
A generalized semi-supervised elastic-net
Juan C. Laria
juancarlos.laria@uc3m.es

The semi-supervised framework
unsupervised

The semi-supervised framework
supervised


The semi-supervised framework
semi-supervised


The semi-supervised framework
semi-supervised
transfer-model


Elastic-net regularization
lasso
ridge


Joint trained elastic-net
Culp, M. (2013). On the semisupervised joint trained elastic net. Journal of Computational and Graphical Statistics 22 (2), 300–318.


Extended linear joint trained framework
Søgaard Larsen, J. et. al (2020). Semi-supervised covariate shift modelling of spectroscopic data. (in-press)


Semi-supervised elastic-net





Optimization
elastic-net with custom loss function
- Fast iterative shrinkage-thresholding algorithm (FISTA)
- Acelerated (block) gradient descent approach


Implementation





-
We develop a flexible and fast implementation for s2net in R, written in C++ using RcppArmadillo and integrated into R via Rcpp modules.
-
The software is available in the s2net package
install.packages("s2net")
library(s2net)
vignette(package = "s2net")

Hyper-parameter tuning



library(cloudml)
cloudml_train("main_script.R", config = "tuning.yml")
FLAGS <- flags(
flag_numeric("lambda1", 0.01, "Lasso weight"),
flag_numeric("lambda2", 0.01, "Ridge weight"),
flag_numeric("gamma1", 0.1, "s2net global weight"),
flag_numeric("gamma2", 100, "s2net covariance parameter"),
flag_numeric("gamma3", 0.5, "s2net shift parameter")
)
- Cloud based solution
- Easy to implement
- Blackbox grid/random search, bayesian optimization available
- Limited resources in the free tier
- Can be pricey
- Slow - not suitable for fast algorithms
- All dependencies should be available from CRAN - otherwise it is very difficult to make it work
Hyper-parameter tuning


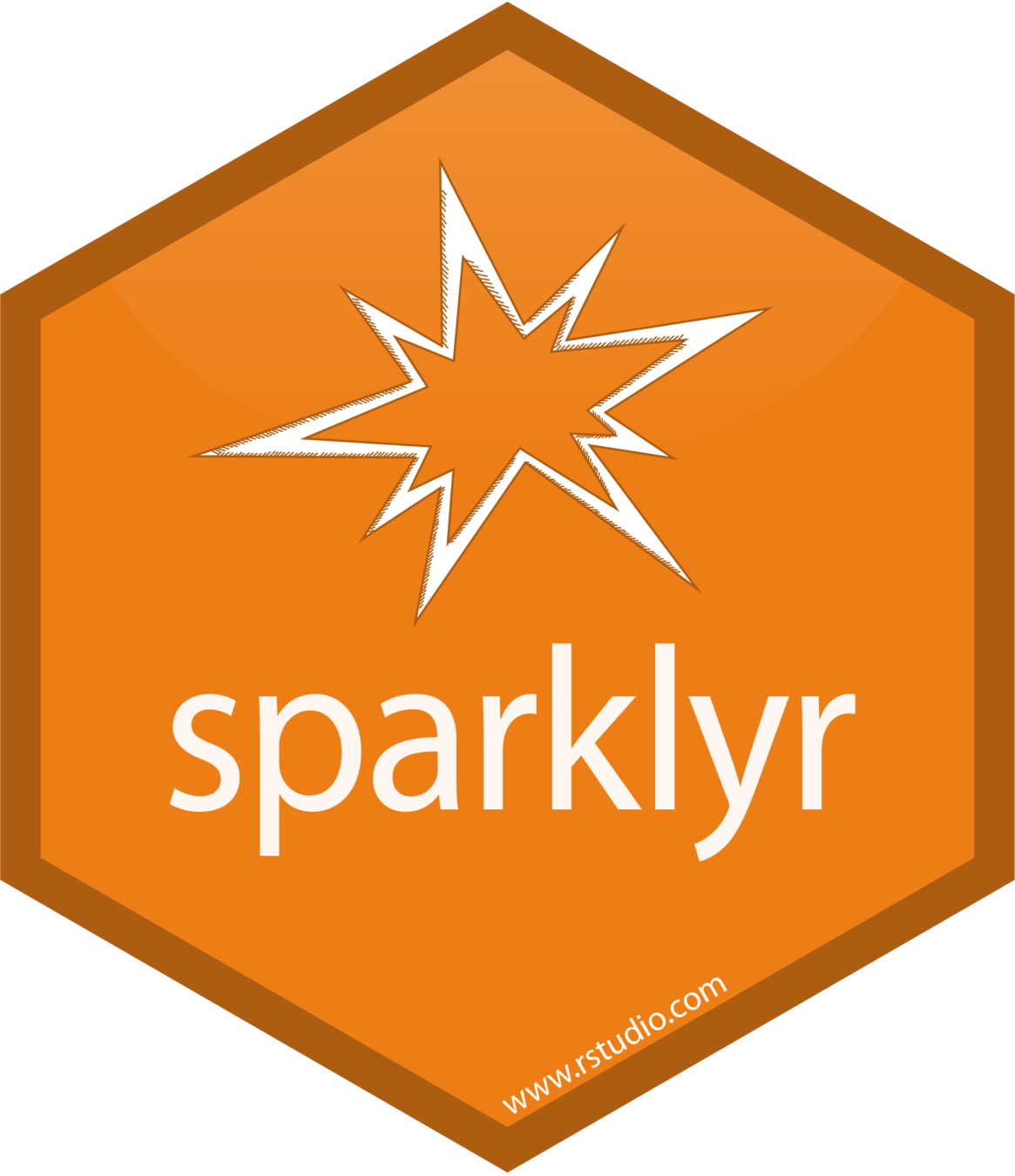

- Cloud based solution that runs locally
- Extremely fast, given the hardware
- Open source
- Very difficult to implement
- Needs local hardware, or renting a cluster in the cloud
- Native scala hyper-parameter tuning functions are only available for popular methods.
- You have to implement your search function that works with your method.
library(sparklyr)
result = spark_apply(grid, my_function, context = datos)
THANK you!
s2net
By Juan Carlos Laria
s2net
- 1,015