Workshop
Conclusion & Discussion
Francois Lanusse
Simons Foundation / CNRS
I can't believe we don't have a solution for that!?!
Data Reduction with AI
Has Deep Learning Really Solved Image Processing?
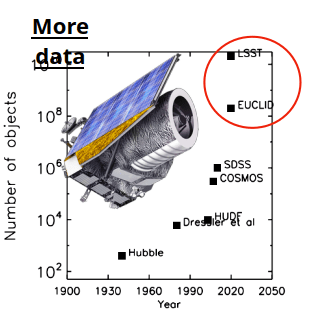
Credit: Marc's Thursdays Talk
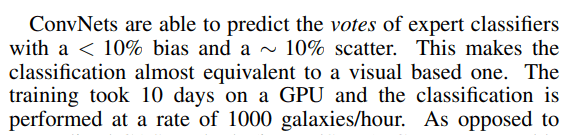
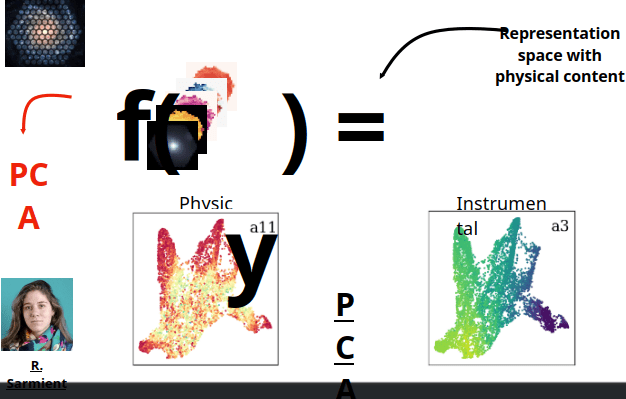
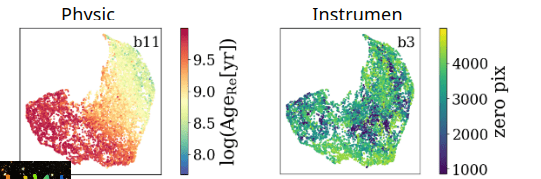
In total we have examined ∼ 50, 000 objects. On average one in 150 of the objects with [high] probability