Thermodynamics
of structure-forming systems
in collaboration with
Simon D. Lindner Tuan Pham Rudolf Hanel Stefan Thurner
CSH Workshop Stochastic dynamics for complex systems
Slides available at: https://slides.com/jankorbel




Thermodynamics
of structure-forming systems
in collaboration with
Simon D. Lindner Tuan Pham Rudolf Hanel Stefan Thurner
based on a recently published paper: Nat. Comm. 12 (2021) 1127
Slides available at: https://slides.com/jankorbel
Workshop title: Stochastic dynamics for complex systems
Talk title: thermodynamics of structure-forming systems
(mostly thermoSTATISTICS)
I hope we will find a common language!
Little warning!
-
Historical review of thermodynamics
-
Main results of stochastic thermodynamics
-
Thermodynamics of structure-forming systems
-
Applications to formation of social groups
Outline
Thermodynamics
Microscopic systems
Classical mechanics (QM,...)
Mesoscopic systems
Stochastic thermodynamics
Macroscopic systems
Thermodynamics
Trajectory TD
Ensemble TD
Stochastic Thermodynamics is a thermodynamic theory
for mesoscopic, non-equilibrium physical systems
interacting with equilibrium thermal (and/or chemical)
reservoirs
Statistical mechanics
Historical review of thermodynamics
History
Equilibrium thermodynamics (19 th century)
- Maxwell, Boltzman, Planck, Claussius, Gibbs...
- Macroscopic systems (\(N \rightarrow \infty\)) in equilibrium (no time dependence of measurable quantities - thermoSTATICS)
- General structure of thermodynamics
- Laws of thermodynamics (general)
- Response coefficients (system-specific)
- Applications: engines, refridgerators, air-condition,...


efficiency \(\leq 1-\frac{T_2}{T_1}\)
Heat engine: Carnot cycle

Car engines: 30-50%
History
Laws of thermodynamics
Zeroth law:
Temperature can be measured. $$T_A = T_B \quad \mathrm{if} \quad A \ \mathrm{and} \ B \ \mathrm{are} \ \mathrm{in} \ \mathrm{equilibrium}.$$
First law (Claussius 1850, Helmholtz 1847):
Energy is conserved.
$${\color{aqua} d}U = {\color{orange} \delta} Q - {\color{orange} \delta} W$$ Second law (Carnot 1824, Claussius 1854, Kelvin):
Heat cannot be fully transformed into work. $${ \color{aqua} d} S \geq \frac{{\color{orange} \delta} Q}{T}$$ Third law: We cannot bring the system into the absolute zero
temperature in a finite number of steps. $$ \lim_{T \rightarrow 0} S(T) = 0$$
History
Local equilibrium thermodynamics (1st half of 20th cent.)
- Onsager, Rayleigh...
- Systems close to equilibrium - linear response theory
- Local equilibrium: subsystems a,b,c are each in equilibrium

Total entropy \(S \approx S^a + S^b + S^c + \dots\)
Entropy production \(\sigma^a = \frac{d S^a}{d t} = \sum_i Y_i^a J_i^a \)
\(Y_i^a\) - thermodynamic forces; \(J_i^a\) - thermodynamic currents
4th Law of thermodynamics (Onsager 1931): \( \sigma = \sum_{ij} L_{ij} \Gamma_i \Gamma_j\)
\(\Gamma_i = Y_i^a - Y_i^b \) - afinity, \(L_{ij}\) - symmetric
History and now
Stochastic thermodynamics (90s of 20th century - present)
- Evans, Jarzynski, Crooks, Seifert, van den Broek,....
- Mesoscopic systems far from equilibrium
- Combines stochastic calculus and non-equilibrium thermodynamics
- Main results: Trajectory thermodynamics, Fluctuation theorems, Thermodynamic uncertainty relations, Speed limit theorems,...
- Applications: colloidal particles and soft matter, biochemistry, molecular motors



