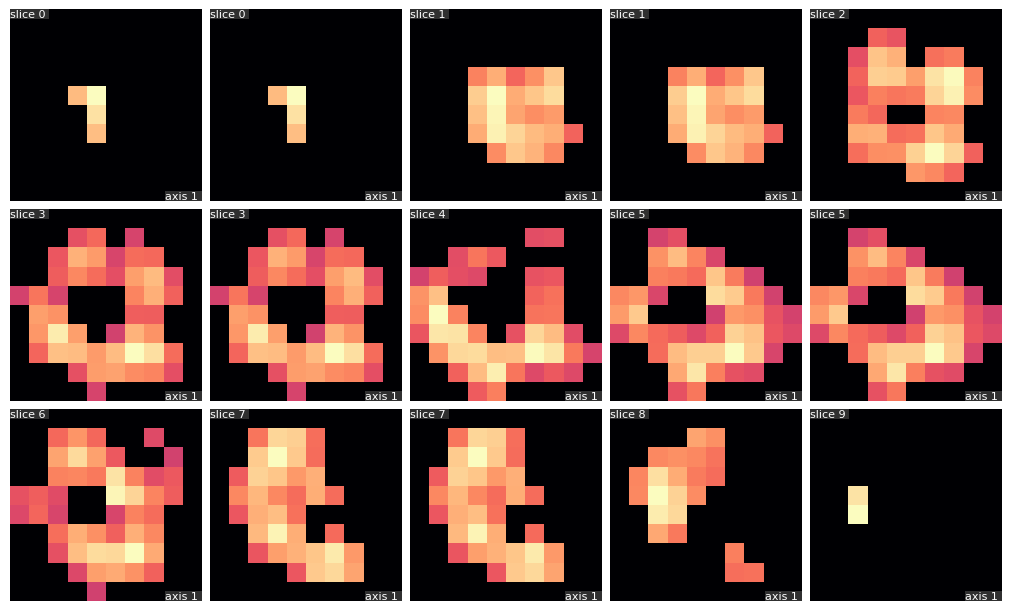
Image analys is different between 2D
and 3D
images
- Operations should be performed in 3D.
- Most tutorials and examples focus on 2D images.
- Dataset size could pose a challenge.
- Data visualization is more complex.
open-source Python library, that focuses on 3D volumetric data
Getting started is easy:
pip install qim3d
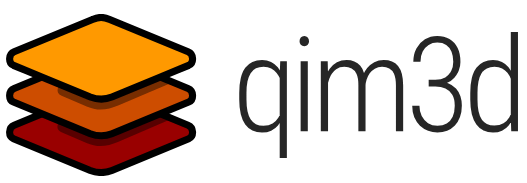
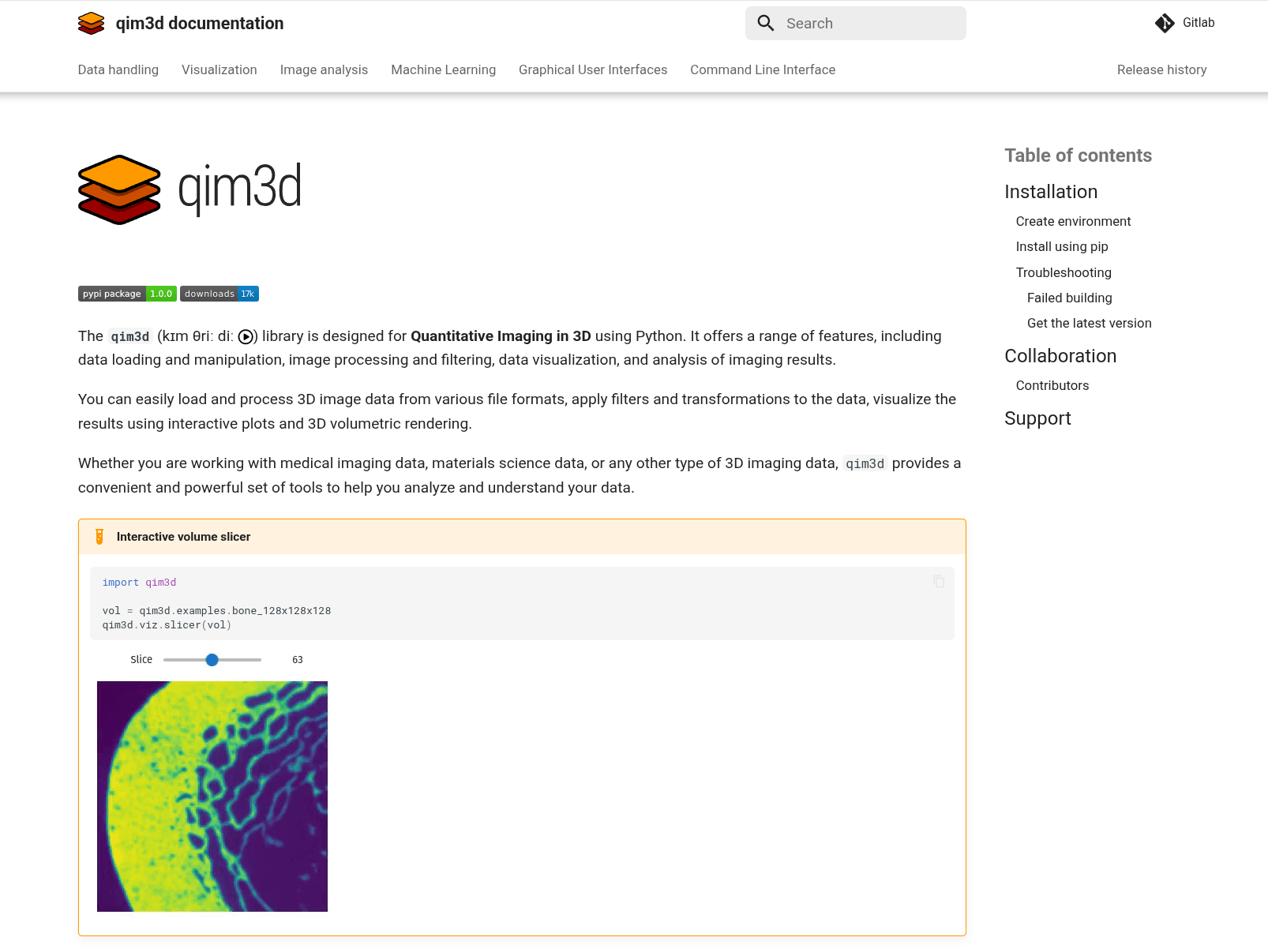
Documentation at platform.qim.dk/qim3d/
Data handling
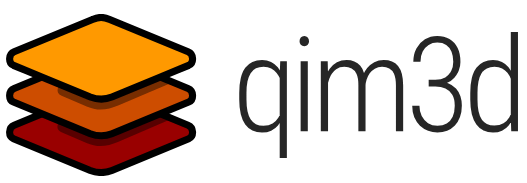
Volumetric data can be stored in a variety of file formats.
This can make the very first step of importing the data more complex than it should be
load and save
Data handling
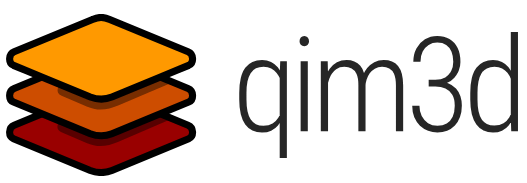
Data handling
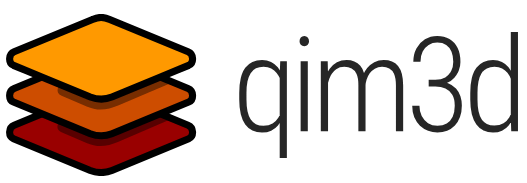
Volumetric data can be stored in a variety of file formats.
This can make the very first step of importing the data more complex than it should be
load and save
qim3d.io.load
and qim3d.io.save
can handle the most common data formats:
-
Tiff
(including file stacks) HDF5
-
TXRM
/TXM
/XRM
NIfTI
-
PIL
(including file stacks) -
VOL
/VGI
DICOM
import qim3d
vol = qim3d.io.load("path/to/image.tif", virtual_stack=True)
Data handling
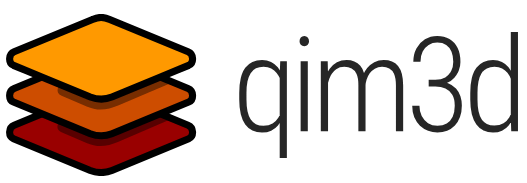
We make a distinction between load/save
and import/export
when the operation includes transformations to the data.
import and export
Currently we're implementing a 3D import/export
following the OME-Zarr
standard, which
uses chunked data with a
ulti-scales scheme.