from the ResNet model is deemed a lens candidate through human inspection.
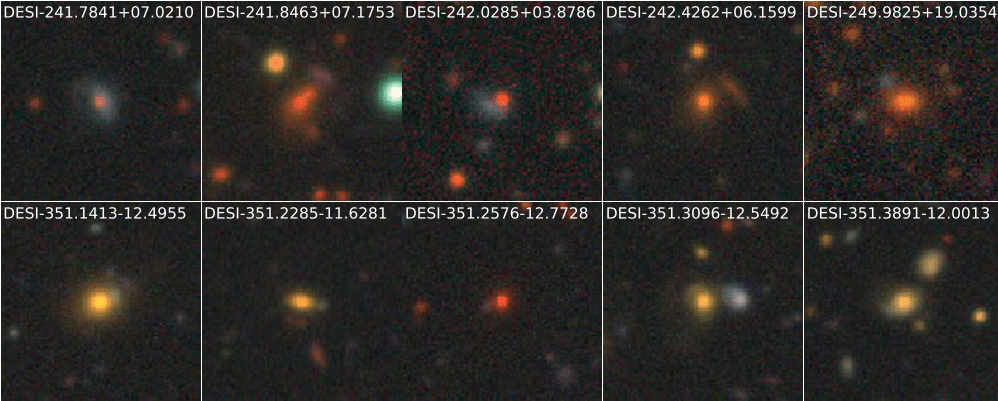
=> At the rate of LSST, this would mean needing human inspection for O(10^7) candidates
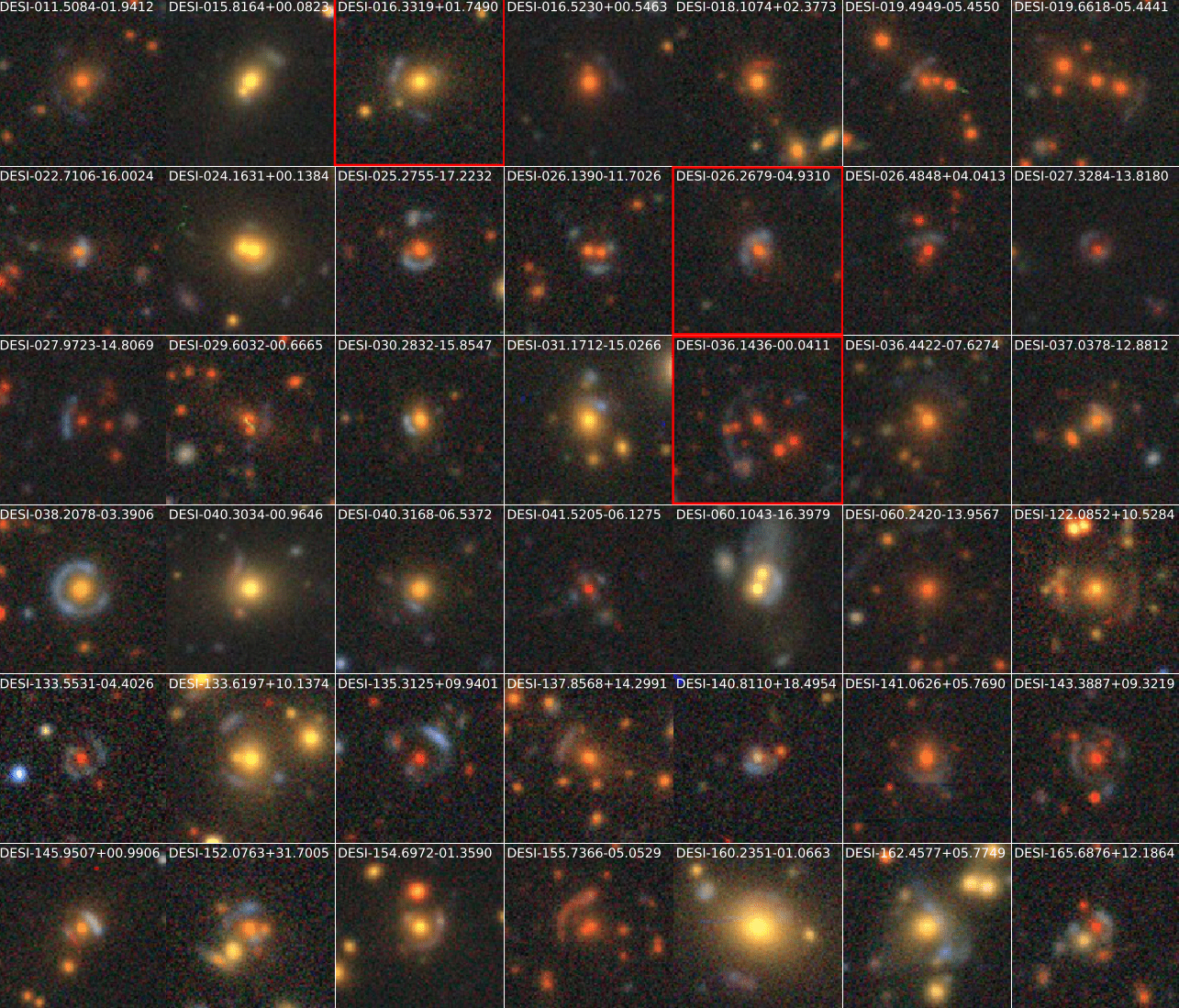
Deep Learning Representations Are Not Very Deep...
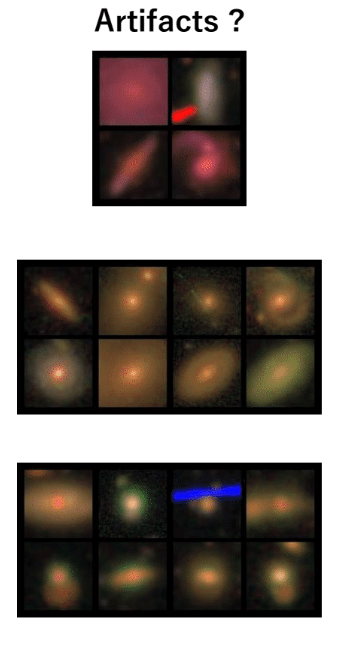
Credit: Tanaka's Thursday Talk
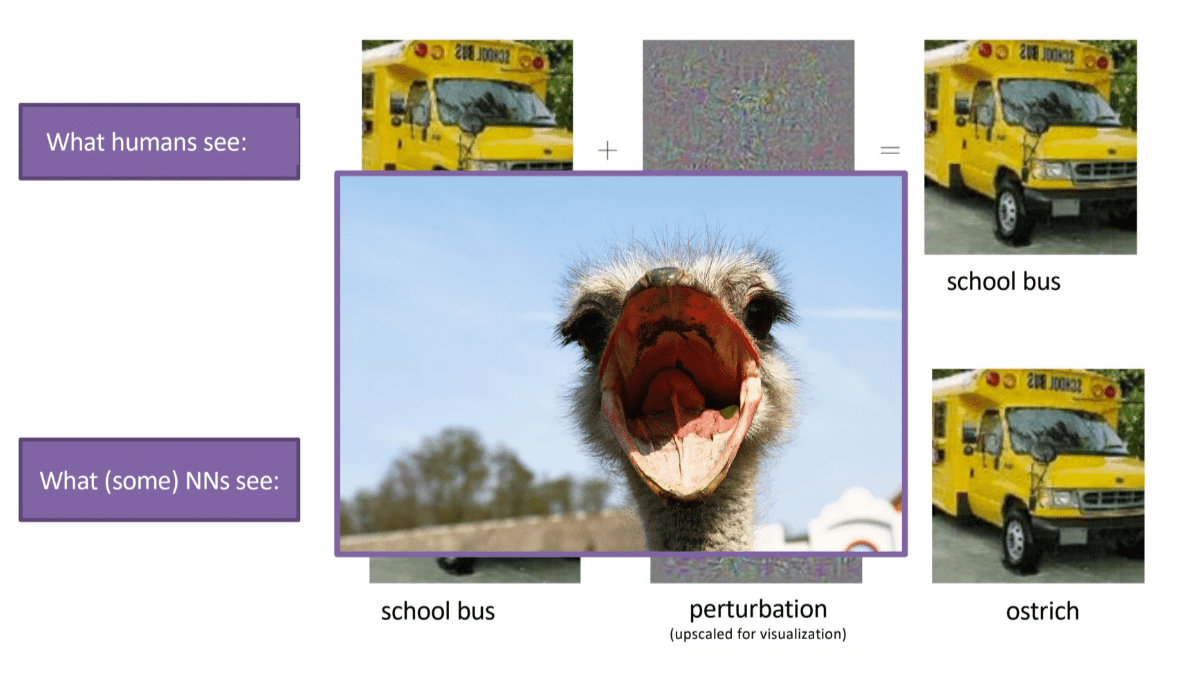
Credit: Anna's Thursday Talk
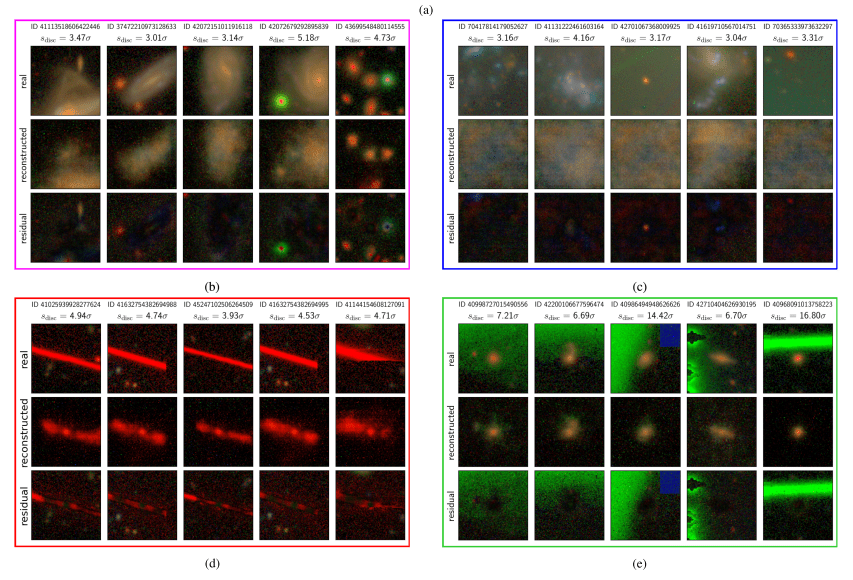
