

Use cases for multiagent simulation in passenger and freight transport
Sebastian Hörl
21 March 2022
Paris School of Economics
IRT SystemX

- Research institute situated in Paris
- Focus on fostering digital transition in a range of fields from transport, cybersecurity to circular economy
- Transferring research results and tools into active application by development and provision of industry platforms
- Various collaborative projects with multiple French companies (Renault, SNCF, ...) and academic partners (Université Paris Saclay, CentraleSupélec, Université Gustave Eiffel)
- Participation in European projects
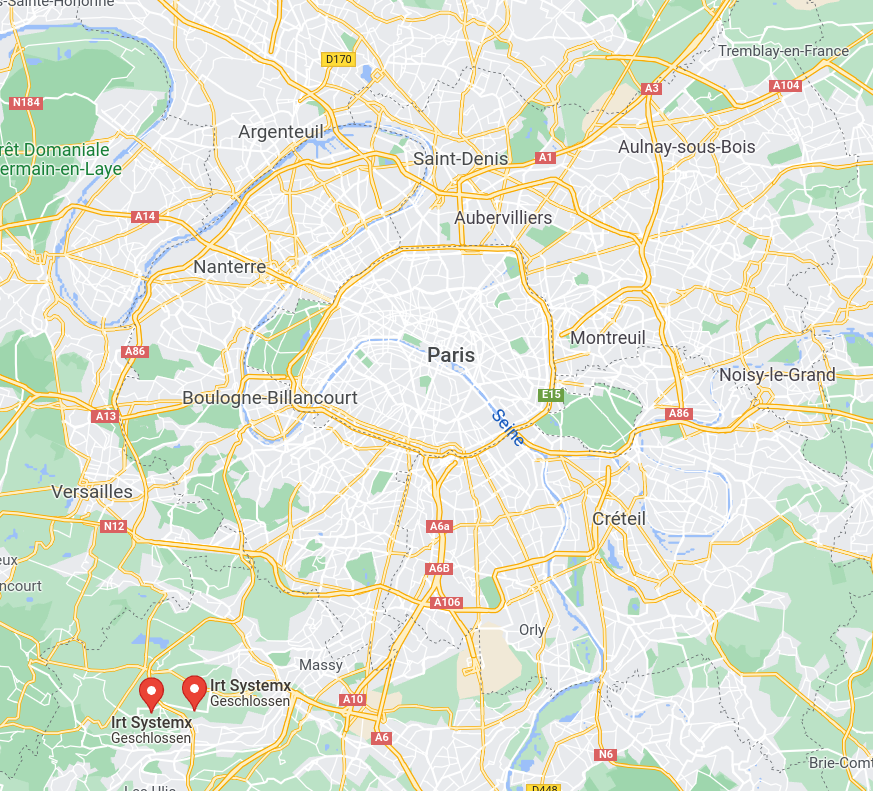
MATSim @ IRT SystemX

- On-demand mobility simulation
- First/last mile mobility simulation
Passenger transport
Freight transport


- First/last mile delivery
- VRP Solvers
- Development of electric VRP for JSprit

http://www.loc.gov/pictures/item/2016800172/
The street in 1900

The street today

https://commons.wikimedia.org/wiki/File:Atlanta_75.85.jpg
- Autonomous Mobility
- Mobility as a Service
- Mobility on Demand
- Electrification
- Aerial Mobility
Julius Bär / Farner
The street of tomorrow?

I. Transport simulation
Classic transport planning



- Zones
- Flows
- Peak hours
- User groups
Aggregated
Agent-based models

0:00 - 8:00
08:30 - 17:00
17:30 - 0:00
0:00 - 9:00
10:00 - 17:30
17:45 - 21:00
22:00 - 0:00
- Discrete locations
- Individual travelers
- Individual behaviour
- Whole day analysis
Disaggregated
Icons on this and following slides: https://fontawesome.com

MATSim



- Flexible, extensible and well-tested open-source transport simulation framework
- Used by many research groups and companies all over the world
- Extensions for parking behaviour, signal control, location choice, freight, ...