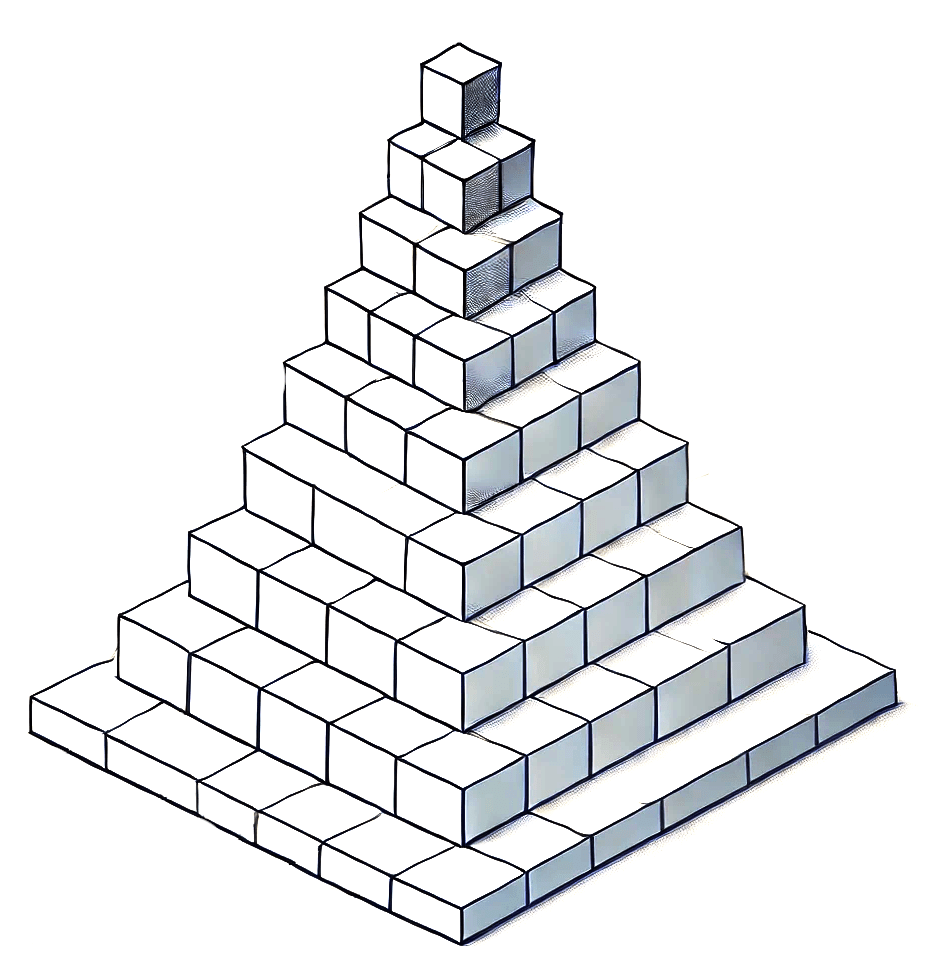
Data handling
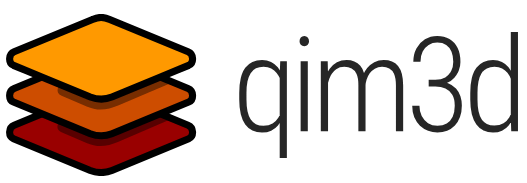
We have a repository of 3D volumetric data that can be freely used, and with qim3d
they can be easily downloaded directly within python code
Downloader
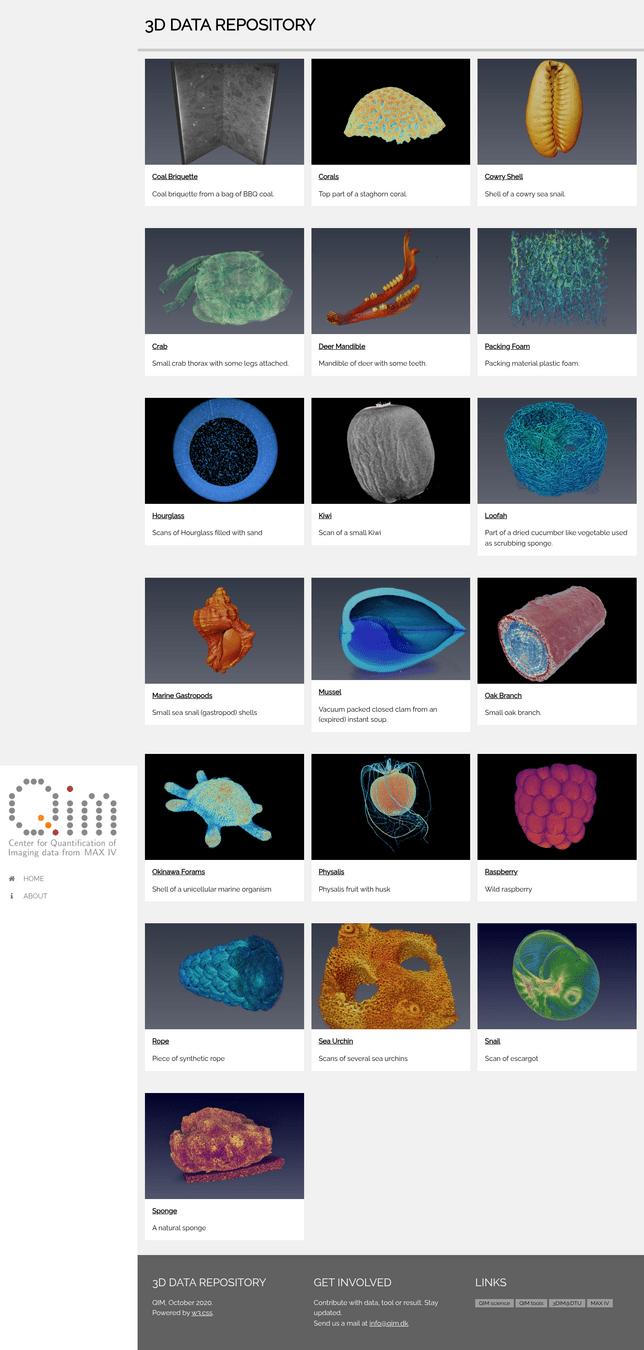
import qim3d
downloader = qim3d.io.Downloader()
data = downloader.Cowry_Shell.Cowry_DOWNSAMPLED(load_file=True)
Data handling
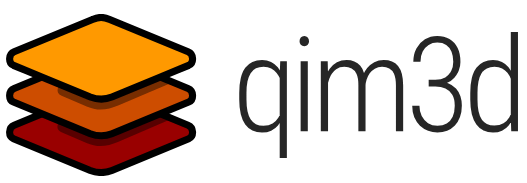
Generating synthetic volumetric data can be very helpful for method development, benchmarking or tutorials
Synthetic data
The function qim3d.generate.volume
uses Perlin noise to generate a variety of complex volumes
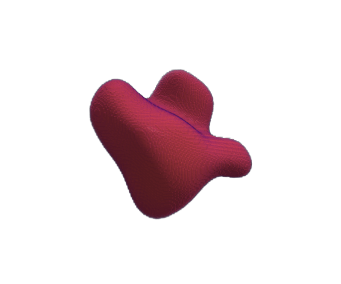
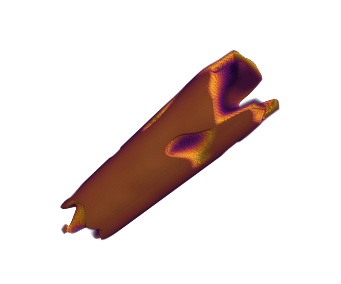
vol = qim3d.generate.volume(noise_scale = 0.015)
vol = qim3d.generate.volume(base_shape = (200, 100, 100),
final_shape = (400, 100, 100),
noise_scale = 0.03,
gamma = 0.12,
threshold = 0.85,
object_shape = "tube"
)
Data handling
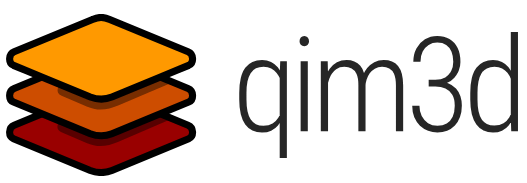
When using qim3d.generate.volume_collection
we can generate groups of objects within the same volume, and also have their individual labels
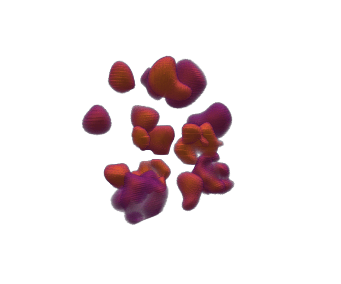
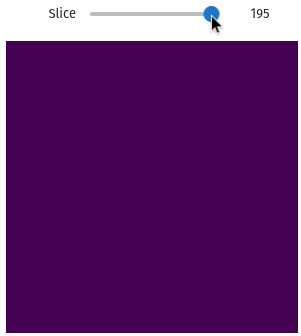
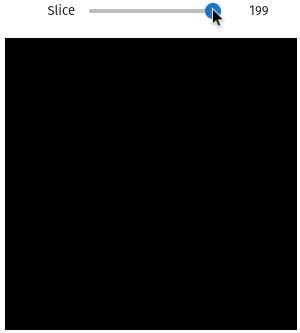
import qim3d
# Generate synthetic collection of objects
num_objects = 15
vol, labels = qim3d.generate.volume_collection(num_objects = num_objects)
vol
labels
Data handling
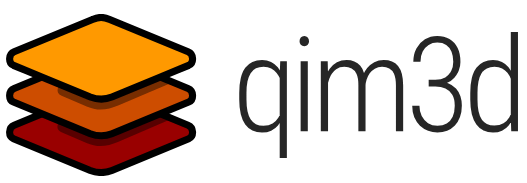
vol, labels = qim3d.generate.volume_collection(num_objects = 40,
collection_shape = (300, 150, 150),
min_shape = (280, 10, 10),
max_shape = (290, 15, 15),
min_object_noise = 0.08,
max_object_noise = 0.09,
max_rotation_degrees = 5,
min_threshold = 0.7,
max_threshold = 0.9,
min_gamma = 0.10,
max_gamma = 0.11,
object_shape = "cylinder"
)
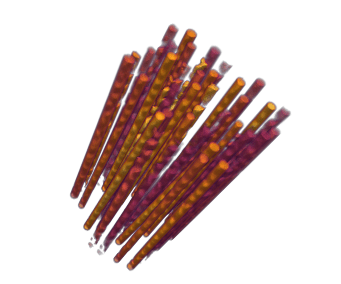
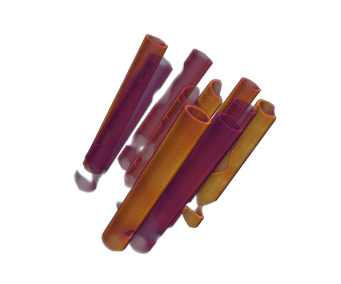
vol, labels = qim3d.generate.volume_collection(num_objects = 10,
collection_shape = (200, 200, 200),