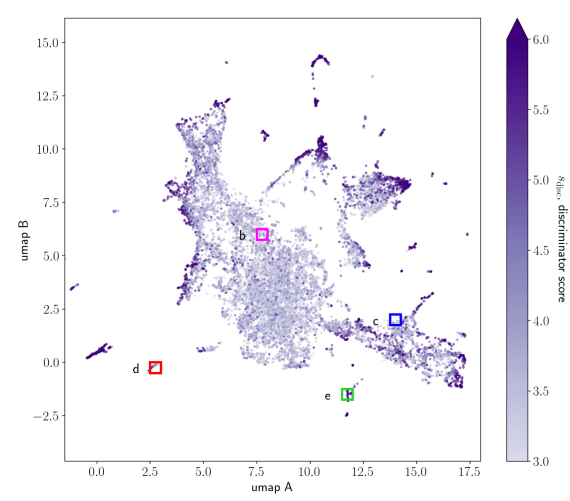
How do we build AI systems with a deep understanding of the data?
Pushing the limits of physical inference with AI
Simulation-Based Inference Works!
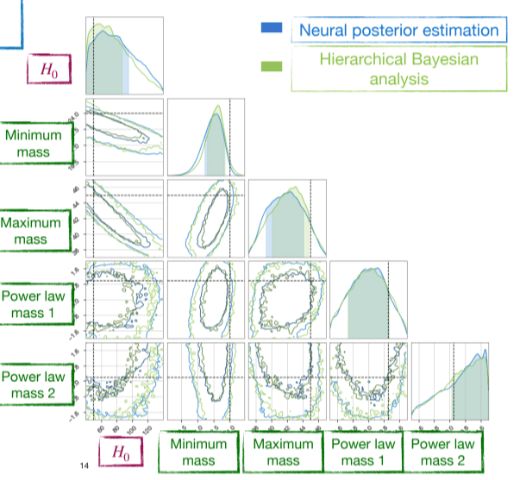
Credit: Konstantin's talk
Important point: If your simulator matches your Bayesian model, traditional MCMC should be equivalent to SBI
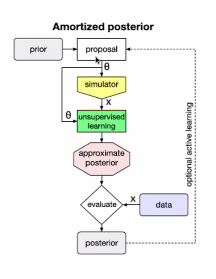
Credit:
Jennifer's talk
SBI is fast!
This is very exciting to make better use of available information!
