ACL 2023





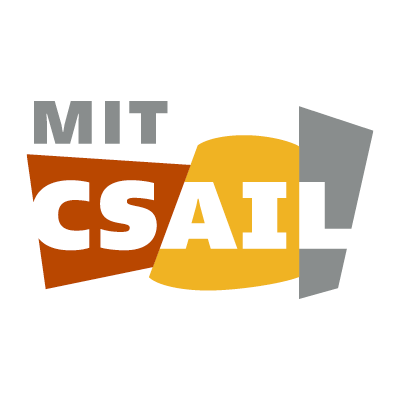
CREST:
A Joint Framework for Rationalization
and Counterfactual Text Generation
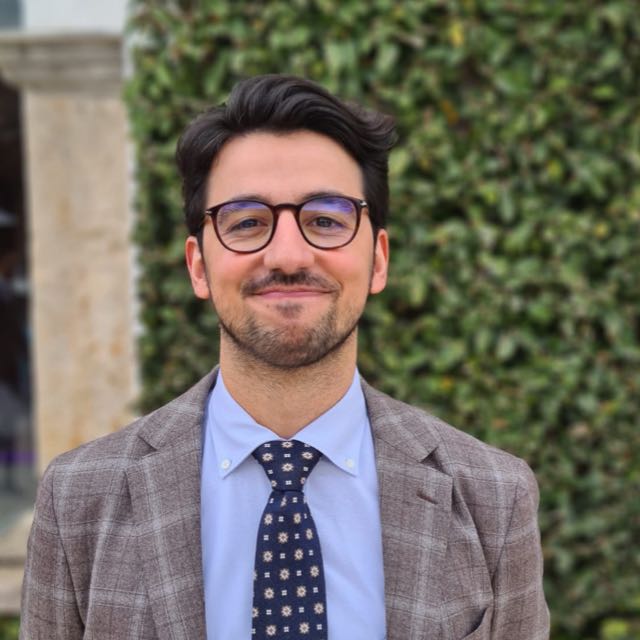
Nuno M. Guerreiro
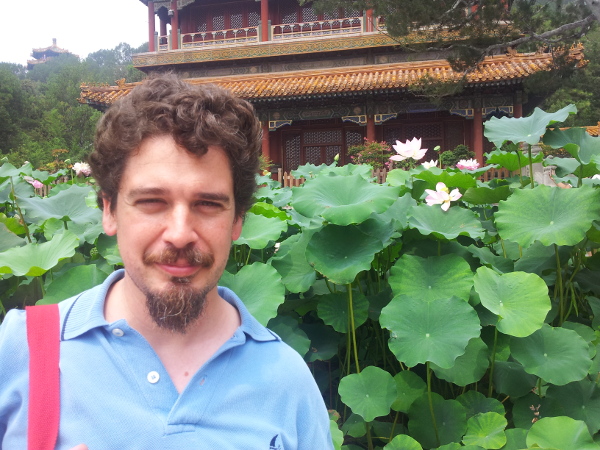
André F. T. Martins
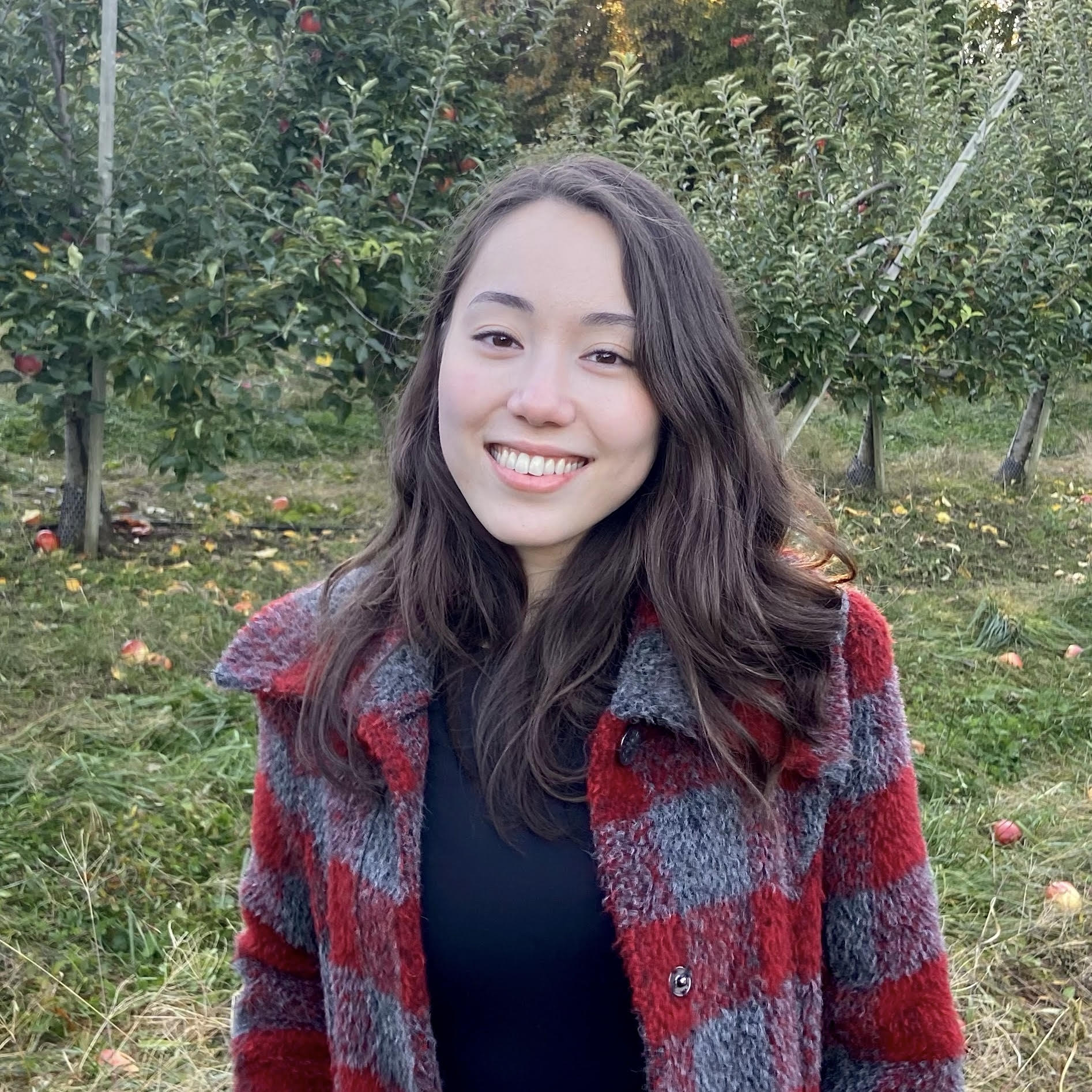
Alexis Ross

