Denser Tensor Spaces
2021 James B. Wilson, Colorado State University

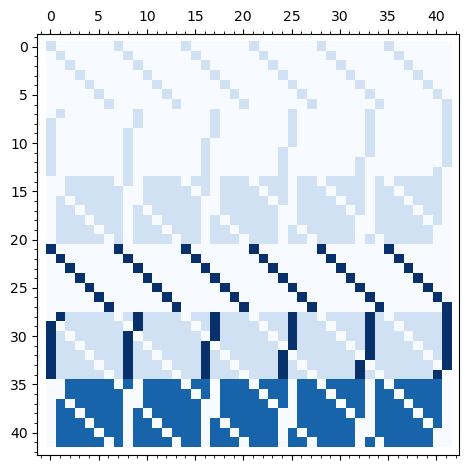
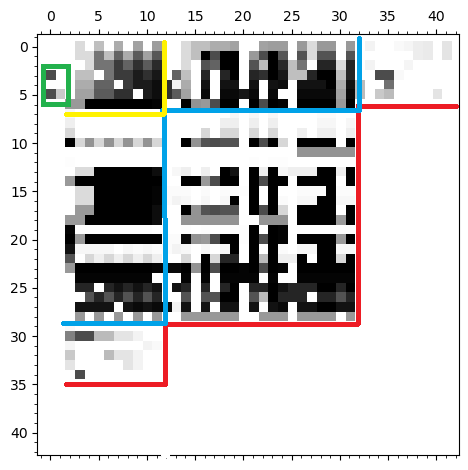
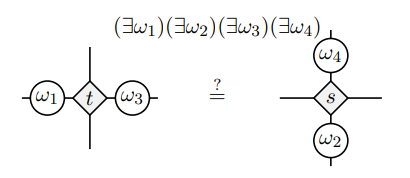
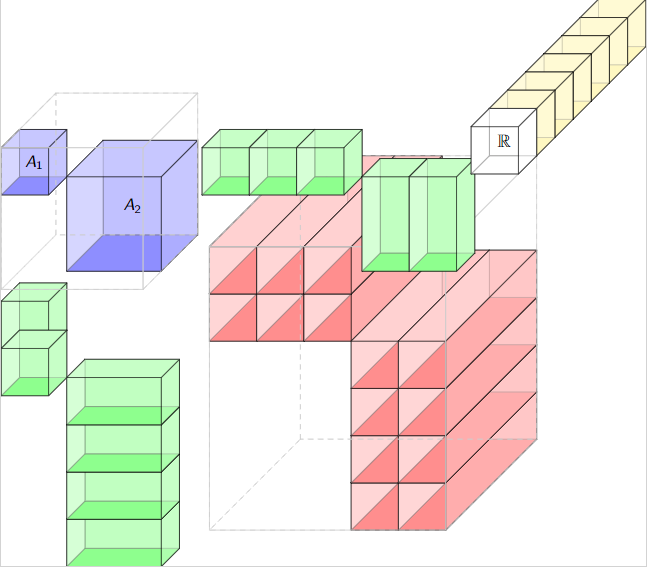

Major credit is owed to...
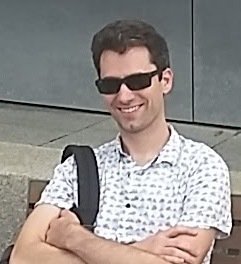
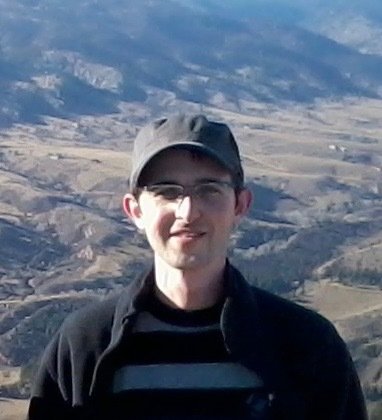
Uriya First
U. Haifa
Joshua Maglione,
Bielefeld
Peter Brooksbank
Bucknell
- The National Science Foundation Grant DMS-1620454
- The Simons Foundation support for Magma CAS
- National Secturity Agency Grants Mathematical Sciences Program
- U. Colorado Dept. Computer Science
- Colorado State U. Dept. Mathematics
Three Goals of This Talk
- Cluster non-zeros in a tensor.
- Compare two tensors up to basis change
- Make the algorithms for the above feasible.
Can we agree on "tensors"?
(I hope!)
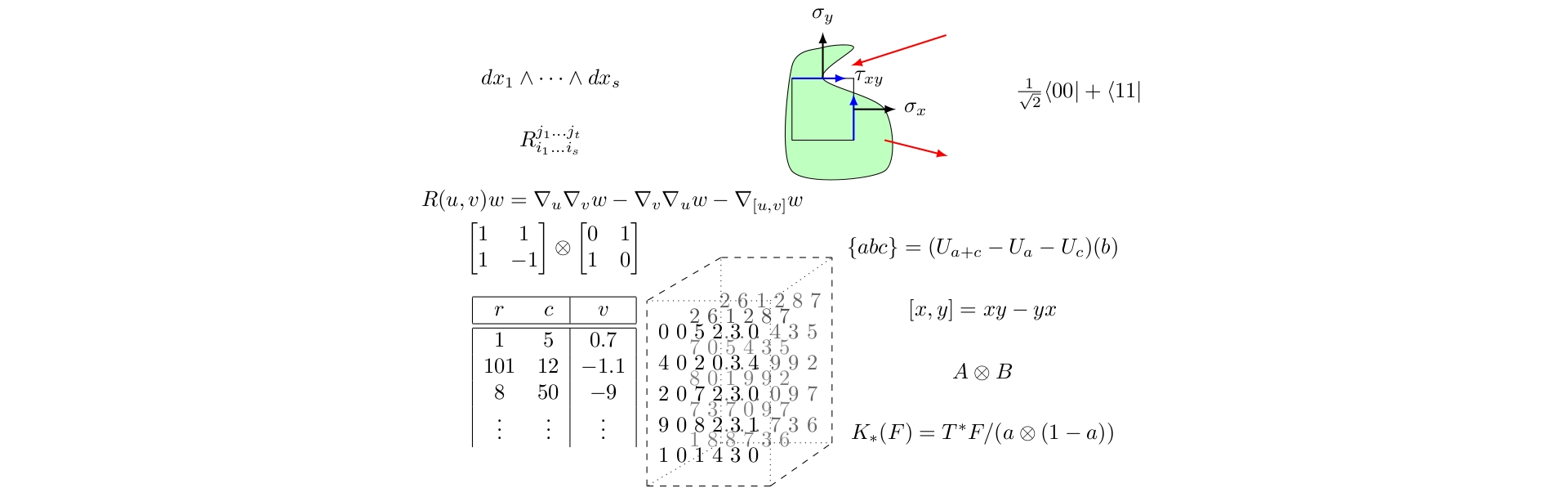
Are all of these tensors?
