Deep NLP for Adverse drug event extraction
Adverse Drug Events
Problem
Motivation
- Adverse reaction caused by drugs is a potentially dangerous
- leads to mortality and morbidity in patients.
- Adverse Drug Event (ADE) extraction is a significant and unsolved problem in biomedical research.
DATA Source
PUBMED Abstracts
I have been on Methotrexate since a year ago. It seemed to be helping and under care of my doctor. I have developed an inflammed stomach lining and two ulcers due to this drug. Other meds I am on do not leave me with any side affects. I have had an ultrasound..am waiting for treatment from the Endoscopy doctor that did the tests. It will be a type of medicine to heal my stomach. I have been very sick and vomiting, dry heaves, and am limited to what I can eat. Please make sure if you have any of these side affects, you inform your doctor immediately. I am off the Methotrexate for good. Not a good experience for me. Thank You. |
Problem Definition
Given a sequence of words <w1, w2, w3, ..., wn> :
- entity extraction: label the sequence whether the word is a drug, disease or neither
- relationship extraction: extract the relationship between the drugs and diseases pairs
Example - Relationship extraction
<methotrexate, sever side effects> - YES
I have suffered sever side effects from the oral methotrexate and have not been able to remain on this medication.
EXAMPLE - Entity extraction
I |
have | suffered | sever | side |
---|---|---|---|---|
O | O | O |
B-Disease |
I-Disease |
effects |
from | the | oral |
methotrexate |
L-Disease |
O | O | O |
U-Drug |
and | have | not | been | able |
O | O | O |
O | O |
to | remain | on | this | medication |
O |
O |
O |
O |
O |
BILOU - Begin, Inside, Last, Outside, Unit
Existing Architectures
Joint Models for Extracting Adverse Drug Events from Biomedical Text
https://www.ijcai.org/Proceedings/16/Papers/403.pdf

- Uses Convolution
- Models entity extraction and relationship extraction as a state transition problem
Fei Li, Yue Zhang, Meishan Zhang, Donghong Ji, 2016
End-to-End Relation Extraction using LSTMs on Sequences and Tree Structures
- Using SDP provides more context
- Uses TreeLSTM

http://arxiv.org/abs/1601.00770
Miwa, M. and Bansal, M., 2016.
A neural joint model for entity and relation extraction from biomedical text
https://bmcbioinformatics.biomedcentral.com/articles/10.1186/s12859-017-1609-9

Fei Li, Yue Zhang, Meishan Zhang, Donghong Ji, 2017
- Everything from above, and
- Character embedding
Our Model

Performance comparison
Entity Extraction | ADE Extraction | |
---|---|---|
Li, et al, 2016 | 79.5 | 63.4 |
Miwa & Bansal | 83.4 | 55.6 |
Li, et al, 2017 | 84.6 | 71.4 |
Our model | 85.30 | 86.78 |
EEAP Framework for nLP
Embed, Encode, Attend, Predict
Embed, encode, attend, Predict
Embed
word-level representation
Frequency based
-
TF-IDF
- TF - Term Frequency
- IDF - Inverse Document Frequency
- Penalty for common words
- Co-occurrence Matrix
- V x N
Word embedding
- Distributed Representation
- Captures semantic meaning
- meaning is relative
- Fundamentally based on co-occurrence
- Prediction based vectorization
- predict neighboring words
Word embedding

