


Open data travel demand synthesis
Sebastian Hörl
9 June 2021
Seminar Series Chaire Anthropolis / Future Cities Lab
The street in 1900

http://www.loc.gov/pictures/item/2016800172/
The street today

https://commons.wikimedia.org/wiki/File:Atlanta_75.85.jpg
The street of tomorrow?

Julius Bär / Farner
Classic transport planning



- Zones
- Flows
- Peak hours
- User groups
Aggregated
Agent-based models

0:00 - 8:00
08:30 - 17:00
17:30 - 0:00
0:00 - 9:00
10:00 - 17:30
17:45 - 21:00
22:00 - 0:00
- Discrete locations
- Individual travelers
- Individual behaviour
- Whole day analysis
Disaggregated
Icons on this and following slides: https://fontawesome.com
Synthetic travel demand

Population census (RP)

Population census (RP)
Income data (FiLoSoFi)
Synthetic travel demand

Population census (RP)
Income data (FiLoSoFi)
Commuting data (RP-MOB)
Synthetic travel demand

Population census (RP)
Income data (FiLoSoFi)
Commuting data (RP-MOB)
Household travel survey (EDGT)
0:00 - 8:00
08:30 - 17:00
17:30 - 0:00
0:00 - 9:00
10:00 - 17:30
17:45 - 21:00
22:00 - 0:00
Synthetic travel demand

Population census (RP)
Income data (FiLoSoFi)
Commuting data (RP-MOB)
Household travel survey (EDGT)
Enterprise census (SIRENE)
Address database (BD-TOPO)
Synthetic travel demand

Population census (RP)
Income data (FiLoSoFi)
Commuting data (RP-MOB)
Household travel survey (EDGT)
Enterprise census (SIRENE)
Address database (BD-TOPO)
Person ID
Age
Gender
Home (X,Y)
1
43
male
(65345, ...)
2
24
female
(65345, ...)
3
9
female
(65345, ...)

Synthetic travel demand

Population census (RP)
Income data (FiLoSoFi)
Commuting data (RP-MOB)
Household travel survey (EDGT)
Enterprise census (SIRENE)
Address database (BD-TOPO)
Person ID
Activity
Start
End
Loc.
523
home
08:00
(x,y)
523
work
08:55
18:12
(x,y)
523
shop
19:10
19:25
(x,y)
523
home
19:40
(x,y)

Synthetic travel demand

Population census (RP)
Income data (FiLoSoFi)
Commuting data (RP-MOB)
Household travel survey (EDGT)
Enterprise census (SIRENE)
OpenStreetMap
GTFS (SYTRAL / SNCF)
Address database (BD-TOPO)

Synthetic travel demand

Population census (RP)
Income data (FiLoSoFi)
Commuting data (RP-MOB)
Household travel survey (EDGT)
Enterprise census (SIRENE)
OpenStreetMap
GTFS (SYTRAL / SNCF)
Address database (BD-TOPO)
Synthetic travel demand

Population census (RP)
Income data (FiLoSoFi)
Commuting data (RP-MOB)
National HTS (ENTD)
Enterprise census (SIRENE)
OpenStreetMap
GTFS (SYTRAL / SNCF)
Address database (BD-TOPO)
Synthetic travel demand
EDGT

Population census (RP)
Income data (FiLoSoFi)
Commuting data (RP-MOB)
Enterprise census (SIRENE)
OpenStreetMap
GTFS (SYTRAL / SNCF)
Address database (BD-TOPO)
Synthetic travel demand
Open
Data
Open
Software
+
=
Reproducible research
Integrated testing
National HTS (ENTD)
EDGT

Population census (RP)
Income data (FiLoSoFi)
Commuting data (RP-MOB)
Enterprise census (SIRENE)
OpenStreetMap
GTFS (SYTRAL / SNCF)
Address database (BD-TOPO)
Synthetic travel demand
Open
Data
Open
Software
+
=
Reproducible research
Integrated testing

National HTS (ENTD)
EDGT

Current use cases


Nantes
- Noise modeling


Current use cases

Lille
- Park & ride applications
- Road pricing



Current use cases


Toulouse
- Placement and use of shared offices

Current use cases

Rennes
- Micromobility simulation



Current use cases



Paris / Île-de-France
- Scenario development for sustainable urban transformation
- New mobility services
Mahdi Zargayouna (GRETTIA / Univ. Gustave Eiffel)
Nicolas Coulombel (LVMT / ENPC)

Current use cases



Paris / Île-de-France
- Cycling simulation

Current use cases


Paris / Île-de-France
- Simulation of dynamic mobility services
- Fleet control through reinforcement learning

Current use cases



Lyon (IRT SystemX)
- Low-emission first/last mile logistics

Current use cases




Current use cases



Balac, M., Hörl, S. (2021) Synthetic population for the state of California based on open-data: examples of San Francisco Bay area and San Diego County, presented at 100th Annual Meeting of the Transportation Research Board, Washington, D.C.
Sallard, A., Balac, M., Hörl, S. (2021) Synthetic travel demand for the Greater São Paulo Metropolitan Region, based on open data, Under Review
Sao Paulo, San Francisco Bay area, Los Angeles five-county area, Switzerland, Montreal, Quebec City, Jakarta, Casablanca, ...

