Do you know...
how much electricity one ChatGPT request consumes ?
Do you know...
how much electricity one ChatGPT request consumes ?
~ 0.001-0.01 kWh / query[1]
10W lamp turned on for one hour !
[1] https://limited.systems/articles/google-search-vs-chatgpt-emissions/

Do you also know...
how much electricity you consume when using GitHub Copilot for one hour?
[shrug.jpg]
Green My LLM: Studying the key factors affecting the energy consumption of code assistants
Coignion Tristan, Quinton Clément, Rouvoy Romain

GT GL-IA 2025 - Rennes

Find the paper online :

AI is everywhere

AI is everywhere
Code Assistant
GitHub Copilot
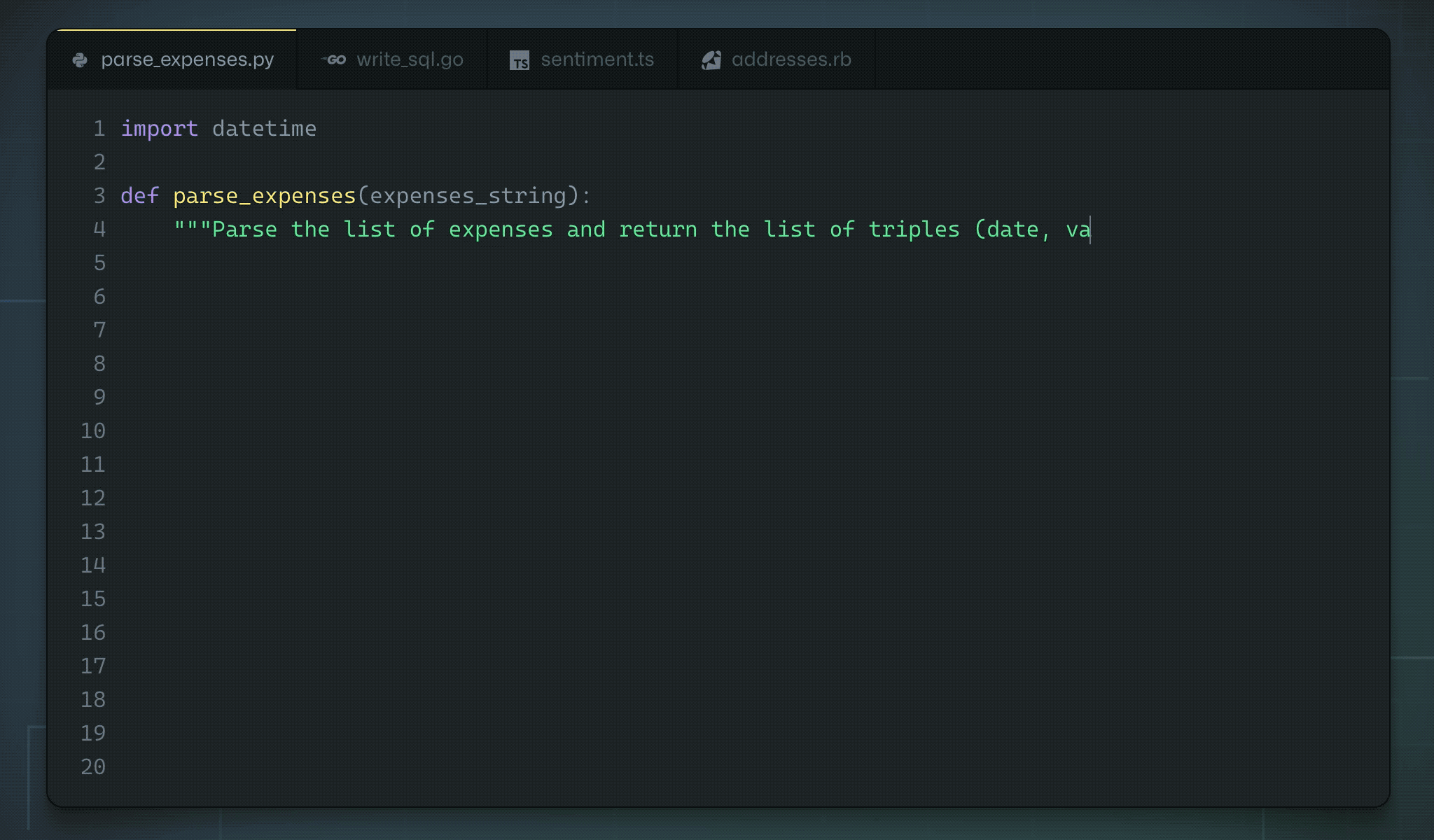
Demo of GitHub Copilot

How much energy am I consuming when using a code assistant like GitHub Copilot?

Sends and receives
generation requests

Inference Server in a datacenter somewhere
Makes code
suggestions

Code assistant
How code assistant usually work

Sends and receives
generation requests

Inference Server in a datacenter somewhere
Makes code
suggestions

Code assistant

This is where we want to measure !