Word2vec : CBOW
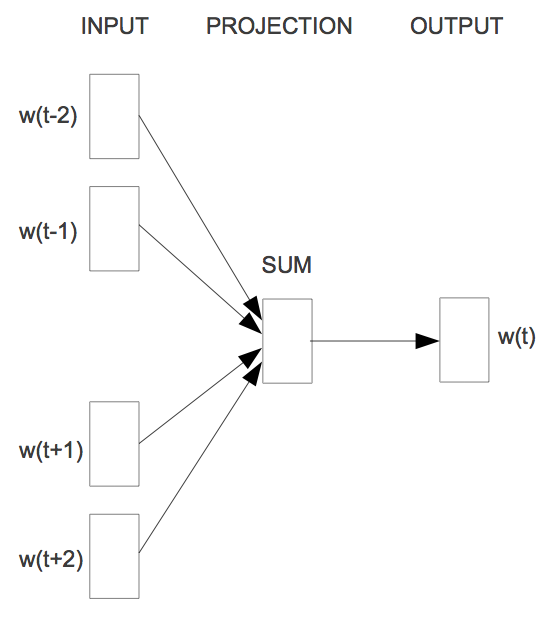
Word2vec : Skipgram
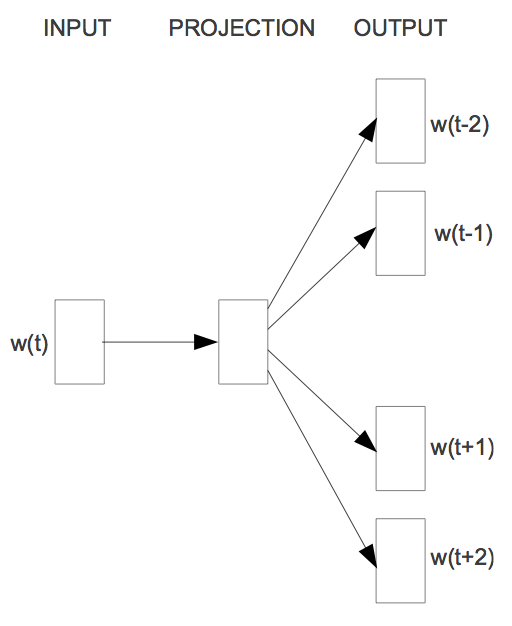
Pre-trained word embeddings
- Word2Vec, Glove
- wikipedia
- common crawl
-
Word vectors induced from
- PubMed, PMC
- Uses word2vec
flaws
- Out-of-vocabulary (OOV) Tokens
- Large Vocabulary size
- Rare words are left out
- Possible Solution
- Average of neighbours
character-level word representation
- Vocabulary of unique characters
- fixed and small
- Morphological Features
- Word as a sequence (RNN)
- Word as a 2D image [count x dim] (CNN)
- Jointly trained along model objective
Hybrid embedding
- Combines
- Morphological features
- Semantic Features
- Combination Method
- Concatenation
- \( embedding(w_i) = [ W_{w_i} ; C_{w_i} ] \)
- Gated Mixing
- \( cg_{w_i} = f(W_{w_i}, C_{w_i})\)
- \( wg_{w_i} = g(W_{w_i}, C_{w_i})\)
- \( embedding(w_i) = cg_{w_i}.C_{w_i} + wg_{w_i}.W_{w_i} \)
- Concatenation
ENcode
sequence-level representation
feed forward neural network

