LLMs selbst betreiben
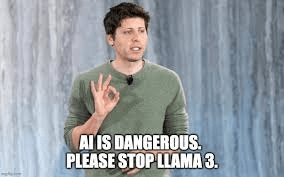
Warum
überhaupt?
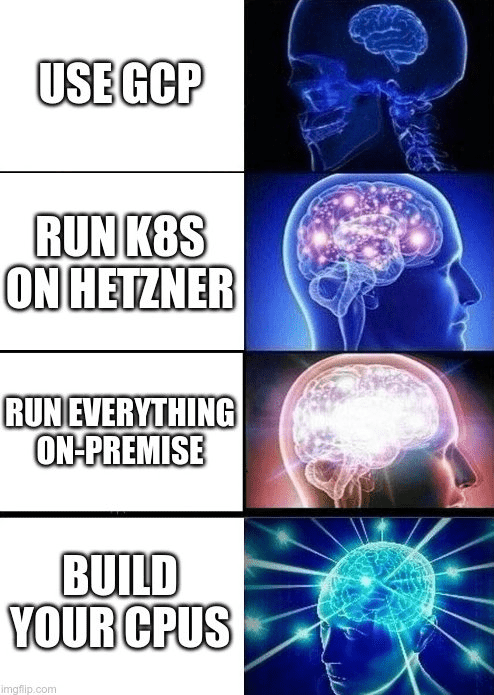
SLM - Small Language
Models
- Zugänglichkeit: jeder kann sie verwenden
-
Anpassbarkeit: selbst trainierbar
-
Spezialisierung: Gut für spezielle Aufgaben
- RAG
- Summarization
- Tool Calling & Agent Reasoning
- Bei hohem Read/Write-Quotienten statt RAG
- Ressourcenbedarf : Training und Inferenz
- Kosten & Sustainability
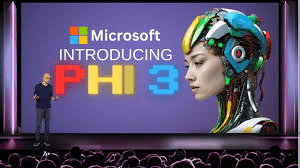
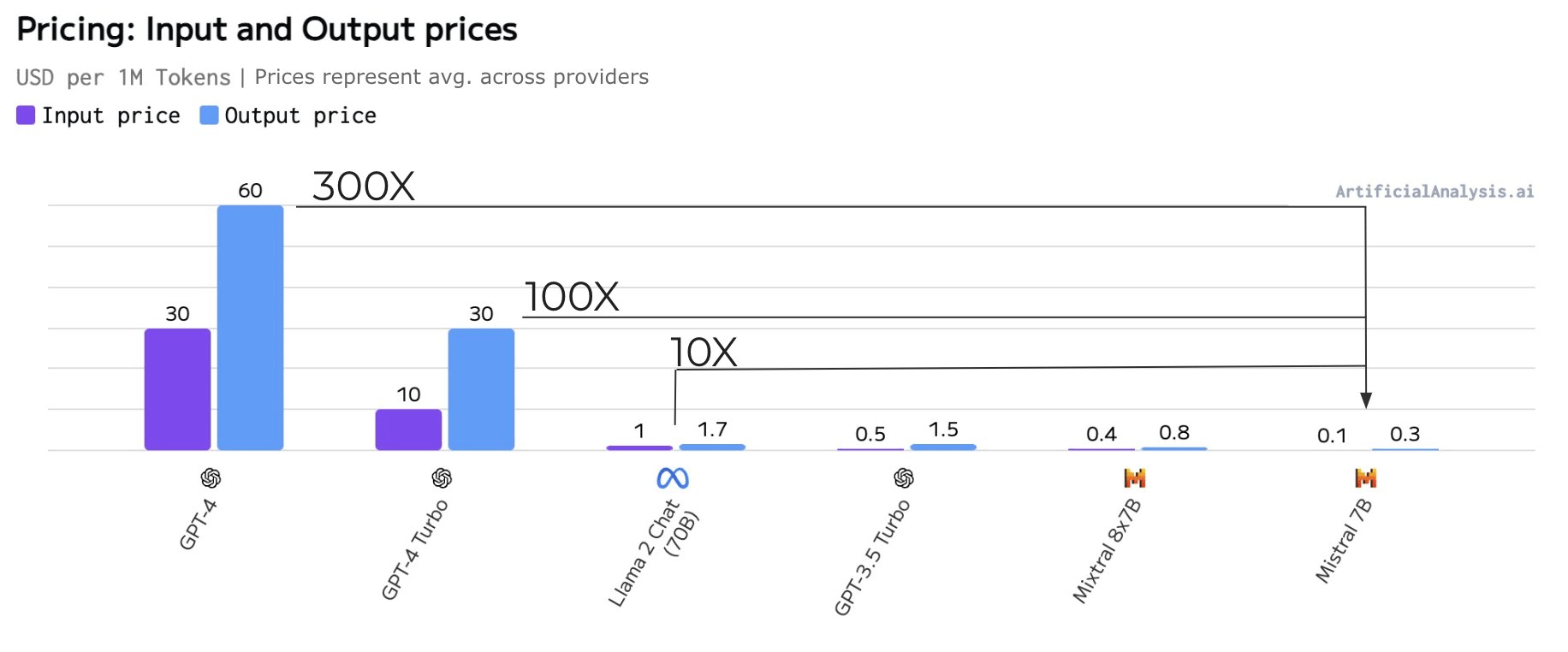
Ressourcenbedarf in $
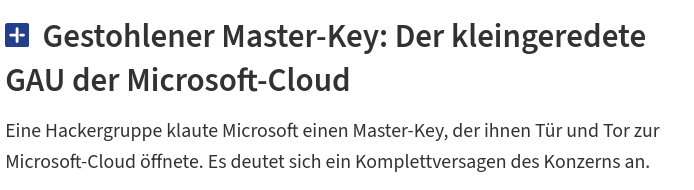
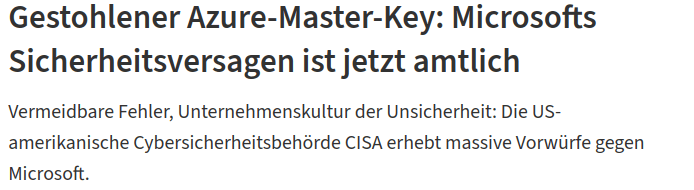
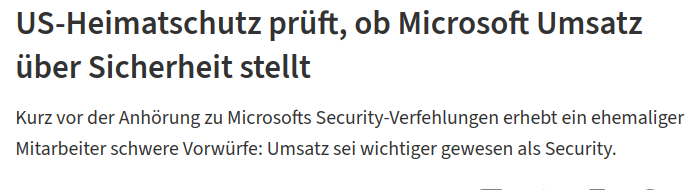
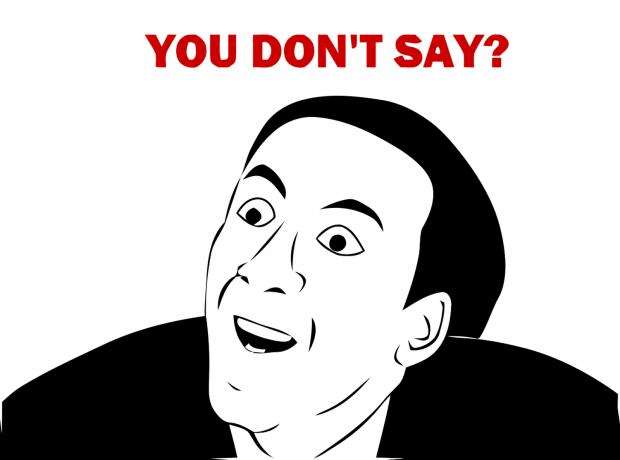
Privacy & Security
- Microsoft ist so mittel in Cloud Security
- Offline Access
- Datensouveränität
- Unabhängigkeit
- Vendor Control
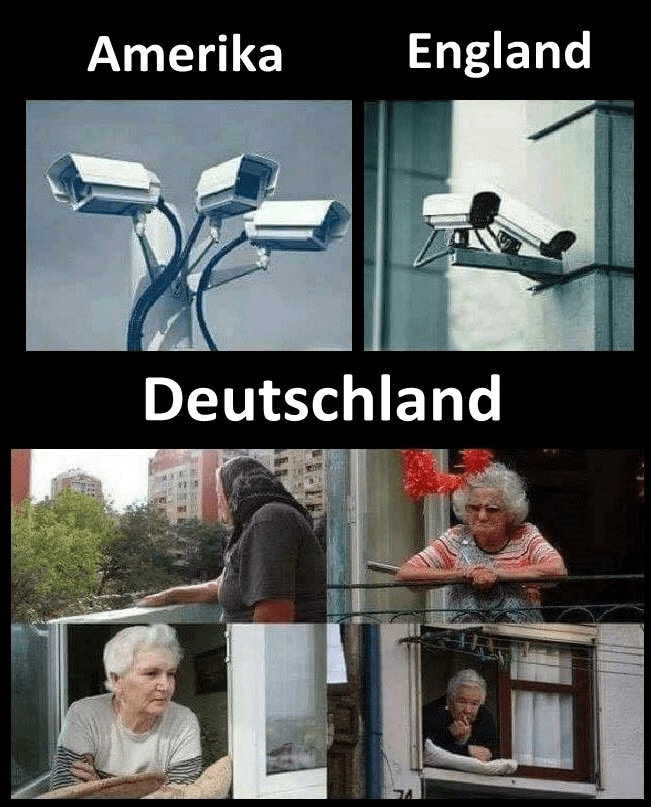
Wann sollte ich
das nicht tun?
Wenn ich die DSGVo
einhalten will.
Praktisch jeder grössere LLM-SAAS bietet inzwischen GDPR-compliant an.
Weil ich möchte, dass auf meinen Daten nicht trainiert wird.
Das ist in praktisch allen kostenpflichtigen Angeboten konfigurierbar.
Weil ich PII-Daten
verarbeite.
Die meisten größeren LLM-Libraries bieten reversible data anonymization an.
Sind offene oder proprietäre LLMs besser?