matsim-org/matsim-libs














MATSim



Synthetic demand

MATSim



Mobility simulation
Synthetic demand

MATSim
Decision-making



10:00 - 17:30
17:45 - 21:00
22:00 - 0:00
Mobility simulation
Synthetic demand

MATSim
Decision-making



Mobility simulation
Synthetic demand

MATSim
Decision-making



Mobility simulation
Analysis
Synthetic demand
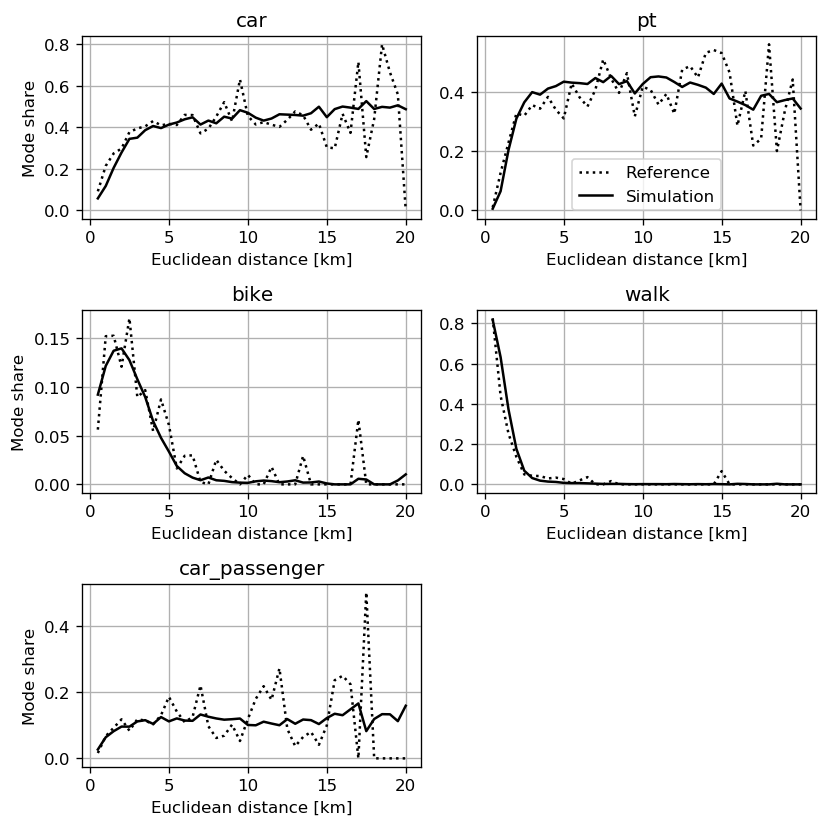
https://pixabay.com/en/zurich-historic-center-churches-933732/
II. AMoD in Zurich
Cost structures?
User preferences?
System impact?
Cost Calculator for automated mobility
Stated preference survey
MATSim simulation
1
2
3
What do we know about automated taxis?

What do we know about automated taxis?


Bösch, P.M., F. Becker, H. Becker and K.W. Axhausen (2018) Cost-based analysis of autonomous mobility services, Transport Policy, 64, 76-91
What do we know about automated taxis?



Felix Becker, Institute for Transport Planning and Systems, ETH Zurich.
VTTS




13 CHF/h
AMoD
Taxi
19 CHF/h
Conventional
Car
12 CHF/h
Public
Transport
AMoD
Car by Adrien Coquet from the Noun Project
Bus by Simon Farkas from the Noun Project
Wait by ibrandify from the Noun Project
VTTS




13 CHF/h
AMoD
Taxi
19 CHF/h
Conventional
Car
12 CHF/h
Public
Transport

21 CHF/h
32 CHF/h
AMoD
Car by Adrien Coquet from the Noun Project
Bus by Simon Farkas from the Noun Project
Wait by ibrandify from the Noun Project
Model structure

Cost calculator
Plan modification
Discrete Mode Choice Extension
Mobility simulation
Prediction
Price
Trips
- Utilization
- Empty distance, ...
- Travel times
- Wait times, ...
Fleet sizing with dynamic demand