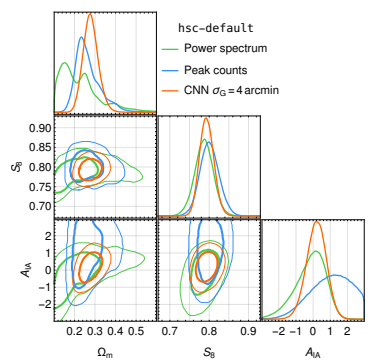
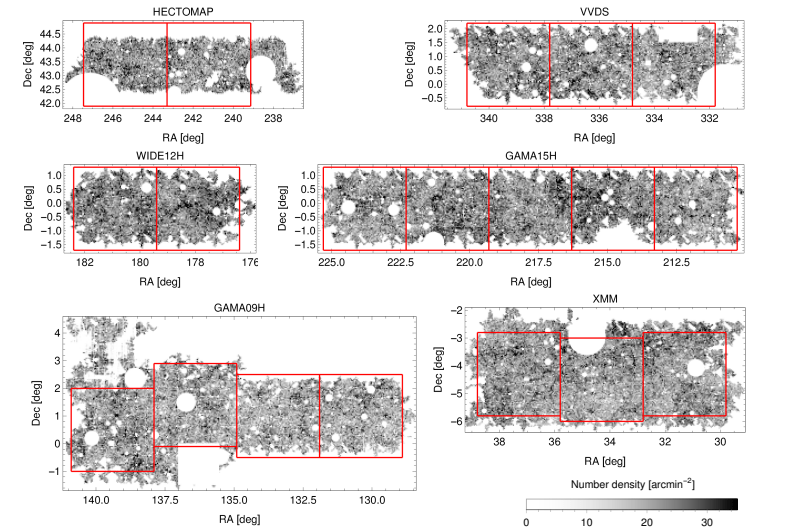
Lu et al. 2023
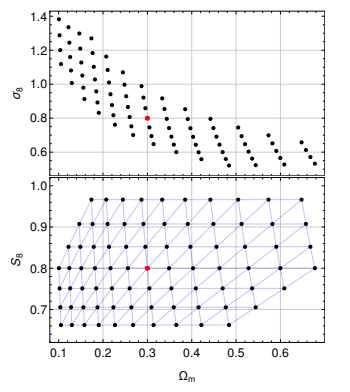
We May Also be Able to Perform Field-Level Inference Explicitly!
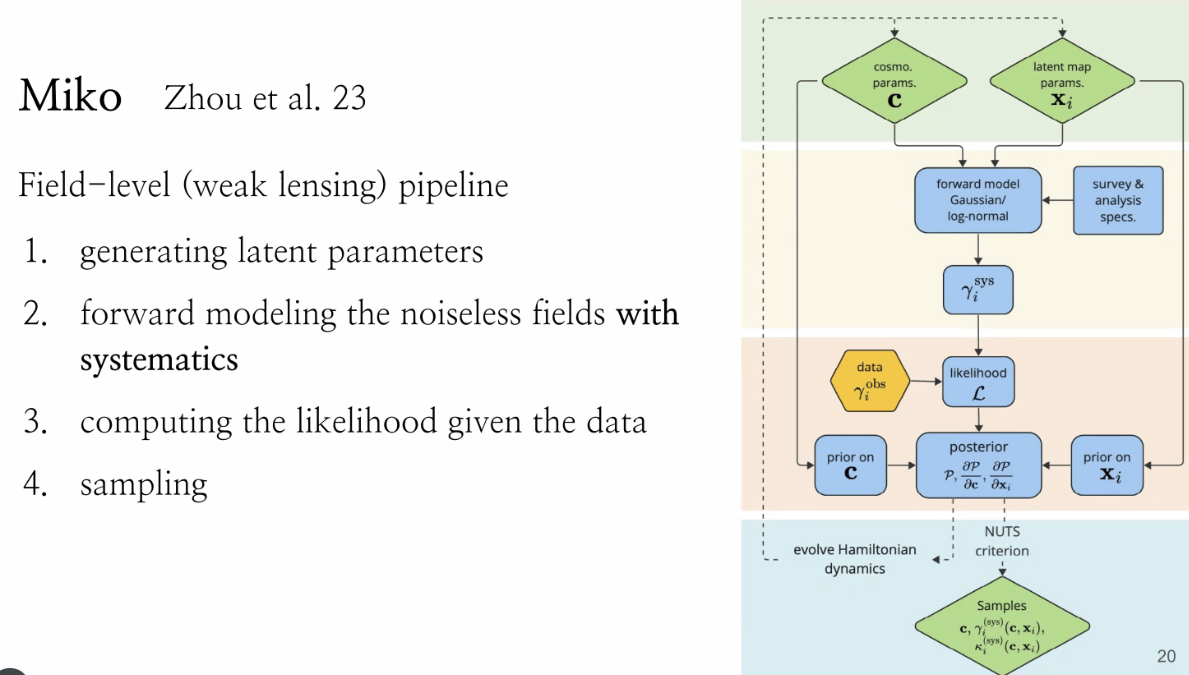
Credit: Alan's talk
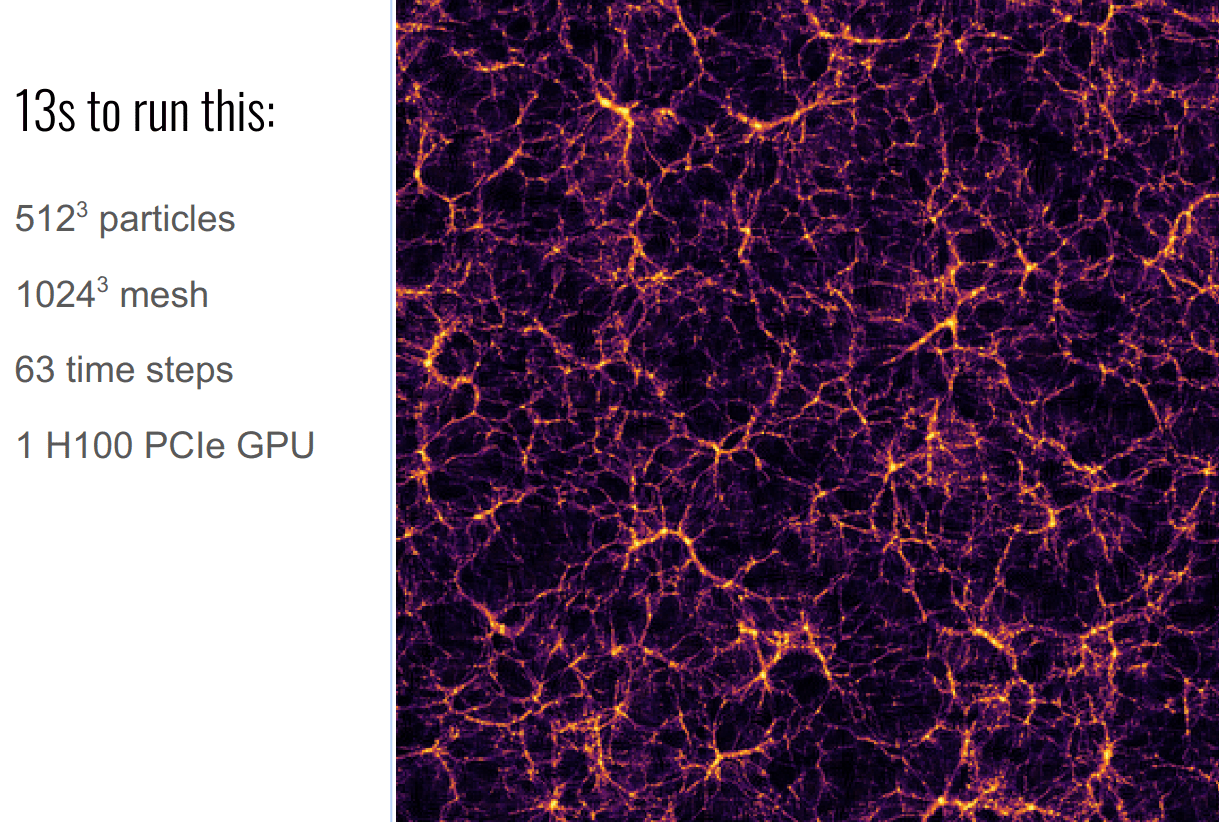
Credit: Yin's talk