Molecular motor: myosin walking on actin filament

efficiency \(\lesssim 1\)
Main results of stochastic thermodynamics
Stochastic thermodynamics
1.) Consider linear Markov (= memoryless) with distribution \(p_i(t)\).
Its evolution is described by master equation
$$ \dot{p}_i(t) = \sum_{j} [w_{ij} p_{j}(t) - w_{ji} p_i(t) ]$$
\(w_{ij}\) is transition rate.
2.) Entropy of the system - Shannon entropy \(S(P) = - \sum_i p_i \log p_i\). Equilibrium distribution is obtained by maximization of \(S(P)\) under the constraint of average energy \( U(P) = \sum_i p_i \epsilon_i \)
$$ p_i^{eq} = \frac{1}{Z} \exp(- \beta \epsilon_i) \quad \mathrm{where} \ \beta=\frac{1}{k_B T}, Z = \sum_j \exp(-\beta \epsilon_j)$$
Stochastic thermodynamics
3.) Detailed balance - stationary state (\(\dot{p}_i = 0\) ) coincides with the equilibrium state (\(p_i^{eq}\)). We obtain
$$\frac{w_{ij}}{w_{ji}} = \frac{p_i^{eq}}{p_j^{eq}} = e^{\beta(\epsilon_j - \epsilon_i)}$$
4.) Second law of thermodynamics:
$$\dot{S} = - \sum_i \dot{p}_i \log p_i = \frac{1}{2} \sum_{ij} (w_{ij} p_j - w_{ji} p_i) \log \frac{p_j}{p_i}$$
$$ =\underbrace{\frac{1}{2} \sum_{ij} (w_{ij} p_j - w_{ji} p_i) \log \frac{w_{ij} p_j}{w_{ji} p_i}}_{\dot{S}_i} + \underbrace{\frac{1}{2} \sum_{ij} (w_{ij} p_j - w_{ji} p_i) \log \frac{w_{ji}}{w_{ij}}}_{\dot{S}_e}$$
\( \dot{S}_i \geq 0 \) - entropy production rate (2nd law of TD)
\(\dot{S}_e = \beta \dot{Q}\) entropy flow rate
Stochastic thermodynamics
5.) Trajectory thermodynamics - consider stochastic trajectory
\(x(t)= (x_0,t_0;x_1,t_1;\dots)\). Energy \(E_x = E_x(\lambda(t))\), \(\lambda(t)\) - control protocol

Probability of observing \( x(t)\): \(\mathcal{P}(x(t)\))
Time reversal \(\tilde{x}(t) = x(T-t)\)
Reversed protocol \(\tilde{\lambda}(t) = \lambda(T-t)\)
Probability of observing reversed trajectory under reversed protocol \(\tilde{\mathcal{P}}(\tilde{x}(t))\)
Stochastic thermodynamics
6.) Fluctuation theorems
Trajectory entropy: \(s(t) = - \log p_x(t)\)
Trajectory 2nd law \(\Delta s = \Delta s_i + \Delta s_e\)
Relation to the trajectory probabilities
$$\log \frac{\mathcal{P}(x(t))}{\tilde{\mathcal{P}}(\tilde{x}(t))} = \Delta s_i$$
Detailed fluctuation theorem
$$\frac{P(\Delta s_i)}{\tilde{P}(-\Delta s_i)} = e^{\Delta s_i}$$
Integrated fluctuation theorem $$ \langle e^{- \Delta s_i} \rangle = 1 \quad \Rightarrow \langle \Delta s_i \rangle = \Delta S_i \geq 0$$

Thermodynamics of structure-forming systems
Motivation
- Many systems form structures: molecules of atoms, clusters of colloidal particles, (bio)polymers or micelles
- We study the thermodynamics of structure-forming systems
- For small systems, we get a correction to Shannon entropy
- We apply the results to several physical systems
- We derive fluctuation theorems for structure-forming systems
Toy model - magnetic coin model
We consider a coin with two states: head and tail
The coins are magnetic and can stick together
How many states we get for N coins?