Marcos Treviso
How to explain the classifier's decision?
This album is terrible and some of the songs are really bad

NEG
NEU
POS
How to explain the classifier's decision?
This album is terrible and some of the songs are really bad
This album is terrible and some of the songs are really bad

NEG
NEU
POS
Selective Rationalization
a.k.a. mask-then-predict
highlights as explanations
How to explain the classifier's decision?
This album is terrible and some of the songs are really bad
This album is terrible and some of the songs are really bad
This album is amazing and some of the songs are very well written

Selective Rationalization
a.k.a. mask-then-predict
highlights as explanations
Counterfactual Generation
a.k.a. what-if questions
edits as explanations
NEG
NEU
POS
How to explain the classifier's decision?
CREST (ContRastive Edits with Sparse raTionalization)
Selective Rationalization
a.k.a. mask-then-predict
highlights as explanations
Counterfactual Generation
a.k.a. what-if questions
edits as explanations
NEG
NEU
POS
This album is terrible and some of the songs are really bad
This album is terrible and some of the songs are really bad
This album is amazing and some of the songs are very well written

CREST-Generation
This album is terrible and some of the songs are really bad
\(\bm{x}\)
CREST-Generation
Trainable Masker
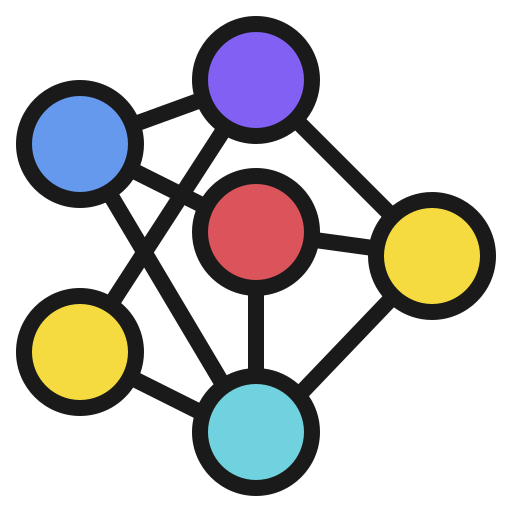
This album is terrible and some of the songs are really bad
\(\bm{x}\)
CREST-Generation
Trainable Masker
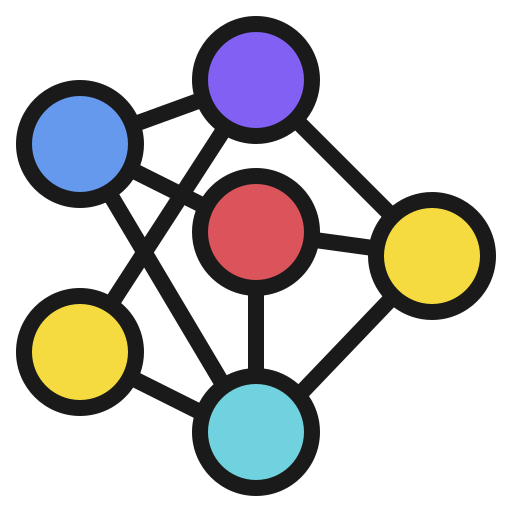
\(\bm{z}\)
This album is terrible and some of the songs are really bad
\(\bm{x}\)
This album is terrible and some of the songs are really bad
CREST-Generation
\(\hat{y}\)
NEG
Predictor

Trainable Masker
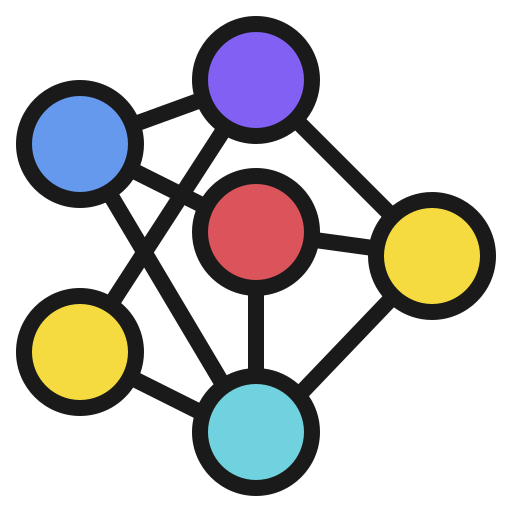
\(\bm{z}\)
This album is terrible and some of the songs are really bad
\(\bm{x}\)
This album is terrible and some of the songs are really bad
CREST-Generation
\(\hat{y}\)
NEG
Predictor

Trainable Masker
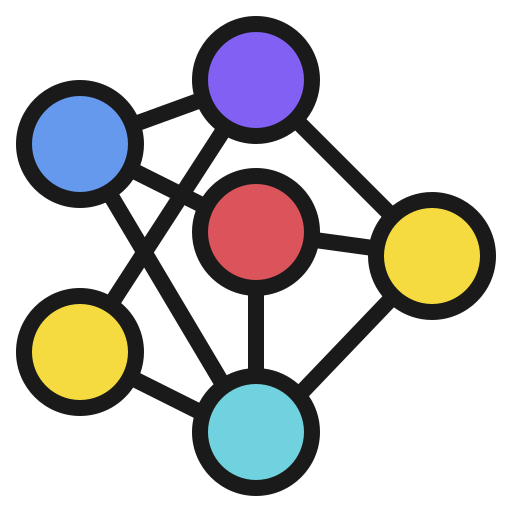
\(\bm{z}\)
This album is terrible and some of the songs are really bad
\(\bm{x}\)
POS: This album is MASK and some of the songs are MASK
This album is terrible and some of the songs are really bad
CREST-Generation
\(\hat{y}\)
NEG
Predictor