LMSYS Chatbot Arena Leaderboard
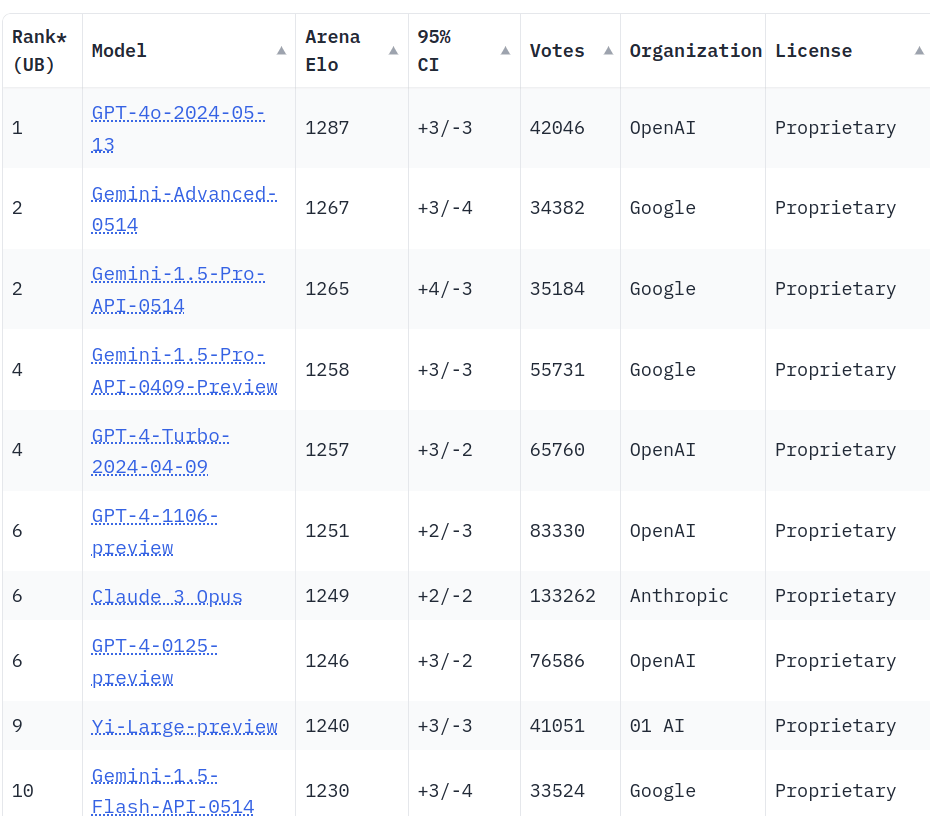
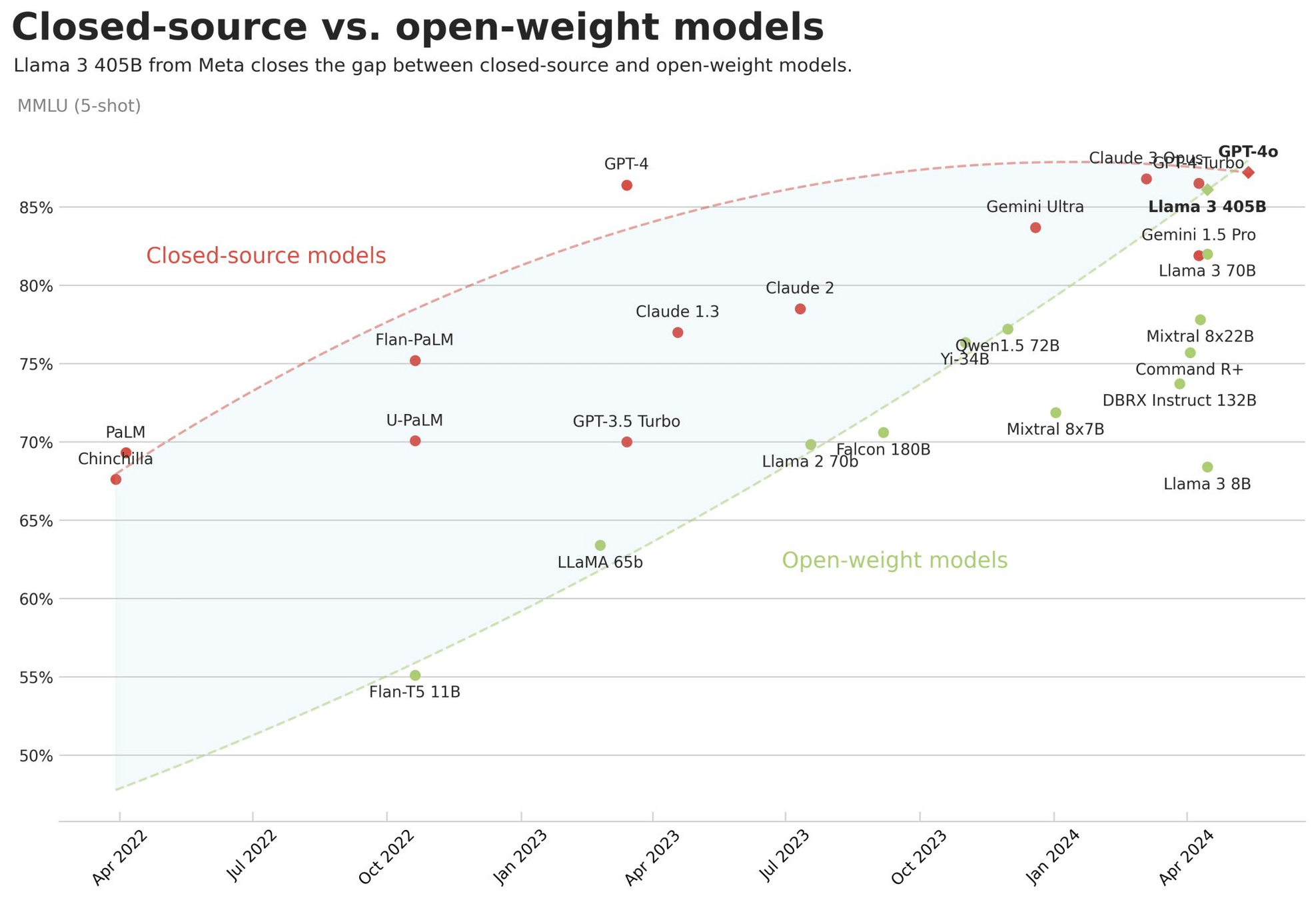
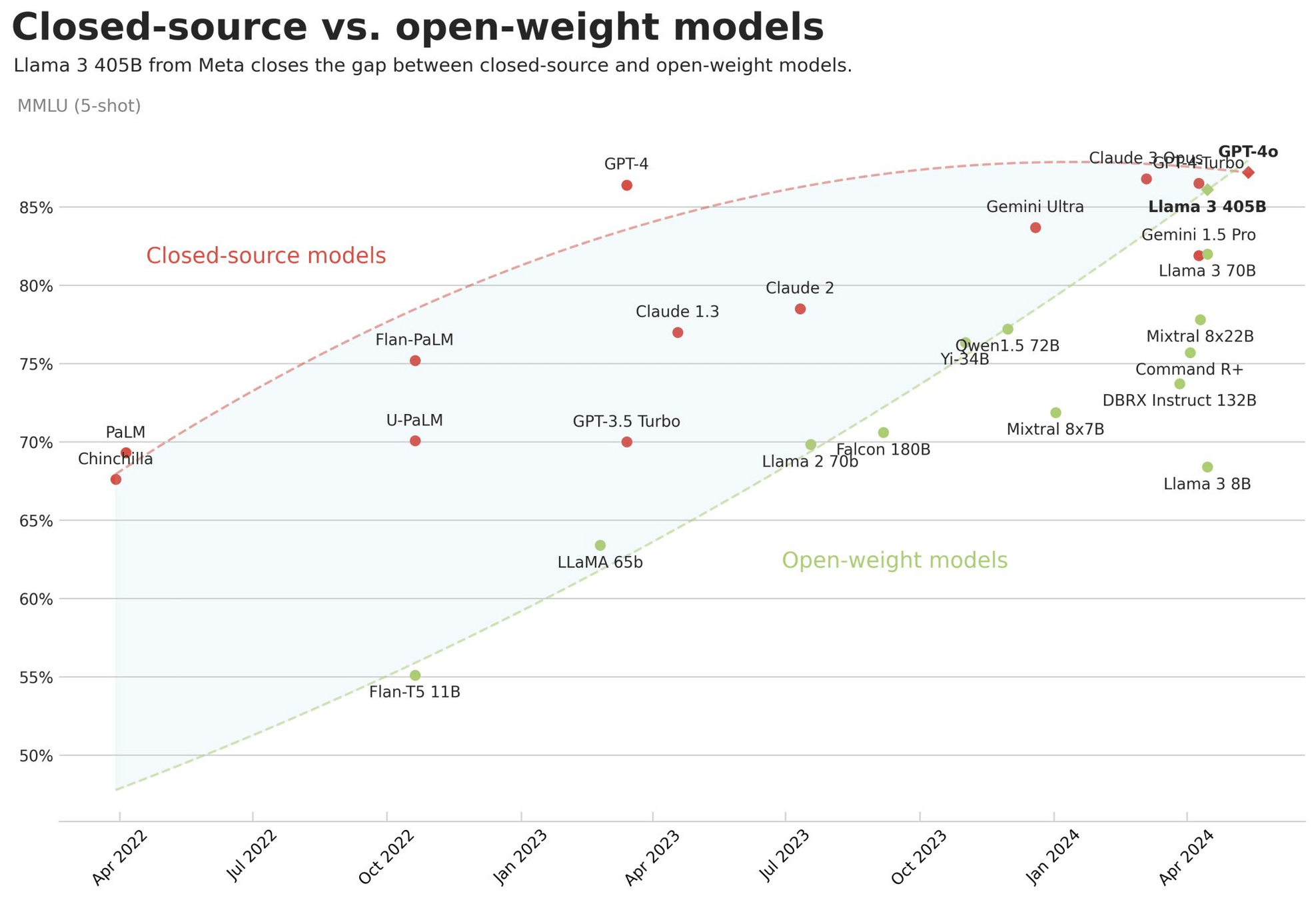
https://huggingface.co/spaces/lmsys/chatbot-arena-leaderboard
GPT-4o | 1287 | ? |
Llama 3 70B-Instruct | 1207 | 70 |
Qwen-2-72B | 1187 | 72 |
Qwen1.5-110B-Chat | 1162 | 110 |
GPT-4-0613 | 1161 | 440 |
YI-1.5-34B-Chat | 1160 | 34 |
Llama-3-8B-Instruct | 1153 | 8 |
Mixtral-8x22b-instruct | 1146 | 44 |
Phi-3-Medium-4K-Instruct | 1122 | 14 |
Starling-LM-7B-Beta | 1119 | 7 |
Mixtral-8*7B-Instruct | 1114 | 16 |
GPT-3.5-Turbo-0314 | 1106 | 178 |
Die offenen Top-Modelle laut Chatbot Arena
https://huggingface.co/spaces/open-llm-leaderboard/open_llm_leaderboard
Model | Average | Size |
---|---|---|
Rhea-72B-v0.5 | 79,15 | 72 |
Mixtral-8x22B | 79,15 | 44 |
Llama3-70B-Instruct-DPO-v0.2 | 78,69 | 72 |
luxia-21.4b-alignment-v1.2 | 78,14 | 21,4 |
MixTAO-7Bx2 | 77,5 | 12,8 |
UNA-SimpleSmaug-34b-v1beta | 77,41 | 34b |
Mistral-7B-Finetunes | 76,67 | 7B |
Die offenen Top-Modelle laut Huggingface-Benchmarks
https://huggingface.co/spaces/open-llm-leaderboard/open_llm_leaderboard
Model | Average | Size |
---|---|---|
Rhea-72B-v0.5 | 79,15 | 72 |
Mixtral-8x22B | 79,15 | 44 |
Llama3-70B-Instruct-DPO-v0.2 | 78,69 | 72 |
luxia-21.4b-alignment-v1.2 | 78,14 | 21,4 |
MixTAO-7Bx2 | 77,5 | 12,8 |
UNA-SimpleSmaug-34b-v1beta | 77,41 | 34b |
Mistral-7B-Finetunes | 76,67 | 7B |
Die offenen Top-Modelle laut Huggingface-Benchmarks
Modelauswahl
- Filtern und Sortieren
- verschiedene Benchmarks
- Größe
- Mehrsprachig:
https://huggingface.co/spaces/uonlp/open_multilingual_llm_leaderboard
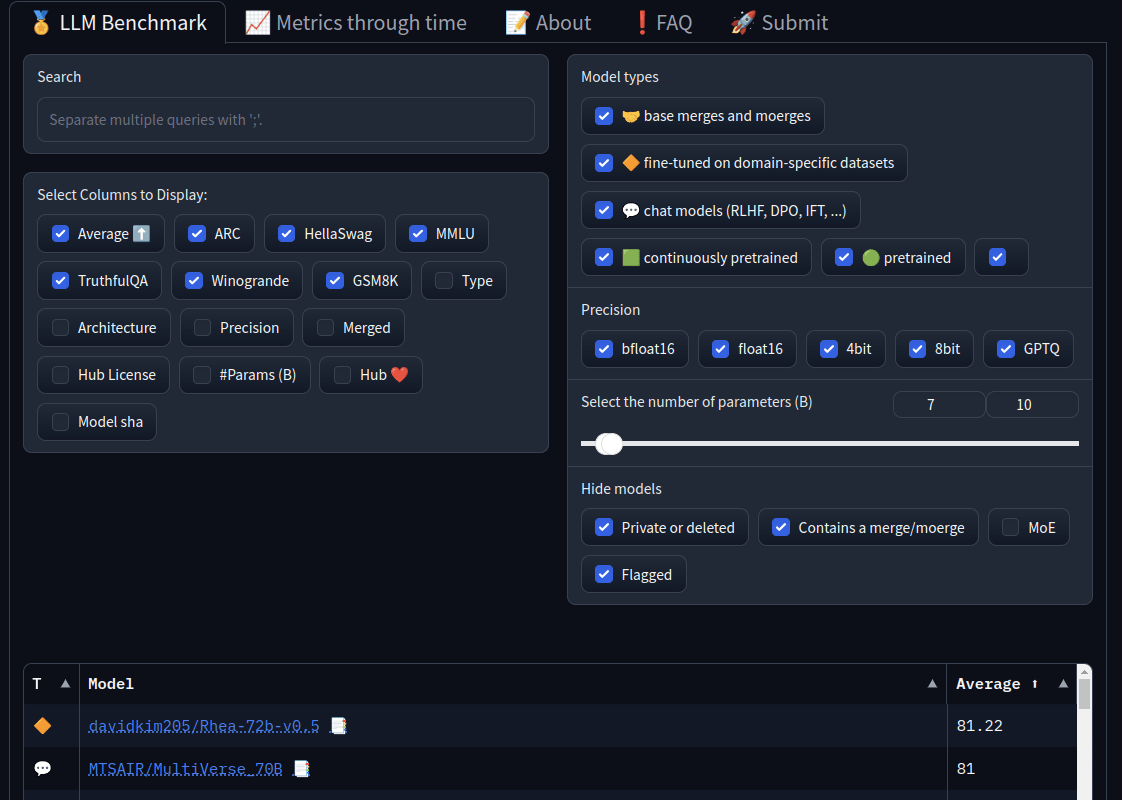
Speicherbedarf
https://llm-system-requirements.streamlit.app/
Faktor | Beschreibung | Größe |
---|---|---|
Parameterzahl | Das eigentliche Model | 7B |
torch.dtype | Prezision der Parameter - fp32, bf16, int4, .. | 29,8GB |
KVCache | Key-Value-Cache für die bisherigen Token für die Berechnung der Attention | 2GB |
Activation Memory | "Zwischenergebnisse" jedes Layers im Forward | 3,56GB |
Inference | 35,4GB | |
Optimizer Memory | Die Lernzustände im Training | 59,6GB |
Gradients Memory | Gradienten für die Backward propagation | 29,8GB |
Training | 124,8GB |
48GB GPU, um
3 parallele Anfragen auf einem 8B-Modell zu liefern?