Fleet sizing with dynamic demand



Fleet sizing with dynamic demand



Visualisation

Automated taxi
Pickup
Dropoff

Hörl, S., F. Becker and K.W. Axhausen (2020) Automated Mobility on Demand: A comprehensive simulation study of cost, behaviour and system impact for Zurich
Hörl, S., F. Becker and K.W. Axhausen (2020) Automated Mobility on Demand: A comprehensive simulation study of cost, behaviour and system impact for Zurich


13% reduction in vehicles
100% increase in VKT
Hörl, S., F. Becker and K.W. Axhausen (2020) Automated Mobility on Demand: A comprehensive simulation study of cost, behaviour and system impact for Zurich
100% increase in VKT
Hörl, S., F. Becker and K.W. Axhausen (2020) Automated Mobility on Demand: A comprehensive simulation study of cost, behaviour and system impact for Zurich

Other aspects



Hörl, S., C. Ruch, F. Becker, E. Frazzoli and K.W. Axhausen (2019) Fleet operational policies for automated mobility: a simulation assessment for Zurich, Transportation Research: Part C, 102, 20-32.
Fleet control


Operational constraints
Spatial constraints
Intermodality
Pooling
III. Demand data
https://pixabay.com/en/paris-eiffel-tower-night-city-view-3296269/
Synthetic travel demand

Population census (RP)

Population census (RP)
Income data (FiLoSoFi)
Synthetic travel demand

Population census (RP)
Income data (FiLoSoFi)
Commuting data (RP-MOB)
Synthetic travel demand

Population census (RP)
Income data (FiLoSoFi)
Commuting data (RP-MOB)
Household travel survey (EDGT)
0:00 - 8:00
08:30 - 17:00
17:30 - 0:00
0:00 - 9:00
10:00 - 17:30
17:45 - 21:00
22:00 - 0:00
Synthetic travel demand

Population census (RP)
Income data (FiLoSoFi)
Commuting data (RP-MOB)
Household travel survey (EDGT)
Enterprise census (SIRENE)
Address database (BD-TOPO)
Synthetic travel demand

Population census (RP)
Income data (FiLoSoFi)
Commuting data (RP-MOB)
Household travel survey (EDGT)
Enterprise census (SIRENE)
Address database (BD-TOPO)
Person ID
Age
Gender
Home (X,Y)
1
43
male
(65345, ...)
2
24
female
(65345, ...)
3
9
female
(65345, ...)

Synthetic travel demand

Population census (RP)
Income data (FiLoSoFi)
Commuting data (RP-MOB)
Household travel survey (EDGT)
Enterprise census (SIRENE)
Address database (BD-TOPO)
Person ID
Activity
Start
End
Loc.
523
home
08:00
(x,y)
523
work
08:55
18:12
(x,y)
523
shop
19:10
19:25
(x,y)
523
home
19:40
(x,y)

Synthetic travel demand

Population census (RP)
Income data (FiLoSoFi)
Commuting data (RP-MOB)
Household travel survey (EDGT)
Enterprise census (SIRENE)
OpenStreetMap
GTFS (SYTRAL / SNCF)
Address database (BD-TOPO)

Synthetic travel demand

Population census (RP)
Income data (FiLoSoFi)
Commuting data (RP-MOB)
Household travel survey (EDGT)
Enterprise census (SIRENE)
OpenStreetMap
GTFS (SYTRAL / SNCF)
Address database (BD-TOPO)
Synthetic travel demand

Population census (RP)
Income data (FiLoSoFi)
Commuting data (RP-MOB)
National HTS (ENTD)
Enterprise census (SIRENE)
OpenStreetMap
GTFS (SYTRAL / SNCF)
Address database (BD-TOPO)
Synthetic travel demand
EDGT

Population census (RP)
Income data (FiLoSoFi)
Commuting data (RP-MOB)
Enterprise census (SIRENE)
OpenStreetMap
GTFS (SYTRAL / SNCF)
Address database (BD-TOPO)
Synthetic travel demand
Open
Data
Open
Software
+
=
Reproducible research
Integrated testing
National HTS (ENTD)
EDGT