min_shape = (180, 25, 25),
max_shape = (190, 35, 35),
min_object_noise = 0.02,
max_object_noise = 0.03,
max_rotation_degrees = 5,
min_threshold = 0.7,
max_threshold = 0.9,
min_gamma = 0.10,
max_gamma = 0.11,
object_shape = "tube"
)
Visualization
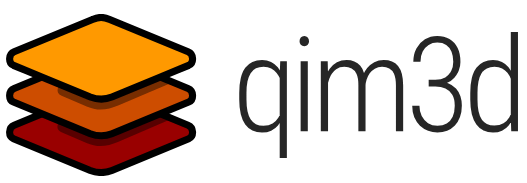
Visualization
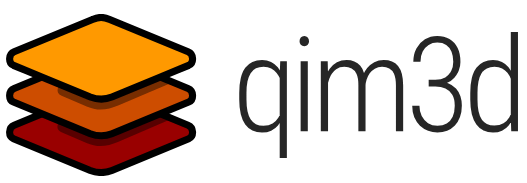
Easily exploring volumetric data may not be so straightforward. The library provides helper functions this task simple and easy, even within Jupyter Notebooks
import qim3d
vol = qim3d.examples.bone_128x128x128
qim3d.viz.slicer(vol)
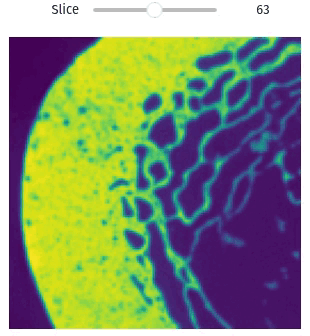
Visualization
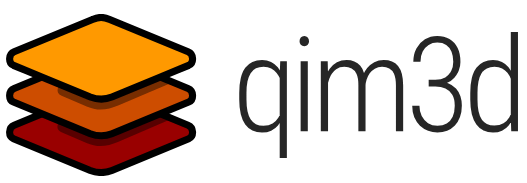
import qim3d
vol = qim3d.examples.shell_225x128x128
qim3d.viz.slices_grid(vol, num_slices=15)
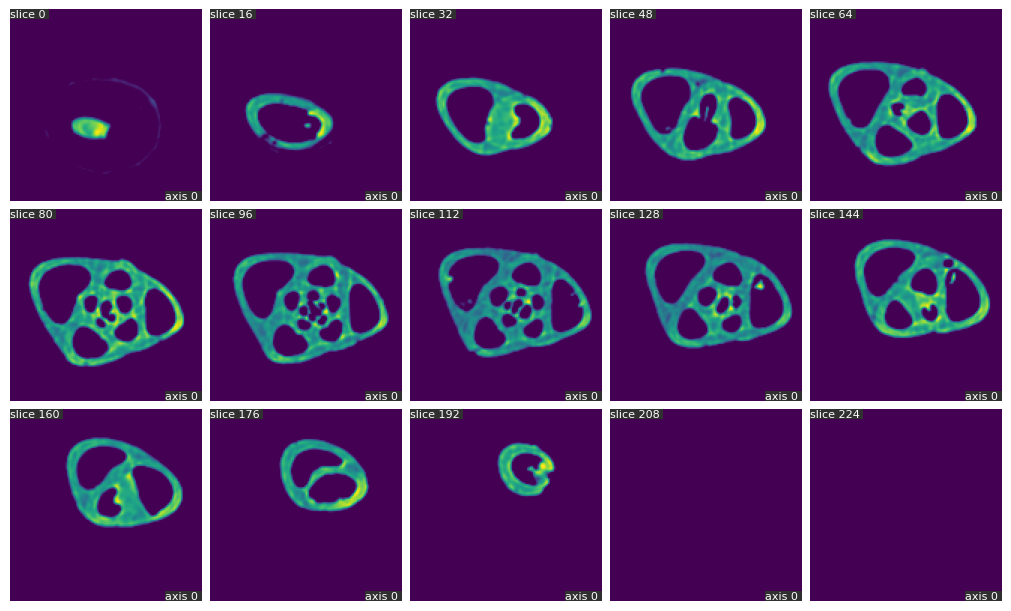
Visualization
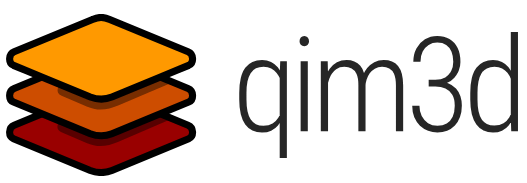
import qim3d
vol = qim3d.examples.cement_128x128x128
binary = qim3d.filters.gaussian(vol, sigma = 2) < 60
labeled_volume, num_labels = qim3d.segmentation.watershed(binary)
color_map = qim3d.viz.colormaps.segmentation(num_labels, style = 'bright')
qim3d.viz.slicer(labeled_volume, slice_axis = 1, color_map=color_map)
qim3d.viz.colormaps.segmentation
creates an colormap designes specifically for segmentation tasks
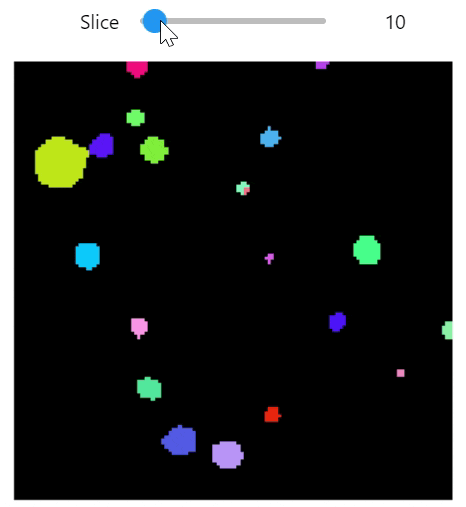

The parameter min_dist
allows us to control how different neighbour colors are
Visualization
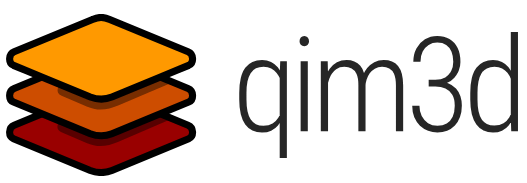
import qim3d
# Generate synthetic collection of objects
num_objects = 15
vol, labels = qim3d.generate.volume_collection(num_objects = num_objects)
# Visualize synthetic collection
qim3d.viz.volumetric(vol)
Visualization
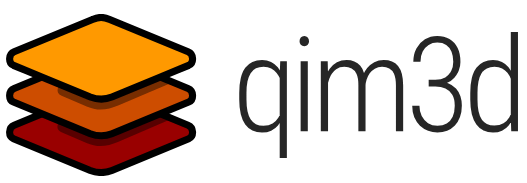
import qim3d
vol = qim3d.generate.volume(base_shape=(128,128,128),
final_shape=(128,128,128),
noise_scale=0.03,
order=1,
gamma=1,
max_value=255,
threshold=0.5,
dtype='uint8'
)
mesh = qim3d.mesh.from_volume(vol, step_size=3)
qim3d.viz.mesh(mesh.vertices, mesh.faces)
Visualization