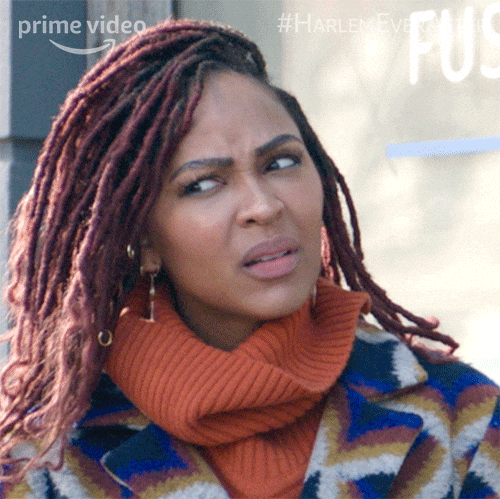
Zwei 80GB-Karten, um mit Batch-Size 6 zu trainieren?
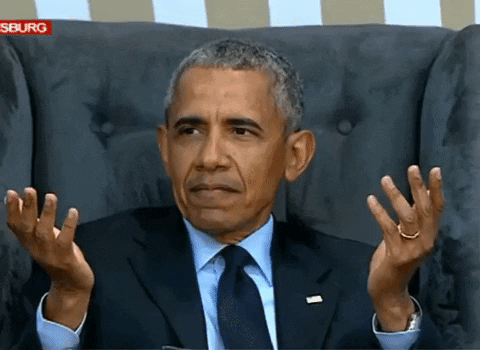
Quantization
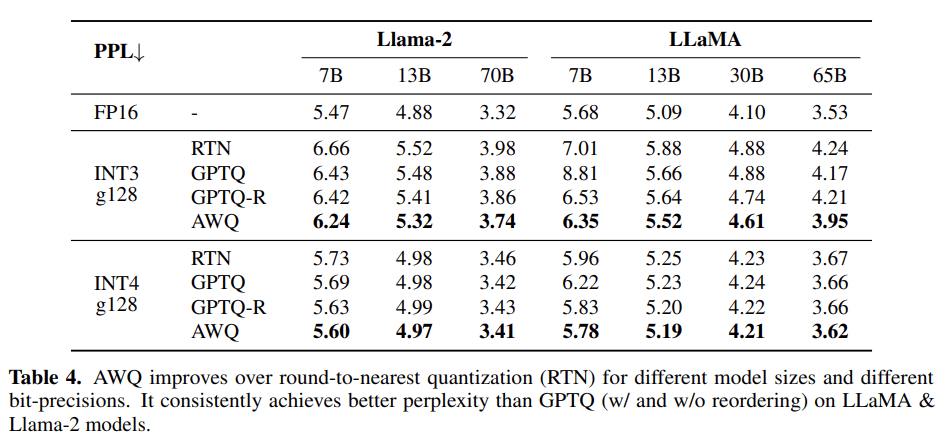
GPTQ, AWQ, GGUF, EXL2 ....
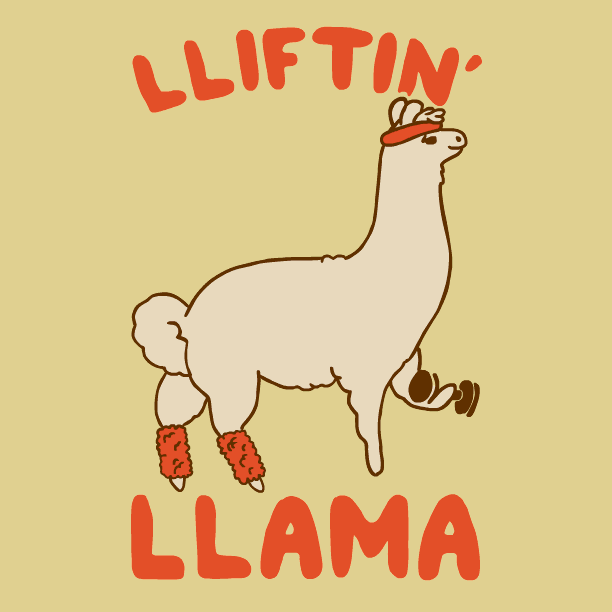
Speicherbedarf mit Quantisierung
https://llm-system-requirements.streamlit.app/
Faktor | Beschreibung | Größe |
---|---|---|
Parameterzahl | Das eigentliche Model | 7B |
torch.dtype | Prezision der Parameter - fp32, bf16, int4, .. | 3,73GB |
KVCache | Key-Value-Cache für die bisherigen Token für die Berechnung der Attention | 0,3GB |
Activation Memory | "Zwischenergebnisse" jedes Layers im Forward | 3,56GB |
Inference | 7,54GB |
Uh, das kann ich ja sogar auf dem Rechner aller Kollegen laufen lassen.
Ja, genau.
LLMing on the Edge: LLamaCPP
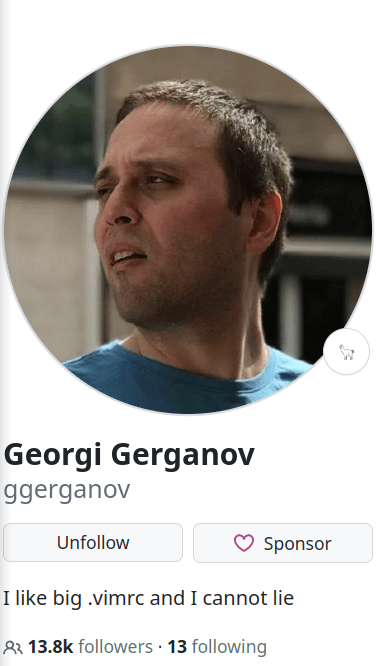
Warum muss man eine GPU haben, wenn man C++ kann?
- 2022: GGML-C-Library für GPT-Inference auf normaler Hardware
- 2023: auf er Basis llama.cpp
- Support für Apple, ARM, Accelerate,
AVX1-512, 1.5-8 bit, Cuda, AMD GPU, - Hybrid & Offloading
- Support für Multimodal
- Built-In Webserver mit OpenAi-API
- ollama basiert auf llama.cpp
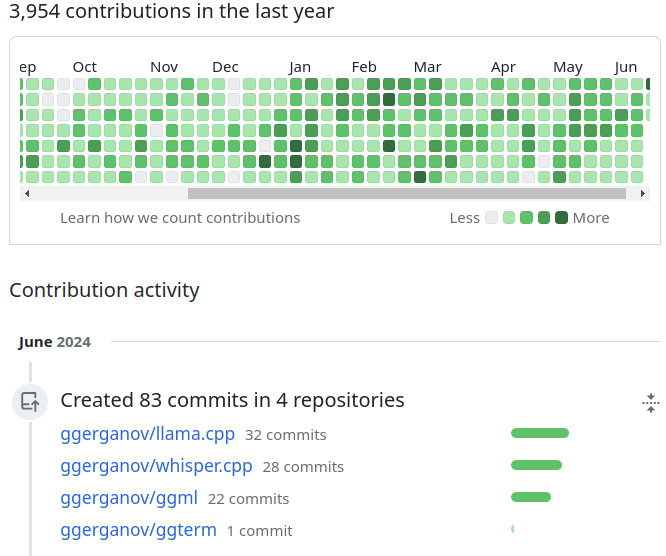
curl -fsSL https://ollama.com/install.sh | sh ollama run llama3 curl -X POST http://localhost:11434/api/generate -d '{ "model": "llama3", "prompt":"Why is the sky blue?" }'
ollama on the Edge
exLlamav2
- Von @turboderp
- Focus auf Consumer GPUs
- 7B-Modell mit 207 token/s auf einer 4090
- OpenAI-Style-API mit TabbyAPI
🤗 Transformers
- Huggingface Transformers lokal
- für in-code-inference
- AWQ, GPTQ-Support
- On-the-Fly-Quantisierung über BitsAndBytes
- Flash-attention für bessere Speichernutzung
Apple MLX
- Apples Antwort auf llama.cpp
- Teil der "Apple Intelligence" Strategie
- Optimiert auf die eigene Hardware
- Training und Inference
- Quantisierung in 4- und 8bit
- 891 Models auf Huggingface
Microsoft CoPilot+
- Teil des Microsoft CoPilot Plus PCs
- Eingebettet in die AI-Strategie
- NPUs von Qualcomm mit Apple-vergleichbarer Performance
- lokale LLMs, MLLMs, TTS, SST und vieles mehr
- DirectLM, ONNX, PyTorch uvm
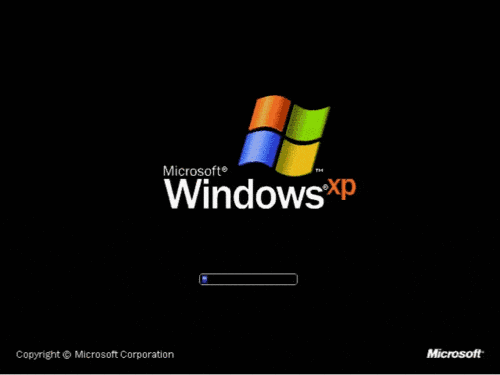
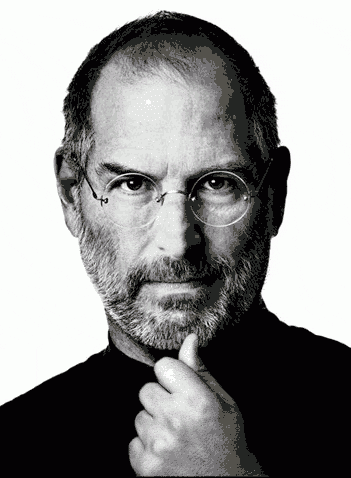
Ok, lokal schön und gut,
aber ich meinte jetzt zentral gesteuert. Durch mich.
