Deep Probabilistic Learning
Capturing Uncertainties with Deep Neural Networks
ML Club, Thursday November 7th 2019

Francois Lanusse @EiffL
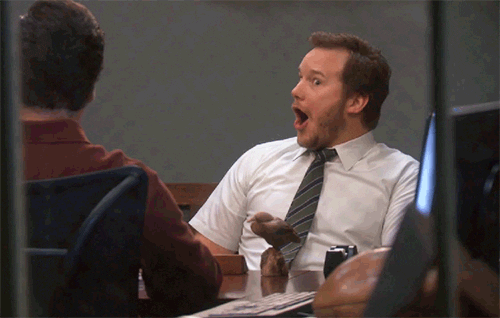
Follow the slides live at
https://slides.com/eiffl/ml_club/live
Outline for this session
- Modeling aleatoric uncertainties
- Conditional Density Estimators
- Likelihood-Free Inference with Neural Posterior Estimators
- Modeling epistemic uncertainties
- Bayesian Neural Networks
- Out of Distribution estimation
Before we dive in...
What uncertainties are we talking about?
A Motivating Example: Probabilistic Linear Regression
From this excellent tutorial:




- Linear regression
- Aleatoric Uncertainties
- Epistemic Uncertainties
- Epistemic+ Aleatoric Uncertainties
Modeling Aleatoric Uncertainties
Let us consider a toy example


There are intrinsic uncertainties in this problem, at each x there is a full
- Option 1) Train a neural network to learn a function under an MSE loss:
- Option 2) Train a neural network to learn a function under an l1 loss:
- Option 3) Train a neural network to learn a distribution using a Maximum Likelihood loss


I have a set of data points {x, y} where I observe x and want to predict y.
Try it out with this notebook





How did we do this?
Step 1: Conditional Neural Density Estimators
We need a parametric conditional distribution to
compute
- Mixture Density Networks
Bishop 1994
- Autoregressive models
e.g. MADE (Germain et al. 2015)
- Normalizing Flows
e.g. MAF (Papamakarios et al. 2017)



How do we fit this conditional distribution?
A distance between distributions: the Kullback-Leibler Divergence
Step 2: We need a tool to compare distributions
Minimizing this KL divergence is equivalent to minimizing the negative log likelihood of the model
How do we do this in practice?
import tensorflow as tf
import tensorflow_probability as tfp
tfd = tfp.distributions
# Build model.
model = tf.keras.Sequential([
tf.keras.layers.Dense(1+1),
tfp.layers.IndependentNormal(1),
])
# Define the loss function:
negloglik = lambda x, q: - q.log_prob(x)
# Do inference.
model.compile(optimizer='adam', loss=negloglik)
model.fit(x, y, epochs=500)
# Make predictions.
yhat = model(x_tst)
A Concrete Example: Estimating Masses of Galaxy Clusters
Try it out at this notebook


We want to make dynamical mass measurements using information from member galaxy velocity dispersion and about the radial distance distribution (see Ho et al. 2019).
First attempt with an MSE loss
regression_model = keras.Sequential([
keras.layers.Dense(units=128, activation='relu', input_shape=(14,)),
keras.layers.Dense(units=128, activation='relu'),
keras.layers.Dense(units=64, activation='tanh'),
keras.layers.Dense(units=1)
])
regression_model.compile(loss='mean_squared_error', optimizer='adam')

- Simple Dense network using 14 features derived from galaxy positions and velocity information
- We see that the predictions are biased compared to the true value of the mass... Not good.
Second attempt: Probabilistic Modeling
num_components = 16
event_shape = [1]
model = keras.Sequential([
keras.layers.Dense(units=128, activation='relu', input_shape=(14,)),
keras.layers.Dense(units=128, activation='relu'),
keras.layers.Dense(units=64, activation='tanh'),
keras.layers.Dense(tfp.layers.MixtureNormal.params_size(num_components, event_shape)),
tfp.layers.MixtureNormal(num_components, event_shape)
])
negloglik = lambda y, p_y: -p_y.log_prob(y)
model.compile(loss=negloglik, optimizer='adam')