Our method
Phase 1 : Build a dataset of development traces
Phase 2 : Use this dataset to simulate the usage of GitHub Copilot and measure the consumption of the inferences

generation requests

Normal GitHub Copilot's inference server
Makes code
suggestions

Code assistant


GitHub's
telemetry server
telemetry data
20 participants
developing for one hour
Phase 1 : Collecting development traces

generation requests

Normal GitHub Copilot's inference server
Makes code
suggestions

Code assistant


GitHub's
telemetry server
telemetry data
20 participants
developing for one hour
Phase 1 : Collecting development traces

generation requests

Normal GitHub Copilot's inference server
Makes code
suggestions

Code assistant

telemetry data
20 participants
developing for one hour

GitHub's
telemetry server
Our telemetry server
Phase 1 : Collecting development traces

generation requests

Normal GitHub Copilot's inference server
Makes code
suggestions

Code assistant

telemetry data
20 participants
developing for one hour

GitHub's
telemetry server
Our telemetry server
Phase 1 : Collecting development traces
Phase 2
Simulating the
code assistant

Our inference server (hosted on G5K)


Our telemetry server

Code assistant simulator
Simulates GitHub Copilot generation requests

`perf` + `nvidia-smi`
telemetry
data

records energy consumption

generation
requests
Configuration options for the simulations
- Number of concurrent developers - [1-500]
- Request Streaming - [Yes, No]
- Manual triggering of the code assistant* - [Yes, No]
- Large Language Model - [StarCoder, StarCoder2-{7,15}B]
- Quantization method - [EETQ, BitsAndBytes, None]
- Number of GPUS - [1-4]
* emulation of a manual triggering by the user.
4,896 possible configurations.
829 simulations with 314 unique configurations
Before the results...
Some limitations
- The measures are only an estimation of the energy GitHub Copilot would consume if it was using the same hardware as us.
- Does not take into account the PUE of the datacenter.
- Only considers the environmental impact from the energy standpoint.
- Needs more diverse configurations (hardware, models, etc.).
- Does not take into account code quality or validity.
Less generation requests == Less energy consumed
The least you use GitHub Copilot, the lower you energy consumption is.
A few stats about our participants' usage of GitHub Copilot
Depending on users, between 2 and 20 requests per minute.
Average of 9 requests per minute
Students accepted more often the suggestions from GitHub Copilot than the professional developers



Final state of suggestions made by GitHub Copilot.

Final state of suggestions made by GitHub Copilot.
- Suggestions happening when the user is typing are often cancelled before the completion of the generation.
-
Empty results happen when a suggestion generation is triggered at the end of sentences or lines.
- Suggestions that were not accepted typically happen because users did not ask for them specifically.*
* and sometimes because they were simply bad
Automatic suggestions can lead to a great waste of computing power.


Saturation point
Energy usage of the whole server and by developer, and latency of the requests depending on the number of concurrent developers

Impact on the energy consumption of switching from one configuration to another
(e.g. adding a GPU, or using another LLM)

Impact on the energy consumption of switching from one configuration to another
(e.g. adding a GPU, or using another LLM)

Impact on the energy consumption of switching from one configuration to another
(e.g. adding a GPU, or using another LLM)

Impact on the energy consumption of switching from one configuration to another
(e.g. adding a GPU, or using another LLM)

Impact on the energy consumption of switching from one configuration to another
(e.g. adding a GPU, or using another LLM)

Impact on the latency of the requests of switching from one configuration to another
Scenarios
-
Small team : A dedicated server for 5 concurrent developers
-
Medium team : A dedicated server for 20 concurrent developers
- Distributed service : A server whose load is maximized (e.g. commercial code assistants)



Scenarios
- Small team : A dedicated server for 5 concurrent developers
- Medium team : A dedicated server for 20 concurrent developers
- Distributed service : A server whose load is maximized (e.g. commercial code assistants)



Objectives
- Frugal objective : Minimize energy consumption
- Performance objective : Minimize latency and user discomfort (automatic suggestions)










Average consumption of one developer :





Average consumption of one developer :



Users of GitHub Copilot = 28.7 millions developers[1] x 41%[2]
[1] https://www.statista.com/statistics/627312/worldwide-developer-population/
[2] 2024 Stack Overflow Developer Survey
= 11.77 million users



x 11,770,000
=
235 MW


Users of GitHub Copilot = 28.7 millions developers[1] x 41%[2]
[1] https://www.statista.com/statistics/627312/worldwide-developer-population/
[2] 2024 Stack Overflow Developer Survey
= 11.77 million users



x 11,770,000
235 MW


Users of GitHub Copilot = 28.7 millions developers[1] x 41%[2]
[1] https://www.statista.com/statistics/627312/worldwide-developer-population/
[2] 2024 Stack Overflow Developer Survey
= 11.77 million users

25% x
235 MW


[1] https://www.edf.fr/groupe-edf/comprendre/production/nucleaire/nucleaire-en-chiffres

25% x
235 MW


[1] https://www.edf.fr/groupe-edf/comprendre/production/nucleaire/nucleaire-en-chiffres
235 MW


Also...

So... What can we do about it ?
Code assistant providers
- Use smaller and more specialized models
- Quantize the models
- Maximize the usage of each server.
- Allow user to easily manually trigger code assistants
So... What can we do about it ?
Code assistant providers
- Use smaller and more specialized models
- Quantize the models
- Maximize the usage of each server.
- Allow user to easily manually trigger code assistants
Users
- Use assistants mindfully
- Don't make them the default
- Disable the automatic suggestions
- Think before prompting. Only use AI when you need it.
So... What can we do about it ?
Code assistant providers
- Use smaller and more specialized models
- Quantize the models
- Maximize the usage of each server.
- Allow user to easily manually trigger code assistants
Users
- Use assistants mindfully
- Don't make them the default
- Disable the automatic suggestions
- Think before prompting. Only use AI when you need it.
Researchers
- Measure the environmental impact of AI.
- Make the measuring process easier.
- Talk about it around you
Thank you for listening !

Green My LLM - GT GL-IA 2025
By Tristan Coignion
Green My LLM - GT GL-IA 2025
- 31