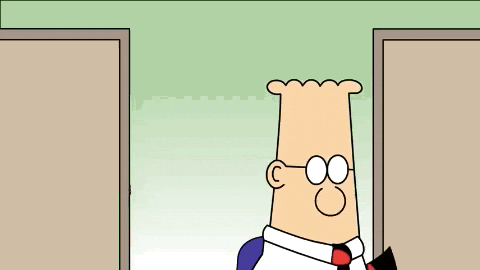
On Premises: 🤗 TGI
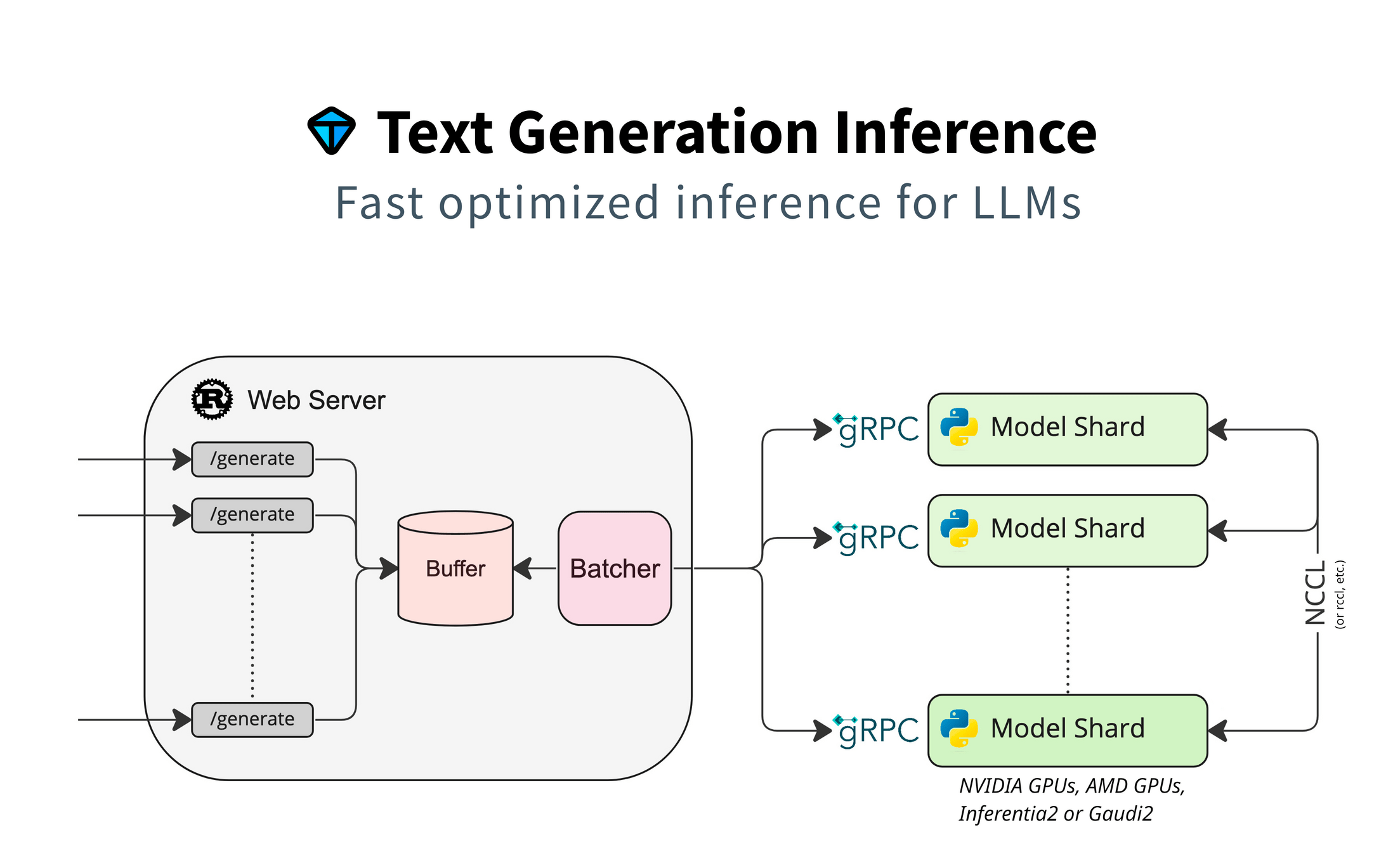
On Premises: 🤗 TGI
-
Production Ready
-
OTel & Prometheus Support
- Zwischendrin eine seltsame Lizenz, jetzt aber wieder gut
-
Nvidia, AMD, Intel Gaudi, AWS Inferentia
-
Docker von HF, Helm Charts verfügbar
- OpenAI-kompatible Messages API
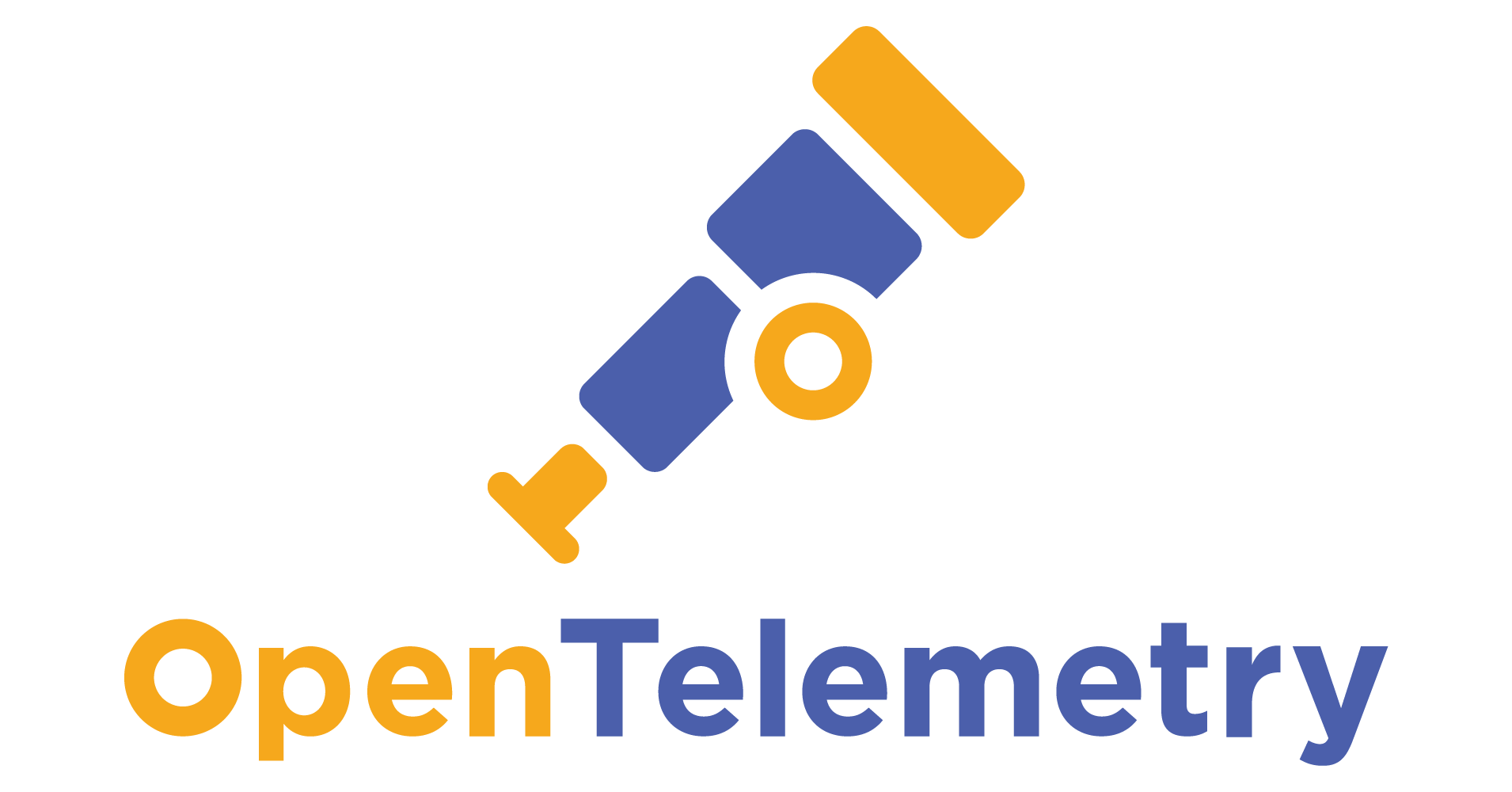
On Premises: 🤗 TGI
- Inzwischen schnell: Flash Attention, Fast Attention,
Tensor Parallelism
- Speculation & noch mehr Performance via Medusa
- Support for JSON & Tool Calling
- "Tools" Parameter unterstützt
-
Pydantic & JSON Schema Integration
- Support für Quantisierung, GPTQ & BitsAndBytes
On Premises:
- Als TGI mal eine komische Lizenz hatte und langsam war
- von den Erfindern von PagedAttention
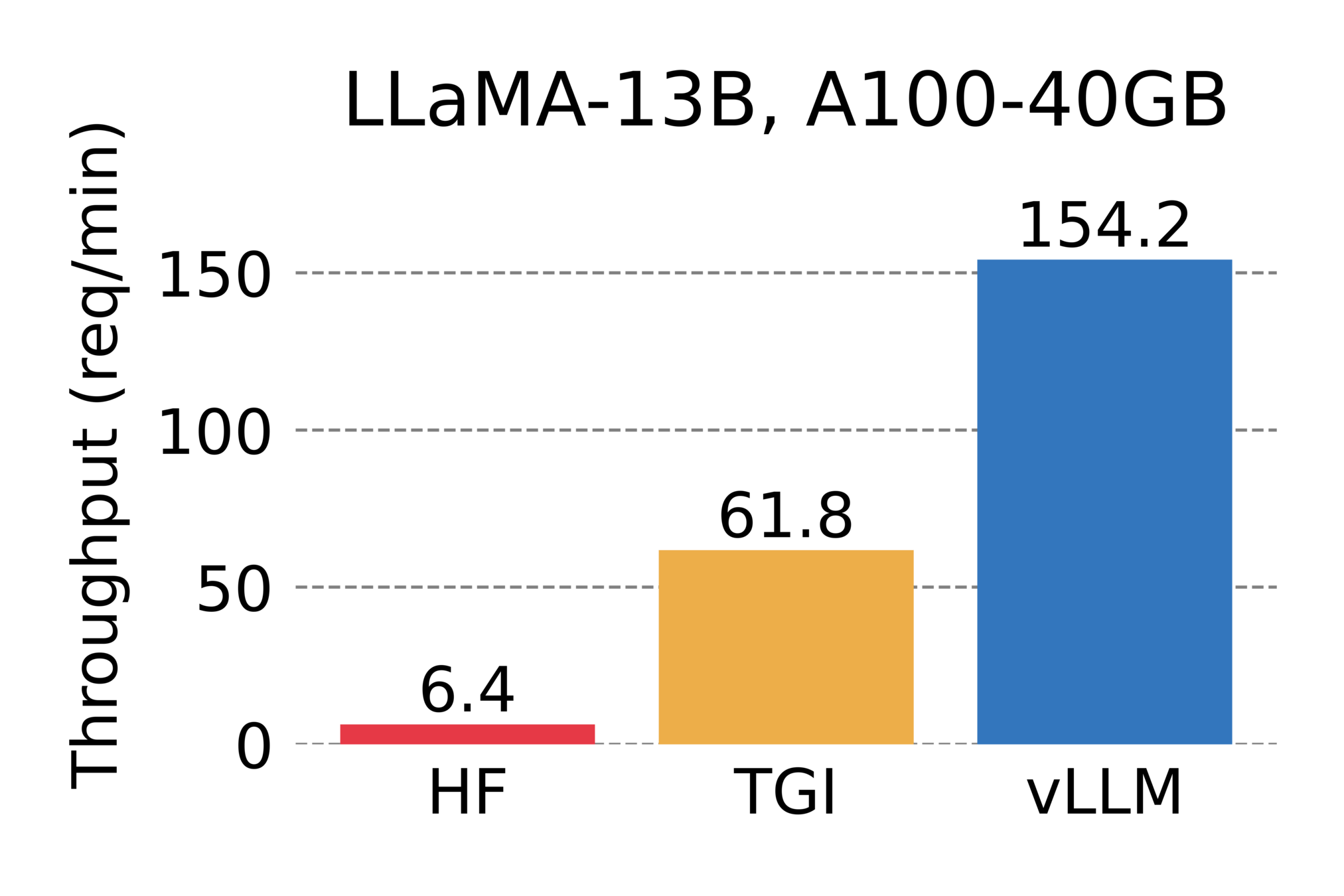
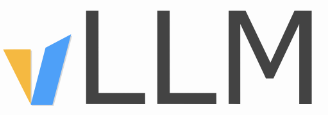
On Premises: vLLM
- Support für GPTQ, AWQ, SqueezeLLM
- Eigene Cuda-Kernels, Support für AMD, Intel
- Prefix-Caching !
- Multi-Lora-Support!