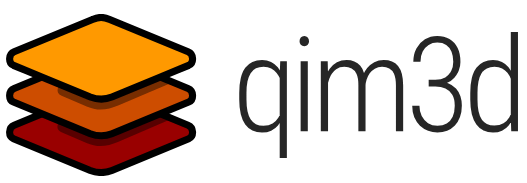
Visualization for OME-Zarr
datasets is more challenging, but very important for large volumes
The qim3d library integrates with the itk-vtk-viewer
, that can be launched locally from the command line
Visualization
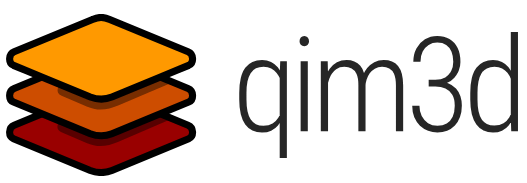
Exploring chunks from a OME-Zarr
dataset is also possible directly on a Jupyter notebook
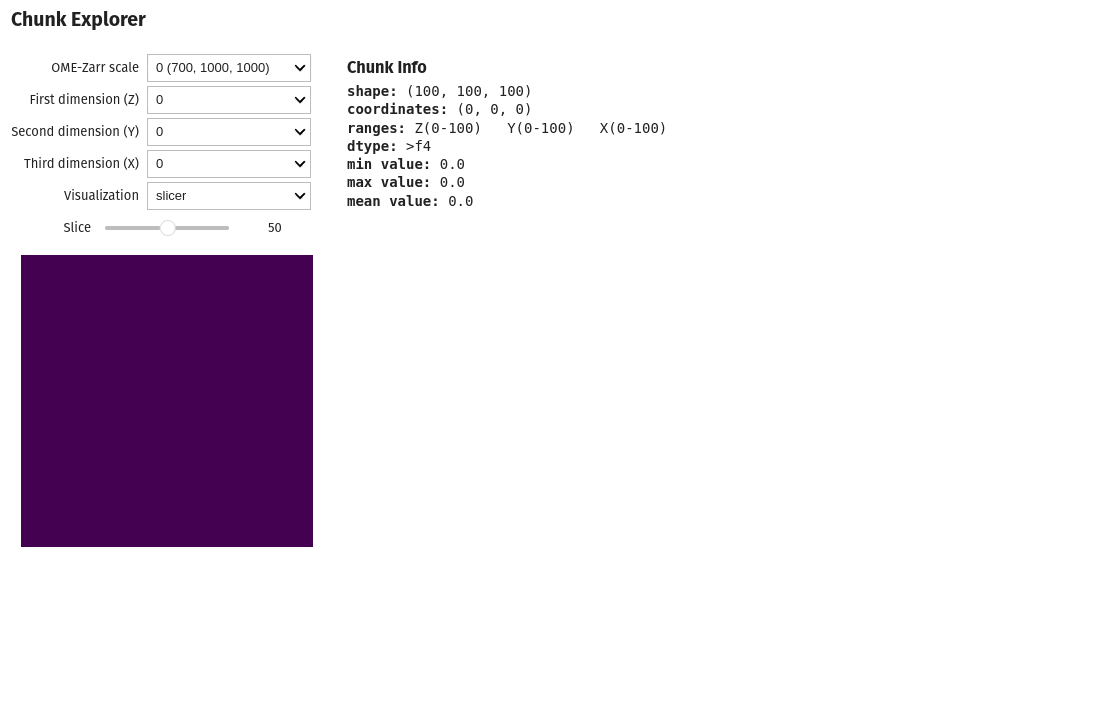
import qim3d
# Download dataset
downloader = qim3d.io.Downloader()
data = downloader.Snail.Escargot(load_file=True)
# Export as OME-Zarr
qim3d.io.export_ome_zarr("Escargot.zarr", data, chunk_size=100, downsample_rate=2, replace=True)
# Explore chunks
qim3d.viz.chunks("Escargot.zarr")
Image analysis
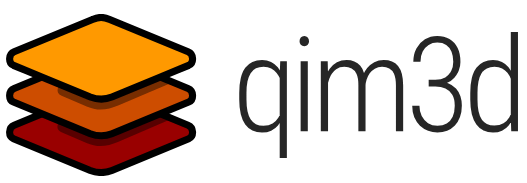
Image analysis
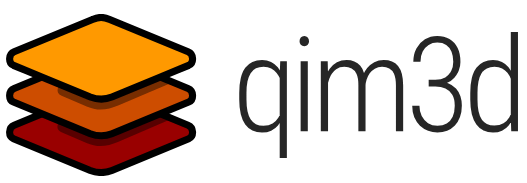
The goal of qim3d
is to cover form basic functionalities that can serve as building blocks for more complex pipelines, up to implementation or wrappers for more complex methods
This will allow the library to be a central point for building pipelines for processing volumetric data
Image analysis
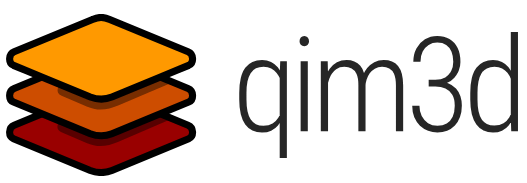
qim3d.filters
contains implementations for common filter operations, including the option to run them on chunked data using Dask
qim3d.filters.Pipeline
allows the creation of classes that act as filter pipelines
import qim3d
from qim3d.filters import Pipeline, Median, Gaussian, Maximum, Minimum
# Get data
vol = qim3d.examples.fly_150x256x256
# Show original
fig1 = qim3d.viz.slices_grid(vol, num_slices=5, display_figure=True)
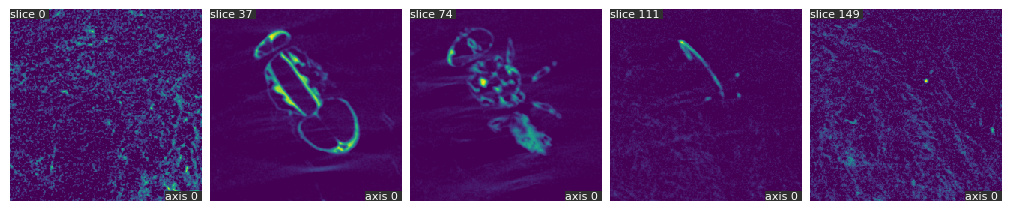
# Create filter pipeline
pipeline = Pipeline(
Median(size=5),
Gaussian(sigma=3, dask = True)
)
# Append a third filter to the pipeline
pipeline.append(Maximum(size=3))
# Apply filter pipeline
vol_filtered = pipeline(vol)
# Show filtered
fig2 = qim3d.viz.slices_grid(vol_filtered, num_slices=5, display_figure=True)
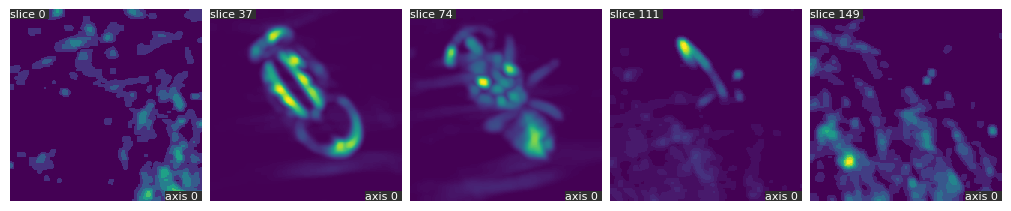
Image analysis