Trainable Masker
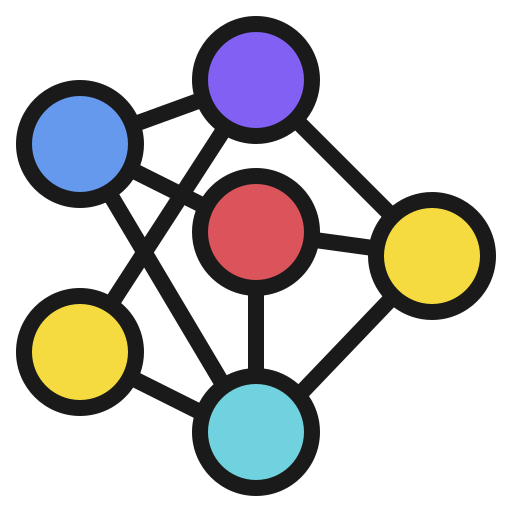
\(\bm{z}\)
This album is terrible and some of the songs are really bad
\(\bm{x}\)
\(\tilde{\bm{x}}\)
POS: This album is MASK and some of the songs are MASK

Editor
This album is terrible and some of the songs are really bad
This album is amazing and some of the songs are very well written
Experiments
• Masker: SPECTRA rationalizer [1]
– Controllable sparsity
– Contiguity
– Robustness
[1] Guerreiro, N.M. and Martins, A. F. T. SPECTRA: Sparse structured text rationalization. EMNLP 2021.
Experiments
• Masker: SPECTRA rationalizer [1]
• Editor: Pre-trained T5 model
– Controllable sparsity
– Contiguity
– Robustness
[1] Guerreiro, N.M. and Martins, A. F. T. SPECTRA: Sparse structured text rationalization. EMNLP 2021.
Experiments
• Masker: SPECTRA rationalizer [1]
• Editor: Pre-trained T5 model
• Two datasets: IMDB and SNLI
– Controllable sparsity
– Contiguity
– Robustness
[1] Guerreiro, N.M. and Martins, A. F. T. SPECTRA: Sparse structured text rationalization. EMNLP 2021.
Experiments
• Masker: SPECTRA rationalizer [1]
• Editor: Pre-trained T5 model
• Two datasets: IMDB and SNLI
– Controllable sparsity
– Contiguity
– Robustness
[1] Guerreiro, N.M. and Martins, A. F. T. SPECTRA: Sparse structured text rationalization. EMNLP 2021.
Experiments
• Masker: SPECTRA rationalizer [1]
• Editor: Pre-trained T5 model
• Two datasets: IMDB and SNLI
– Controllable sparsity
– Contiguity
– Robustness
[1] Guerreiro, N.M. and Martins, A. F. T. SPECTRA: Sparse structured text rationalization. EMNLP 2021.

Experiments
• Masker: SPECTRA rationalizer [1]
• Editor: Pre-trained T5 model
• Two datasets: IMDB and SNLI
– Controllable sparsity
– Contiguity
– Robustness
[1] Guerreiro, N.M. and Martins, A. F. T. SPECTRA: Sparse structured text rationalization. EMNLP 2021.

Experiments
• Masker: SPECTRA rationalizer [1]
• Editor: Pre-trained T5 model
• Two datasets: IMDB and SNLI
– Controllable sparsity
– Contiguity
– Robustness
[1] Guerreiro, N.M. and Martins, A. F. T. SPECTRA: Sparse structured text rationalization. EMNLP 2021.

Human Evaluation
• 100 examples (IMDB + SNLI)
• Validity and naturalness (e.g., based on style, tone, and grammar)
• 5-point Likert scale


Human Evaluation
• 100 examples (IMDB + SNLI)
• Validity and naturalness (e.g., based on style, tone, and grammar)
• 5-point Likert scale

Human Evaluation
• 100 examples (IMDB + SNLI)
• Validity and naturalness (e.g., based on style, tone, and grammar)
• 5-point Likert scale
How to leverage counterfactuals?
CREST-Generation
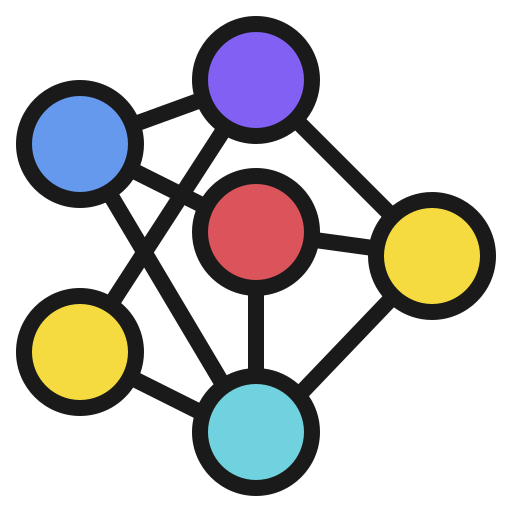

✅
High-quality counterfactuals
How to leverage counterfactuals?
CREST-Generation
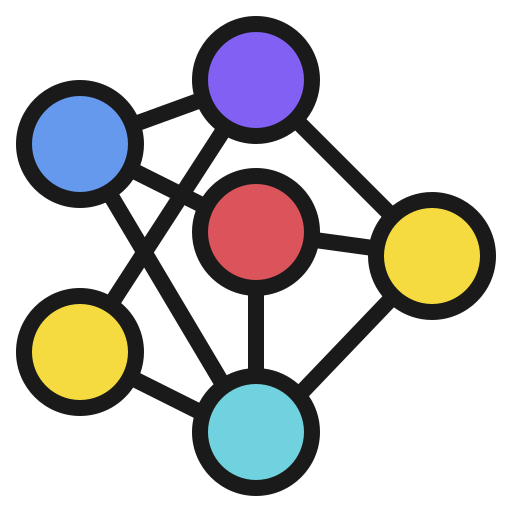