- Super lebendige Community ❤️
- Served die LMSYS ChatBot Arena
- Seit 2 Wochen auch Tool Support mit Guided Json
- Docker, Kubernetes: Check
- OpenAI-kompatible API
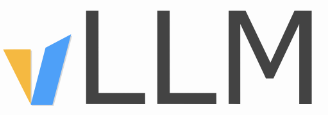
On Premises: vLLM
- Continuous Batching
- Docker Support
- Kubernetes-Verteilung über KubeRay oder KServe
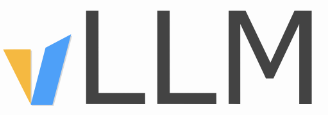
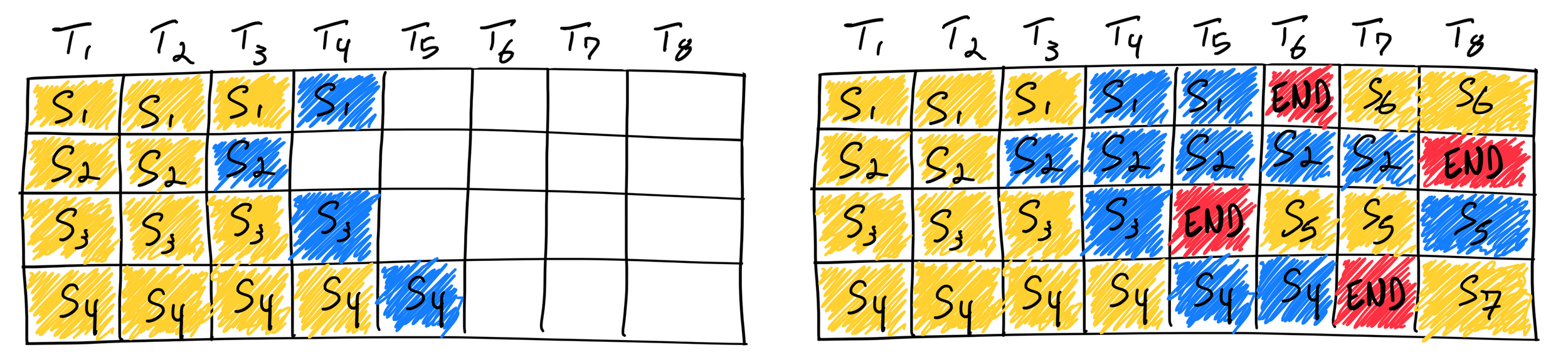
On Premises: LIGHTLLM
- Apache-2-Lizenz
- OpenAI-kompatible API
- Speicher-Effizient mit Token Attention
- zum Teil (LLaMa) schneller als TGI und vLLM
- Support für multimodale Modelle
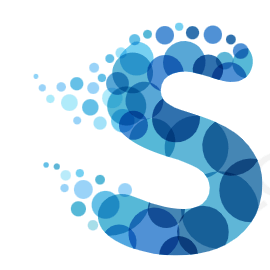
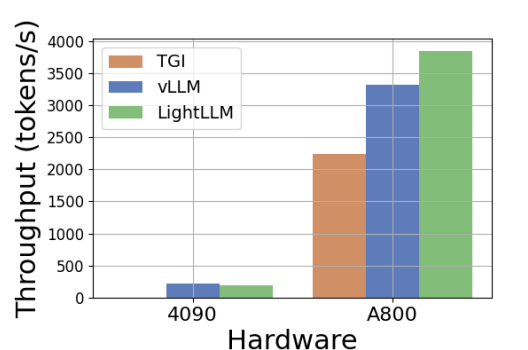
https://arxiv.org/pdf/2311.03687
On Premises:
NVIDIA TensorRT-LLM
- Python-API analog zu PyTorch
- Apache2-lizensiert
- Eigene CUDA-Kernel und Quantisierungen
- Wirklich schnell
- keine openai-messages-API, aber:
https://github.com/npuichigo/openai_trtllm - Support für Docker, Kubernetes
- Backend-Integration für NVIDIA-Triton
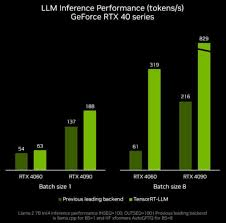
On Premises:
NVIDIA NIM
- Weil: Nvidia verdient nicht genug Geld.
- Models als einfache Container
- Direkt in Docker oder per Helm in Kubernetes
- Supported die OpenAI API
- Prometheus-Metrics
- Kommerziell, $4.500 per GPU/Jahr oder
$1 pro Cloud-GPU/Stunde - laut Werbung viel schneller und einfacher.
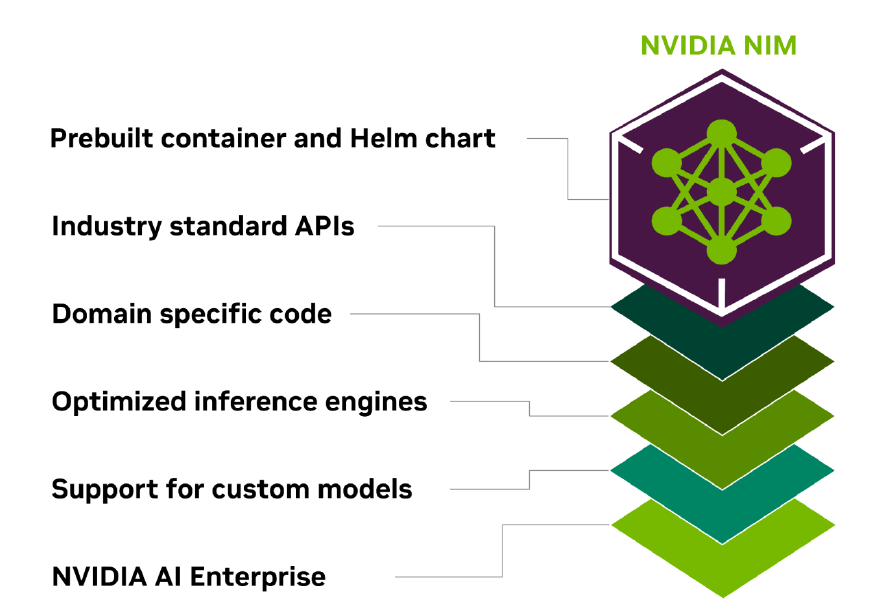
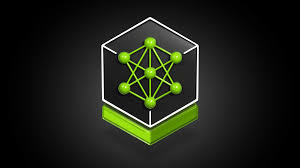
https://github.com/lapp0/lm-inference-engines
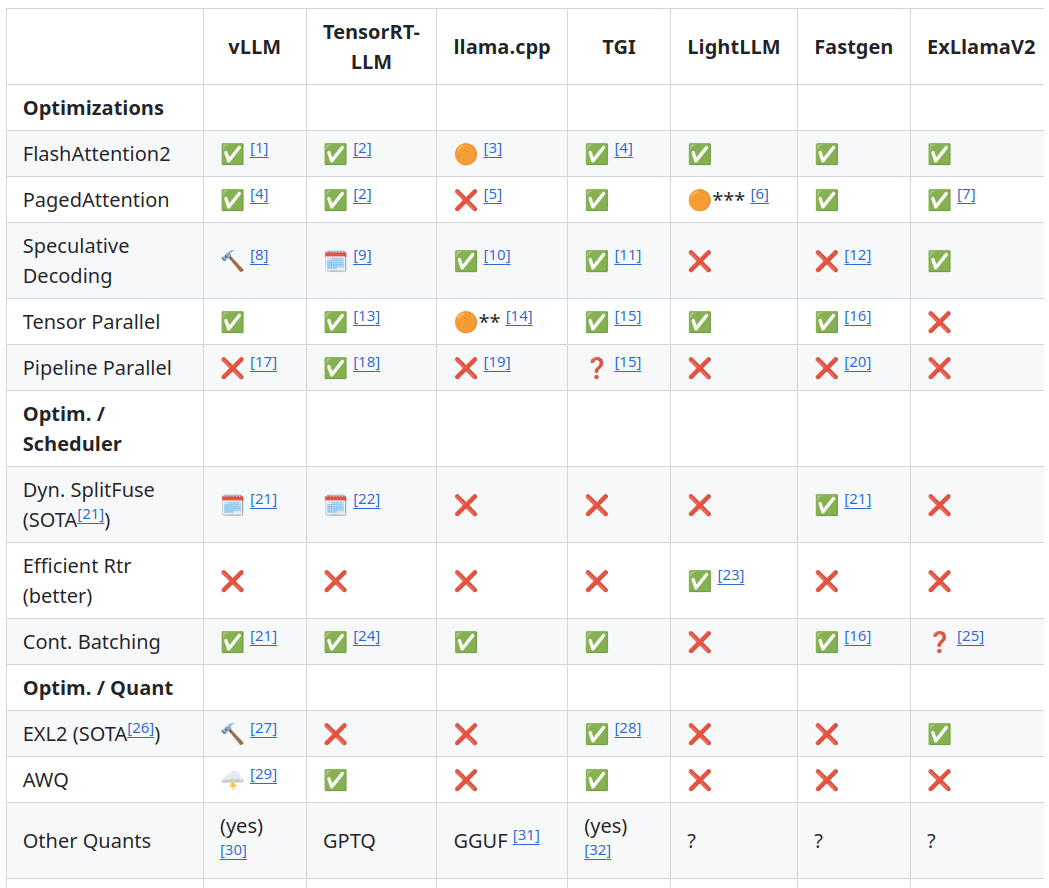
Auswahl
On Premises: Hardware
Device | Speicher | Cuda Cores | Kosten/Devices |
---|---|---|---|
RTX6000 | 48GB | 18176 | 7.245,- |
H100 PCIe | 80GB | 14592 | 29.354,- |
RTX4090 | 24GB | 16384 | 1.769,- |
Ampere A16 | 64G | 5120 | 3.100,- |
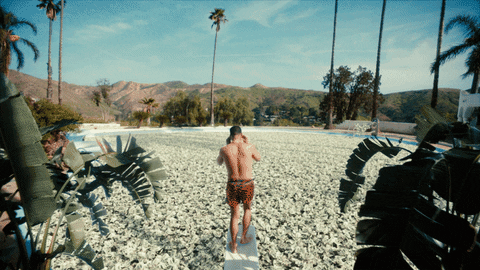
Memory als Kostentreiber
Model | Batchsize | Mem bf16 | A16 | H100 |
---|---|---|---|---|
LLaMa3-70B-Instruct | 1 | 239 | 12.400,- | 88.062,- |
LLaMa3-70B-Instruct | 5 | 673 | 34.100,- | 264.186,- |
LLaMa3-70B-Instruct | 50 | 5430 | 263.500,- | 1.996.072,- |
LLaMa3-8B-Instruct | 50 | 243 | 12.400,- | 117,416,- |
Aber:
- A16 sind deutlich langsamer, für die gleichen token/s braucht mal also deutlich mehr Geräte
- LlaMa3-8B ist deutlich schneller, sprich viel mehr token/s parallel
- NVidia NIM sieht auf einmal gar nicht mehr so teuer aus
Ok, dann vielleicht doch mieten.
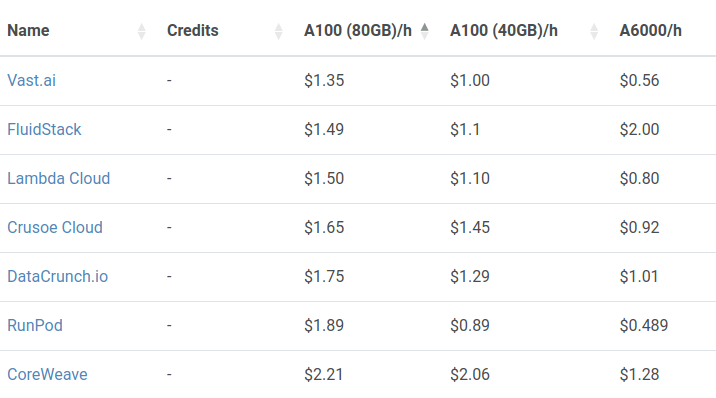
https://cloud-gpus.com/
3xH100 | Preis | Monatlich (3 Jahre) |
---|---|---|
Gekauft | 100.000,- | 2.800,- |
Gemietet | 0 | 4.300,- |
Rent or Buy?
Aber was ist, wenn ich nur zwischen 8 und 18 Uhr wirklich zugreife?
Spot-Instanzen < Reserviert < On Demand
Deployment mit SkyPilot
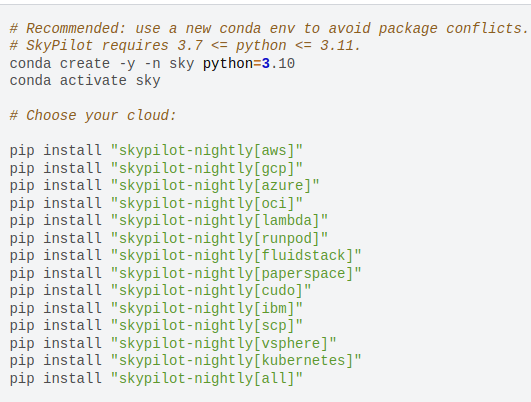
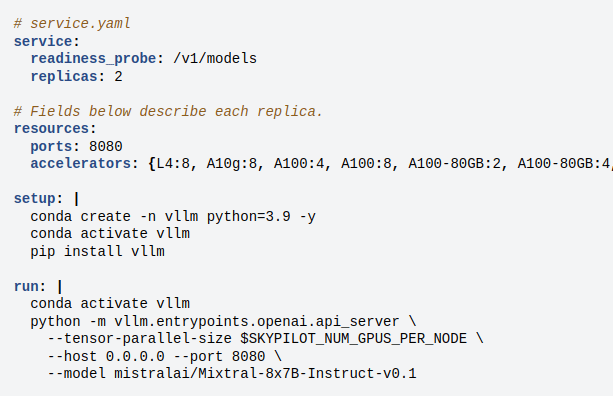
Deployment mit dstack
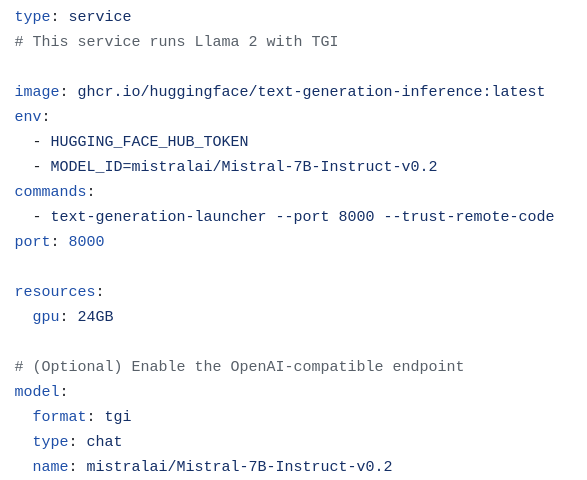
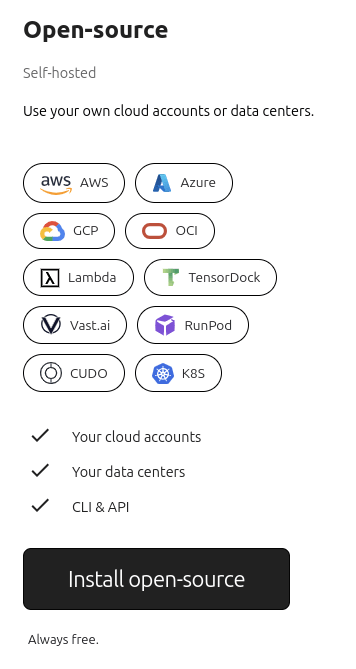
Und selbst trainieren?