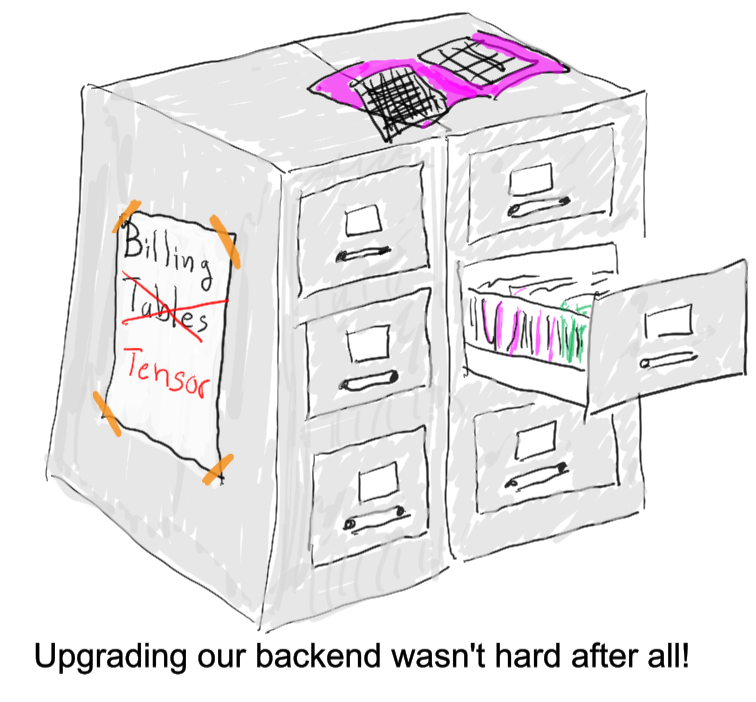
Don't be a joke
If you don't use linear combinations on some axis of your data...
then its not actually a tensor, sorry.
"What is a vector?"
"An element of a vector space."
U0⊘U1={f:U1→U0∣f(u+λv)=f(u)+λf(v)}
U0,U1,… are vector spaces (or modules).
Linear maps:
U0⊘U1⊘U2:={f:U2→U0⊘U1∣f(u+λv)=f(u)+λf(v)}
Bi-Linear maps:
U0⊘⋯Uk−1⊘Uk:=(U0⊘⋯⊘Uk−1)⊘Uk
k-multi-linear maps:
"What is a tensor?"
"An element of a tensor space."
U0,U1,… are vector spaces (or modules).
U0⊘⋯Uk−1⊘Uk:=(U0⊘⋯⊘Uk−1)⊘Uk
k-multi-linear maps:
Defn. A Tensor Space T is a vector space an a linear map ⟨⋅∣:T→U0⊘⋯⊘Uk
Tensors are elements of tensor spaces.
T=M2×3(R) is a tensor space in at least 3 ways!
⟨⋅∣:T↦R2⊘R3
⟨M∣u⟩:=Mu
∣⋅⟩:T↦R3⊘R2
⟨v∣M⟩:=v†M
∣⋅∣:T↦R⊘R2⊘R3
⟨v∣M∣u⟩:=v†Mu
Matrix as linear map on right.
Matrix as linear map on left.
Matrix as bilinear form.
This abstraction does wonders for creating a fluid tensor software package.
Operating on Tensors
Tier I
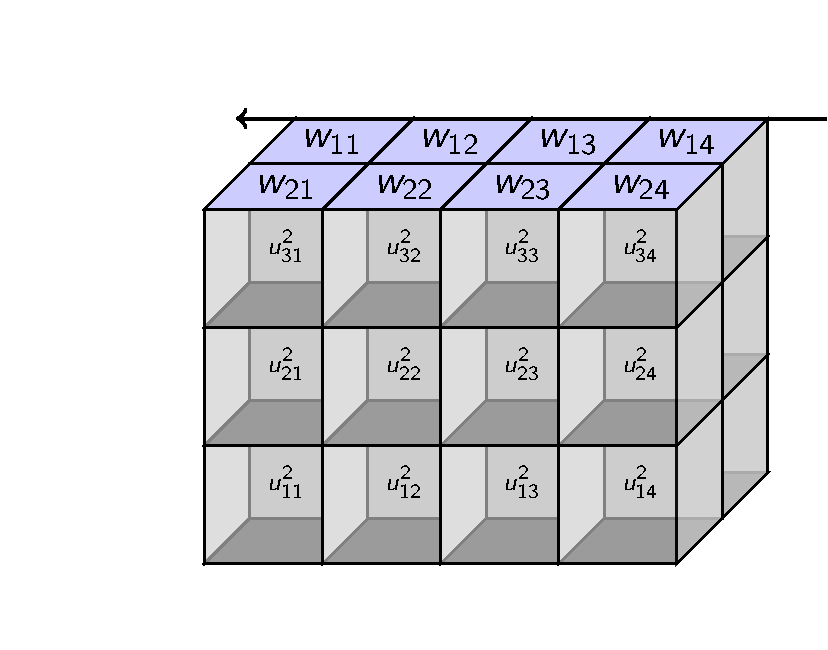
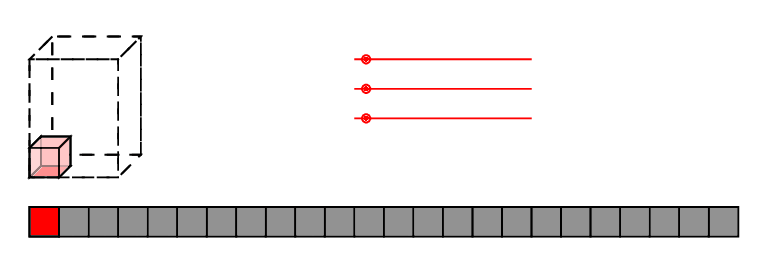
low-brow: reindex,
high-brow: affine transforms of polytopes
Evaluation
Contractions
Layout data so nothing moves!