MATSim



- Flexible, extensible and well-tested open-source transport simulation framework
- Used by many research groups and companies all over the world
- Extensions for parking behaviour, signal control, location choice, freight, ...

matsim-org/matsim-libs














MATSim



Synthetic demand

MATSim



Mobility simulation
Synthetic demand

MATSim
Decision-making



10:00 - 17:30
17:45 - 21:00
22:00 - 0:00
Mobility simulation
Synthetic demand

MATSim
Decision-making



Mobility simulation
Synthetic demand


MATSim
Decision-making



Mobility simulation
Analysis
Synthetic demand
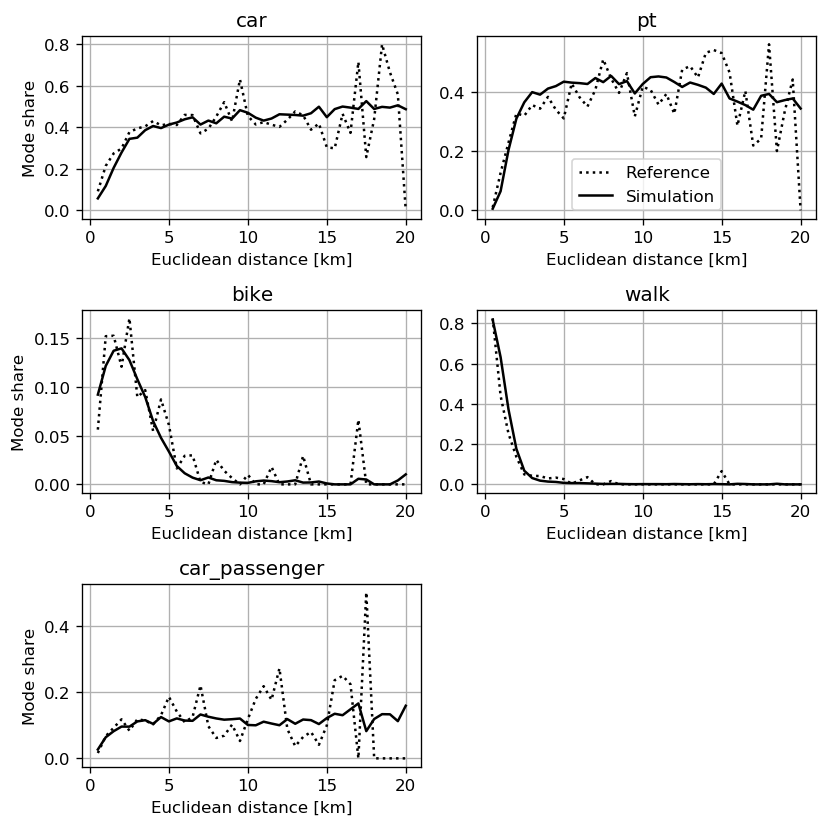

Use cases: Emissions in Île-de-France





Use cases: Automated taxis in Paris





Use cases: Automated taxis in Paris






Use cases: Grand Paris Express




- Sustainable first-/last-mile delivery services in the Confluence quarter of Lyon
- Focus on household parcel delivery
- Need for demand estimation
- Detailed understanding of operational constraints for a delivery service
LEAD: Study idea




Parcel demand

Synthetic population
Out-of-home purchase survey
Achats découplés des ménages
Gardrat, M., 2019. Méthodologie d’enquête: le découplage de l’achat et de la récupération des marchandises par les ménages. LAET, Lyon, France.
+


Parcel demand

Synthetic population
Gardrat, M., 2019. Méthodologie d’enquête: le découplage de l’achat et de la récupération des marchandises par les ménages. LAET, Lyon, France.
+

Out-of-home purchase survey
Achats découplés des ménages

Parcel demand

Synthetic population
Gardrat, M., 2019. Méthodologie d’enquête: le découplage de l’achat et de la récupération des marchandises par les ménages. LAET, Lyon, France.
+