\(W(N) \sim N^N\)
(non-magnetic coins \(W(N) = 2^N\))



picture taken from: H. J. Jensen et al 2018 J. Phys. A: Math. Theor. 51 375002
Multiplicity and entropy
of structure-forming systems
Boltzmann entropy formula: \(S(n_i) = k_B \log W(n_i)\)
where \(W\) is multiplicity
(number of microstates corresponding to a mesostate \(n_i\))
Microstate: state of each particle
if more particles are bound to a molecule, then state of each molecule
Mesostate: how many particles and/or molecules are in given state
Example: magnetic coin model: 3 coins, magnetic
microstates mesostate multiplicity






2 x 1x
1 x 1x
3
3




How to calculate a multiplicity?
- Consider a mesostate
- Make all permutations of particles
- Some microstates are overrepresented - calculate how many permutations belong to the same microstate
Examples
2 x 1x
1 x 1x










1 1 2 2 3 3
2 3 1 3 1 2
3 2 3 1 2 1
1 1 2 2 3 3
2 3 1 3 1 2
3 2 3 1 2 1



= (1,2,3) , (2,1,3)
= (1,3,2) , (3,1,2)
= (2,3,1) , (3,2,1)



= (1,2,3) , (1,3,2)
= (2,1,3) , (2,3,1)
= (3,1,2) , (3,2,1)
General formula for multiplicity

General formula: \(W(n_i^{(j)}) = \frac{n!}{\prod_{ij} n_i^{(j)}! {\color{aqua} (j!)^{n_i^{(j)}}}}\)
we have \(n_i^{(j)}\) molecules of size \(j\) in a state \(s_i^{(j)}\)



Boltzmann's 1884 paper

Boltzmann's gravestone at Vienna Zentralfriedhof
\( S = k \cdot \log W\)
Entropy of structure-forming systems
$$ S = \log W \approx n \log n - \sum_{ij} \left(n_i^{(j)} \log n_i^{(j)} - n_i^{(j)} + {\color{aqua} n_i^{(j)} \log j!}\right)$$
Introduce "probabilities" \(\wp_i^{(j)} = n_i^{(j)}/n\)
$$\mathcal{S} = S/n = - \sum_{ij} \wp_i^{(j)} (\log \wp_i^{(j)} {\color{aqua}- 1}) {\color{aqua}- \sum_{ij} \wp_i^{(j)}\log \frac{j!}{n^{j-1}}}$$
Finite interaction range: concentration \(c = n/b\)
$$\mathcal{S} = S/n = - \sum_{ij} \wp_i^{(j)} (\log \wp_i^{(j)} {\color{aqua}- 1}) {\color{aqua}- \sum_{ij} \wp_i^{(j)}\log \frac{j!}{{\color{orange}c^{j-1}}}}$$
Equilibrium distribution:
$$\hat{\wp}_i^{(j)} = \frac{c^{j-1}}{j!} \exp(-\alpha j - \beta \epsilon_i^{(j)})$$
normalization by solving
\(\sum_{ij} j \wp_i^{(j)} = \sum_{ij} \frac{c^{j-1}}{(j-1)!} e^{-{\color{aqua} \alpha} j - \beta \epsilon_i^{(j)}} = 1\) for \({\color{aqua} \alpha}\)
Entropy of structure-forming systems
Main properties:
- The entropy fulfills Shannon Khinchin axioms 1,3,4 but does not fulfill axiom SK 2 (it is not maximized by uniform distribution)
- The entropy fulfills Lieb-Yngvason axioms (it is additive, and it is extensive for \(c=const\) )
- The entropy fulfills Shore-Johnson axioms 1,3,4 but does not fulfill axioms SJ 2 (permutation/coordinate invariance)
- The entropy fulfills Tempesta group-composability axiom but is not symmetric in its arguments
- The scaling exponents according to Hanel-Thurner axioms are \(c=0,d=1\), the same as for Shannon entropy
\( \Rightarrow\) The entropy satisfies all common axiomatic schemes but it is not symmetric in probabilities