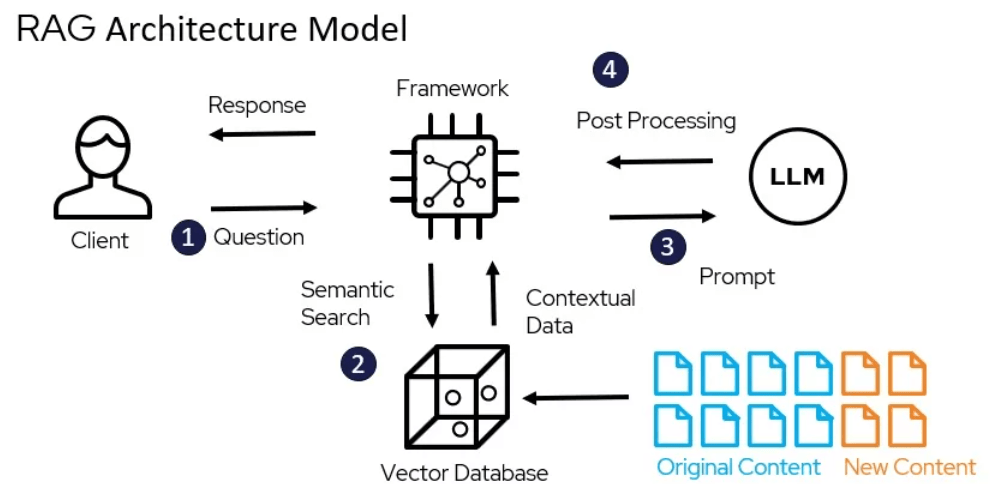
Selbst trainieren
Internal
Sources
Human
Feedback
DataSet
Generation
Supervised
Finetuning
DPO-
Training
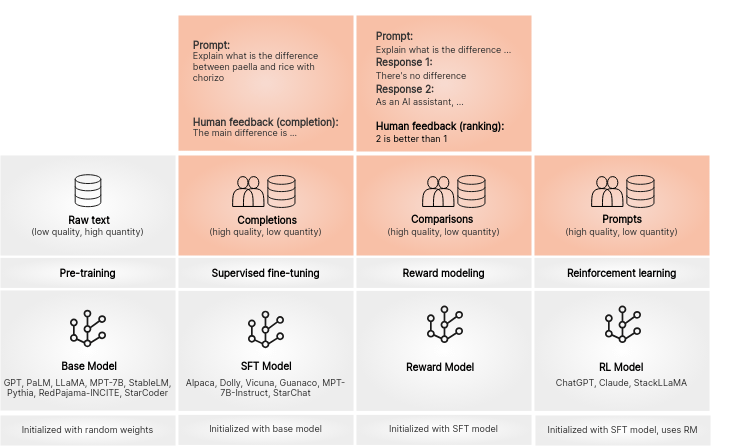
https://argilla.io/blog/argilla-for-llms/
Human
Feedback
DPO
Datasets aus eigenen Quellen
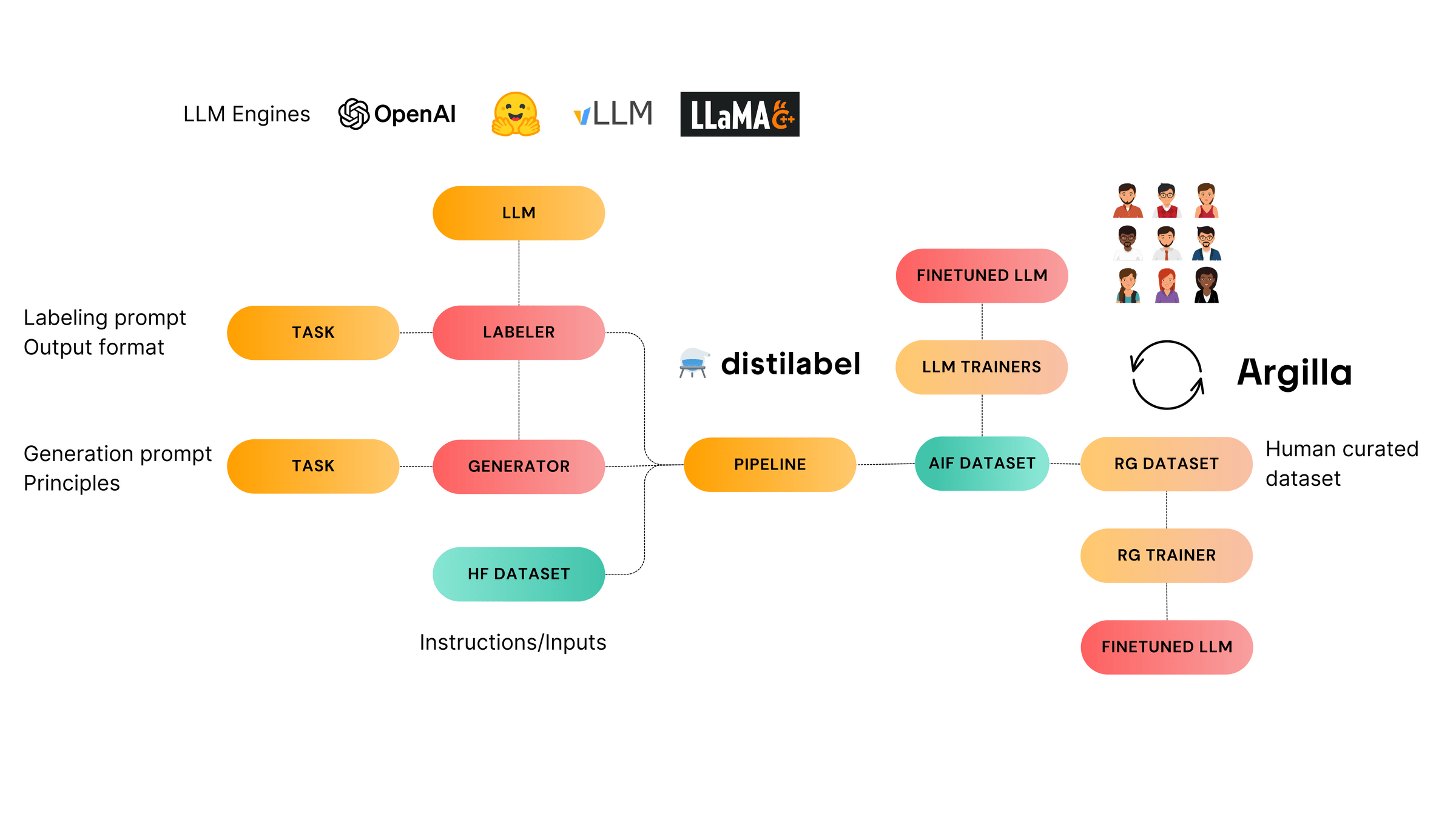
- Ebenfalls von Argilla: Distilabel - https://distilabel.argilla.io/
- Pipelines zur Erzeugung von Datasets.
- Automatisches Erzeugen von Instruktions per selfinstruct
- Aus Dokumenten, Datenbanken, etc
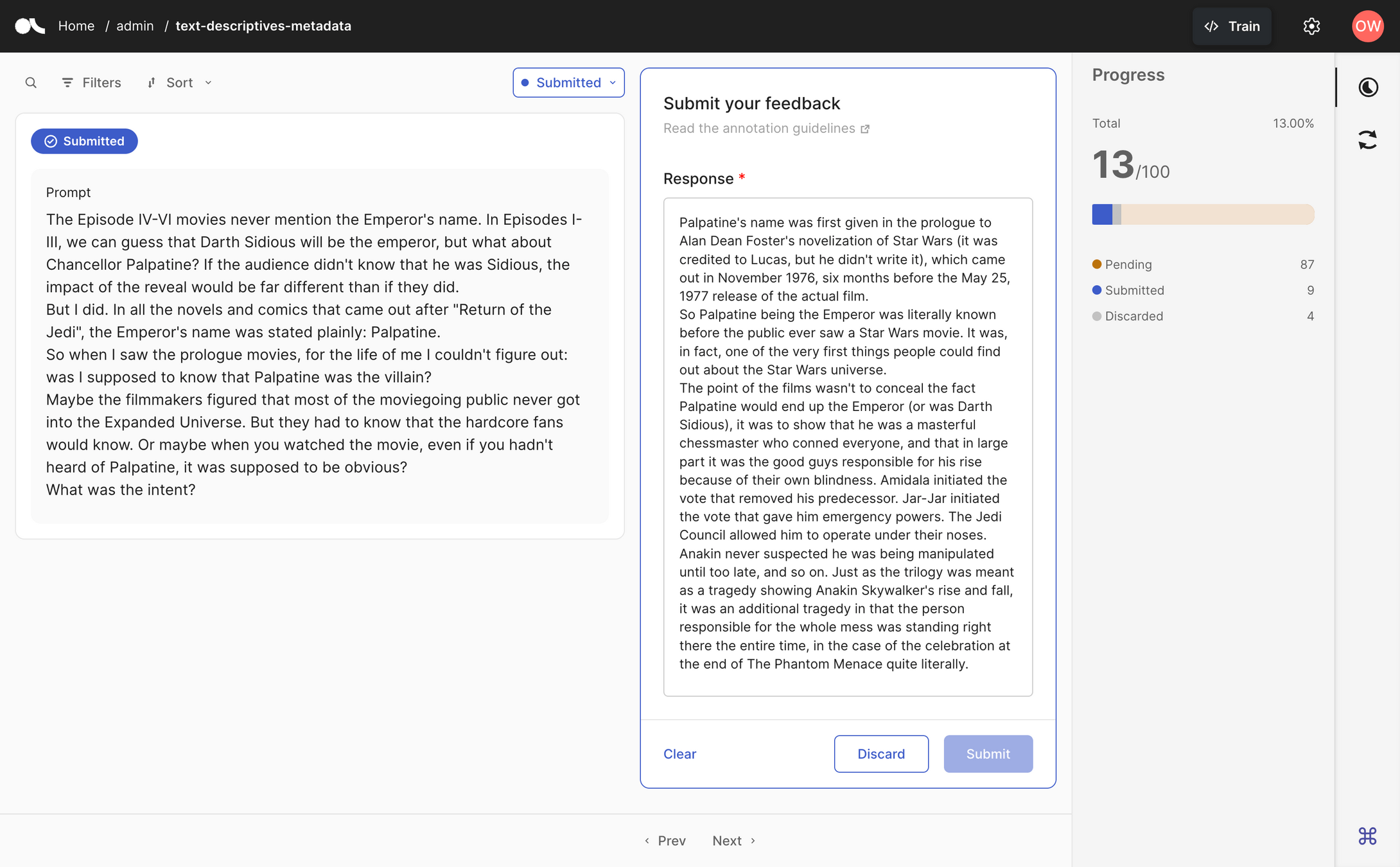

- Tool zum Kuratieren von Datasets
- Korrektur falscher Antworten aus Produktion oder Dataset
- Kann automatisch Daten sammeln
- zB aus LangChain-Applikationen
- Ranking für DPO

- Von Redhat/ IBM, um OpenSource zu "unterstützen"
- Grundidee:
- man liefert seine Trainingdaten in ein Repository,
- IBM trainiert darauf.