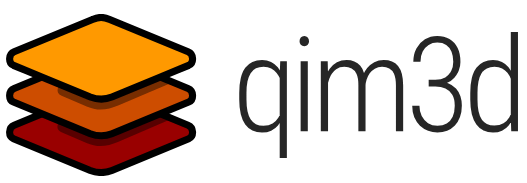
The modules qim3d.detection
and qim3d.segmentation
contain wrappers for common methods that are useful when building larger pipelines
import qim3d
# Get data
vol = qim3d.examples.cement_128x128x128
vol_blurred = qim3d.filters.gaussian(vol, sigma=2)
# Detect blobs, and get binary_volume
blobs, binary_volume = qim3d.detection.blobs(
vol_blurred,
min_sigma=1,
max_sigma=8,
threshold=0.001,
overlap=0.1,
background="bright"
)
# Visualize detected blobs
qim3d.viz.circles(blobs, vol, alpha=0.8, color='blue')
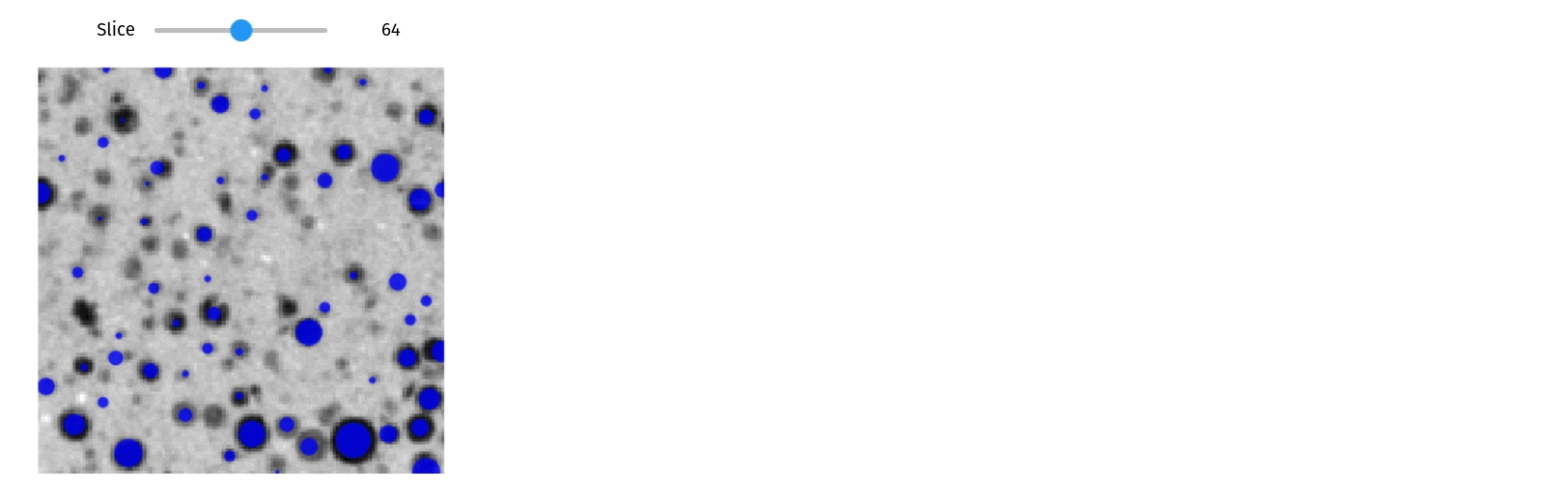
Image analysis
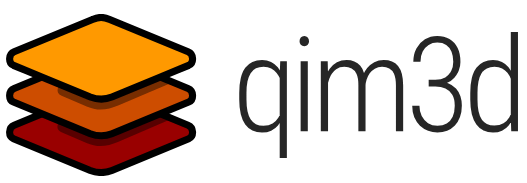
The modules qim3d.detection
and qim3d.segmentation
contain wrappers for common methods that are useful when building larger pipelines
import qim3d
vol = qim3d.examples.cement_128x128x128
bin_vol = qim3d.filters.gaussian(vol, sigma = 2)<60
fig1 = qim3d.viz.slices_grid(bin_vol, slice_axis=1, display_figure=True)
labeled_volume, num_labels = qim3d.segmentation.watershed(bin_vol)
cmap = qim3d.viz.colormaps.segmentation(num_labels)
fig2 = qim3d.viz.slices_grid(labeled_volume, slice_axis=1, color_map=cmap, display_figure=True)
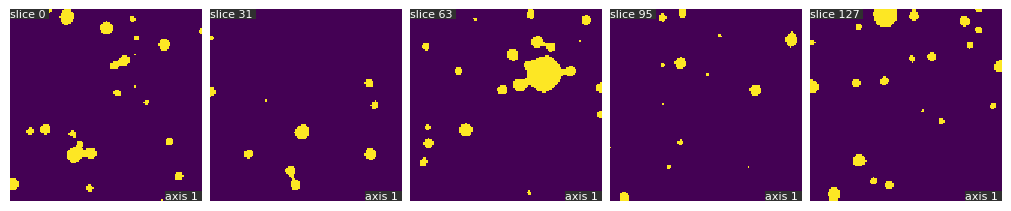
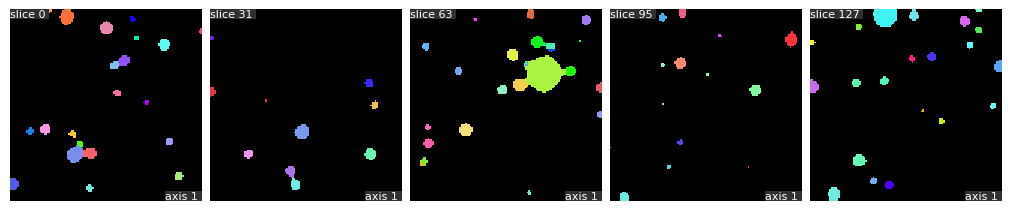
Image analysis
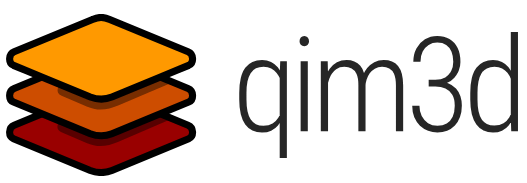
qim3d.operations
gathers image analysis operations that perform more specific tasks. For example, here we use fade_mask
to remove artifacts form the edge of a dataset
import qim3d
vol = qim3d.io.load('heartScan.tif')
qim3d.viz.volumetric(vol)
vol_faded = qim3d.operations.fade_mask(vol, decay_rate=4, ratio=0.45, geometric='cylindrical')
qim3d.viz.volumetrics(vol_faded)
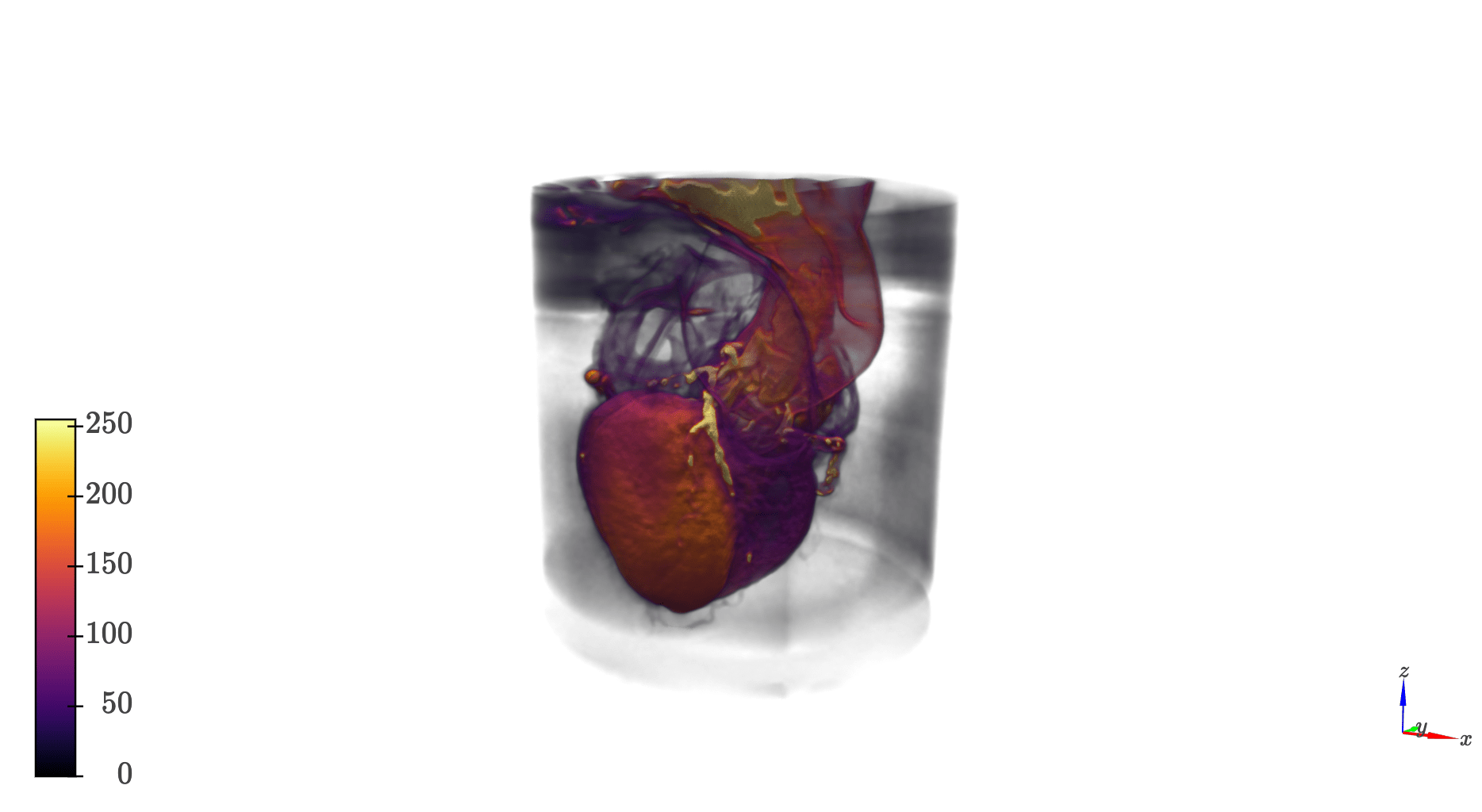
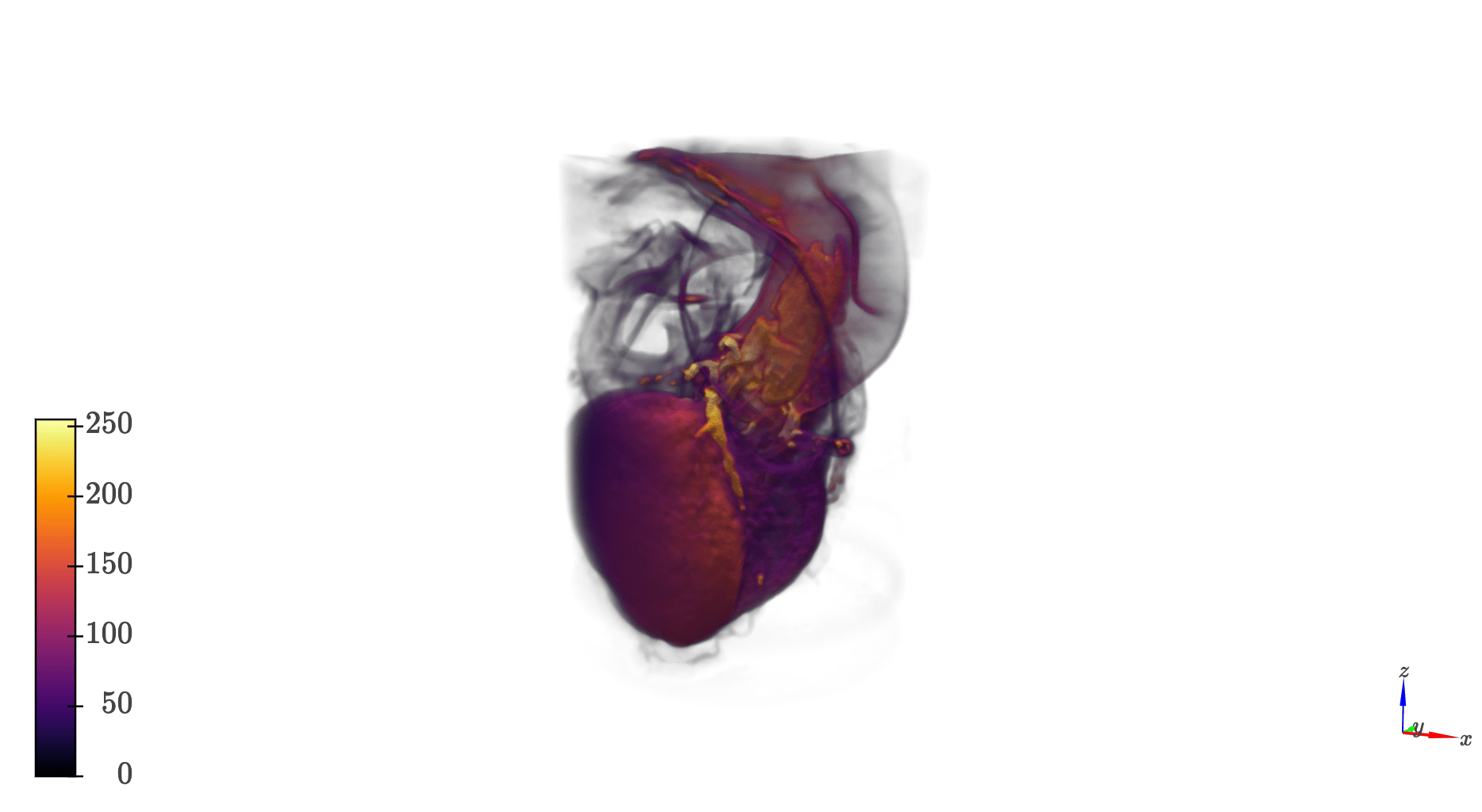
Image analysis
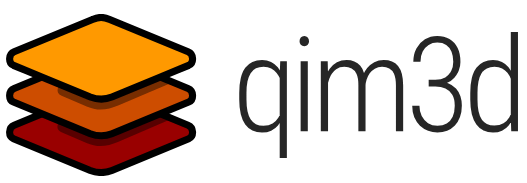
qim3d.processing
is meant for more complex methods, that perform quantification tasks that are specific but common for volumetric data
The current implementations are:
- qim3d.processing.structure_tensor
- qim3d.processing.local_thickness
Image analysis
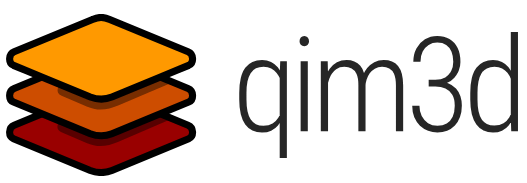
Structure tensor
import qim3d
vol = qim3d.examples.NT_128x128x128
val, vec = qim3d.processing.structure_tensor(vol, visualize=True, axis=2)
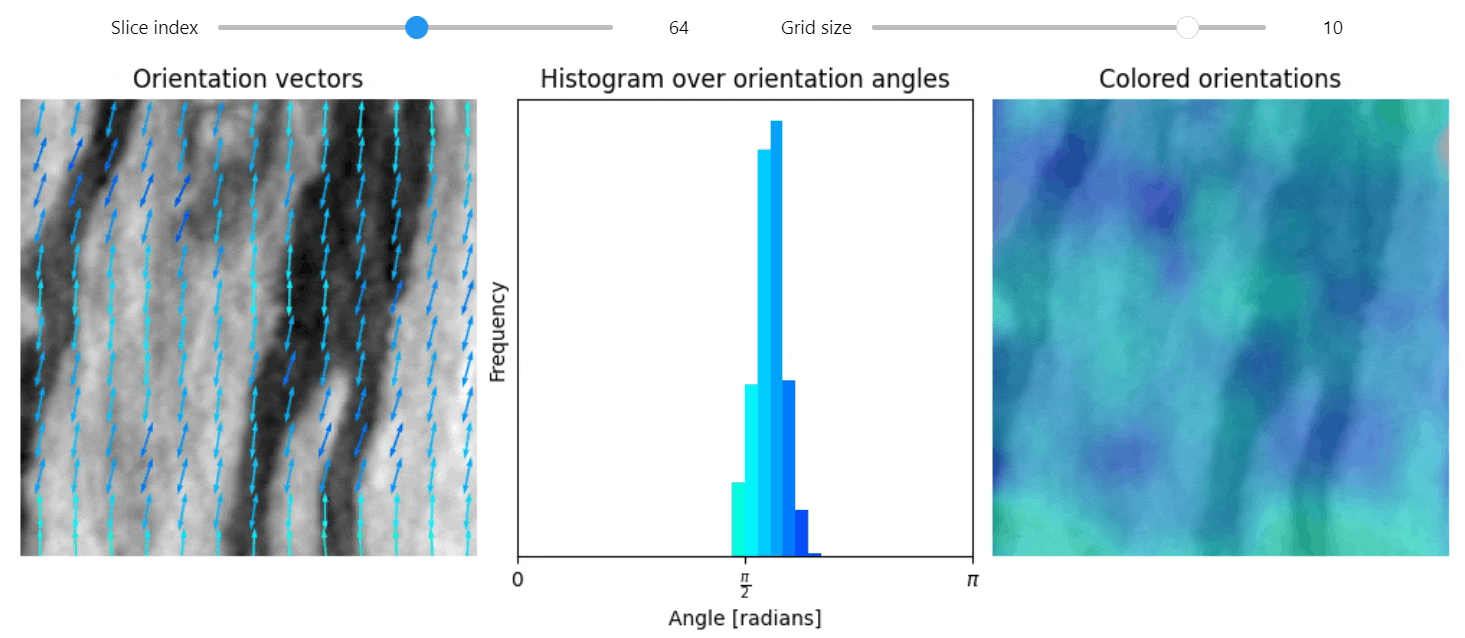
Image analysis
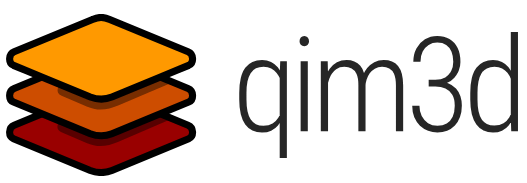
Local thickness
import qim3d
vol = qim3d.examples.fly_150x256x256
lt_vol = qim3d.processing.local_thickness(vol, visualize=True, axis=0)
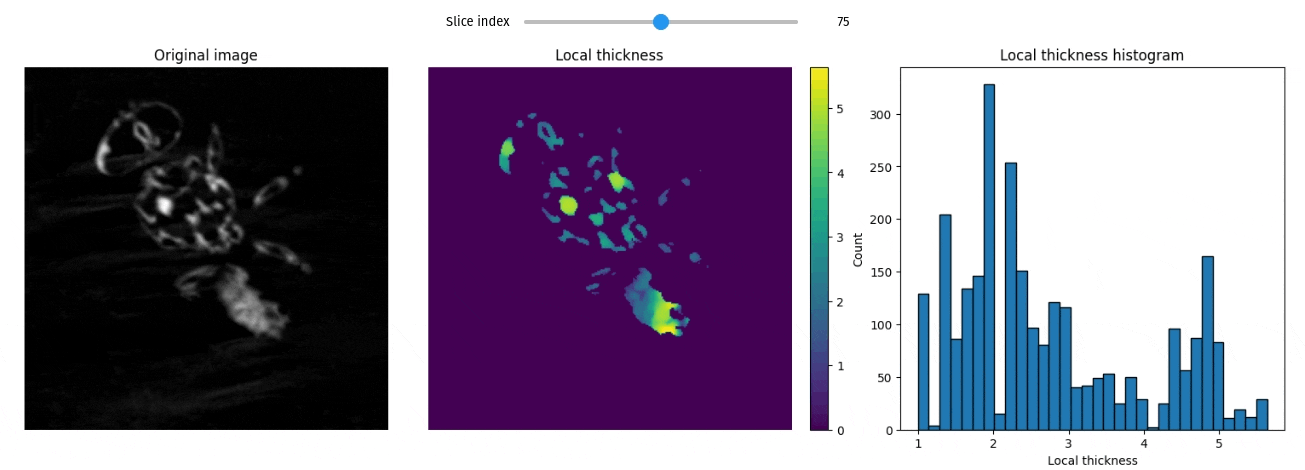
Machine learning
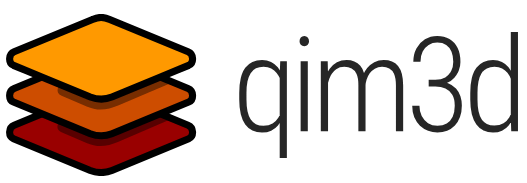
Machine learning
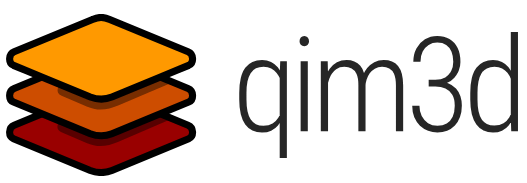
This module is still in the early stages of development, but our goal is to have a set of tools that can streamline the implementation of ML & DL pipelines for volumetric data
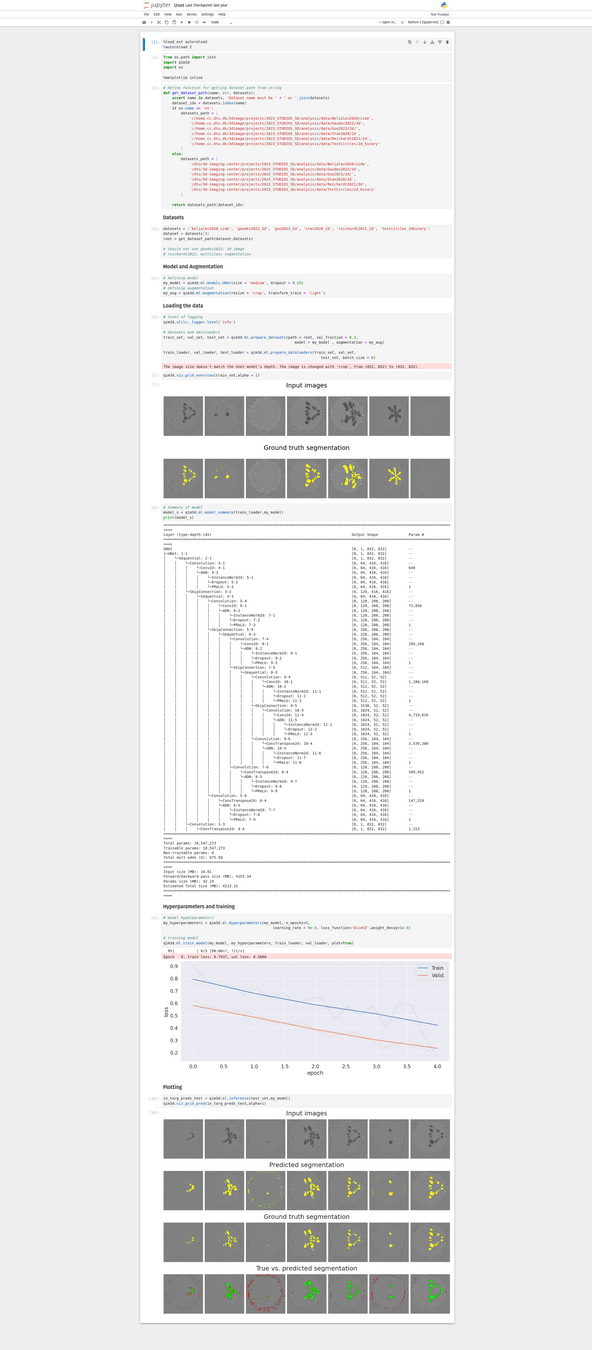
For example, currently, there are a few helper functions for setting DL archtechtures
# Make an Unet
my_model = qim3d.ml.models.UNet(size = 'medium', dropout = 0.25)
# Set augmentations
my_aug = qim3d.ml.Augmentation(resize = 'crop', transform_train = 'light')
# Set hyperparameters
my_hyperparameters = qim3d.ml.Hyperparameters(my_model, n_epochs=5, learning_rate = 5e-3,
loss_function='DiceCE',weight_decay=1e-3)
# training model
qim3d.ml.train_model(my_model, my_hyperparameters, train_loader, val_loader, plot=True)
Command line interface
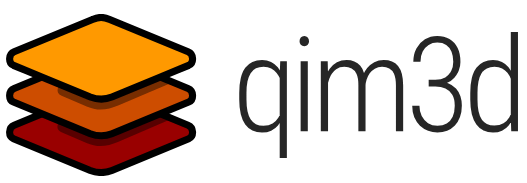
Command line interface
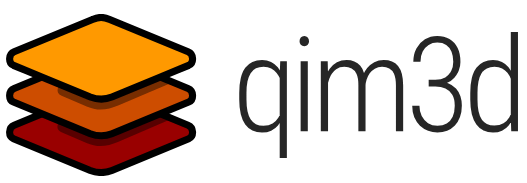
fima@pop-os ~ $ qim3d viz Okinawa_Foram_1.zarr/
itk-vtk-viewer
=> Serving /home/fima/Notebooks/Qim3d on port 3000
enp0s31f6 => http://10.52.0.158:3000/
wlp0s20f3 => http://10.197.104.229:3000/
Serving directory '/home/fima/Notebooks/Qim3d'
http://localhost:8042/
Visualization url:
http://localhost:3000/?rotate=false&fileToLoad=http://localhost:8042/Okinawa_Foram_1.zarr
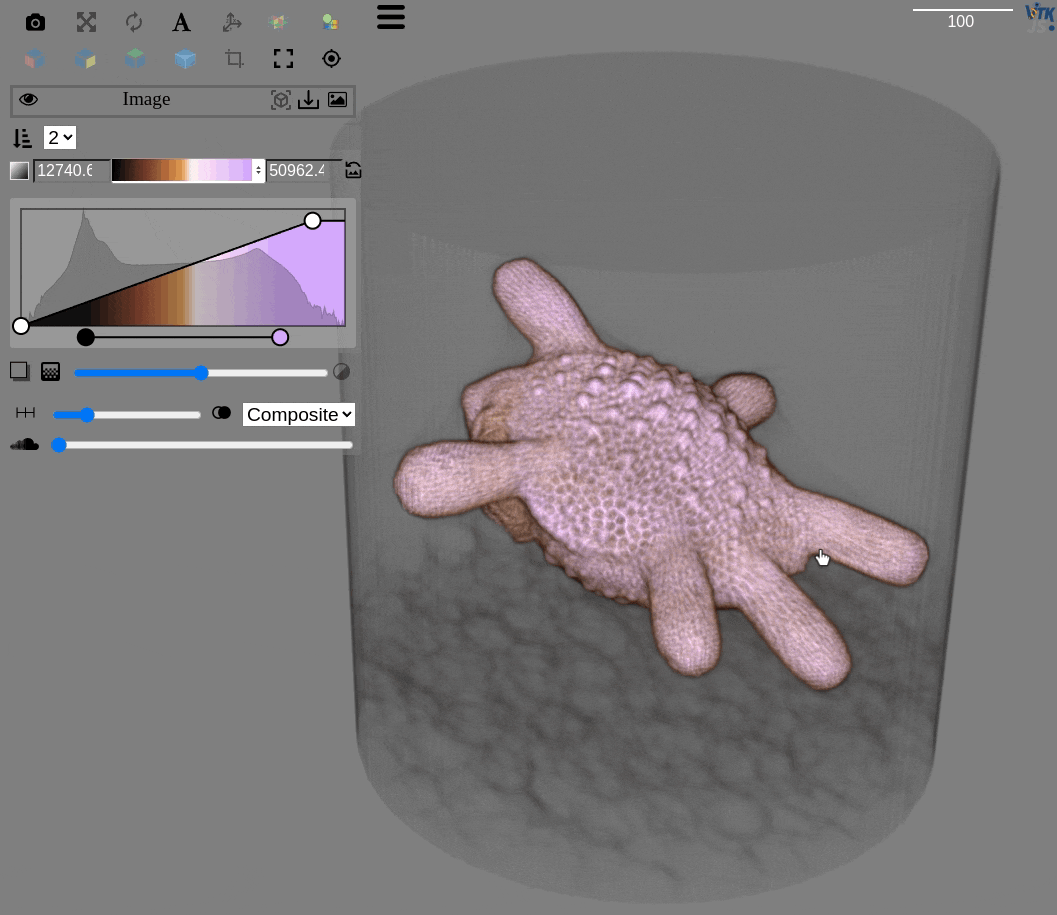
The library is an open-source project, and you're all welcome to contribute!