Recurrence

Unfolding

Unfolding
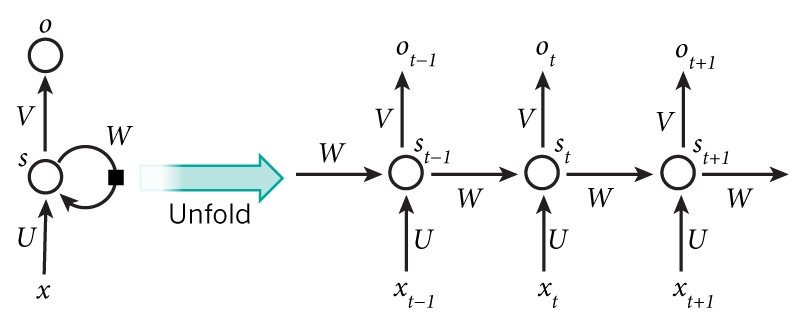
Forward Propagation

Bidirectional RNN

Vanilla RNN
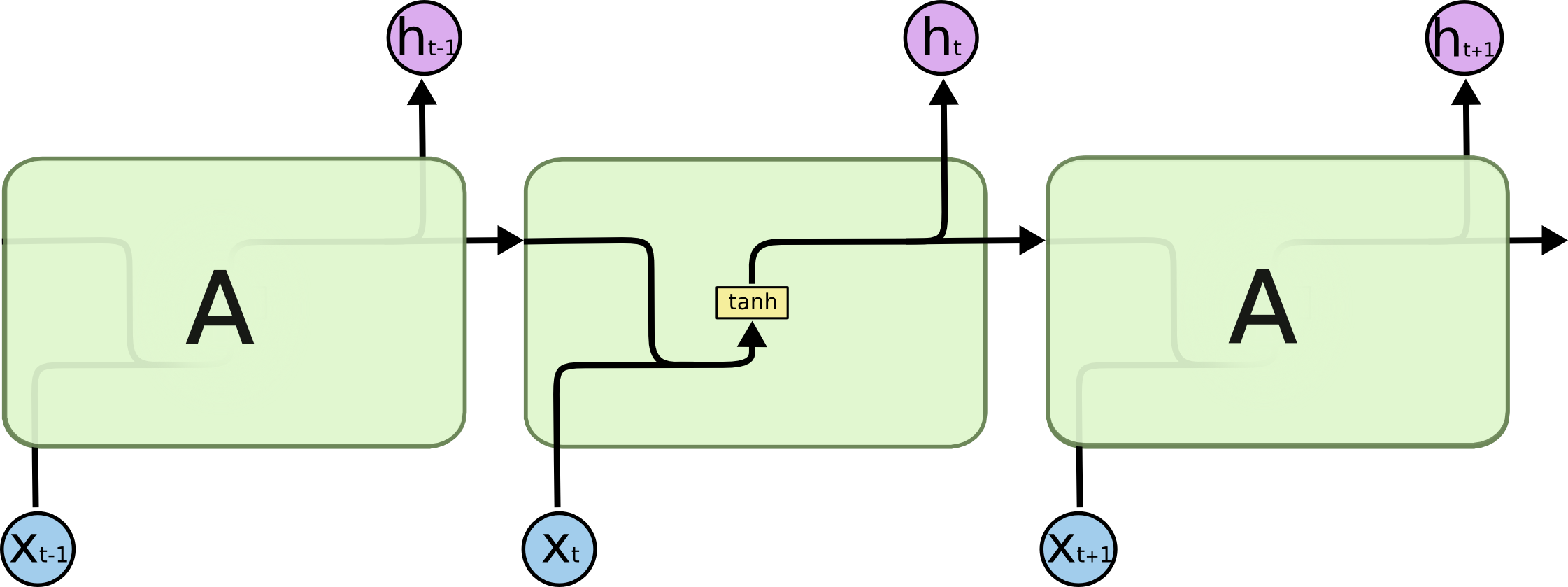
Gating mechanism

LSTM - Long Short term memory
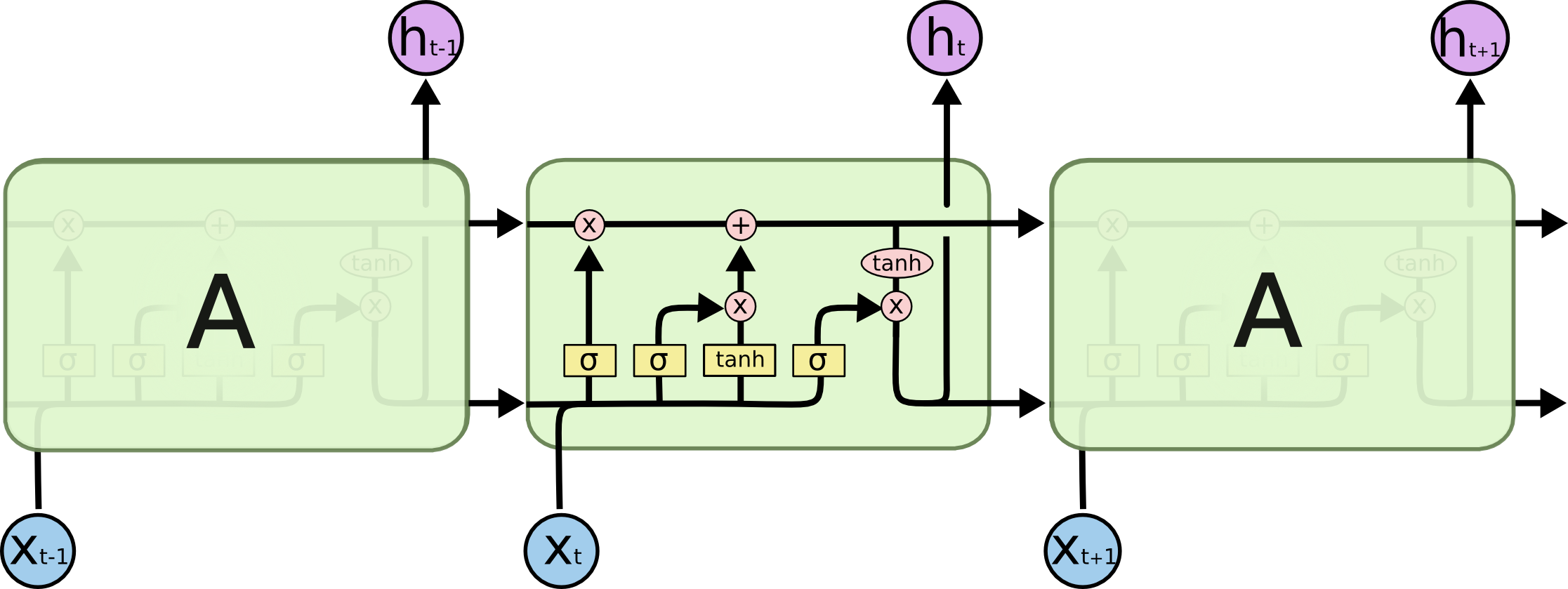
Attend
reduction by attention pooling
Attention Mechanism
- Reference, an array of units
- Query
- Attention weights
- signify which parts of reference are relevant to query
- Which parts of the context are relevant to the query?
- Weighted or Blended Representation
Attention Mechanism
-
Multiplicative attention
- \(a_{ij} = h_i^TW_as_j\)
- \(a_{ij} = h_i^Ts_j\)
-
Additive attention
- \(a_{ij} = v_a^T tanh(W_1h_i + W_2s_j)\)
- \(a_{ij} = v_a^T tanh(W_a [h_i ; s_j])\)
-
Blended Representation
- \(c_i = \sum_j a_{ij} s_j\)
Attention Mechanism