- Methode
- Frage und Antwort-Paare erzeugen
- Diese in Taxonomien einordnen und speichern
- InstructLab baut auf der Basis synthetische Daten
- Überraschend gute kleine Modelle sind das Resultat
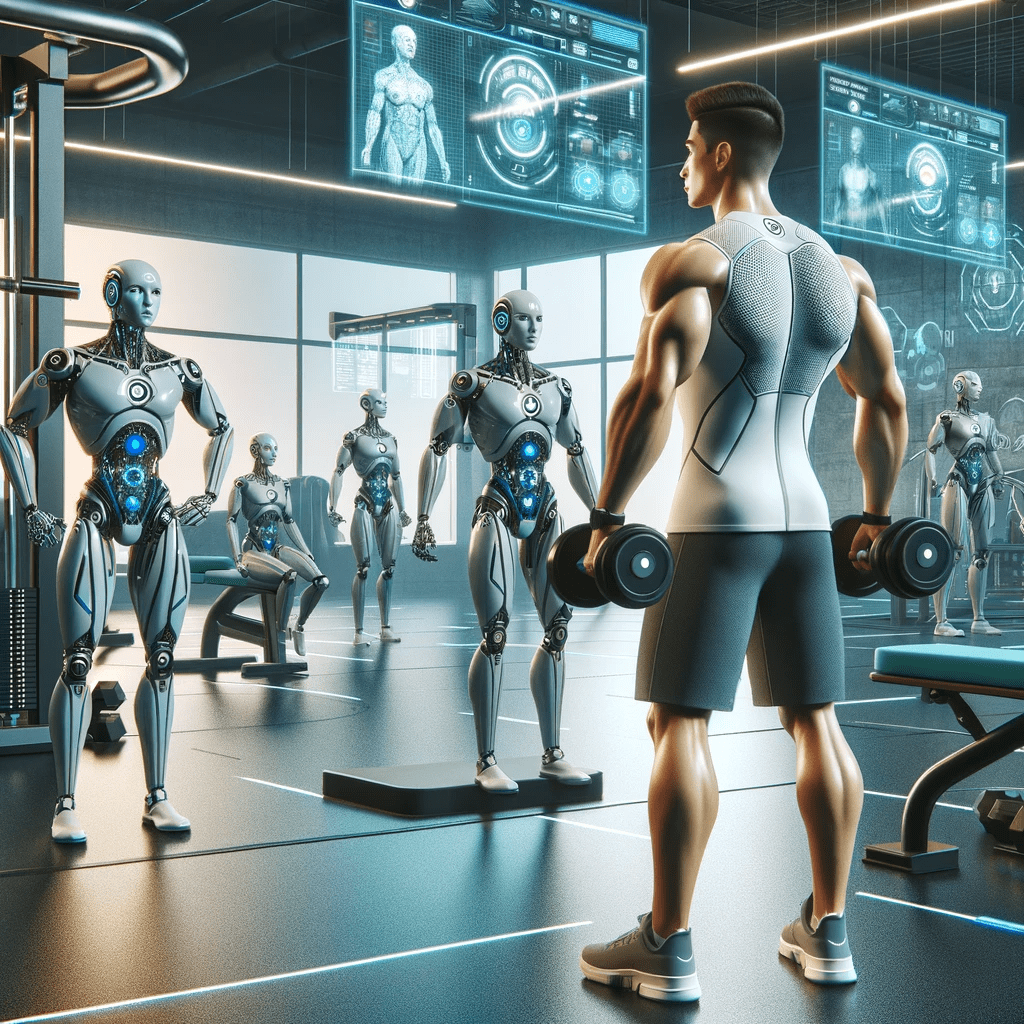
Trainieren
- 8,4 TRL von Huggingface
- 6,3 Axolotl vom OpenAI Collective
- 23,1 LLama_Factory von Yaowei Zheng
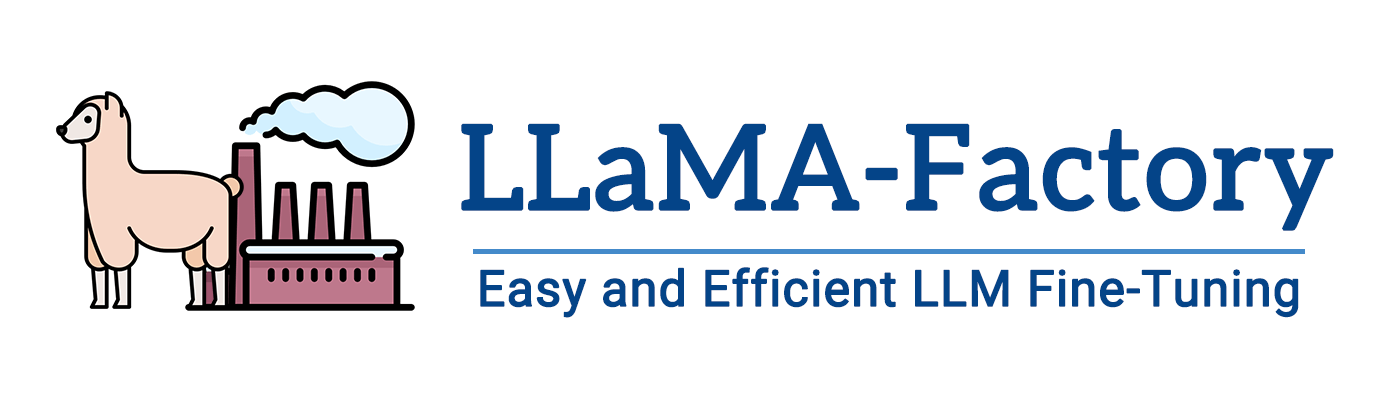
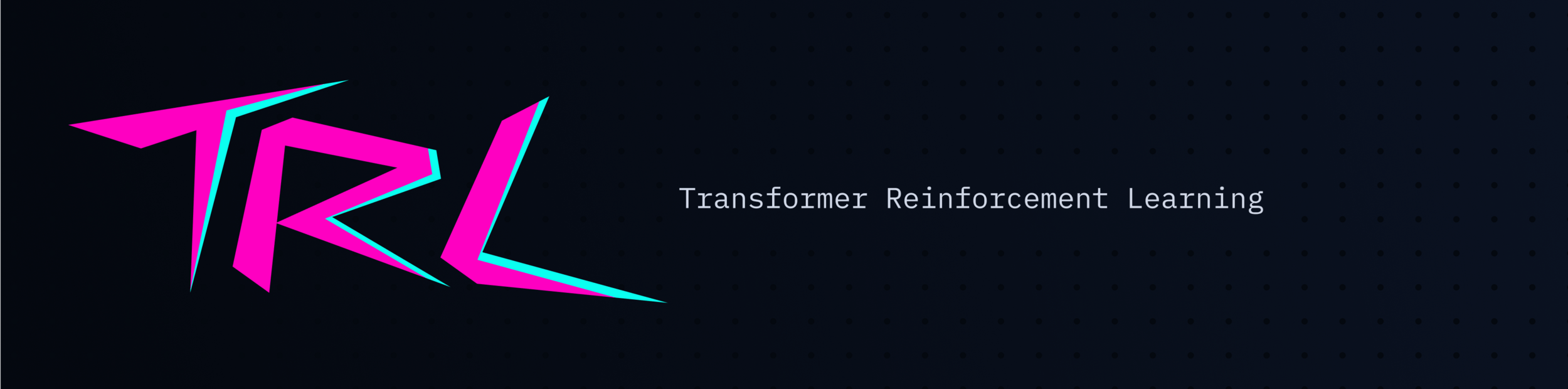
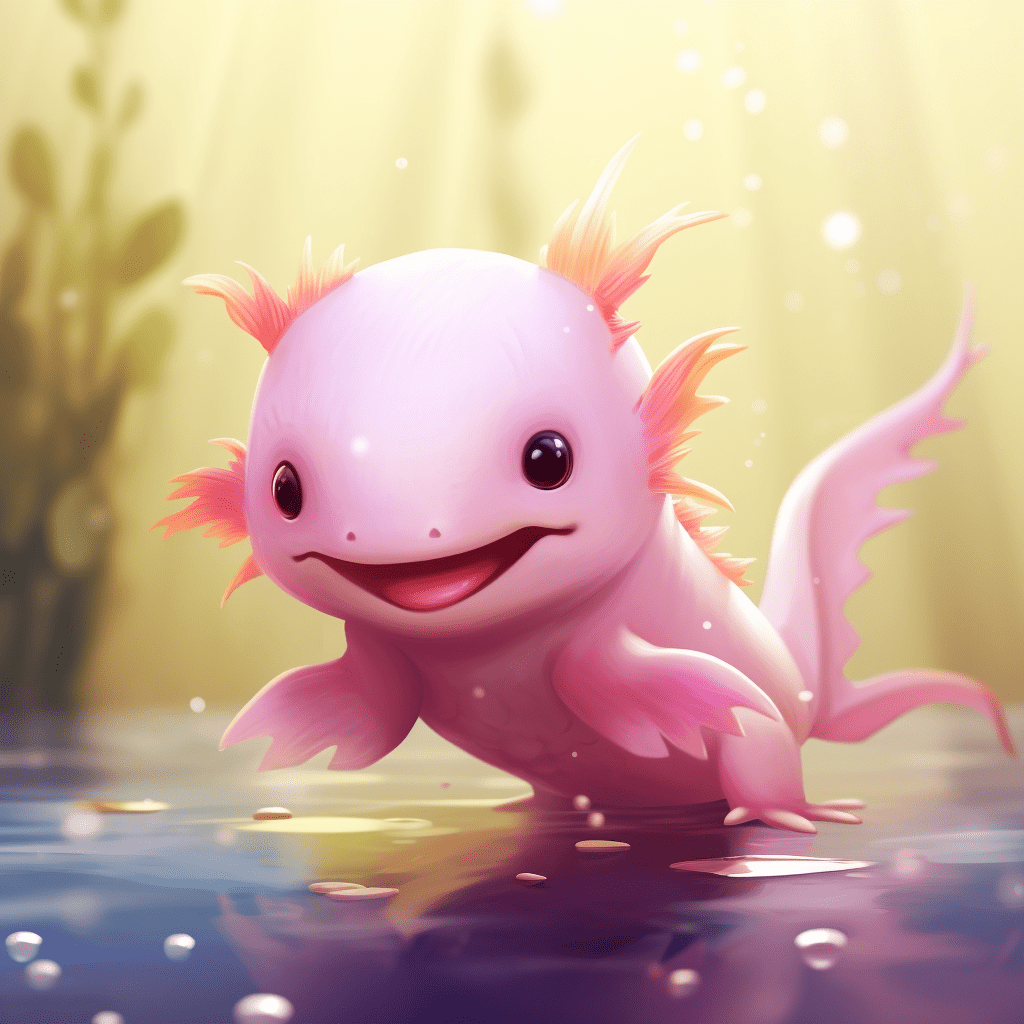
Hugging Face TRL
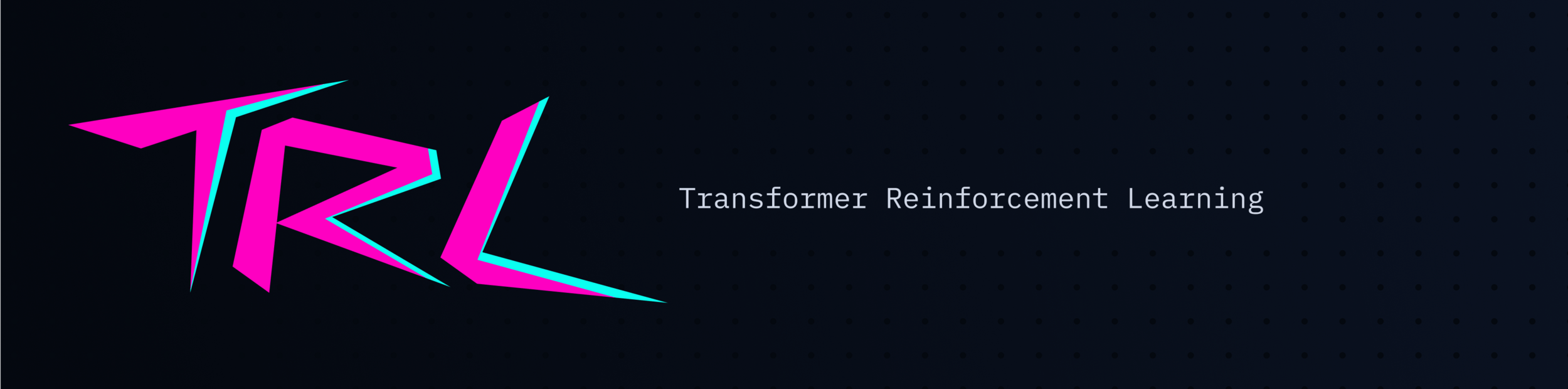
Features
- SFT, DPO, PPO, ORPO
- Unsloth-Support
- Eingebettet in HF-Stack
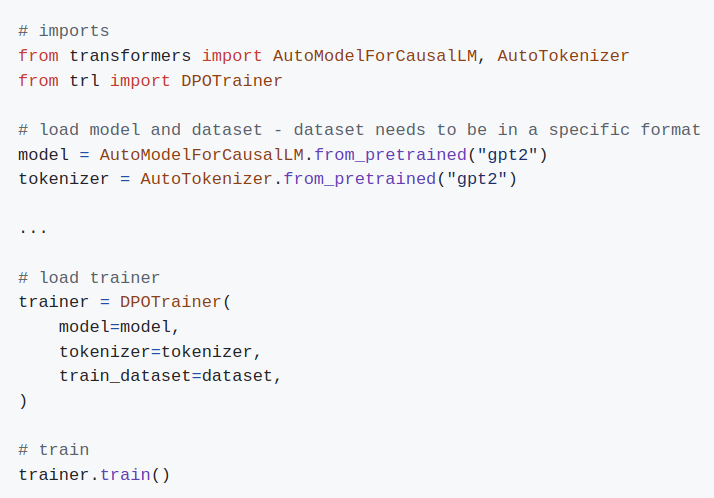
Axolotl
Features
- SFT, Lora, qlora, flashattn
- Config-Getrieben
- Viele Beispiele
- Gute Community
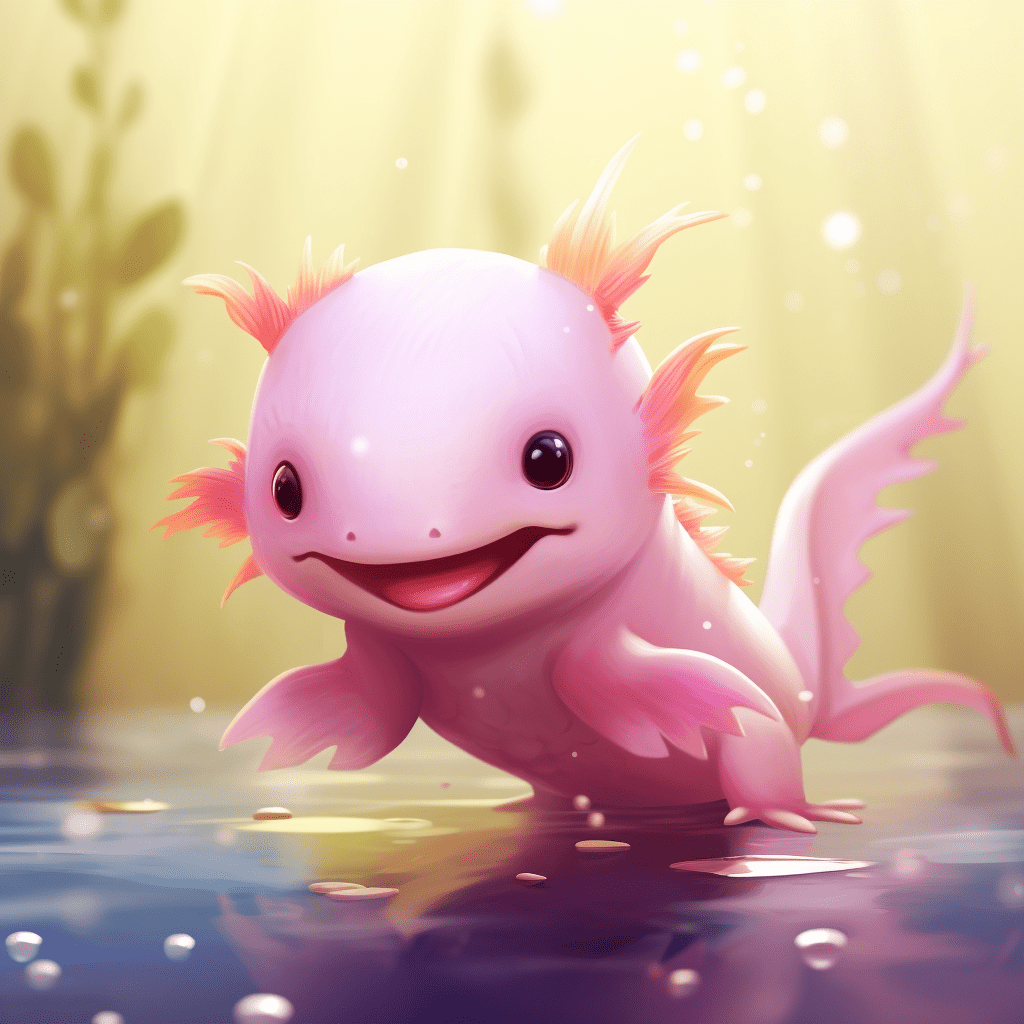
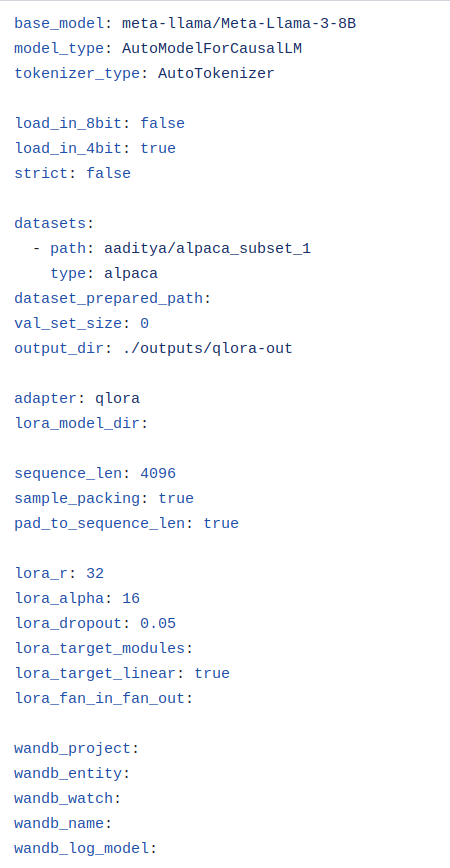
LLaMA-Factory
Features
- Sehr grosse Community ...
- ... in Asien
- adaptiert unglaublich schnell
- Web-Interface & Config
- praktisch alle Algorithmen enthalten, inkl. Unsloth
- Diverse deutsche Datasets enthalten
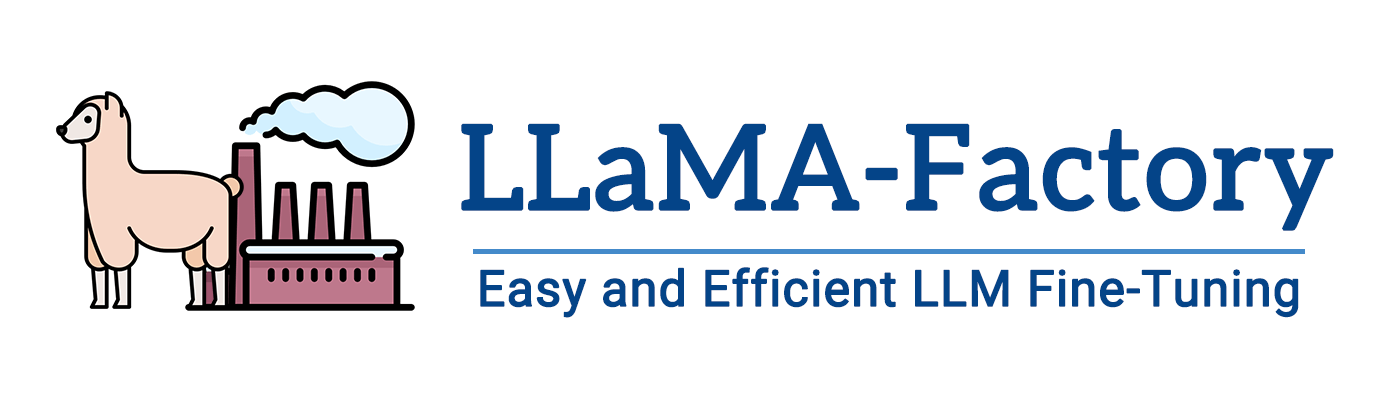
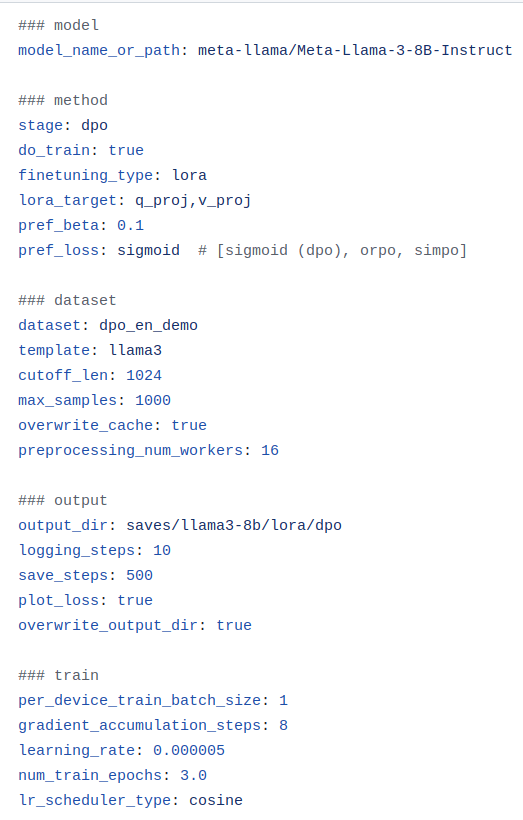
Benchmarking
- Strategie 1: programmatisch
- mein LLM wählt aus einer Liste von Optionen
- die Distanz zur "richtigen" Antwort wird gemessen
- Strategie 2: "LLM as a Judge"
- Ein Teacher-LLM prüft das Ergebnis
- Sehr schnell und einfach
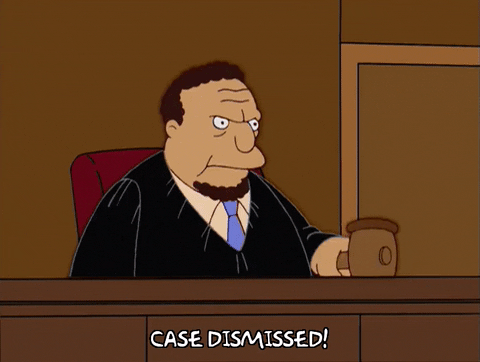
Benchmarking
https://github.com/occiglot/euro-lm-evaluation-harness