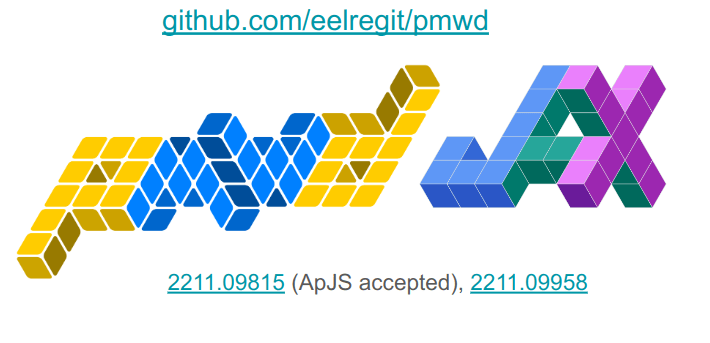
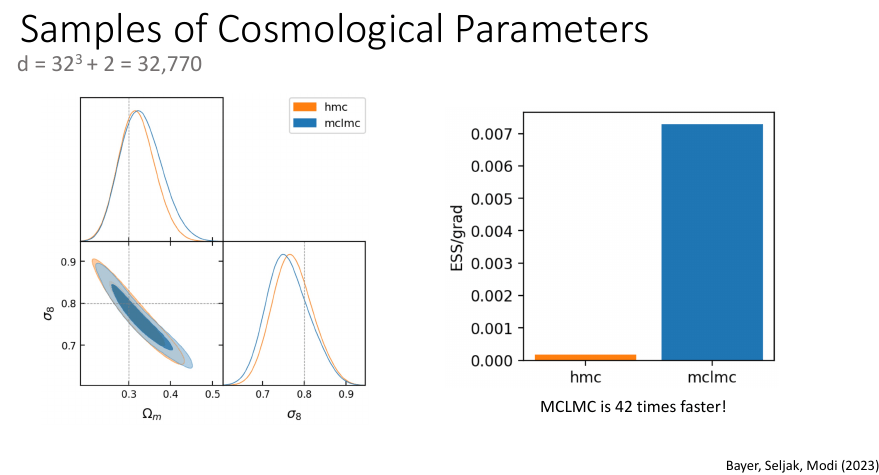
Credit: Adrian's talk
Emulating Complex Simulations
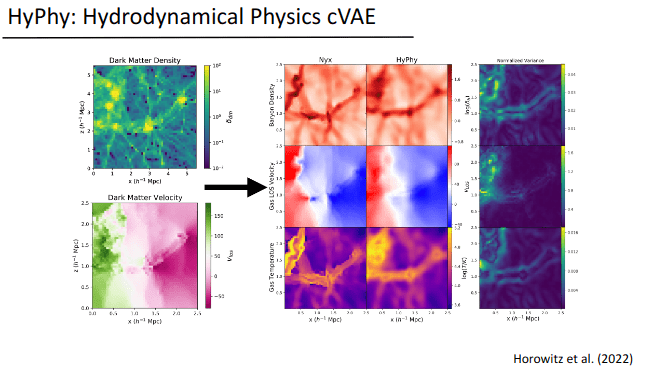
Credit: Ben's talk
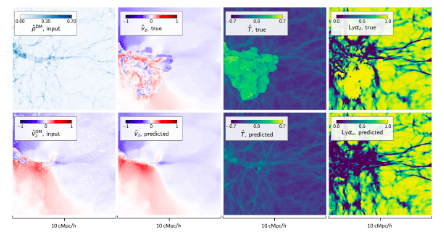
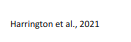
It's not necessarily an easy problem!