Comparison with Grand-canonical ensemble
Stochastic thermodynamics of structure-forming systems
1. Linear Markov (= memoryless) with distribution \(\wp_i(t)\).
Its evolution is described by master equation
$$ \dot{\wp}_i(t) = \sum_{j} [w_{ij} \wp_{j}(t) - w_{ji} \wp_i(t) ]$$
\(w_{ij}\) is transition rate.
2. Detailed balance
$$\frac{{w}_{ik}^{jl}}{{w}_{ki}^{lj}}= \frac{\hat{\wp}_i^{(j)}}{\hat{\wp}_{k}^{(l)}} = {\color{aqua}\frac{j!}{l!}{c}^{l-j}}\exp \left[{\color{aqua}\alpha (l-j)}+\beta \left({\epsilon }_{k}^{(l)}-{\epsilon }_{i}^{(j)}\right)\right]$$ |
Assumptions
Stochastic thermodynamics of structure-forming systems
Results
1. Second law of thermodynamics for non-equilibrium systems
$$\frac{{\rm{d}}{\mathcal{S}}}{{\rm{d}}t}={\dot{{\mathcal{S}}}}_{i}+\beta \dot{{\mathcal{Q}}}$$ where \(\dot{\mathcal{S}}_i \geq 0\) is entropy production flow and \(\dot{\mathcal{Q}}\) is the heat flow |
2. Detailed fluctuation theorem for structure forming systems
$$\frac{P(\Delta \sigma)}{\tilde{P}(-\Delta \sigma)} = e^{\Delta \sigma}$$
where \(\Delta \sigma = \Delta s_i + {\color{aqua} \log j_0 - \log j_f}\)
\(\Delta s_i\) is the trajectory entropy production
Applications to physics
Self-assembly of Janus particles



Kern-Frenkel model
Pair-wise potential: \(U^{KF}(r_{ij},n_i,n_j) = u(r_{ij}) \Omega(r_{ij},n_i,n_j) \)
Square-well interaction with hard sphere:
$$ u(r_{ij}) = \left\{ \begin{array}{rl} \infty, & r_{ij} \leq \sigma \\ - \epsilon, & \sigma < r_{ij} < \sigma + \Delta \\ 0, & r_{ij} > \sigma + \Delta. \end{array} \right.$$
\(\Omega\) decribes orientation of particles:
Particle coverage \(\chi = \sin^2(\theta/2) = \frac{1-\cos{\theta}}{2}\)
Polymers: \(\chi = 0.3\)
Janus particles: \(\chi = 0.5\)
Crystalic structures: \(\chi = 0.6\) (stable lamellar crystals)
$$\Omega(r_{ij},n_i,n_j) = \left\{\begin{array}{rl} -1 & \mathrm{if} \ r_{ij} \cdot n_i > \cos(\theta) \ \mathrm{and} \ r_{ij} \cdot n_j > \cos(\theta)\\ 0 & \mathrm{otherwise} \end{array} \right.$$

Phase diagram of Janus particles for average cluster size \(M\)
Currie-Weiss model with molecules
(= fully connected Ising model with bound states)
$$ H(s_i) = - \frac{J}{n-1} \sum_{i \neq j, \ free} s_i s_j - h \sum_{j, \ free} s_j $$

Applications to
group formation

Homophily in social networks
Two individuals are friends if they have more similar opinions
Two individuals are enemies if they have more different opinions
Group formation based on homophily
Hamiltonian of a group \(\mathcal{G}\)
\(H(\mathbf{s}_{i_1},\dots,\mathbf{s}_{i_k}) = \underbrace{- \phi \, \frac{J}{2} \sum_{ij \in \mathcal{G}} A_{ij} \mathbf{s}_i \cdot \mathbf{s}_j}_{\textcolor{red}{intra-group \ social \ stress}}+ \underbrace{(1-\phi) \frac{J}{2} \sum_{i \in \mathcal{G}, j \notin \mathcal{G}} A_{ij} \mathbf{s}_{i} \cdot \mathbf{s}_j}_{\textcolor{blue}{inter-group \ social \ stress}} \\ \qquad \qquad \qquad \qquad - \underbrace{h \sum_{i \in \mathcal{G}} \mathbf{s}_i \cdot \mathbf{w}}_{external \ field}\)
Group formation based on opinion= self-assembly of spin glass
Group 1
Group 2