???
✅
High-quality counterfactuals
How to leverage counterfactuals?
CREST-Generation
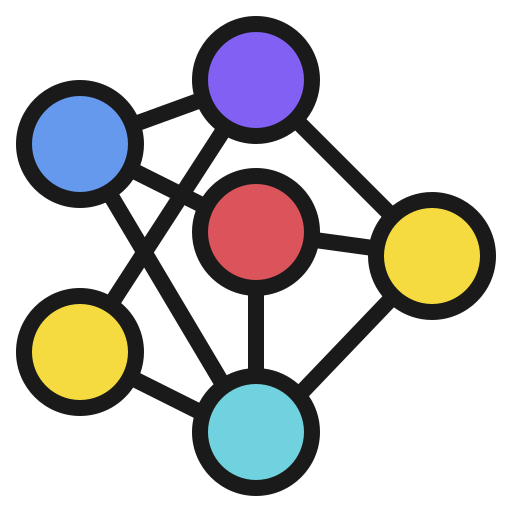

🤔
Data augmentation?
✅
High-quality counterfactuals
How to leverage counterfactuals?
CREST-Generation
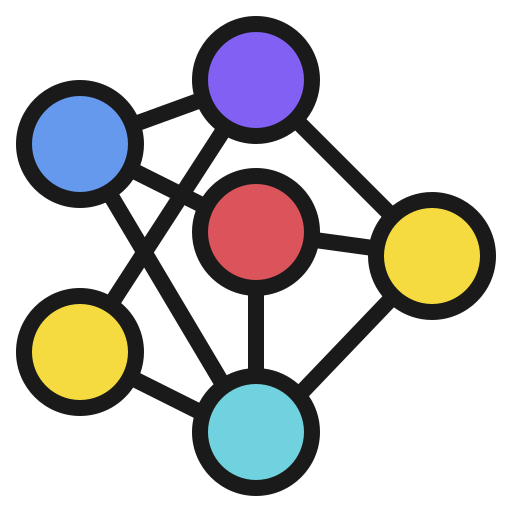

✅
High-quality counterfactuals
🥰
Exploit the paired structure of factual and counterfactual inputs
CREST-Rationalization
CREST-Rationalization
CREST-Generation
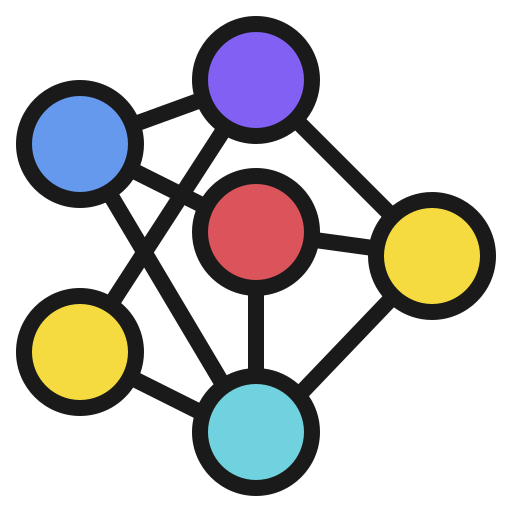

CREST-Rationalization
\(\bm{z}^\star\)
\(\bm{x}\)
This album is terrible and some of the songs are really bad
CREST-Generation
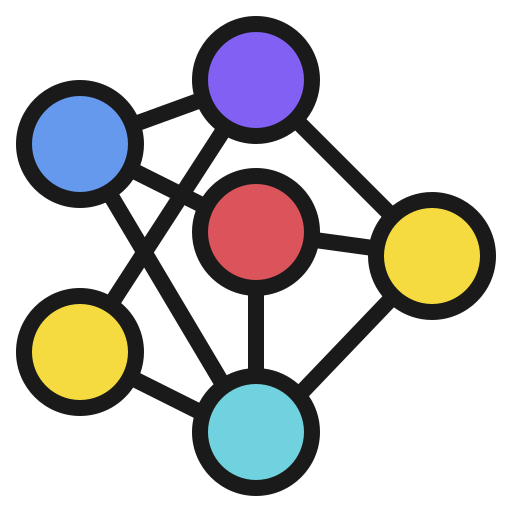

CREST-Rationalization
\(\bm{z}^\star\)
\(\bm{x}\)
This album is terrible and some of the songs are really bad
This album is amazing and some of the songs are very well written
CREST-Generation
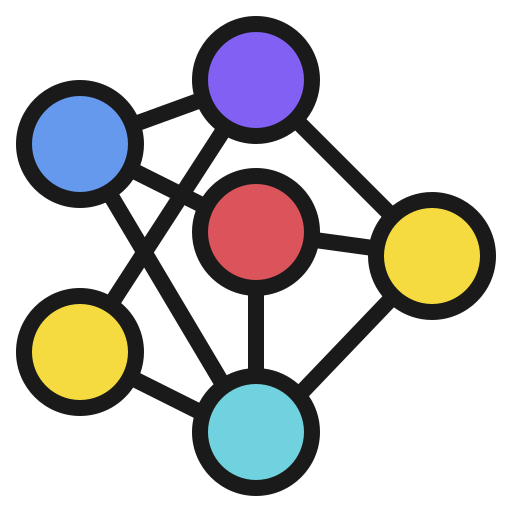

\(\tilde{\bm{x}}\)
\(\tilde{\bm{z}}^\star\)
CREST-Rationalization
\(\bm{z}^\star\)
\(\bm{x}\)
This album is terrible and some of the songs are really bad
This album is amazing and some of the songs are very well written
CREST-Generation
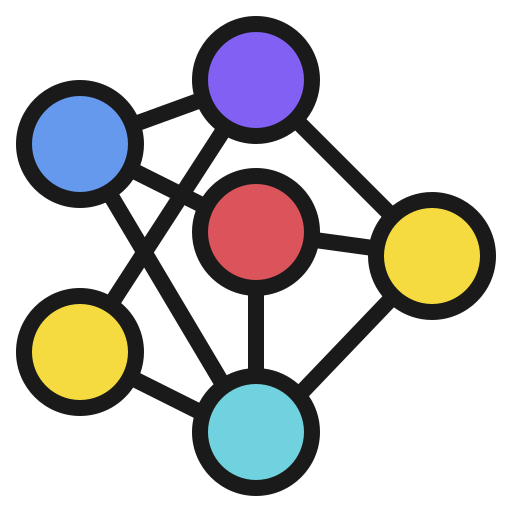