But can we build accurate enough models?
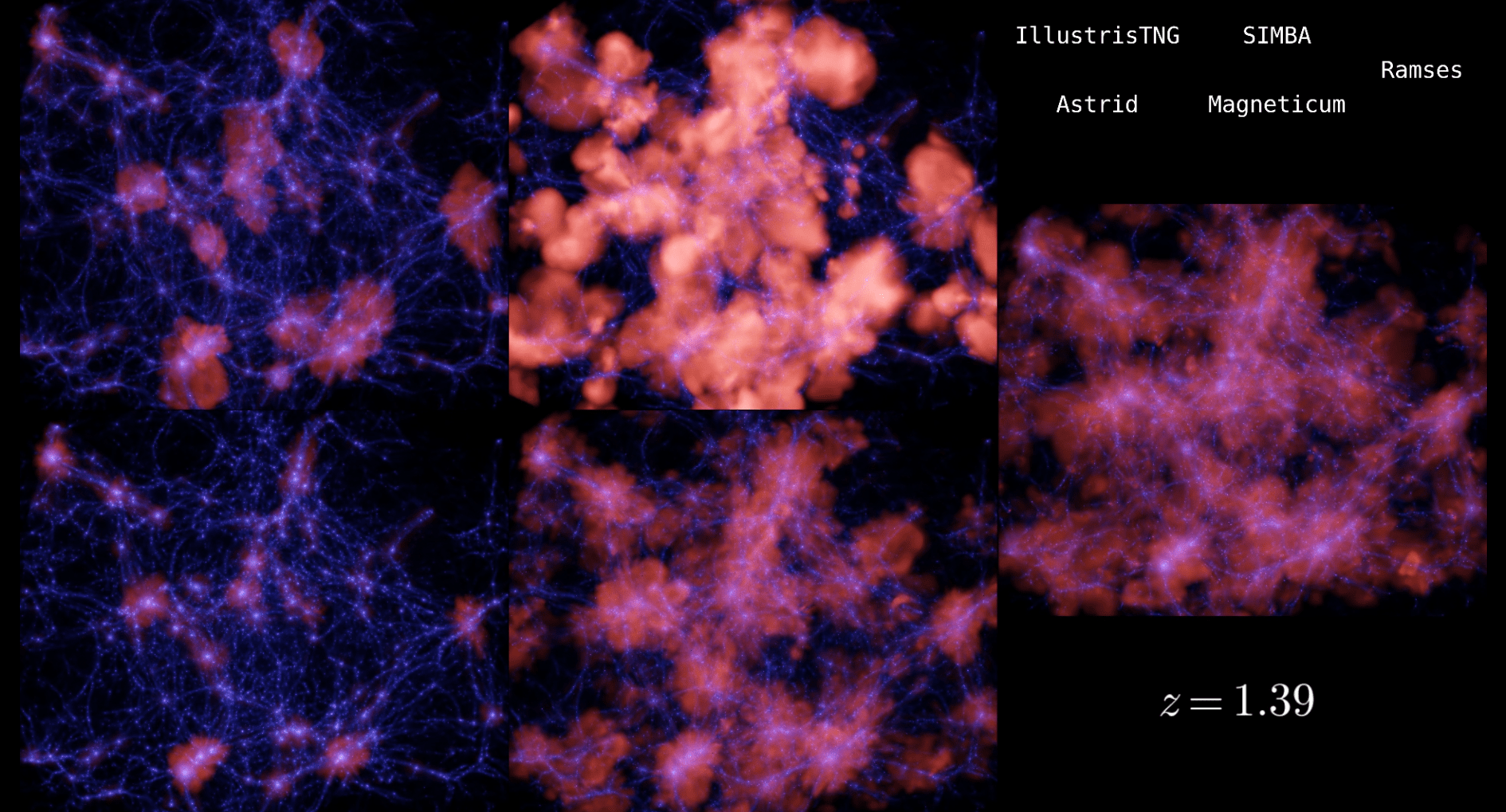
Credit: Paco's talk
And it's even worse than that!