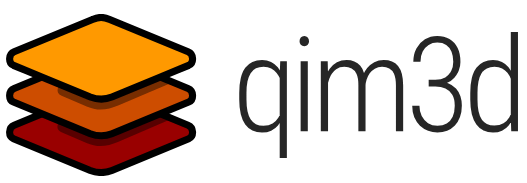
documentation and
installation instructions:
Large datasets
and the
OME-Zarr
standard
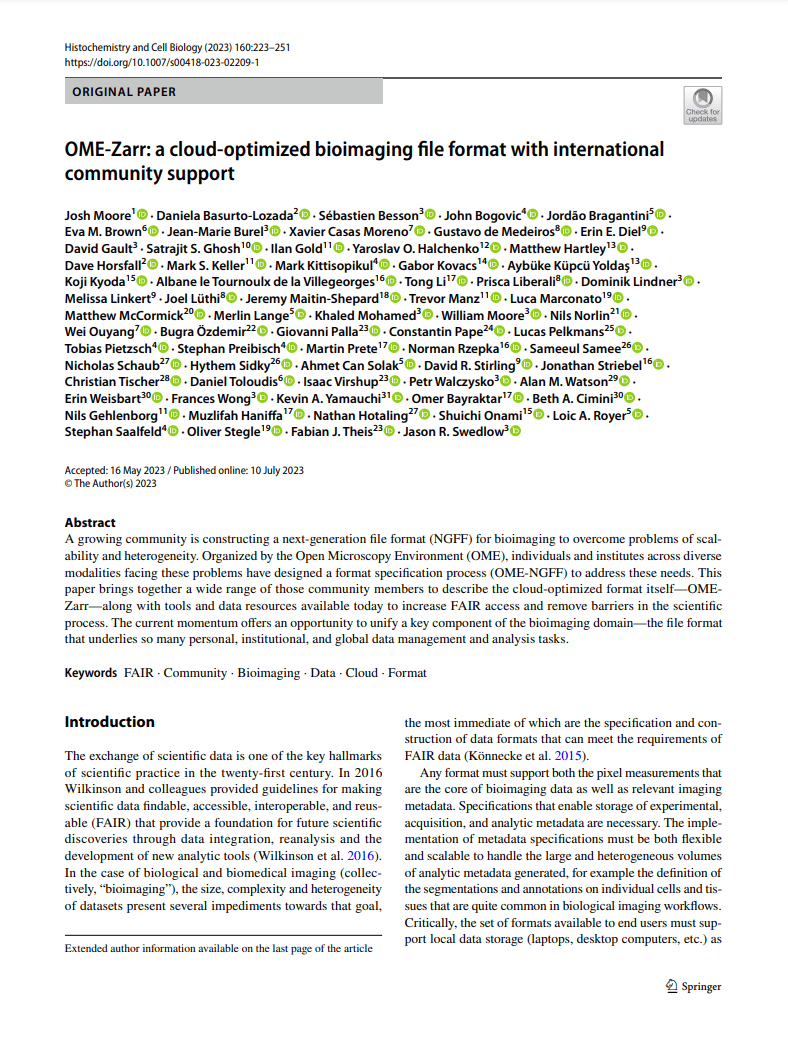
16Gb
a commom amount of memory for a computer
512Gb
memory of a good HPC node
1000Gb (1Tb)
Data size that we're supposed to handle
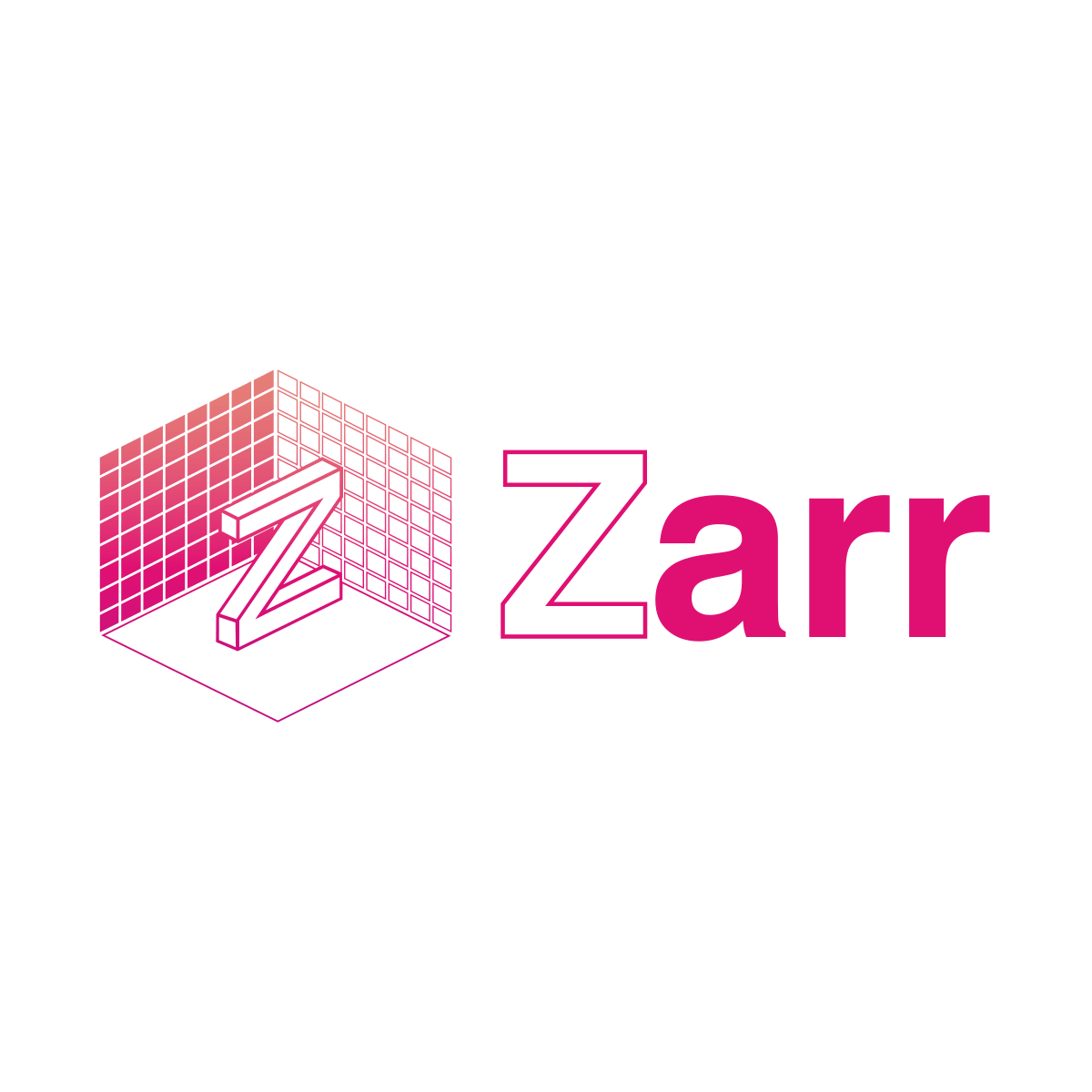
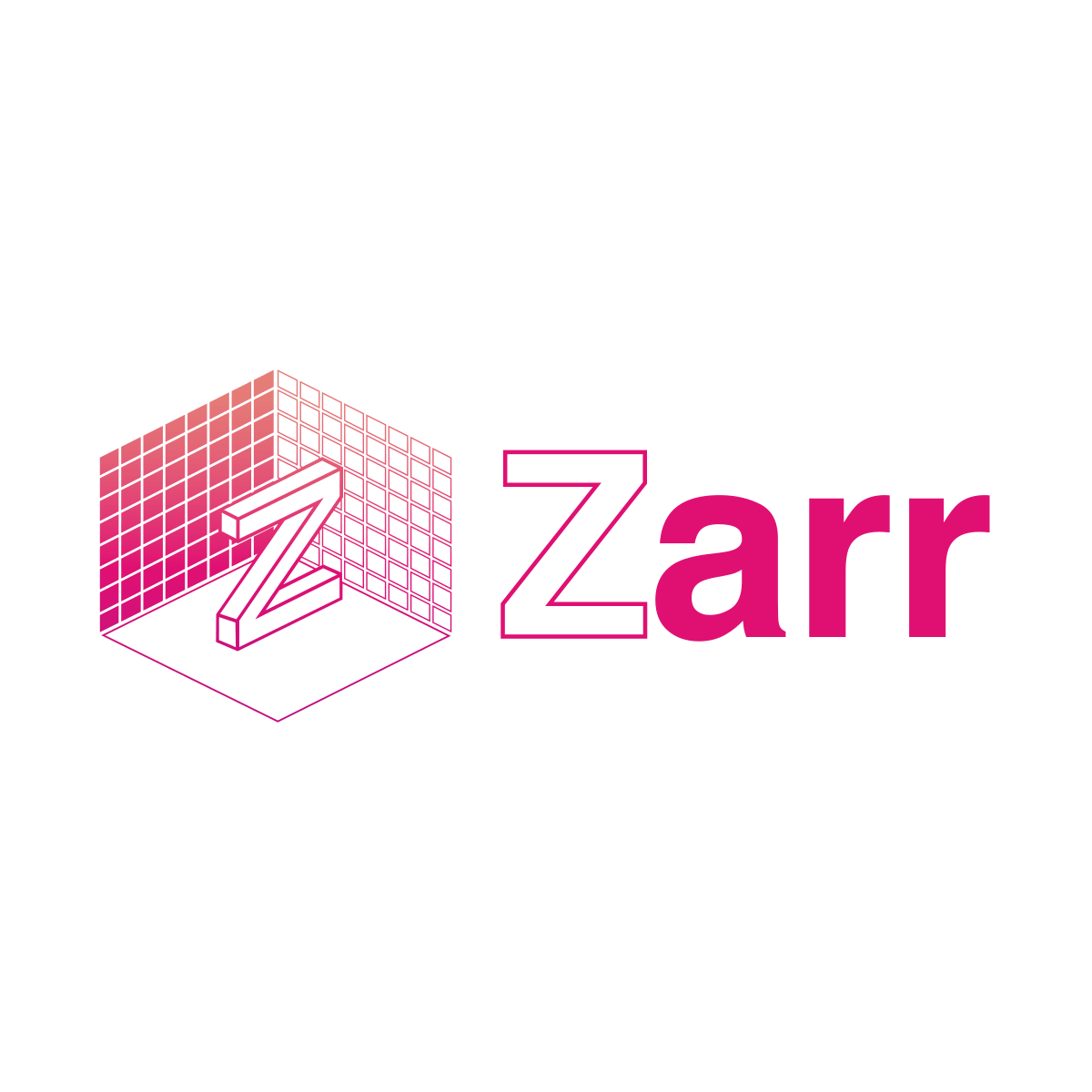
-
Chunked storage: Data is divided into chunks, allowing efficient access and processing of subsets.