Programmatisch, Version des EleutherAI-Frameworks
Internationale Standard-Evals auf Deutsch
https://github.com/mayflower/FastEval
Einfache Implementierung von mt-bench mit LLM-as-a-judge
https://github.com/ScandEval/ScandEval
Programmatisch, Skandinavische Test-Suite mit Support für Deutsch
https://github.com/EQ-bench/EQ-Bench
Benchmark mit EQ und Kreativität im Fokus, LLM-as-a-judge.
Model
im Betrieb
Fazit - der volle Loop
Feedback
Collection
Dataset
Creation
Model
Training
Unternehmens-
Daten/Skills
vLLM
TGI
On-the-Edge
Argilla
LangSmith
LangFuse
Haystack
LangChain
Distilabel
InstructLab
Axolotl
LLaMa_Factory
Vielen Dank!
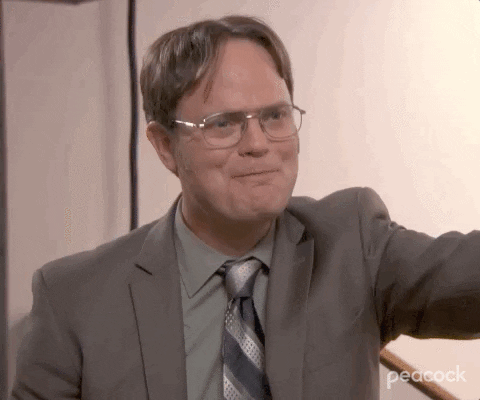
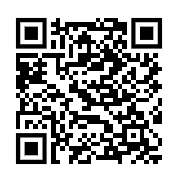
Ich freue mich über Gespräche oder Fragen per Linked-In (QR links) :-)
Slides finden sich im QR-Code rechts.
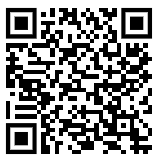
LLMs im Selbstbetrieb
By Johann-Peter Hartmann
LLMs im Selbstbetrieb
Wenn man über AI nicht nur redet, sondern es macht, dann merkt man schnell, dass es eine ganz normale Technologie ist, mit den ganz normalen Aufgaben, die mit dem Betrieb einhergehen. Auf welche technische Plattform setzt man? On Premises, in der eigenen, in der fremden Cloud oder as a Service? Wie skaliert man LLms elastisch, wie baut man Pipelines zu Training und Finetuning auf? Wir geben einen Überblick über die sich rasant entwickelnde Welt der Werkzeuge für offene LLMs, und mit welchen Hürden man heute noch rechnen muss.
- 332