Population census (RP)
Income data (FiLoSoFi)
Commuting data (RP-MOB)
Enterprise census (SIRENE)
OpenStreetMap
GTFS (SYTRAL / SNCF)
Address database (BD-TOPO)
Synthetic travel demand
Open
Data
Open
Software
+
=
Reproducible research
Integrated testing
National HTS (ENTD)
EDGT


Current use cases


Nantes
- Noise modeling


Current use cases

Lille
- Park & ride applications
- Road pricing



Current use cases


Toulouse
- Placement and use of shared offices

Current use cases

Rennes
- Micromobility simulation



Current use cases



Paris / Île-de-France
- Scenario development for sustainable urban transformation
- New mobility services
Mahdi Zargayouna (GRETTIA / Univ. Gustave Eiffel)
Nicolas Coulombel (LVMT / ENPC)

Current use cases



Paris / Île-de-France
- Cycling simulation

Current use cases


Paris / Île-de-France
- Simulation of dynamic mobility services
- Fleet control through reinforcement learning

Current use cases



Lyon (IRT SystemX)
- Low-emission first/last mile logistics

Current use cases




Current use cases



Balac, M., Hörl, S. (2021) Synthetic population for the state of California based on open-data: examples of San Francisco Bay area and San Diego County, presented at 100th Annual Meeting of the Transportation Research Board, Washington, D.C.
Sallard, A., Balac, M., Hörl, S. (2021) Synthetic travel demand for the Greater São Paulo Metropolitan Region, based on open data, Under Review
Sao Paulo, San Francisco Bay area, Los Angeles five-county area, Switzerland, Montreal, Quebec City, Jakarta, Casablanca, ...

Emissions in Paris




Grand Paris Express





Automated taxis in Paris





Automated taxis in Paris





IV. Logistics
LEAD Project

Low-emission Adaptive last-mile logistics supporting on-demand economy through Digital Twins
- H2020 Project from 2020 to 2023
- Six living labs with different innovative logistics concepts
- Lyon, The Hague, Madrid, Budapest, Porto, Oslo
- One partner for implementation and one for research each
- Development of a generic modeling library for last-mile logistics scenario simulation and analysis

Lyon Living Lab

- Peninsula Confluence between Saône and Rhône
- Interesting use case as there are limited access points
- Implementation of an urban consolidation center (UCC) to collect the flow of goods and organize last-mile distribution
- using cargo-bikes
- using electric robots
- and others
- Analysis and modeling through
- Flow estimation through cameras
- Simulation of future scenarios
- Focus on parcel deliveries due to data availability


Modeling methodology






Agent-based simulation of Lyon
Demand in parcel deliveries
Distribution by operators
KPI Calculation
- Main question: What is the impact on traffic and population of implementing an Urban Consolidation Center in Confluence?
- Focus on B2C parcel deliveries due to data availability

Methodology: Parcel demand

Synthetic population
+
Synthetic population
Out-of-home purchase survey
Achats découplés des ménages

Based on sociodemographic attributes of the households, parcels are generated for the city on an average day.

Gardrat, M., 2019. Méthodologie d’enquête: le découplage de l’achat et de la récupération des marchandises par les ménages. LAET, Lyon, France.
Methodology: Parcel demand

Synthetic population
+
Synthetic population
Out-of-home purchase survey
Achats découplés des ménages

Based on sociodemographic attributes of the households, parcels are generated for the city on an average day.


Gardrat, M., 2019. Méthodologie d’enquête: le découplage de l’achat et de la récupération des marchandises par les ménages. LAET, Lyon, France.
Methodology: Parcel demand

Synthetic population
+
Synthetic population
Out-of-home purchase survey
Achats découplés des ménages

Based on sociodemographic attributes of the households, parcels are generated for the city on an average day.



Gardrat, M., 2019. Méthodologie d’enquête: le découplage de l’achat et de la récupération des marchandises par les ménages. LAET, Lyon, France.
Methodology: Parcel demand

Synthetic population
+
Synthetic population
Out-of-home purchase survey
Achats découplés des ménages

Based on sociodemographic attributes of the households, parcels are generated for the city on an average day.



