


Parcel delivery simulations for Lyon
Sebastian Hörl
11 May 2021
H2020 Project LEAD
- Sustainable first-/last-mile delivery services in the Confluence quarter of Lyon
- Focus on household parcel delivery
- Need for demand estimation
- Detailed understanding of operational constraints for a delivery service
Study idea



Synthetic population

Population census (RP)
Income data (FiLoSoFi)
Commuting data (RP-MOB)
Household travel survey (EDGT)
Enterprise census (SIRENE)
OpenStreetMap
GTFS (SYTRAL / SNCF)
Address database (BD-TOPO)

Parcel demand

Synthetic population
Out-of-home purchase survey
Achats découplés des ménages
Gardrat, M., 2019. Méthodologie d’enquête: le découplage de l’achat et de la récupération des marchandises par les ménages. LAET, Lyon, France.
+




Parcel demand

Synthetic population
Gardrat, M., 2019. Méthodologie d’enquête: le découplage de l’achat et de la récupération des marchandises par les ménages. LAET, Lyon, France.
+
Synthetic population

Out-of-home purchase survey
Achats découplés des ménages
Parcel demand

Synthetic population
Gardrat, M., 2019. Méthodologie d’enquête: le découplage de l’achat et de la récupération des marchandises par les ménages. LAET, Lyon, France.
+
Synthetic population

Out-of-home purchase survey
Achats découplés des ménages
Parcel demand

Synthetic population
Gardrat, M., 2019. Méthodologie d’enquête: le découplage de l’achat et de la récupération des marchandises par les ménages. LAET, Lyon, France.
+
Synthetic population

Out-of-home purchase survey
Achats découplés des ménages
Parcel demand

Synthetic population
Gardrat, M., 2019. Méthodologie d’enquête: le découplage de l’achat et de la récupération des marchandises par les ménages. LAET, Lyon, France.
+
Synthetic population

Out-of-home purchase survey
Achats découplés des ménages
First case study

- Synthetic population parcel
demand (101 parcels)
- Parcel size: 1
- Available vehicles: 10
- Vehicle capacity: 5
- Vehicle speed: 10 km/h
- Pickup time (at depot):
60s
- Delivery time (at customer):
5min
- Minimize driven distance
- Three vehicles used
- Total distance 41.35 km
Next steps

Analysis
- Define baseline case
- Define more realistic cases (capacities, parcel sizes, ...)
- Run series of scenarios (estimating hub size, ...)
- Refinement of scenario with LL partners
Technical
- More refined solution algorithms (charging stations, ...)
Future scenarios
- General population growth up to 2050
- Specific development of Confluence area
Scaling up to the digital twin

Synthetic popualtion
- Simulation of Lyon (and Rhônes-Alpes) with influence of traffic, including freight traffic
MATSim
MASS-GT
- Simulation of shipper behaviour (B2C and B2B)
- For instance: What will be the effect of a zero-emission zone in the city on traffic?
Scaling up to the digital twin

- Simulation of Lyon (and Rhônes-Alpes) with influence of traffic, including freight traffic
- Simulation of shipper behaviour (B2C and B2B)
- For instance: What will be the effect of a zero-emission zone in the city on traffic?

Questions?




Baseline
Study idea

- Synthetic population
- Generated deliveries
- Integration of background freight traffic
Baseline
Study idea

Hub analysis
Case scenarios
- Delivery problem simulation
- Sizing of distribution center
- Sizing of distribution fleet
- Size of distribution vehicles
- Shared of deliveries
(Strict / soft zero-emission zone)
- Which configurations are feasible and effective?
- Synthetic population
- Generated deliveries
- Integration of background freight traffic
Baseline
- Synthetic population
- Generated deliveries
- Integration of background freight traffic
Study idea

Hub analysis
Case scenarios
- Delivery problem simulation
- Sizing of distribution center
- Sizing of distribution fleet
- Size of distribution vehicles
- Shared of deliveries
(Strict / soft zero-emission zone)
- Which configurations are feasible and effective?
Hub analysis
Future scenarios
- Increase of population
- Change of demographics
- ... and generated deliveries
- Static increase of background freight (Interface Transport)
+
Baseline
- Synthetic population
- Generated deliveries
Study idea

Hub analysis
Case scenarios
Hub analysis
Future scenarios
- Increase of population
- Change of demographics
- ... and generated deliveries
- Static increase of background freight (Interface Transport)
+
- Integration of background freight traffic
- Sizing of distribution center
- Sizing of distribution fleet
- Size of distribution vehicles
- Shared of deliveries
(Strict / soft zero-emission zone)
- Which configurations are feasible and effective?
- Delivery problem simulation
Synthetic population