\(\tilde{\bm{x}}\)
\(\tilde{\bm{z}}^\star\)
FACTUAL
FLOW
COUNTERFACTUAL
FLOW
CREST-Rationalization
\(\bm{z}^\star\)
\(\bm{x}\)
This album is terrible and some of the songs are really bad
This album is amazing and some of the songs are very well written
CREST-Generation
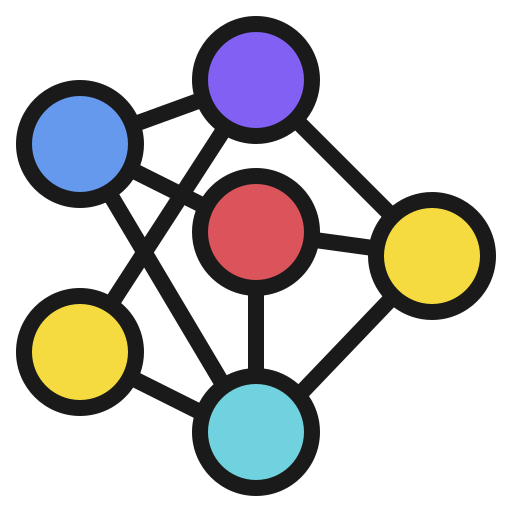

\(\tilde{\bm{x}}\)
\(\tilde{\bm{z}}^\star\)
FACTUAL
FLOW
COUNTERFACTUAL
FLOW
Trainable Masker
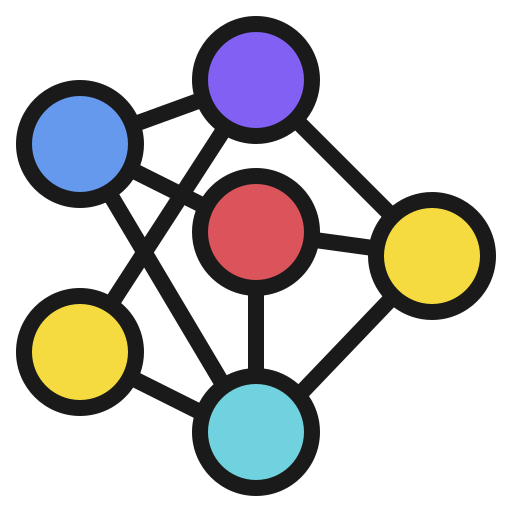
Trainable Masker
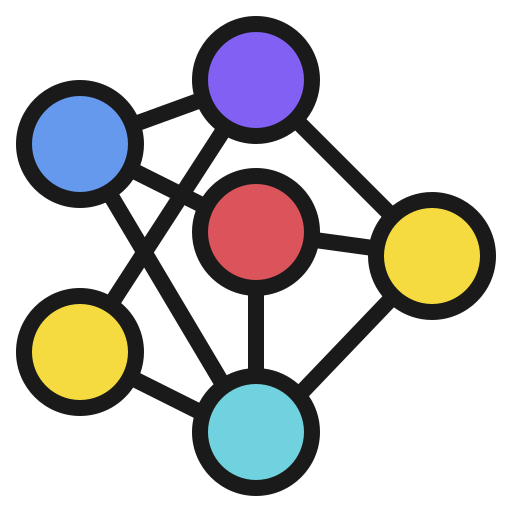
shared
CREST-Rationalization
\(\bm{z}^\star\)
\(\bm{x}\)
This album is terrible and some of the songs are really bad
This album is amazing and some of the songs are very well written
CREST-Generation
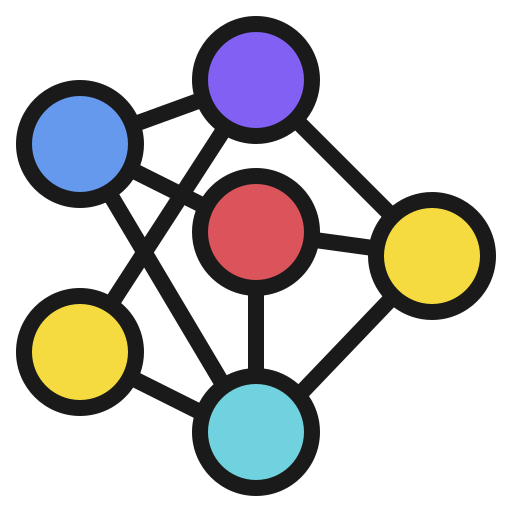

\(\tilde{\bm{x}}\)
\(\tilde{\bm{z}}^\star\)
FACTUAL
FLOW
COUNTERFACTUAL
FLOW
Trainable Masker
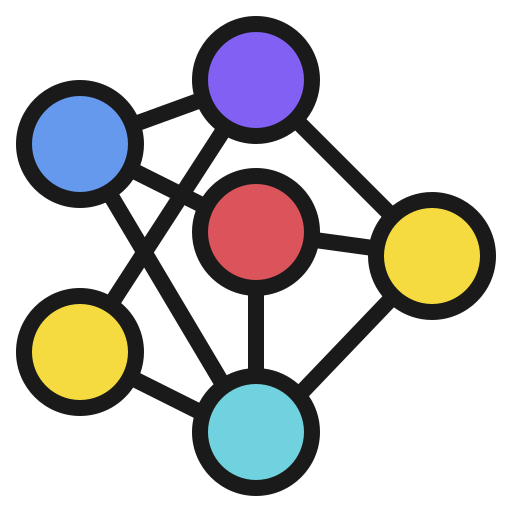
Trainable Masker
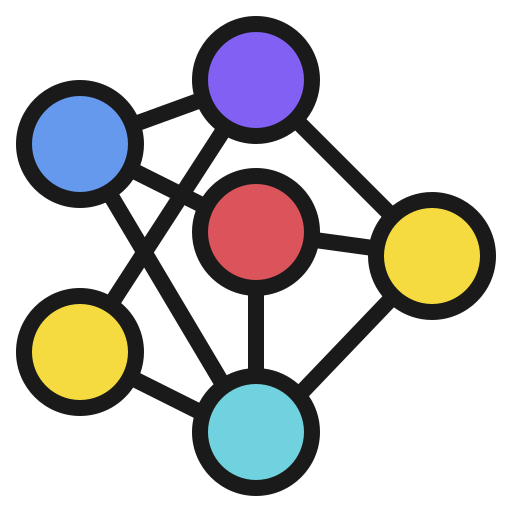
\(\bm{z}\)
\(\tilde{\bm{z}}\)
This album is terrible and some of the songs are really bad
This album is amazing and some of the songs are very well written
shared
CREST-Rationalization
\(\bm{z}^\star\)
\(\bm{x}\)
This album is terrible and some of the songs are really bad
This album is amazing and some of the songs are very well written
CREST-Generation
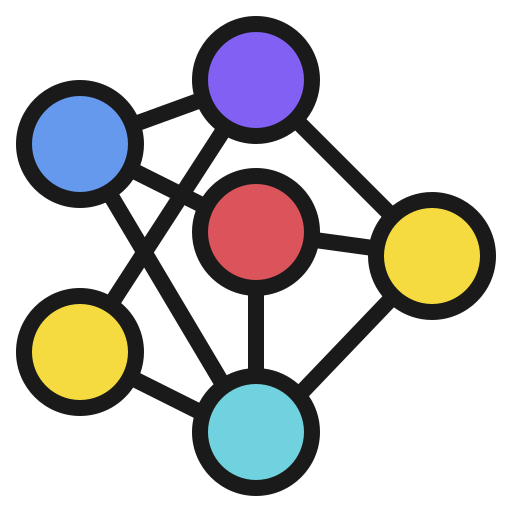