Logically equivalent circuits
Fight eager evaluation
Tier II
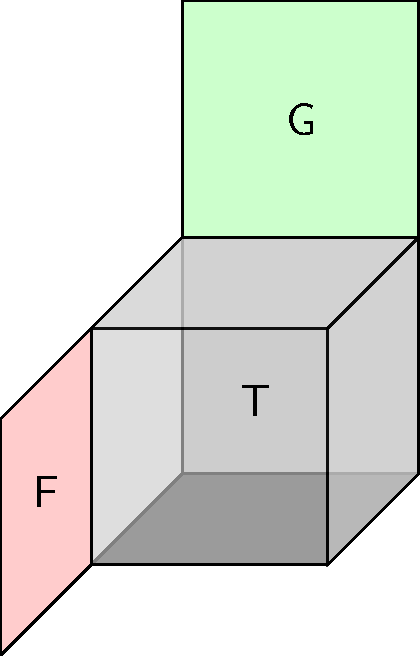
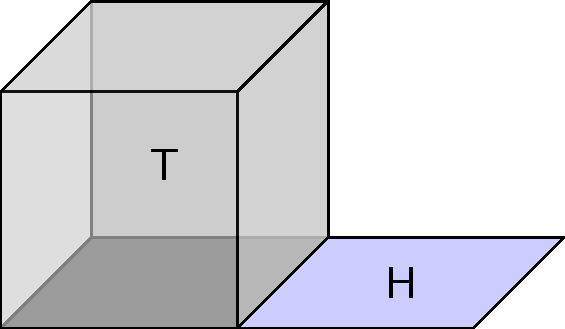
=
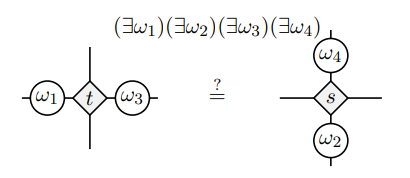
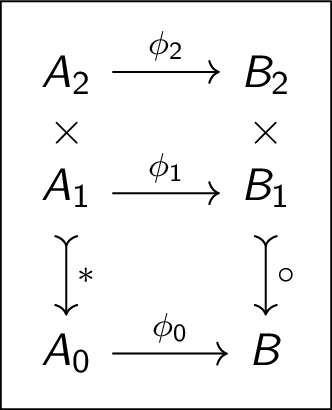
(Data) Acting on Tensors as arrays
(Lin. Alg.) Acting on tensors as functions
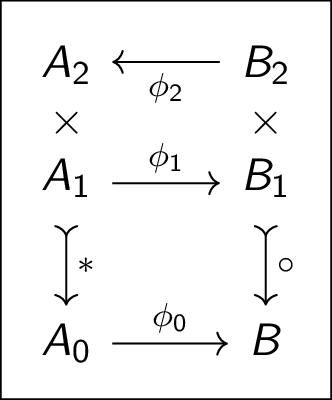
(Physics/Algebra) Acting on Tensors as Operads/Networks
Tier II key: Iterate
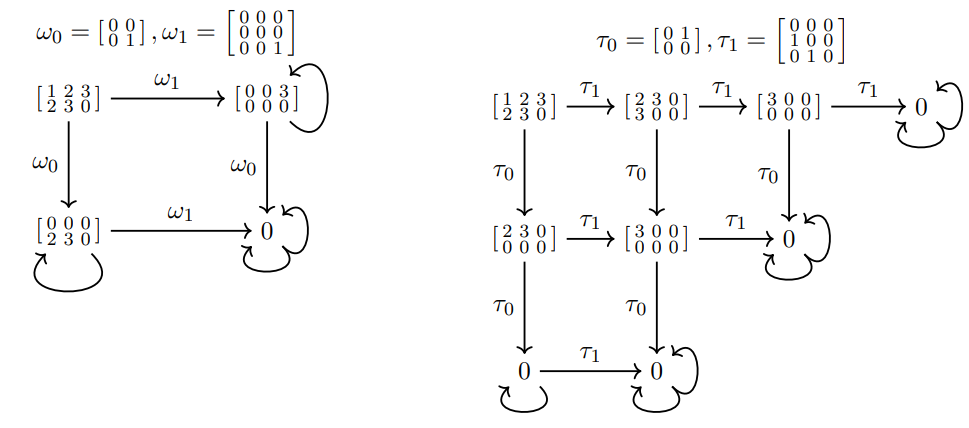
Generalizes Characteristic polynomial to ideals
I(t,ω)=(x2−x,y2−y,xy)I(t,τ)=(x2,y3,xy−y2)
Multi-spectrum Rule = ⟨t∣p(ω)=0
Thm FMW-Connection. S set of tensors, P⊂R[X], Ω⊂∏aMda(R)
T(P,Ω)={t∣P in multi-spec t at Ω}
I(S,Ω)={p∣p in multi-spec S at Ω}
Z(S,P)={ω∣P in multi-spec S at ω}
Then S⊂T(P,Ω)⇔P⊂I(S,Ω)⇔Ω⊂Z(S,P)
Thm FMW-Construction.