-
Compression support: Chunks can be compressed using various algorithms (e.g., Blosc, Zstd, Gzip) to reduce storage requirements.
-
Scalability: Works well with large datasets, enabling parallel I/O and distributed computing.
- Efficient random access: Since data is chunked, specific regions can be read or written without loading the entire dataset.
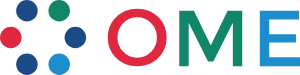
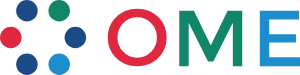
-
Interoperability: Ensures compatibility across imaging software, hardware, and analysis tools.
-
OME Data Model: Defines a standardized way to represent metadata (e.g., microscope settings, acquisition parameters) for reproducibility.
-
OME Files & Formats: Provides tools like Bio-Formats for reading and writing various imaging file formats.
- OME-NGFF (Next-Generation File Format): A vision for scalable, cloud-native bioimaging data storage.
(Open Microscopy Environment)
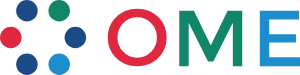
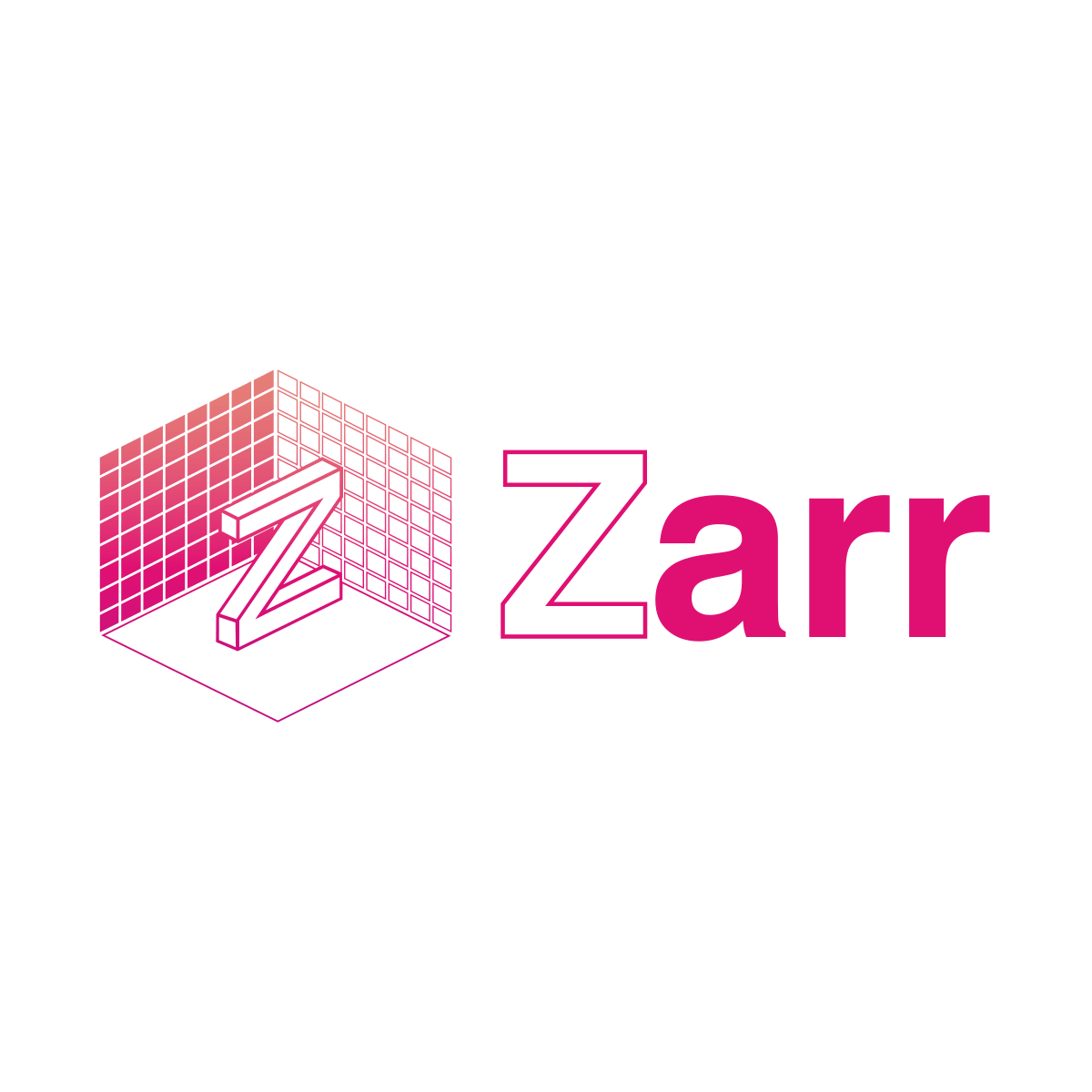
-
specifications for imaging data
chunked file storage
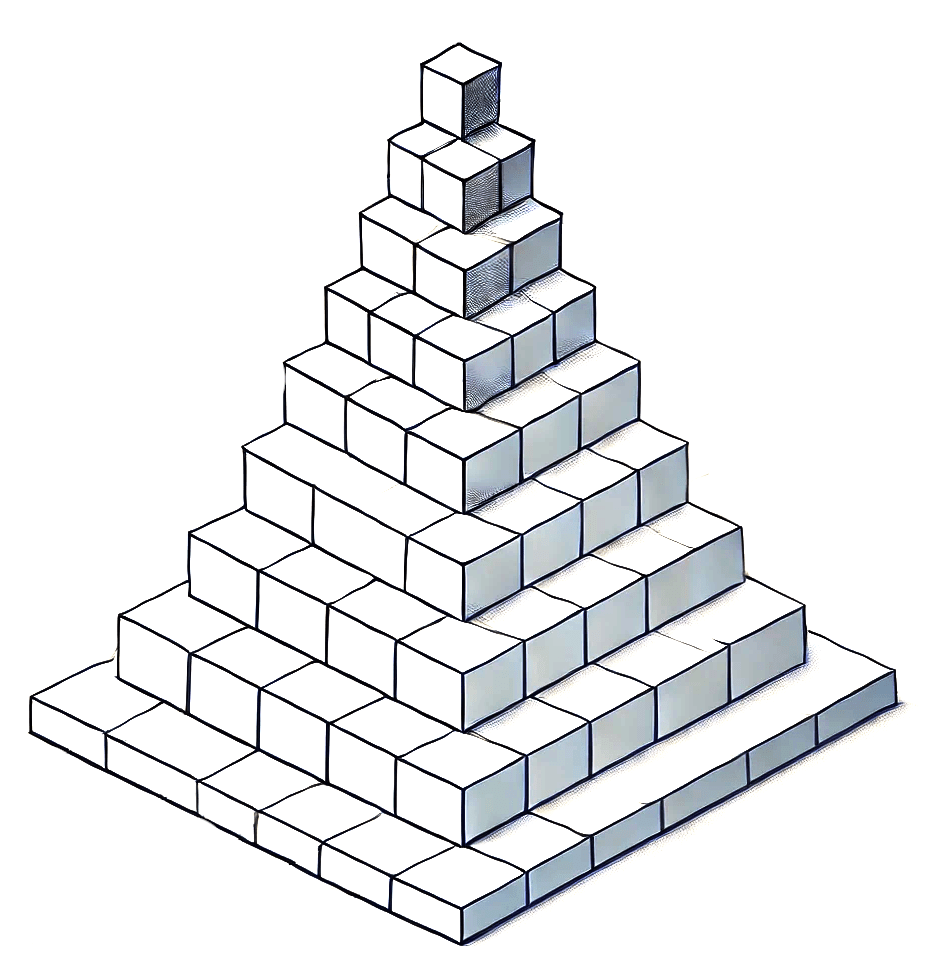
lower resolution scales of the data
original high resolution data
dataset is divided into managable chunks
Practical session notes
conda create -n qim3d python=3.11
conda activate qim3d
pip install qim3d
test by running qim3d
on the terminal, or pip show qim3d
pip install jupyter
Install jupyter
documentation for qim3d: platform.qim.dk/qim3d/
Data visualization for cultural heritage science
By Felipe Delestro
Data visualization for cultural heritage science
- 43