\(\tilde{\bm{x}}\)
\(\tilde{\bm{z}}^\star\)
FACTUAL
FLOW
COUNTERFACTUAL
FLOW
Trainable Masker
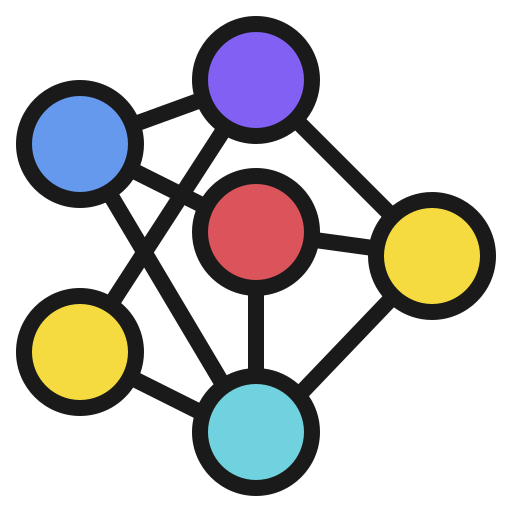
Trainable Masker
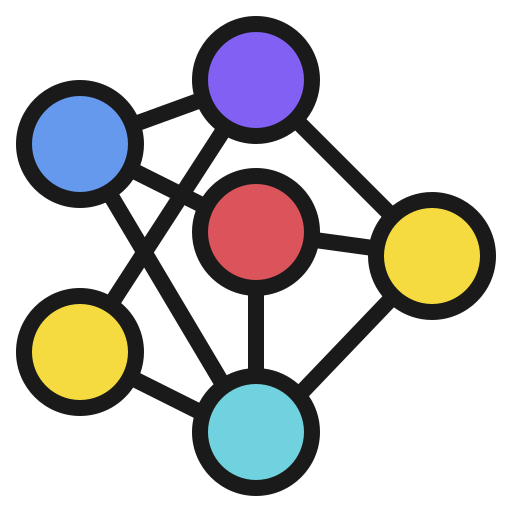
\(\bm{z}\)
\(\tilde{\bm{z}}\)
This album is terrible and some of the songs are really bad
This album is amazing and some of the songs are very well written
NEG
Predictor

POS
Predictor

\(\hat{y}\)
\(\tilde{y}\)
shared
shared
CREST-Rationalization
\(\bm{z}^\star\)
\(\bm{x}\)
This album is terrible and some of the songs are really bad
This album is amazing and some of the songs are very well written
CREST-Generation
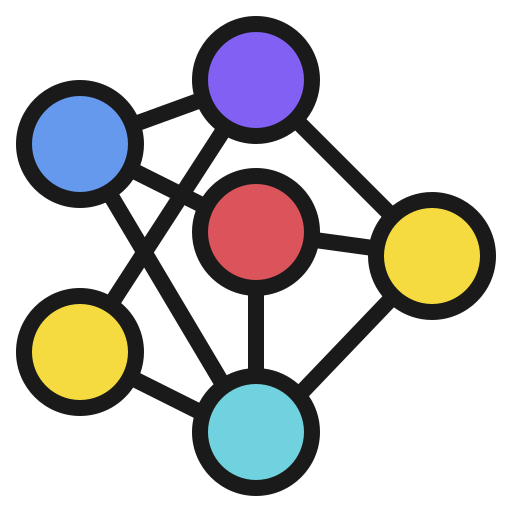

\(\tilde{\bm{x}}\)
\(\tilde{\bm{z}}^\star\)
FACTUAL
FLOW
COUNTERFACTUAL
FLOW
Trainable Masker
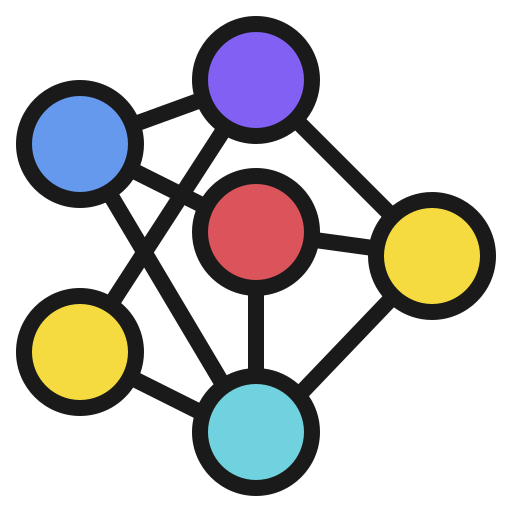
Trainable Masker
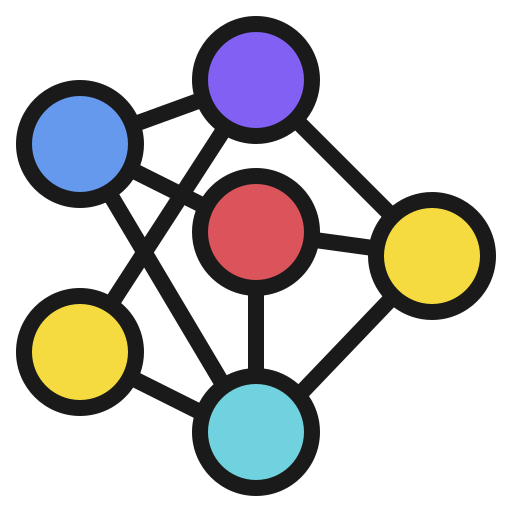
\(\bm{z}\)
\(\tilde{\bm{z}}\)
This album is terrible and some of the songs are really bad
This album is amazing and some of the songs are very well written
NEG
Predictor