These are each polynomial time computable.
Multi-spectrum Rule = ⟨t∣p(ω)=0
Tier III
Functors on tensors, e.g. (U0⊘⋯⊘UK)→(Ui⊗⋯⊗Uk→U0⊘⋯⊘Ui−1)
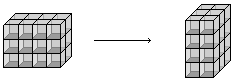
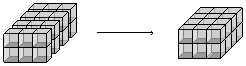
Save yourself time if you program these functors and avoid boiler plate later
How to shrink a tensor space
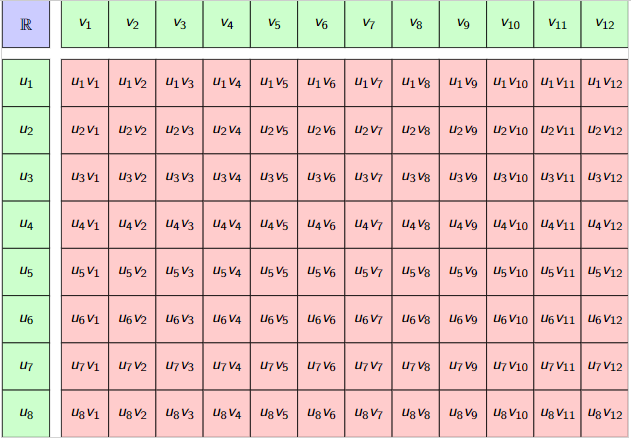
Red is the space we search/work within.
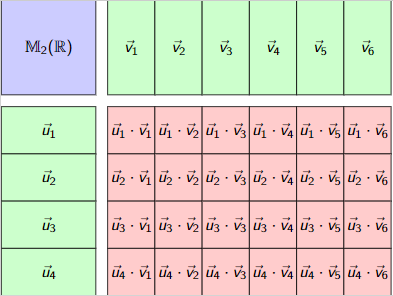
Add some algebra A in the form of U⊗AV
The bigger the algebra the better.
Rule of thumb
dim(U⊗AV)≈dimAdimUdimV
Some effort now in working with the algebra & modules, yet you can at least prove and plan for that.
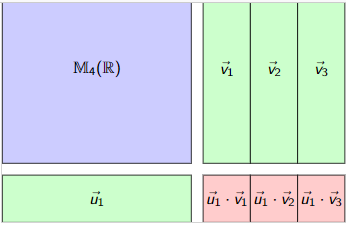
Adjoint-Tensor Theorem
- Given: 2-tensors S⊂Ma×b(R)
- Want: Algebra to shrink space around S
Theorem Brooksbank-W. (2012) Adj(S)={(F,G)∈Ma(R)×Mb(R)∣(∀T∈S)(FT=TGt)} is an optimal choice and unique up to isomorphism.
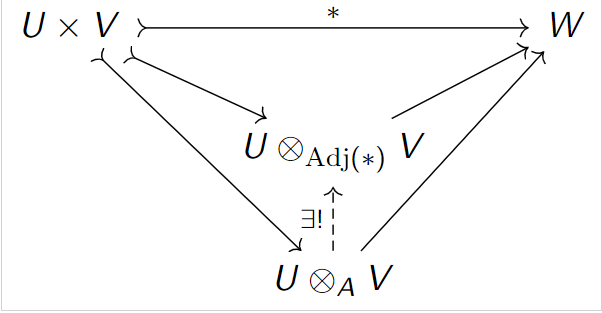
How not to shrink a tensor space
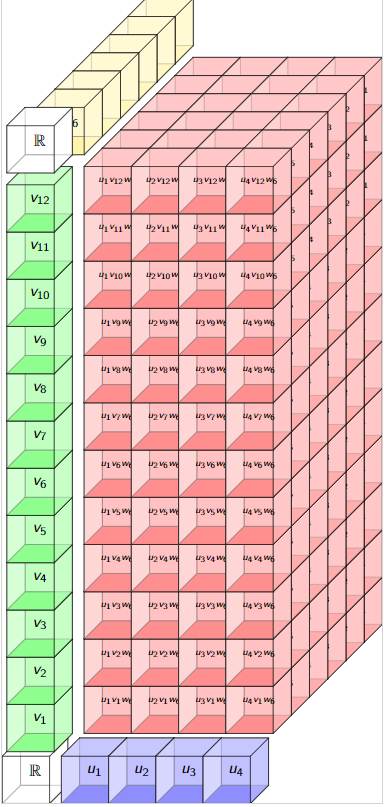
R4⊗RR12⊗RR6
R4⊗M2(R)R12⊗M3(R)R6
≅R2⊗RR2⊗RR2
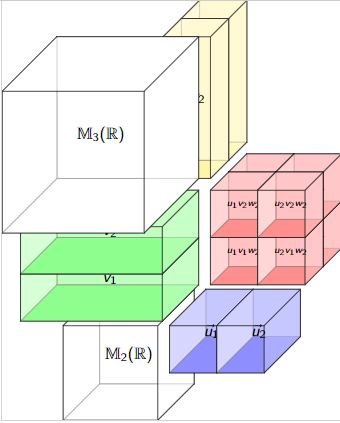
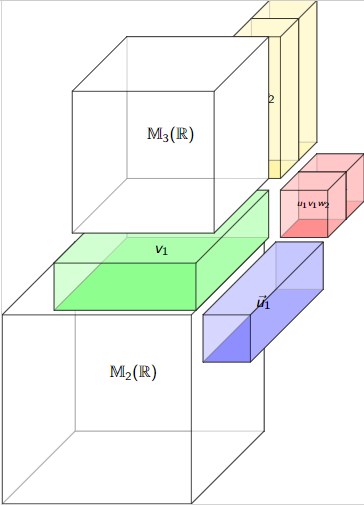
R4⊗M4(R)R12⊗M3(R)R6
≅R⊗RR⊗RR2
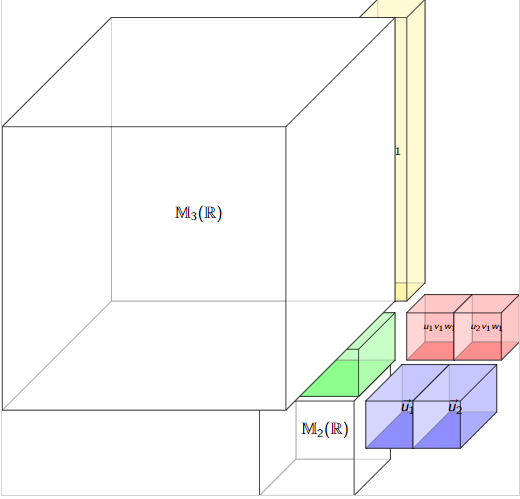
R4⊗M2(R)R12⊗M6(R)R6
≅R2⊗RR⊗RR
Adjoint-tensor methods in valence >2
- S⊂U1⊗⋯⊗Uv get (2v) generalized adjoints i.e. Adj(S)ij⊂Mdi(R)×Mdj(R)
- But the product only has v−1 spots to hang them...U1⊗A12U2⊗A23U3⊗⋯⊗A(v−1)vUv.