- Same Dense network but now using a Mixture Density output.
- Using the mean of the predicted distribution as our mass estimate: We see the exact same behaviour
What am I doing wrong???
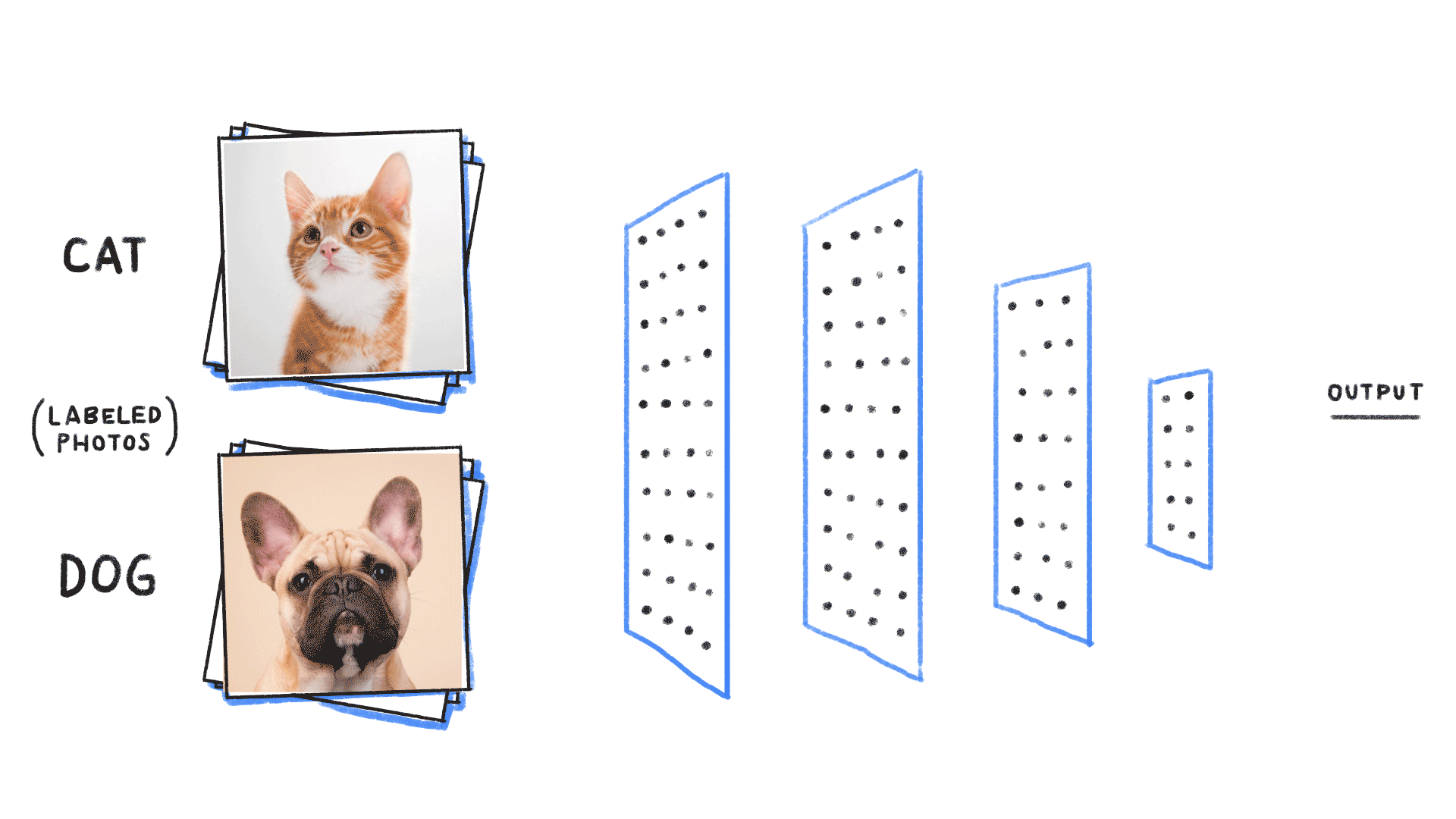
credit: Venkatesh Tata
Let's start with binary classification
=> This means expressing the posterior as a Bernoulli distribution with parameter predicted by a neural network
How do we adjust this parametric distribution to match the true posterior ?
Step 1: We neeed some data
cat or dog image
label 1 for cat, 0 for dog
Probability of including cats and dogs in my dataset

Google Image search results for cats and dogs
Minimizing this KL divergence is equivalent to minimizing the negative log likelihood of the model
At minimum negative log likelihood, up to a prior term, the model recovers the Bayesian posterior
with
How do we adjust this parametric distribution to match the true posterior ?
In our case of binary classification:
We recover the binary cross entropy loss function !
The Probabilistic Deep Learning Recipe for Neural Posterior Estimation
- Express the output of the model as a distribution
- Optimize for the negative log likelihood
- Maybe adjust by a ratio of proposal to prior if the training set is not distributed according to the prior
- Profit!
Back to our Dynamical Mass Predictions

Distribution of masses in our training data


We can reweight the predictions for a desired prior

Last detail, use the mode instead of the mean posterior


Takeaway Message
- Using a model that outputs distributions instead of scalars is always better!
- It's 2 lines of TensorFlow Probability
- Careful about interpreting these distributions as a Bayesian posterior, the training set acts as an Interim Prior, not necessarily matching your Bayesian prior.
Modeling Epistemic Uncertainties
A Quick reminder
From this excellent tutorial:




- Linear regression
- Aleatoric Uncertainties
- Epistemic Uncertainties
- Epistemic+ Aleatoric Uncertainties
The idea behind Bayesian Neural Networks


Given a training set D = {X,Y}, the predictions from a Neural Network can be expressed as:

Weight Estimation by Maximum Likelihood

Weight Estimation by Variational Inference

A first approach to BNNs:
Bayes by Backprop (Blundel et al. 2015)
-
Step 1: Assume a variational distribution for the weights of the Neural Network
-
Step 2: Assume a prior distribution for these weights
- Step 3: Learn the parameters of the variational distribution by minimizing the ELBO


What happens in practice


TensorFlow Probability implementation
A different approach:
Dropout as a Bayesian Approximation (Gal & Ghahramani, 2015)
Quick reminder on dropout
Hinton 2012, Srivastava 2014


Variational Distribution of Weights under Dropout
-
Step 1: Assume a Variational Distribution for the weights
-
Step 2: Assume a Gaussian prior for the weights, with "length scale" l
- Step 3: Fit the parameters of the variational distribution by optimizing the ELBO


Example

These are not the only methods
- Noise contrastive priors: https://arxiv.org/abs/1807.09289

Takeaway message on Bayesian Neural Networks
- They give a practical way to model epistemic uncertainties, aka unknowns unknows, aka errors on errors
- Be very careful when interpreting their output distributions, they are Bayesian posterior, yes, but under what priors?
- Having access to model uncertainties can be used for active sampling


Putting it all together

Deep Probabilistic Learning
By eiffl
Deep Probabilistic Learning
ML Club session of Thursday November 7th 2019
- 1,041