Gardrat, M., 2019. Méthodologie d’enquête: le découplage de l’achat et de la récupération des marchandises par les ménages. LAET, Lyon, France.

Methodology: Parcel demand

Synthetic population
Gardrat, M., 2019. Méthodologie d’enquête: le découplage de l’achat et de la récupération des marchandises par les ménages. LAET, Lyon, France.
+
Synthetic population
Out-of-home purchase survey
Achats découplés des ménages

Based on sociodemographic attributes of the households, parcels are generated for the city on an average day.
Iterative proportional fitting (IFP)
- Based on synthetic population, find average number of purchases delivered to a household defined by socioprofesional class, age of the reference person and household size per day.

Methodology: Parcel demand

Synthetic population
Gardrat, M., 2019. Méthodologie d’enquête: le découplage de l’achat et de la récupération des marchandises par les ménages. LAET, Lyon, France.
+
Synthetic population
Out-of-home purchase survey
Achats découplés des ménages

Based on sociodemographic attributes of the households, parcels are generated for the city on an average day.
Maximum entropy approach
- We now the average number of parcels, but we do not now the distribution of the number of parcels for a household on an average day.
- We know it must be non-negative, and we know the mean.
- Without additional data, we assume maximum entropy distribution, which is Exponential in this case.
Methodology: Parcel demand

Synthetic population
Gardrat, M., 2019. Méthodologie d’enquête: le découplage de l’achat et de la récupération des marchandises par les ménages. LAET, Lyon, France.
+
Synthetic population
Out-of-home purchase survey
Achats découplés des ménages

Based on sociodemographic attributes of the households, parcels are generated for the city on an average day.

Methodology: Parcel demand

Synthetic population
Gardrat, M., 2019. Méthodologie d’enquête: le découplage de l’achat et de la récupération des marchandises par les ménages. LAET, Lyon, France.
+
Synthetic population
Out-of-home purchase survey
Achats découplés des ménages

Based on sociodemographic attributes of the households, parcels are generated for the city on an average day.

Methodology: Parcel demand

Synthetic population
Gardrat, M., 2019. Méthodologie d’enquête: le découplage de l’achat et de la récupération des marchandises par les ménages. LAET, Lyon, France.
+
Synthetic population
Out-of-home purchase survey
Achats découplés des ménages

Based on sociodemographic attributes of the households, parcels are generated for the city on an average day.

Methodology: Parcel demand

Synthetic population
Gardrat, M., 2019. Méthodologie d’enquête: le découplage de l’achat et de la récupération des marchandises par les ménages. LAET, Lyon, France.
+
Synthetic population
Out-of-home purchase survey
Achats découplés des ménages

Based on sociodemographic attributes of the households, parcels are generated for the city on an average day.

Presence of household members
Methodology: Route optimization


Using a heursitic routing solver, an optimal distribution scheme per distribution center is obtained to arrive at a lower bound estimate for the distance covered for the deliveries.


JSprit
First case study



Hörl, S. and J. Puchinger (2022) From synthetic population to parcel demand: Modeling pipeline and case study for last-mile deliveries in Lyon, TRA 2022, Lisbon.
Solve VRP-TW based on generated parcels and household presence
JSprit
First case study



Solve VRP-TW based on generated parcels and household presence
JSprit

Hörl, S. and J. Puchinger (2022) From synthetic population to parcel demand: Modeling pipeline and case study for last-mile deliveries in Lyon, TRA 2022, Lisbon.
Methodology: Study area


How many parcels need to be delivered on one day?
Methodology: Study area


How many parcels need to be delivered on one day?
Perimeter
- City of Lyon
- Grand Lyon metropolitan region (dashed)
- Bordering municipalities including relevant logistics infrastructure
Demand
- 1.6M personas
- 790k households
- 16,252 parcels

Methodology: Distribution centers


From where do operators delivery the parcels?
Methodology: Distribution centers


From where do operators delivery the parcels?
Approach
- Facilities per operator extracted from SIRENE
- Geolocated using public BAN API
- La Poste: Facilities with 20+ employees


Methodology: Market shares


How many parcels are delivered by each operator?
Methodology: Market shares


How many parcels are delivered by each operator?
Approach
- For some operators, we know the annual national volumes from gray literature
- We know that La Poste (Colissimo + DPD + Chronopost) add up to about 65% of all parcels in France
- For the rest, we approximate their market share using their annual turnover values

Methodology: Market shares


How many parcels are delivered by each operator?