- We can permute...but rather arbitrary.
Things I wonder about....
Why can't we just act on one side?
E.g. U⊗AV needs UA,AV. Worse, U⊗AV⊗BW needs AVB a "bi-module".
Why do we tolerate "natural" isomorphisms U⊗(V⊗W)≅(U⊗V)⊗W
If its natural, can't we just write these down as equal?!
A new tensor product
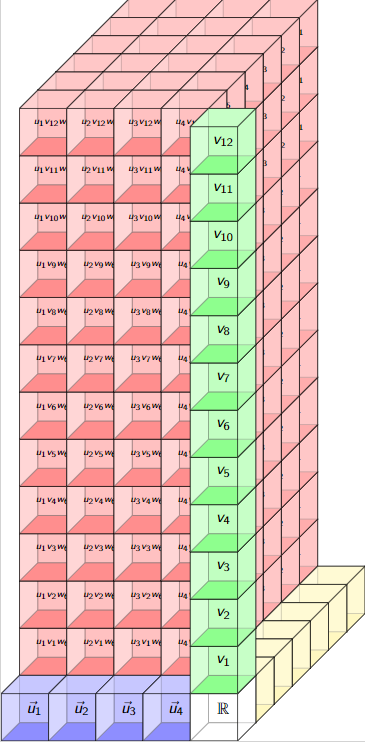
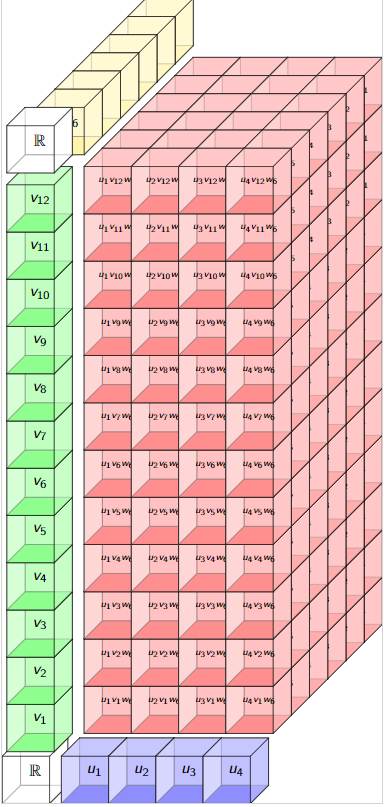
Whitney Tensor Product
A Different Tensor Product
New Tensor product:
Ω⊂Md1(R)×⋯×Mdk(R); P⊂R[x1,…,xk]
Ξ(P,Ω)=⟨e∑λeω1e1⊗⋯⊗ωkek e∑λeXe∈P,ω∈Ω⟩
(]U1,…,Uk[)ΩP:=(U1⊗⋯⊗Uk)/Ξ(P,ω)
Then we have:
(]⋯[):U1×⋯×Uk↪(]U1,…,Uk[)ΩP
defined by
(]u1,…,uk[):=u1⊗⋯⊗uk+Ξ(P,Ω)
Condensing Whitney Tensor Products
Condensing our alternative
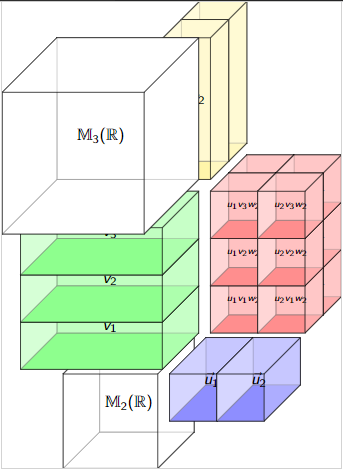
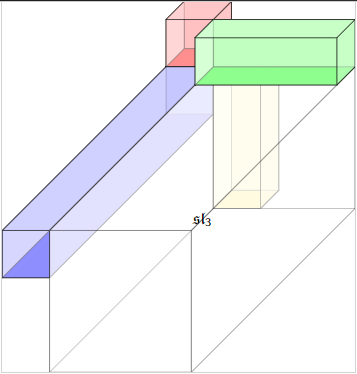
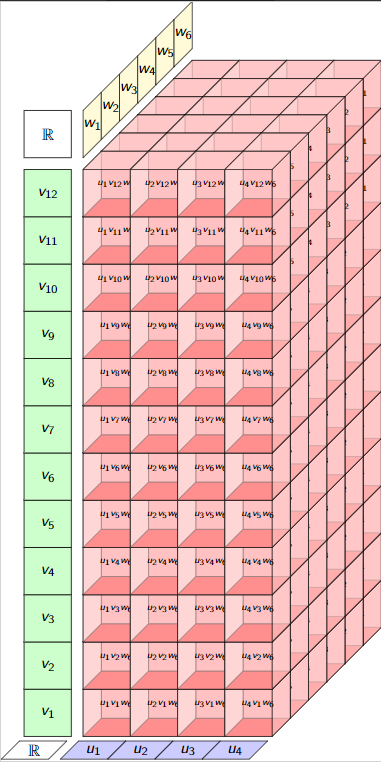
One corner to contract makes each axis independent.