POS
Predictor

\(\hat{y}\)
\(\tilde{y}\)
shared
shared
Agreement regularization:
factual similarity
counterfactual similarity
Experiments
• SPECTRA rationalizer as the masker
• Experiments on IMDB and SNLI

Original inputs
Original + Human CFs
Original + CREST CFs
CREST-Rationalization
Data Augmentation
in-domain
IMDB
Revised IMDB
Contrast IMDB
RotTom
SST-2
Amazon
Yelp
contrastive
out-of-domain
Experiments
• SPECTRA rationalizer as the masker
• Experiments on IMDB and SNLI

Original inputs
Original + Human CFs
Original + CREST CFs
CREST-Rationalization
Data Augmentation
in-domain
IMDB
Revised IMDB
Contrast IMDB
RotTom
SST-2
Amazon
Yelp
contrastive
out-of-domain
Experiments
• SPECTRA rationalizer as the masker
• Experiments on IMDB and SNLI

Original inputs
Original + Human CFs
Original + CREST CFs
CREST-Rationalization
Data Augmentation
in-domain
IMDB
Revised IMDB
Contrast IMDB
RotTom
SST-2
Amazon
Yelp
contrastive
out-of-domain
Experiments
• SPECTRA rationalizer as the masker
• Experiments on IMDB and SNLI

Original inputs
Original + Human CFs
Original + CREST CFs
CREST-Rationalization
Data Augmentation
in-domain
IMDB
Revised IMDB
Contrast IMDB
RotTom
SST-2
Amazon
Yelp
contrastive
out-of-domain
Experiments
• SPECTRA rationalizer as the masker
• Experiments on IMDB and SNLI

Original inputs
Original + Human CFs
Original + CREST CFs
CREST-Rationalization
Data Augmentation
in-domain
IMDB
Revised IMDB
Contrast IMDB
RotTom
SST-2
Amazon
Yelp
contrastive
out-of-domain
Experiments
• SPECTRA rationalizer as the masker
• Experiments on IMDB and SNLI

Original inputs
Original + Human CFs
Original + CREST CFs
CREST-Rationalization
Data Augmentation
in-domain
IMDB
Revised IMDB
Contrast IMDB
RotTom
SST-2
Amazon
Yelp
contrastive
out-of-domain
Experiments
• SPECTRA rationalizer as the masker
• Experiments on IMDB and SNLI

Original inputs
Original + Human CFs
Original + CREST CFs
CREST-Rationalization
Data Augmentation
in-domain
IMDB
Revised IMDB
Contrast IMDB
RotTom
SST-2
Amazon
Yelp
contrastive
out-of-domain
Interpretability Analysis
Are the rationales generated by CREST-Rationalization interpretable?
Interpretability Analysis
Are the rationales generated by CREST-Rationalization interpretable?
• Plausibility (human-likeness)
• Forward simulability (informativeness)
• Counterfactual simulability:
Interpretability Analysis
Are the rationales generated by CREST-Rationalization interpretable?
input with infilled tokens by \(G\) in the positions marked by \(\bm{z}\)
• Plausibility (human-likeness)
• Forward simulability (informativeness)
• Counterfactual simulability:
ability of a rationale \(\bm{z}\) to change the classifier's prediction when the classifier receives a rationale-guided contrastive edit as input
Interpretability Analysis
Are the rationales generated by CREST-Rationalization interpretable?
\(F\)
\(F + C_H\)
\(F + C_S\)
\(F \,\&\, C_S\)
0.6733 ± 0.02 91.70 ± 0.92 81.18 ± 2.79
0.6718 ± 0.04 91.44 ± 1.46 80.53 ± 4.17
0.6758 ± 0.01 91.68 ± 0.59 84.54 ± 1.09
0.6904 ± 0.02 91.93 ± 0.83 86.43 ± 1.56
Setup
Plausibility
(AUC)
Forward Sim.
(ACC)
Counterfactual Sim.
(ACC)
Data augmentation:
CREST-Rationalization:
Interpretability Analysis
Are the rationales generated by CREST-Rationalization interpretable?
\(F\)
\(F + C_H\)
\(F + C_S\)
\(F \,\&\, C_S\)
0.6733 ± 0.02 91.70 ± 0.92 81.18 ± 2.79
0.6718 ± 0.04 91.44 ± 1.46 80.53 ± 4.17
0.6758 ± 0.01 91.68 ± 0.59 84.54 ± 1.09
0.6904 ± 0.02 91.93 ± 0.83 86.43 ± 1.56
Setup
Plausibility
(AUC)
Forward Sim.
(ACC)
Counterfactual Sim.
(ACC)
Data augmentation:
CREST-Rationalization:
Interpretability Analysis
Are the rationales generated by CREST-Rationalization interpretable?
\(F\)
\(F + C_H\)
\(F + C_S\)
\(F \,\&\, C_S\)
0.6733 ± 0.02 91.70 ± 0.92 81.18 ± 2.79
0.6718 ± 0.04 91.44 ± 1.46 80.53 ± 4.17
0.6758 ± 0.01 91.68 ± 0.59 84.54 ± 1.09
0.6904 ± 0.02 91.93 ± 0.83 86.43 ± 1.56
Setup
Plausibility
(AUC)
Forward Sim.
(ACC)
Counterfactual Sim.
(ACC)
Data augmentation:
CREST-Rationalization:
Conclusions
• produces valid, fluent, and diverse counterfactuals
• controls the amount of perturbation
• leads to plausible explanations
• achieves high counterfactual simulability