Population census (RP)
Synthetic population

Population census (RP)
Income data (FiLoSoFi)
Synthetic population

Population census (RP)
Income data (FiLoSoFi)
Commuting data (RP-MOB)
Synthetic population

Population census (RP)
Income data (FiLoSoFi)
Commuting data (RP-MOB)
Household travel survey (EDGT)
0:00 - 8:00
08:30 - 17:00
17:30 - 0:00
0:00 - 9:00
10:00 - 17:30
17:45 - 21:00
22:00 - 0:00
Synthetic population

Population census (RP)
Income data (FiLoSoFi)
Commuting data (RP-MOB)
Household travel survey (EDGT)
Enterprise census (SIRENE)
Address database (BD-TOPO)
Synthetic population

Population census (RP)
Income data (FiLoSoFi)
Commuting data (RP-MOB)
Household travel survey (EDGT)
Enterprise census (SIRENE)
Address database (BD-TOPO)

Person ID
Age
Gender
Home (X,Y)
1
43
male
(65345, ...)
2
24
female
(65345, ...)
3
9
female
(65345, ...)
Synthetic population

Population census (RP)
Income data (FiLoSoFi)
Commuting data (RP-MOB)
Household travel survey (EDGT)
Enterprise census (SIRENE)
Address database (BD-TOPO)
Person ID
Activity
Start
End
Loc.
523
home
08:00
(x,y)
523
work
08:55
18:12
(x,y)
523
shop
19:10
19:25
(x,y)
523
home
19:40
(x,y)

Synthetic population

Population census (RP)
Income data (FiLoSoFi)
Commuting data (RP-MOB)
Household travel survey (EDGT)
Enterprise census (SIRENE)
OpenStreetMap
GTFS (SYTRAL / SNCF)
Address database (BD-TOPO)

Parcel demand

Synthetic population
Gardrat, M., 2019. Méthodologie d’enquête: le découplage de l’achat et de la récupération des marchandises par les ménages. LAET, Lyon, France.
+
Synthetic population
Iterative proportional fitting (IFP)
- Based on synthetic population, find average number of purchases delivered to a household defined by socioprofesional class, age of the reference person and household size per day.

Out-of-home purchase survey
Achats découplés des ménages
Parcel demand

Synthetic population
Gardrat, M., 2019. Méthodologie d’enquête: le découplage de l’achat et de la récupération des marchandises par les ménages. LAET, Lyon, France.
+
Synthetic population
Maximum entropy approach
- We now the average number of parcels, but we do not now the distribution of the number of parcels for a household on an average day.
- We know it must be non-negative, and we know the mean.
- Without additional data, we assume maximum entropy distribution, which is Exponential in this case.
Out-of-home purchase survey
Achats découplés des ménages
Routing problem

Vehicle routing problem with time windows (VRPTW)
- We have a fleet of N vehicles (capacity, speed).
- They need to delivery the parcels when somebody is home (i.e. they need to respect time windows).
- Problem: Find optimal route that minimizes the distance covered while fulfilling the criteria.

Routing problem

Vehicle routing problem with time windows (VRPTW)
- We have a fleet of N vehicles (capacity, speed).
- They need to delivery the parcels when somebody is home (i.e. they need to respect time windows).
- Problem: Find optimal route that minimizes the distance covered while fulfilling the criteria.

Routing problem

Vehicle routing problem with time windows (VRPTW)
- We have a fleet of N vehicles (capacity, speed).
- They need to delivery the parcels when somebody is home (i.e. they need to respect time windows).
- Problem: Find optimal route that minimizes the distance covered while fulfilling the criteria.


First case study

- Synthetic population parcel
demand (101 parcels)
- Parcel size: 1
- Available vehicles: 10
- Vehicle capacity: 5
- Vehicle speed: 10 km/h
- Pickup time (at depot):
60s
- Delivery time (at customer):
5min
- Minimize driven distance
Agent-based models
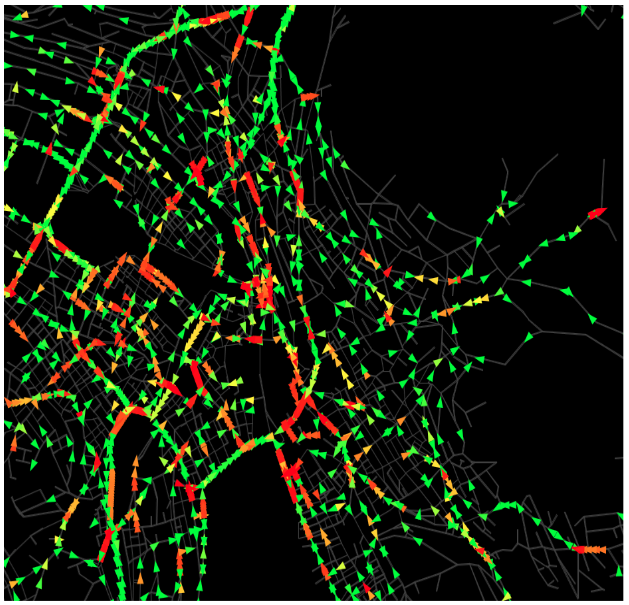
Senozon VIA

https://pixabay.com/en/traffic-jam-stop-and-go-rush-hour-143391/
MATSim
Senozon VIA
- Flexible, extensible and well-tested open-source transport simulation framework
- Used by many research groups and companies all over the world
- Extensions for parking behaviour, signal control, location choice, freight, ...