(No bimodules, no "associative" rules)
(]⋯[):U1×⋯×Uk↪(]U1,…,Uk[)ΩP
is the universal tensor such that every ω∈Ω has P in its multi-spectrum.
Intuition....force the spectrum
Consequence:
- If P is homogeneous linear (so zero's are some affine subspace)
- Then it is contained in a hyperplane.
- Generically all hyperplanes are equal up to the torus action!
- Maybe there is a universally smallest product....?
Derivation-Densor Theorem
- Given: tensors t∈Rd1⊗⋯⊗Rdv
- Want: Algebra to shrink space around t
Theorem First-Maglione-W. Der(t) is all (δi)i∈∏Mdi(R) satisfying 0=⟨t∣δ1u1,…,un⟩+⋯+⟨t∣u1,…,δvuv⟩ is an optimal choice and unique up to isomorphism.
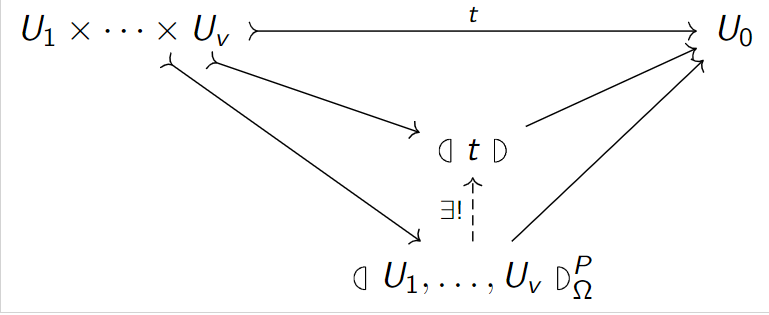
Lie algebras are required
Theorem First-Maglione-W. If P=(ΛX), Λ∈Mr×k is full rank and if Z(t,P)={ω∣P in the multi-spec t}
is an algebra, then it is a Lie algebra in at least k−2r coordinates.
U⊗AV for A associative is a fluke, it is the r=1 case when k=2.
Lie algebras are a good thing
- No bimodule condition as Lie is skew-commutative.
- Unlike square matrix rings, a fixed simple Lie algebra can act faithfully and irreducibly on unbounded dimensions.
- Hence compression like this exists even with just 3-dimensional derivations!
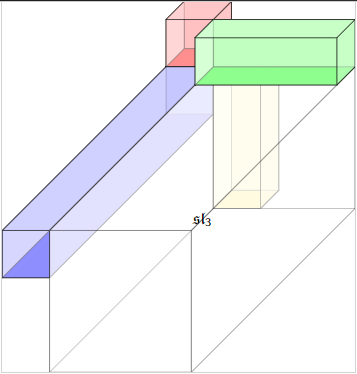
Orthogonalizing data
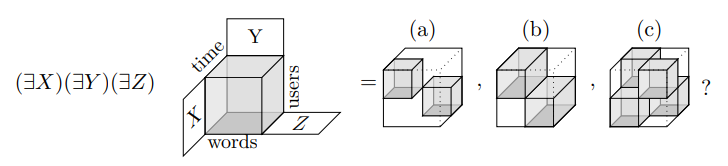
Problem posed in:
Acar, Camtepe, and Yener, Collective Sampling and Analysis of High Order Tensors for Chatroom Communications, Proc. 4th IEEE Int. Conf.Intel. and Sec. Info., 2006, pp. 213–224
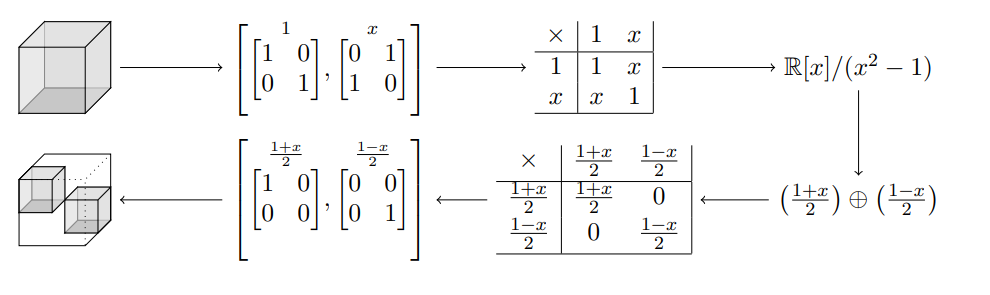
Orthogonalizing a tensor is an algebra problem.
Reality
The algebra is never there,
never that nice,
not even associative.
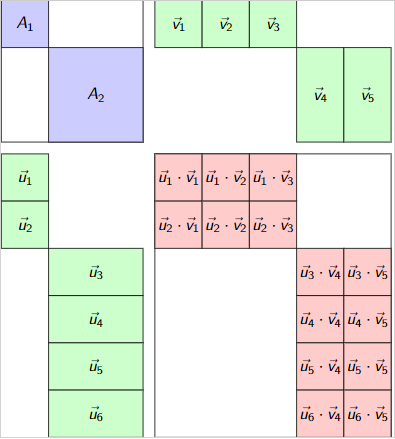
No algebra? Make one by enrichment!
Its decompositions do the job.