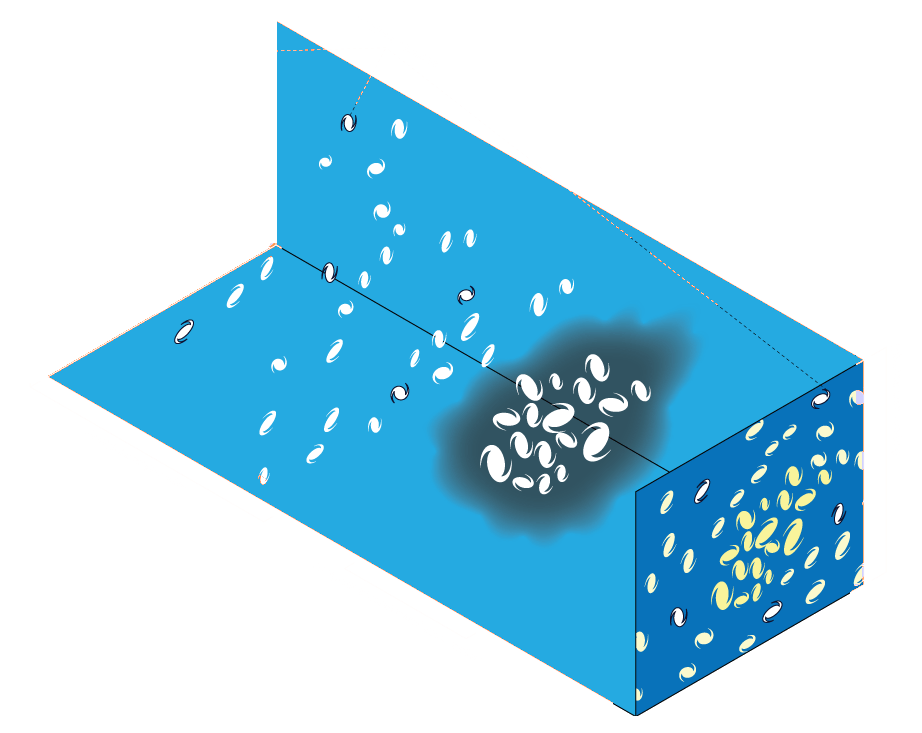
Gatti, Jeffrey, Whiteway et al. (2023)
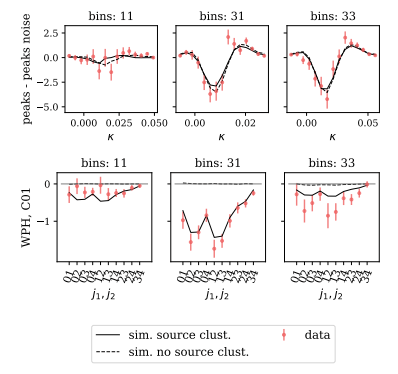
Impact of source clustering in weak lensing simulations
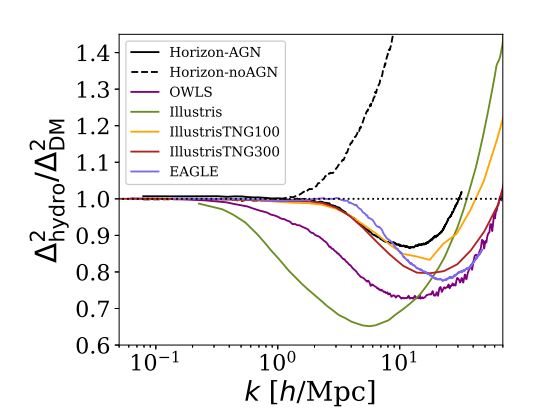
Impact of baryons on the power spectrum
Chisari et al. (2018)
What Solutions Can we Imagine for This Model Misspecifiation Problem ?
What would you like to be able to do with AI?
Francois' Answers
How do we build AI systems with a deep understanding of the data?
Creating a Deeper Connection to the Physics