Selective Rationalization ❤︎ Counterfactual Generation
Experiments
• Masker: SPECTRA rationalizer [1]
• Editor: Pre-trained T5 model
[1] Guerreiro, N.M. and Martins, A. F. T. SPECTRA: Sparse structured text rationalization. EMNLP 2021.
– Controllable sparsity
– Contiguity
– Robustness

Experiments
• Masker: SPECTRA rationalizer [1]
• Editor: Pre-trained T5 model
[1] Guerreiro, N.M. and Martins, A. F. T. SPECTRA: Sparse structured text rationalization. EMNLP 2021.
– Controllable sparsity
– Contiguity
– Robustness

validity
fluency
diversity
closeness
Experiments
• Masker: SPECTRA rationalizer [1]
• Editor: Pre-trained T5 model
[1] Guerreiro, N.M. and Martins, A. F. T. SPECTRA: Sparse structured text rationalization. EMNLP 2021.
– Controllable sparsity
– Contiguity
– Robustness

validity
fluency
diversity
closeness
Experiments
• Masker: SPECTRA rationalizer [1]
• Editor: Pre-trained T5 model
[1] Guerreiro, N.M. and Martins, A. F. T. SPECTRA: Sparse structured text rationalization. EMNLP 2021.
– Controllable sparsity
– Contiguity
– Robustness

validity
fluency
diversity
closeness
Experiments
• Masker: SPECTRA rationalizer [1]
• Editor: Pre-trained T5 model
[1] Guerreiro, N.M. and Martins, A. F. T. SPECTRA: Sparse structured text rationalization. EMNLP 2021.
– Controllable sparsity
– Contiguity
– Robustness

validity
fluency
diversity
closeness
Experiments
• SPECTRA rationalizer as the masker
• Experiments on IMDB and SNLI
in-domain contrast out-of-domain
Experiments
• SPECTRA rationalizer as the masker
• Experiments on IMDB and SNLI
in-domain contrast out-of-domain
\(F\)
\(F + C_H\)
\(F + C_S\)
\(F \,\&\, C_S\)
Data augmentation:
CREST-Rationalization:
Setup
IMDB
Revised IMDB
Contrast IMDB
RotTom
SST-2
Amazon
Yelp
Experiments
• SPECTRA rationalizer as the masker
• Experiments on IMDB and SNLI
in-domain contrast out-of-domain
\(F\)
\(F + C_H\)
\(F + C_S\)
\(F \,\&\, C_S\)
Data augmentation:
CREST-Rationalization:
Setup
IMDB
Revised IMDB
Contrast IMDB
RotTom
SST-2
Amazon
Yelp
Experiments
• SPECTRA rationalizer as the masker
• Experiments on IMDB and SNLI
in-domain contrast out-of-domain
\(F\)
\(F + C_H\)
\(F + C_S\)
\(F \,\&\, C_S\)
Data augmentation:
CREST-Rationalization:
Setup
IMDB
Revised IMDB
Contrast IMDB
RotTom
SST-2
Amazon
Yelp
Experiments
• SPECTRA rationalizer as the masker
• Experiments on IMDB and SNLI
in-domain contrast out-of-domain
\(F\)
\(F + C_H\)
\(F + C_S\)
\(F \,\&\, C_S\)
Data augmentation:
CREST-Rationalization:
Setup
IMDB
Revised IMDB
Contrast IMDB
RotTom
SST-2
Amazon
Yelp
Experiments
• SPECTRA rationalizer as the masker
• Experiments on IMDB and SNLI
in-domain contrast out-of-domain
\(F\)
\(F + C_H\)
\(F + C_S\)
\(F \,\&\, C_S\)
Data augmentation:
CREST-Rationalization:
Setup
IMDB
Revised IMDB
Contrast IMDB
RotTom
SST-2
Amazon
Yelp
Experiments
• SPECTRA rationalizer as the masker
• Experiments on IMDB and SNLI
in-domain contrast out-of-domain
\(F\)
\(F + C_H\)
\(F + C_S\)
\(F \,\&\, C_S\)
91.1 ± 0.3 91.4 ± 0.8 88.5 ± 0.9 76.5 ± 1.6 79.8 ± 1.6 86.0 ± 0.7 88.5 ± 0.7
90.9 ± 0.5 92.9 ± 0.9 90.4 ± 1.6 76.6 ± 1.5 80.7 ± 1.3 86.3 ± 1.0 89.1 ± 1.2
90.8 ± 0.2 91.6 ± 1.3 89.2 ± 0.4 76.7 ± 1.0 80.6 ± 0.6 86.4 ± 0.6 89.1 ± 0.5
91.2 ± 0.5 92.9 ± 0.5 89.7 ± 1.1 77.3 ± 2.3 81.1 ± 2.4 86.8 ± 0.8 89.3 ± 0.7
Data augmentation:
CREST-Rationalization:
Setup
IMDB
Revised IMDB
Contrast IMDB
RotTom
SST-2
Amazon
Yelp
Experiments
• SPECTRA rationalizer as the masker
• Experiments on IMDB and SNLI
in-domain contrast out-of-domain
\(F\)
\(F + C_H\)
\(F + C_S\)
\(F \,\&\, C_S\)
91.1 ± 0.3 91.4 ± 0.8 88.5 ± 0.9 76.5 ± 1.6 79.8 ± 1.6 86.0 ± 0.7 88.5 ± 0.7
90.9 ± 0.5 92.9 ± 0.9 90.4 ± 1.6 76.6 ± 1.5 80.7 ± 1.3 86.3 ± 1.0 89.1 ± 1.2
90.8 ± 0.2 91.6 ± 1.3 89.2 ± 0.4 76.7 ± 1.0 80.6 ± 0.6 86.4 ± 0.6 89.1 ± 0.5
91.2 ± 0.5 92.9 ± 0.5 89.7 ± 1.1 77.3 ± 2.3 81.1 ± 2.4 86.8 ± 0.8 89.3 ± 0.7
Data augmentation:
CREST-Rationalization:
Setup
IMDB
Revised IMDB
Contrast IMDB
RotTom
SST-2
Amazon
Yelp
CREST
By mtreviso
CREST
CREST: A Joint Framework for Rationalization and Counterfactual Text Generation. ACL 2023
- 137