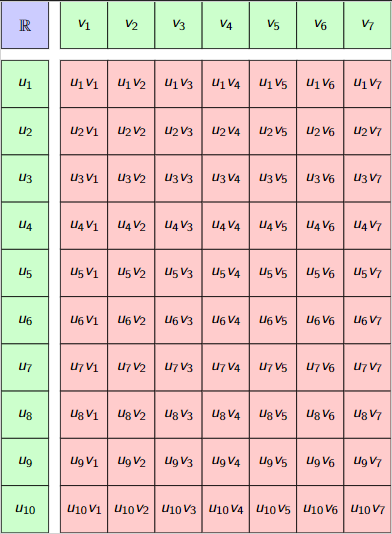
t∈R10⊗R7
Adj(t)≅R⊕M2(R) t∈R10⊗Adj(t)R7≅(R10⊗R⊕0R7)⊕(R10⊗0⊕M2(R)R7)=(R2⊗R3)⊕(R4⊗R2)
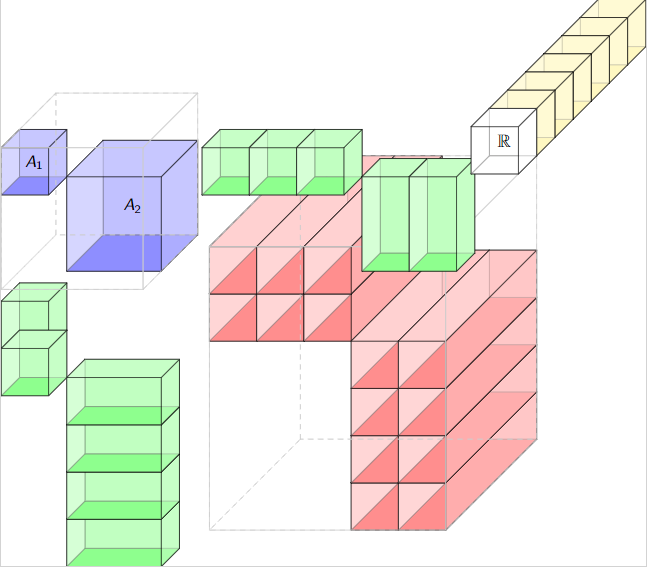
U⊗A1⊕A2V⊗W≅(U1⊗A1V1⊗W)⊕(U2⊕A2V2⊗W)
Orthogonalizes in higher valence
Did we get all decomposition types?
Thm. (FMW-Singular)
- Singularities types are in bijection with simplicial complexes Δ.
- The multi-spectrum of operators supported on singularities contain the Stanely-Reisner ideal (Xe∣supp(e)∈/Δ)
Valence 2
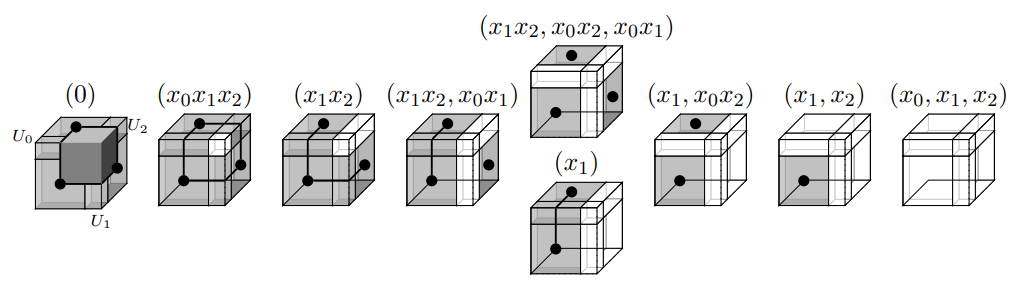
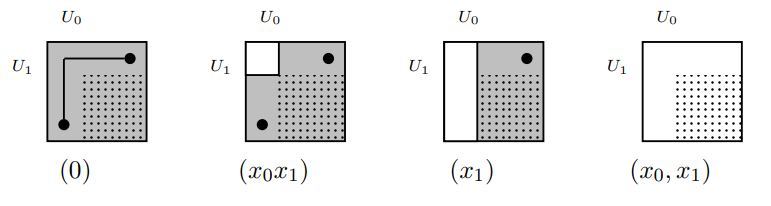
Valence 3
Theory & Practice
Parker-Norton 1975 MeatAxe: polynomial time algorithm for XTX−1=T1⊕⋯⊕Tℓ.
Performance: Dense 1/2 million dimensions in an hour, on desktop.
W. 2008: Proved uniqueness and polytime-algorithms for XTX†XTY=T1⊥⋯⊥Tℓ=T1⊕⋯⊕Tℓ
Generalizations being explored now.
Pros.
- Exact solution, no missing outliers, no need to train AI.
- Comes with uniqueness theorems (Jordan-Holder, Krull-Schmidt)
- Polynomial-time, in fact nearly linear time.
Cons.
- The algebra is tough (non-associative, hard modules) (...solution...hire algebraist...)
- Implementations are in Computer Algebra Systems (with increased funding this will change)
- Noise model is unexplored (Statisticians I've asked are more optimistic than me...hmm.)
Structure in Networks
Data credit to Frank W. Marrs III
Los Alamos National Labs
Further Fact of spectra:
If derivation and nilpotent then...
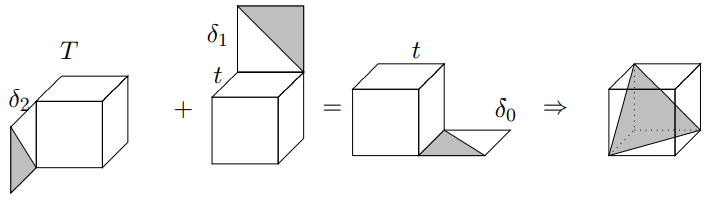
How to apply?
- Compute Der,
- Use Lie theory algorithms to locate such δ
- change coordinates to make data structured.