Methodology: Assignment


How many parcels are delivered by each distribution center?
Methodology: Assignment


How many parcels are delivered by each distribution center?
Approach
- For each parcel, sample an operator
- Find the operator's distribution center that is closest (shortest distance) to the parcel



Methodology: Assignment


Approach
- For each parcel, sample an operator
- Find the operator's distribution center that is closest (shortest distance) to the parcel
Outcome
- Nine centers with 300+ parcels
- Remaining centers with less than 300

How many parcels are delivered by each distribution center?
Methodology: Cost structures


What influences operators decisions?
Methodology: Cost structures


What influences operators decisions?
Salaries
Vehicles
Distance
Daily cost
+
+
=
Methodology: Cost structures


What influences operators decisions?
Assumption (from grey literature)
- 1,300 EUR net per month
- 1,700 EUR gross per month
- 3,400 EUR staff cost per month
- 25 active days per month
- 136 EUR per day
Salaries
Vehicles
Distance
Daily cost
+
+
=
Methodology: Cost structures


What influences operators decisions?
Salaries
Distance
Daily cost
+
+
=

- We examined long-duration rental offers (LLD) of French vehicle manufacturers
Vehicles
Methodology: Cost structures


What influences operators decisions?
Daily cost
=
- Insight: Rental costs depend linearly on the transport volume

Salaries
Distance
+
+
Vehicles
Methodology: Cost structures


What influences operators decisions?
Daily cost
=
- Seven prototypical vehicle types: 3 sizes thermal or electric plus cargo-bike


Salaries
Distance
+
+
Vehicles
Methodology: Cost structures


What influences operators decisions?
Daily cost
=
- Distance-costs depend on consumption of fuel and electricity
- Multiplied by fuel or electricity prices (example 1.45 EUR/L and 9ct/kWh)



Salaries
Distance
+
+
Vehicles
Methodology: Cost structures


What influences operators decisions?
Daily cost
=
- Additional information from our manufacturer analysis: Emissions
- Assuming 90 gCO2eq/kWh for electric vehicles


Salaries
Distance
+
+
Vehicles
Methodology: Optimization


Minimize costs
Methodology: Optimization


Heterogeneous Vehicle Routing Problem
- Minimize cost per distribution center
- Operator can choose vehicles (7 types) and routes
- Operator must deliver all assigned parcels
- Maximum active time per day 10h
- Active time is travel time + 120s delivery + 60s pick-up per parcel
- Vehicles can not exceed capacity (we assume 10 parcels per m3)
- Multiple tours per vehicle are allowed
- Vehicles start and end the day at the distribution center
Minimize costs
Methodology: Optimization


Heterogeneous Vehicle Routing Problem
- Minimize cost per distribution center
- Operator can choose vehicles (7 types) and routes
- Operator must deliver all assigned parcels
- Maximum active time per day 10h
- Active time is travel time + 120s delivery + 60s pick-up per parcel
- Vehicles can not exceed capacity (we assume 10 parcels per m3)
- Multiple tours per vehicle are allowed
- Vehicles start and end the day at the distribution center
Minimize costs
Data
- OpenStreetMap network
- Extracted using osmnx
- Distance matrix between parcels and depot
- Travel time matrix using congestion factors
Solver
- Open-source
- VROOM
Visualisation platform



UCC platform



Questions?



Use cases for multi-agent simulation in passenger and freight transport
By Sebastian Hörl
Use cases for multi-agent simulation in passenger and freight transport
Paris School of Economics, 21 March 2023
- 220