Out-of-home purchase survey
Achats découplés des ménages

Parcel demand

Synthetic population
Gardrat, M., 2019. Méthodologie d’enquête: le découplage de l’achat et de la récupération des marchandises par les ménages. LAET, Lyon, France.
+

Out-of-home purchase survey
Achats découplés des ménages

Parcel demand

Synthetic population
Gardrat, M., 2019. Méthodologie d’enquête: le découplage de l’achat et de la récupération des marchandises par les ménages. LAET, Lyon, France.
+
Synthetic population
Iterative proportional fitting (IFP)
- Based on synthetic population, find average number of purchases delivered to a household defined by socioprofesional class, age of the reference person and household size per day.

Out-of-home purchase survey
Achats découplés des ménages

Parcel demand

Synthetic population
Gardrat, M., 2019. Méthodologie d’enquête: le découplage de l’achat et de la récupération des marchandises par les ménages. LAET, Lyon, France.
+
Synthetic population
Maximum entropy approach
- We now the average number of parcels, but we do not now the distribution of the number of parcels for a household on an average day.
- We know it must be non-negative, and we know the mean.
- Without additional data, we assume maximum entropy distribution, which is Exponential in this case.
Out-of-home purchase survey
Achats découplés des ménages

Parcel demand

Synthetic population
Gardrat, M., 2019. Méthodologie d’enquête: le découplage de l’achat et de la récupération des marchandises par les ménages. LAET, Lyon, France.
+
Synthetic population

Out-of-home purchase survey
Achats découplés des ménages

Parcel demand

Synthetic population
Gardrat, M., 2019. Méthodologie d’enquête: le découplage de l’achat et de la récupération des marchandises par les ménages. LAET, Lyon, France.
+
Synthetic population

Out-of-home purchase survey
Achats découplés des ménages

Parcel demand

Synthetic population
Gardrat, M., 2019. Méthodologie d’enquête: le découplage de l’achat et de la récupération des marchandises par les ménages. LAET, Lyon, France.
+
Synthetic population

Out-of-home purchase survey
Achats découplés des ménages

Parcel demand

Synthetic population
Gardrat, M., 2019. Méthodologie d’enquête: le découplage de l’achat et de la récupération des marchandises par les ménages. LAET, Lyon, France.
+
Synthetic population

Out-of-home purchase survey
Achats découplés des ménages

Routing problem

Vehicle routing problem with time windows (VRPTW)
- We have a fleet of N vehicles (capacity, speed).
- They need to delivery the parcels when somebody is home (i.e. they need to respect time windows).
- Problem: Find optimal route that minimizes the distance covered while fulfilling the criteria.


Routing problem

Vehicle routing problem with time windows (VRPTW)
- We have a fleet of N vehicles (capacity, speed).
- They need to delivery the parcels when somebody is home (i.e. they need to respect time windows).
- Problem: Find optimal route that minimizes the distance covered while fulfilling the criteria.


Routing problem

Vehicle routing problem with time windows (VRPTW)
- We have a fleet of N vehicles (capacity, speed).
- They need to delivery the parcels when somebody is home (i.e. they need to respect time windows).
- Problem: Find optimal route that minimizes the distance covered while fulfilling the criteria.



First case study

- Synthetic population parcel
demand (101 parcels)
- Parcel size: 1
- Available vehicles: 10
- Vehicle capacity: 5
- Vehicle speed: 10 km/h
- Pickup time (at depot):
60s
- Delivery time (at customer):
5min
- Minimize driven distance

First case study

- Synthetic population parcel
demand (101 parcels)
- Parcel size: 1
- Available vehicles: 10
- Vehicle capacity: 5
- Vehicle speed: 10 km/h
- Pickup time (at depot):
60s
- Delivery time (at customer):
5min
- Minimize driven distance
- Three vehicles used
- Total distance 41.35 km

Scaling up to the digital twin

Synthetic popualtion
- Simulation of Lyon (and Rhônes-Alpes) with influence of traffic, including freight traffic
MATSim
MASS-GT
- Simulation of shipper behaviour (B2C and B2B)
- For instance: What will be the effect of a zero-emission zone in the city on traffic?

Scaling up to the digital twin


- Simulation of Lyon (and Rhônes-Alpes) with influence of traffic, including freight traffic
- Simulation of shipper behaviour (B2C and B2B)
- For instance: What will be the effect of a zero-emission zone in the city on traffic?

Questions?




Open data travel demand synthesis
By Sebastian Hörl
Open data travel demand synthesis
9 June 2021, Seminar Series Chaire Anthropolis / Future Cities Lab
- 1,234