predict
sequence labelling, classification
Classification
- Final/Target Representation
- Affine Transformation
- Optional Non-linearity
- Log-Likelihood
- Softmax
- Probability distribution across classes
our architecture
attentive sequence model for ADE extraction
Redefining the problem
- Model ADE Extraction as a Question Answering Problem
- Inspired by Reading Comprehension Literature
- Given a sequence and a drug
- Is the t_th word in the sequence an Adverse Drug Event


Architecture

embedding
-
Word Embedding
-
Fixed
-
Variable
-
-
Character-level Word Representation
-
CharCNN
-
Multiple filters of different widths
-
Max-pooling across word length dimension
-
-
PoS and Label Embedding
-
PoS embedding helps when learning from small dataset
-
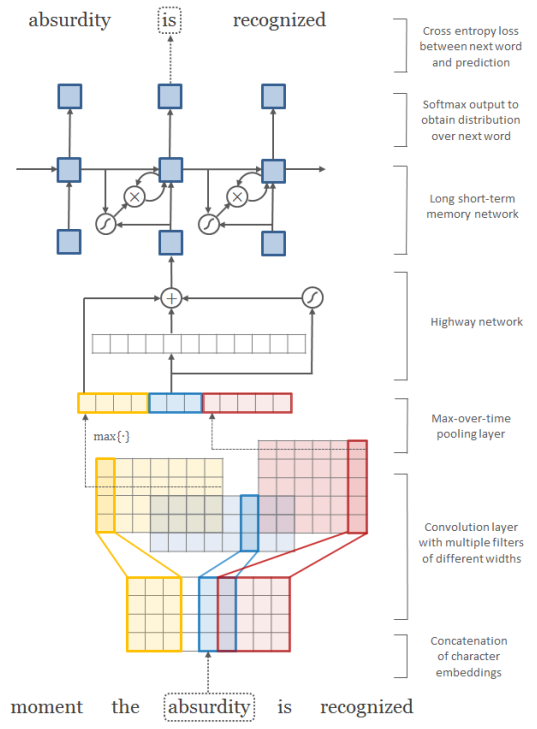
interaction layer

entity recognition

ade extraction

state of the art

Feature augmentation

f1 histogram

ER f1 vs ade f1

heatmap


Deep NLP for ADE Extraction in BIomedical text
By Suriyadeepan R
Deep NLP for ADE Extraction in BIomedical text
- 1,651