http://www.matsim.org
matsim-org/matsim-libs













MATSim
Mobility simulation
Decision-making
Analysis
Scenario
- Flexible, extensible and well-tested open-source transport simulation framework
- Used by many research groups and companies all over the world
- Extensions for parking behaviour, signal control, location choice, freight, ...
MATSim
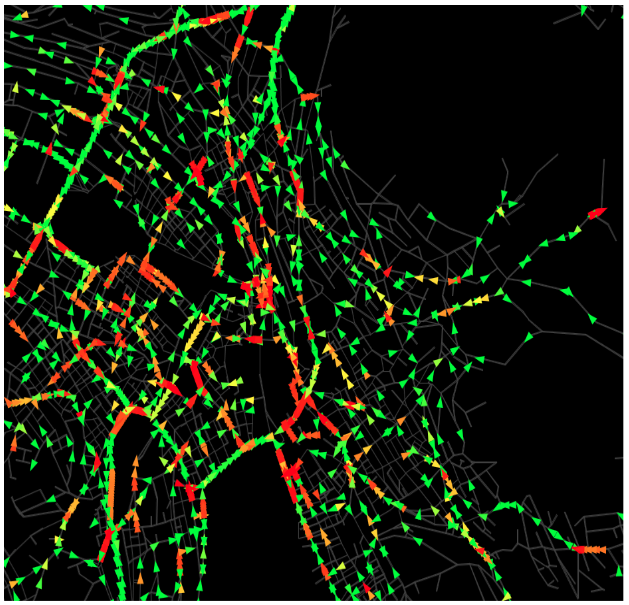
Senozon VIA

MATSim
Home
Work
Shop
Home
until 8am
9am to 6pm
6:15m to 6:30pm
from 6:45pm
walk
public
transport
walk
Calibration
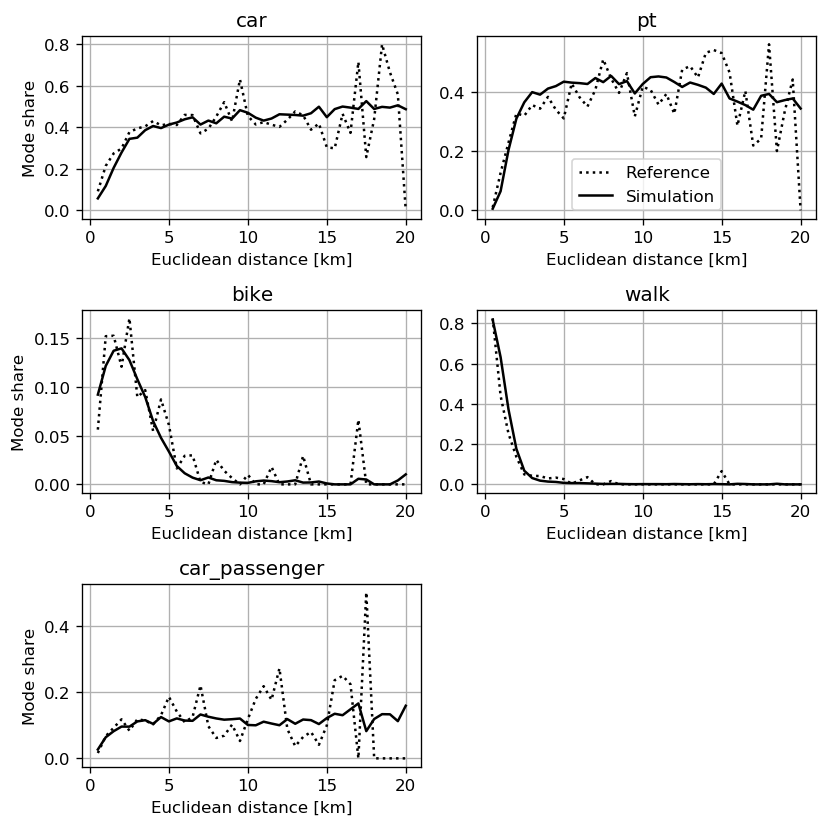


LEAD: Lyon use case
By Sebastian Hörl
LEAD: Lyon use case
11 May 2021
- 916