Workflow and tool management
Johannes Köster
AEBC 2017
https://koesterlab.github.io
dataset
results
Data analysis
"Let me do that by hand..."

dataset
results
dataset
dataset
dataset
dataset
dataset


"Let me do that by hand..."
Data analysis
dataset
results
dataset
dataset
dataset
dataset
dataset
automation
From raw data to final figures:
- document parameters, tools, versions
- execute without manual intervention
Reproducible data analysis
dataset
results
dataset
dataset
dataset
dataset
dataset
scalability
Handle parallelization:
- execute for tens to thousands of datasets
- efficiently use any computing platform
automation
Reproducible data analysis
dataset
results
dataset
dataset
dataset
dataset
dataset
Handle deployment:
be able to easily execute analyses on a different machine
portability
scalability
automation
Reproducible data analysis
dataset
results
dataset
dataset
dataset
dataset
dataset
scalability
automation
portability
Workflow management
Nextflow
Galaxy
Bpipe
Taverna
Bcbio-nexgen
Hadoop
KNIME
Snakemake
CWL

Genome of the Netherlands:
GoNL consortium. Nature Genetics 2014.
Cancer:
Townsend et al. Cancer Cell 2016.
Schramm et al. Nature Genetics 2015.
Martin et al. Nature Genetics 2013.
Ebola:
Park et al. Cell 2015
iPSC:
Burrows et al. PLOS Genetics 2016.
Computational methods:
Ziller et al. Nature Methods 2015.
Schmied et al. Bioinformatics 2015.
Břinda et al. Bioinformatics 2015
Chang et al. Molecular Cell 2014.
Marschall et al. Bioinformatics 2012.




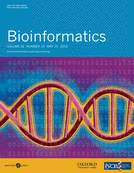



dataset
results
dataset
dataset
dataset
dataset
dataset
Define workflows
in terms of rules
Define workflows
in terms of rules
rule mytask:
input:
"path/to/{dataset}.txt"
output:
"result/{dataset}.txt"
script:
"scripts/myscript.R"
rule myfiltration:
input:
"result/{dataset}.txt"
output:
"result/{dataset}.filtered.txt"
shell:
"mycommand {input} > {output}"
rule aggregate:
input:
"results/dataset1.filtered.txt",
"results/dataset2.filtered.txt"
output:
"plots/myplot.pdf"
script:
"scripts/myplot.R"
Define workflows
in terms of rules
Define workflows
in terms of rules
rule mytask:
input:
"data/{sample}.txt"
output:
"result/{sample}.txt"
conda:
"software-envs/some-tool.yaml"
shell:
"some-tool {input} > {output}"
rule name
refer to input and output from shell command
how to create output from input
(shell, Python, R)
Directed acyclic graph (DAG) of jobs
dataset
results
dataset
dataset
dataset
dataset
dataset
scalability
automation
portability
Scheduling
Paradigm:
Workflow definition shall be independent of computing platform and available resources
Rules:
define resource usage (threads, memory, ...)
Scheduler:
- solves multidimensional knapsack problem
- schedules independent jobs in parallel
- passes resource requirements to any backend
Scalable to any platform