Emergent Features in Self-Supervised Represenation
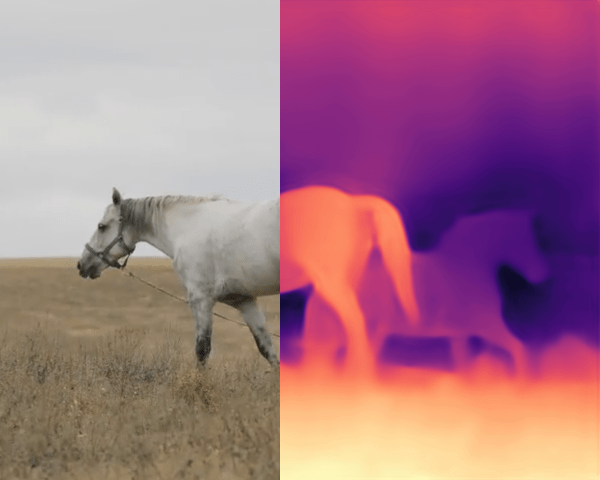
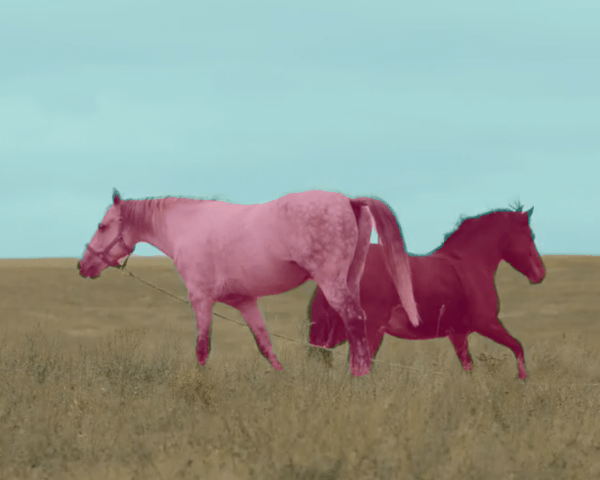
Dino v2
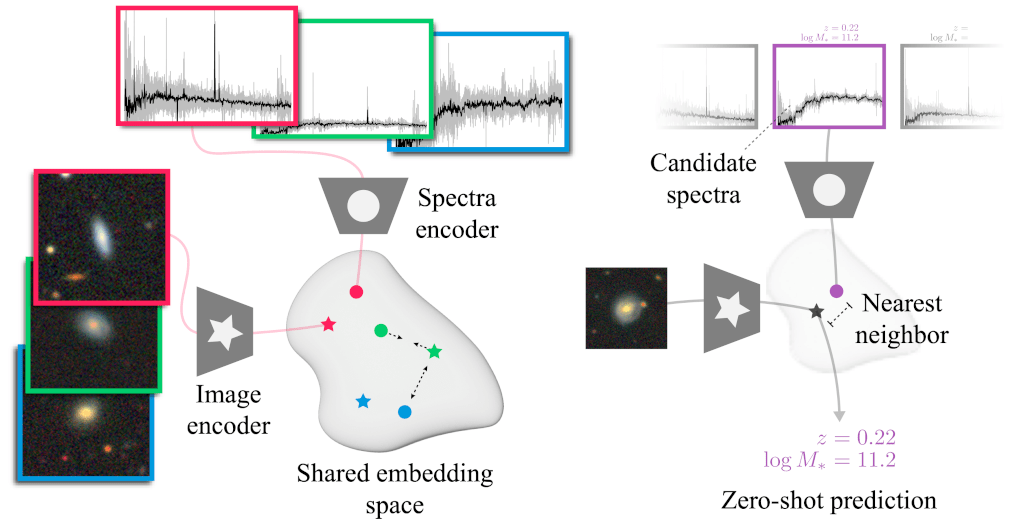
Towards more flexible architectures
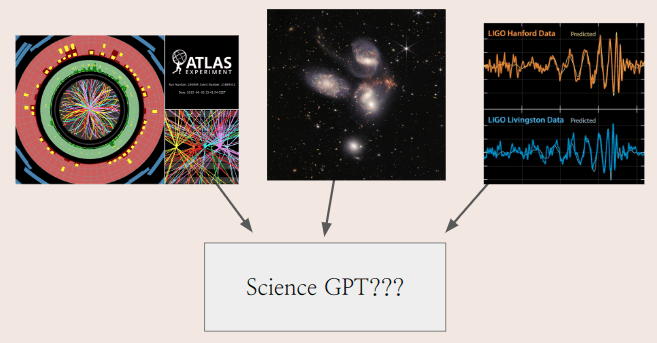
Credit: Mariel's talk
What Solutions Can we Imagine for This Model Misspecifiation Problem ?
Hybrid Parametrization of Forward Model with Nuisance Parameters
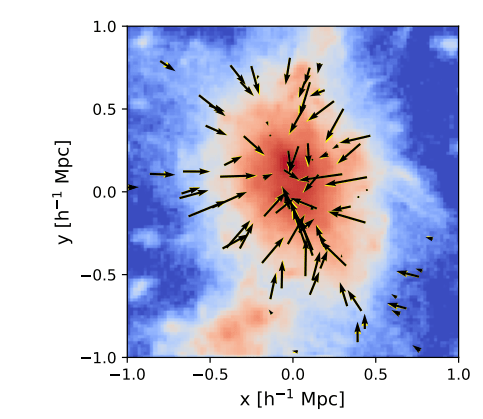
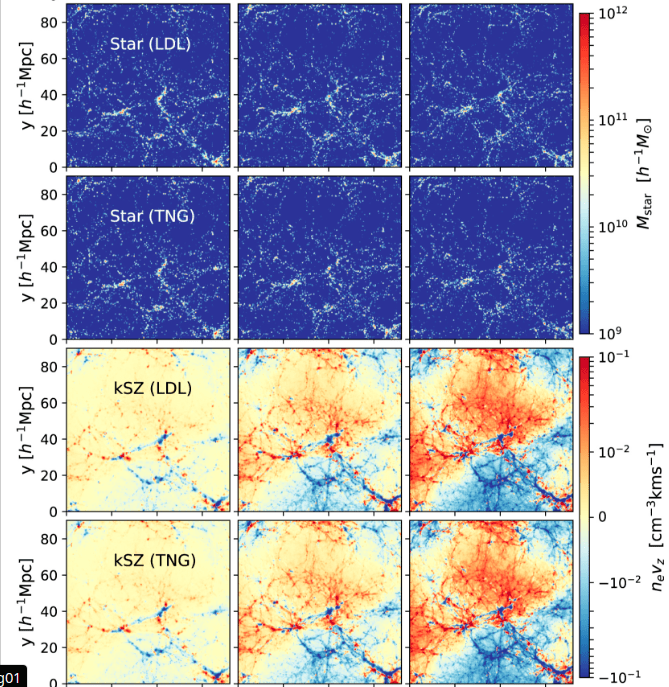
Dai et al. 2018
Dai & Seljak 2021
AI4Phys
By eiffl
AI4Phys
- 280