Phase diagram of group size

Theory
MC simulation
Dependence on external field
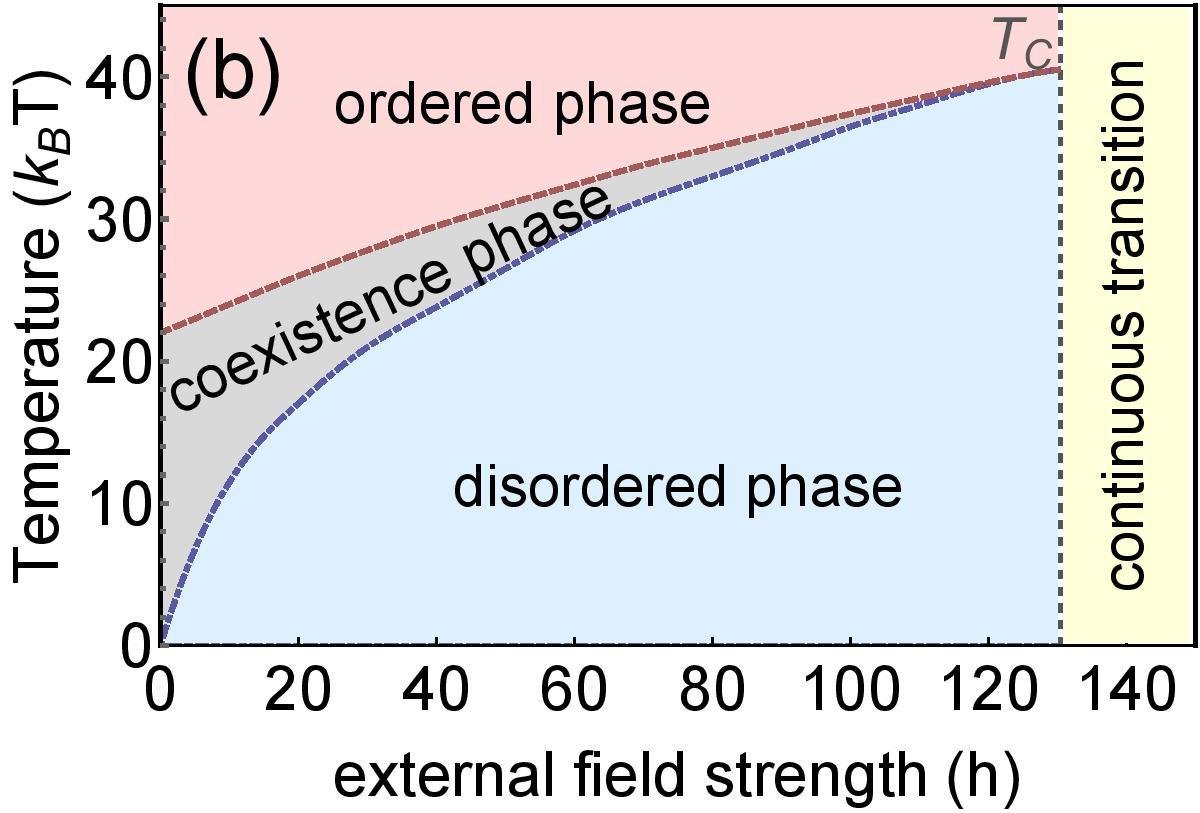


Application to real example of a massive online multiplayer game (PARDUS)
Summary
More details in: J. K., S. D. L., R. H. and S. T., Nat. Comm. 12 (2021) 1127
- We derived the formula for entropy of structure-forming systems
- For large systems and low concentrations, it is equivalent to the grand-canonical ensemble
- We derived second law of thermodynamics and detailed fluctuation theorem for structure-forming systems arbitrarily far from equilibrium
- We showed several applications in self-assembly Currie-Weis model with molecule states, or group formation of social groups
Thermodynamics of structure-forming systems
By Jan Korbel
Thermodynamics of structure-forming systems
- 94