workstation
compute server
cluster






grid computing
cloud computing

Command-line interface
# execute workflow locally with 16 CPU cores
snakemake --cores 16
# execute on cluster
snakemake --cluster qsub --jobs 100
# execute in the cloud
snakemake --kubernetes --jobs 1000 --default-remote-provider GS --default-remote-prefix mybucket
dataset
results
dataset
dataset
dataset
dataset
dataset
Full reproducibility:
install required software and all dependencies in exact versions
portability
scalability
automation
Software installation is a pain
source("https://bioconductor.org/biocLite.R")
biocLite("DESeq2")
easy_install snakemake
./configure --prefix=/usr/local
make
make install
cp lib/amd64/jli/*.so lib
cp lib/amd64/*.so lib
cp * $PREFIX
cpan -i bioperl
cmake ../../my_project \
-DCMAKE_MODULE_PATH=~/devel/seqan/util/cmake \
-DSEQAN_INCLUDE_PATH=~/devel/seqan/include
make
make install
apt-get install bwa
yum install python-h5py
install.packages("matrixpls")
Example:
ISMB 2016 "Wall of Shame"
Of 47 open-access publications, ...
https://github.com/bioconda/bioconda-recipes/pull/1951
status | count |
---|---|
properly documented, easy to install | 4 |
web service | 4 |
Docker image | 1 |
R packages, not (yet) on CRAN or Bioconductor | 3 |
no software implementation | 7 |
MATLAB code | 4 |
available upon request | 2 |
collection of scripts without proper way to install | 12 |
demo only although README promises a release before ISMB | 1 |
either unclear, no, or errorneous installation instructions | 3 |
missing download URL | 1 |
invalid links | 4 |
build error | 1 |
Example:
ISMB 2016 "Wall of Shame"
Of 47 open-access publications, ...
https://github.com/bioconda/bioconda-recipes/pull/1951
status | count |
---|---|
properly documented, easy to install | 4 |
web service | 4 |
Docker image | 1 |
R packages, not (yet) on CRAN or Bioconductor | 3 |
no software implementation | 7 |
MATLAB code | 4 |
available upon request | 2 |
collection of scripts without proper way to install | 12 |
demo only although README promises a release before ISMB | 1 |
either unclear, no, or errorneous installation instructions | 3 |
missing download URL | 1 |
invalid links | 4 |
build error | 1 |
good (26%)
bad (53%)
ugly (21%)
Package management with
package:
name: seqtk
version: 1.2
source:
fn: v1.2.tar.gz
url: https://github.com/lh3/seqtk/archive/v1.2.tar.gz
requirements:
build:
- gcc
- zlib
run:
- zlib
about:
home: https://github.com/lh3/seqtk
license: MIT License
summary: Seqtk is a fast and lightweight tool for processing sequences
test:
commands:
- seqtk seq
Idea:
Normalization installation via recipes
#!/bin/bash
export C_INCLUDE_PATH=${PREFIX}/include
export LIBRARY_PATH=${PREFIX}/lib
make all
mkdir -p $PREFIX/bin
cp seqtk $PREFIX/bin
- source or binary
- recipe and build script
- package
Easy installation and management:
no admin rights needed
conda install pandas
conda update pandas
conda remove pandas
conda env create -f myenv.yaml -n myenv
Isolated environments:
channels:
- conda-forge
- defaults
dependencies:
- pandas ==0.20.3
- statsmodels ==0.8.0
- r-dplyr ==0.7.0
- r-base ==3.4.1
- python ==3.6.0
Package management with
rule mytask:
input:
"path/to/{dataset}.txt"
output:
"result/{dataset}.txt"
conda:
"envs/mycommand.yaml"
shell:
"mycommand {input} > {output}"
Integration with Snakemake
channels:
- conda-forge
- defaults
dependencies:
- mycommand ==2.3.1
Over 2800 bioinformatics related packages
Over 200 contributors



Bioconda workflow
- recipe
- pull request
- automatic linting
- building
- testing
- human review
- merge
- upload
Builds and tests:
Paradigm:
- transparency
- open source build framework
- public logs
dataset
results
dataset
dataset
dataset
dataset
dataset
portability
scalability
automation
How to publish this in a sustainable way?
Sustainable publishing
Goal:
- persistently store reproducible data analysis
- ensure it is always accessible
Minimize dependencies:
- third-party resources
- proprietary formats
Solution:
- store everything (including packages) in an archive
- upload to persistent storage like https://zenodo.org
- obtain document object identifier (DOI)
Sustainable publishing
# archive workflow (including Conda packages)
snakemake --archive myworkflow.tar.gz
Author:
- Upload to Zenodo and acquire DOI.
- Cite DOI in paper.
Reader:
- Download and unpack workflow archive from DOI.
# execute workflow (Conda packages are deployed automatically)
snakemake --use-conda --cores 16
Conclusion
- For reproducible data analysis, three dimensions have to be considered.
- A lightweight yet flexible approach to achieve this is to use Snakemake and Bioconda/Conda.
portability
scalability
automation
Acknowledgements
The Snakemake core team
- Christopher Tomkins-Tinch
- David Koppstein
- Tim Booth
- Manuel Holtgrewe
- Christian Arnold
- Wibowo Arindrarto
The Bioconda core team
- Ryan Dale
- Brad Chapman
- Chris Tomkins-Tinch
- Björn Grüning
- Andreas Sjödin
- Jillian Rowe
- Renan Valieris
Workflow and tool management
By Johannes Köster
Workflow and tool management
Keynote at AEBC 2017
- 3,105