Szabo Roland Teodor
UBB
A Novel Machine Learning Based Approach for Retrieving Information from Receipt Images
OuR Aims
- investigate machine learning algorithms for creating an Optical Character Recognition engine tailored for receipts
- use that OCR engine to create an application that simplifies personal finance manangement
- compare to other OCR engines
Problem Statement
OCR
Best free and open source OCR engine: Tesseract
A Mathematical Theory of Communication, C.E. Shannon
The recent development of various methods of modulation such as PCM and PPM which exchange bandwith for signal-to-noise ratio has ....

- document layout analysis
- character segmentation
- character recognition
Our Model
Using raw pixel data, no feature extraction
Random forests for character segmentation
-
tried between 150 and 250 trees
- number of features used between 8 and 120
Suppor Vector Machine for character recognition
- linear and RBF kernel
- regularization from 0.01 to 10000 on a log scale
Dataset
- 20 receipts were annotated by hand
- ~7000 characters
- 74 classes (different characters)
BUILT USING:
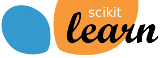


All three free, open-source libraries for scientific computing, machine learning and computer vision
results
Character Recognition
- best accuracy with RBF kernel and regularization of 100: 91.01% on validation set
- regularization is a must for RBF - using a value of 0.01 lead to accuracy of 9.16%
- it matters less for linear kernel - accuracy between 70% and 89.5%
Most common missclassifications
- , and . - 40 times
- O and 0 - 39 times
- 1, l and I - 14 times
Character segmentation
- best F1 score of 87.936% on validation set
- number of trees or features influenced < 0.1%
- consistent with theory established by Leo Breiman
Confusion Matrix
|
No split
|
Split
|
Predicted no split
|
4556
|
363
|
Predicted split
|
255
|
2546
|
Model is more specific, rather than sensitive
Comparison To other REsults
- recognition results obtained by others on MNIST are better
- SVM with RBF kernel - 98.6% accuracy
- state-of-the-art - 99.7%
- 10x bigger dataset
- 7x fewer classes
- segmentation results for license plate are better
- 96% accuracy
- fixed number of letters to be segmented
Tesseract
ReceiptBudget
S.C. HRTIHH 5.9. ELUJ NHPUCH. STR. BUCEGI. NR. 19 9.9.1. 99 11735629 RUN 1.999 x 3.19 BRTISTE N9Z.CLHS|C3S 3.19 9 1.999 x 2.59 STICKLETTI CHRTUF 99 2.59 9 9.399 x 7.99 HHNDHRINE 3.12 H 9.446 x 7.99 99511 3.56 9 1.999 x 11.29 SHLHH C959 USCHT 299 11.29 9 SUBTUTHL 23.5? SUBTUTRL _____ ‘-29:99 TUTHL 23.57
S.C. ARTIMA S.A. CLUJMAPOCA, STR. BUCEGI, NR.19 C .U.1,R O1 1735628 RON 1.000 x 3,19 BATISTE NAZ.CLASIC3S 3,19% 1,000x 2,50 STICNLETTI CARTOF 80 2,50 A 0,390 x 7,99 MANDARINE 3,12A 0,446 x 7,99 R0SI 3,56 A 1.000 x 11,20 SALANIASAOSCAT 290 11,20A SUBTOTAL 23,57 SUBTOTAL 23,57 TOTAL 27,57
The Application
- ReceiptBudget has an interactive dashboard
- The goal is to get some insight into spending patterns
THE map
The charts

Built USING

Conclusion
- OCR results are better than by using off-the-shelf components
- but there is still some work to do
- deep learning shows promise
- need a larger dataset
- I saved some money by looking at those graphs :)
Questions?
SCSS
By rolisz
SCSS
- 1,632