Actor Pair Exchange Conditions
Partners
Action/Reaction
Benafactor
Between pairs, 6 total (3 pictured)
⋮
Actor Pair Exchange on 7 actors
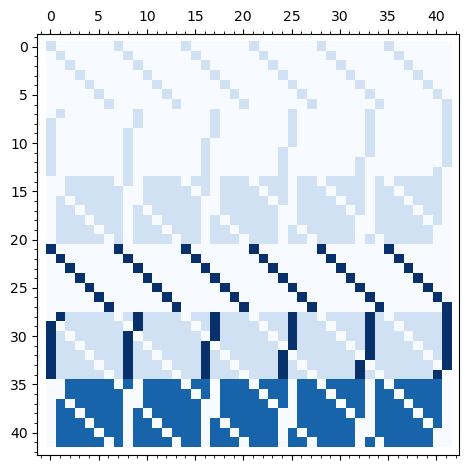
Tensor:
- Rows/Columns are pairs (a,b)
- each slice of tensor is a exchange pattern.
- Very combinatorial but 10,584 params.
- Calls for spectural "graph theory" but on hypergraphs
Actor Pair Exchange on 7 actors
Tensor:
- Algebras identify 2 outliers
- Cluster data into 4 layers (ideals) ⇒ breakup into 4 iterations
- Reduced to 250 parameters.
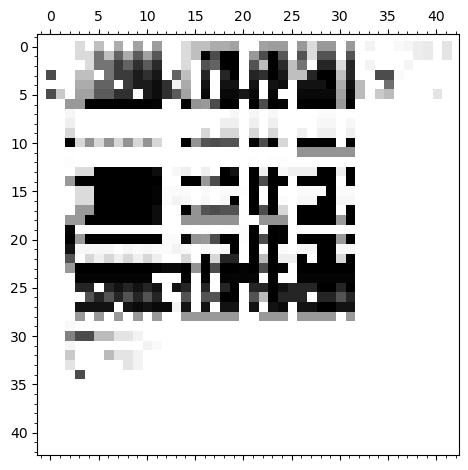
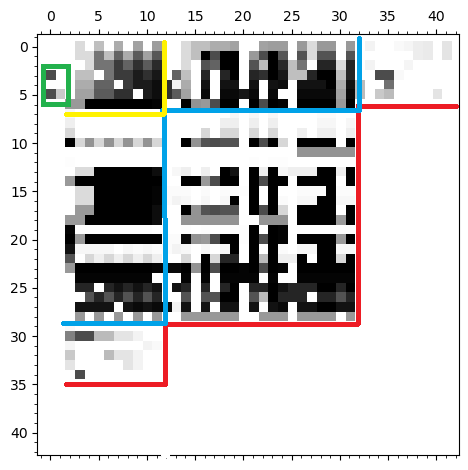
Entanglement Classes
Verstraete, Dehaene, De Moor, Verschelde, Four qubits can be entangled in nine different ways, Phys. Rev. A 65 (2002)
D. and B. Williamson,Mari¨en, Matrix product operators for symmetry-protected topological phases: Gauging and edge theories, Phys. Rev. B 94 (2016)

Quantum Particles modeled as vectors in Cd
Entangled Particles as in Cd1⊗⋯⊗Cdk
Visualize as n-gon.
Objective: What is the large-scale physics of a many body quantum material?
Comes down to symmetries of then tensors.


Valence 4?
What qualifies as a symmetry of a tensor? Not just anything...surprisingly combinatorial...
Valance 3
Yes
No.
Thm FMW-Groupoid.
Z(t,p)×={ω∣p in multi-spec t} is a group in some tensor category if, and only if, p=Xg(Xe−Xf) where e,f have disjoint support and are {0,1} valued.
Solution: chase the algebraic geometry of the spectra.... it turns out to be toric and thus combinatorial!
QuickSylver
Solving (∀i)(XAi+BiY=Ci) in nearly linear time
Derivations require Solving
(∀i)(XAi+BiY=Ci) and variations.
Naive:
Solving (∀i)(XAi+BiY=Ci) is linear in d2 variables so O(d2ω)⊂O(d6) work.
Good enough in theory, but hard to fit in memory and unrealistic at scale.
Bartels-Stewart Type Solution forXA+BY=C
- Choose E and F low rank matrices with pseudo-inverses E∗,F∗.
- Solve E(XA)F+E(BY)F=ECF which has lower dimension.
- Pullback solution using E∗,F∗.
Yields O(dω) time algorithms, ω≤3
Tensor Bartels-Stewart Solving(∀i)(XAi+BiY=Ci)?
- Choose [E] and [F] low rank tensors with pseudo-inverses [E]∗,[F]∗.
- Solve [E](X[A])[F]+[E]([B]Y)[F]=[E]C[F] which has lower dimension.
- OVERLAPS DESTROY EACH OTHER'S WORK

δA12(u⊗v⊗w)=u⊗v⊗w−u1ℓ=2∑eℓ⊗eℓAv⊗w
Prop. δA12∘δB13=δB13∘δA12
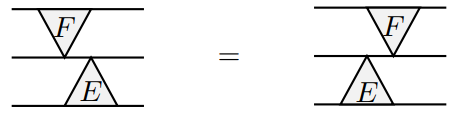
E=δB13 and F=δA12
Face Elimination: a tensor solution
Tensor Bartels-Stewart Solving(∀i)(XAi+BiY=Ci)?
- Choose [E] and [F] low rank tensors with pseudo-inverses [E]∗,[F]∗.
- Solve [E](X[A])[F]+[E]([B]Y)[F]=[E]C[F] which has lower dimension.
- OVERLAPS SLIDE PAST EACH OTHER


Thm Collery-Maglione-W.
QuickSylver solves simultaneous generalized Sylvester equations in time O(d3) (for 3-tensors).
Thank You!
Want details?
Several related videos/software/resources at
https://thetensor.space/
A recently updated version of some of the main results at
https://www.math.colostate.edu/~jwilson/papers/Densor-Final-arxiv.pdf
Denser Tensor Spaces
By James Wilson
Denser Tensor Spaces
Definitions and properties of tensors, tensor spaces, and their operators.
- 518