

Robotics at Google
Language as Robot Middleware
Andy Zeng
Habitat Rearrangement Challenge

Manipulation

TossingBot
Transporter Nets
Implicit Behavior Cloning
Manipulation

TossingBot
"Definition 5. Manipulation refers to an agent’s control of its environment through selective contact."
Toward Robotic Manipulation
Matthew T. Mason, Annual Reviews 2018
Transporter Nets
Implicit Behavior Cloning
Rearrangement
"Definition 5. Manipulation refers to an agent’s control of its environment through selective contact."
Toward Robotic Manipulation
Matthew T. Mason, Annual Reviews 2018
Rearrangement is central to this!
Rearrangement: A Challenge for Embodied AI
Dhruv Batra, Angel X. Chang, Sonia Chernova, Andrew J. Davison, Jia Deng, Vladlen Koltun, Sergey Levine, Jitendra Malik, Igor Mordatch, Roozbeh Mottaghi, Manolis Savva, Hao Su
Habitat Rearrangement Challenge 2022
Andrew Szot, Karmesh Yadav, Alex Clegg, Vincent-Pierre Berges, Aaron Gokaslan, Angel Chang, Manolis Savva, Zsolt Kira, Dhruv Batra
Rearrangement
"Definition 5. Manipulation refers to an agent’s control of its environment through selective contact."
Toward Robotic Manipulation
Matthew T. Mason, Annual Reviews 2018
Rearrangement is central to this!

BEHAVIOR-1K: A Benchmark for Embodied AI with 1,000 Everyday Activities and Realistic Simulation
Chengshu Li, Ruohan Zhang, Josiah Wong, Cem Gokmen, Sanjana Srivastava, Roberto Martín-Martín, Chen Wang, Gabrael Levine, Michael Lingelbach, Jiankai Sun, Mona Anvari, Minjune Hwang, Manasi Sharma, Arman Aydin, Dhruva Bansal, Samuel Hunter, Kyu-Young Kim, Alan Lou, Caleb R Matthews, Ivan Villa-Renteria, Jerry Huayang Tang, Claire Tang, Fei Xia, Silvio Savarese, Hyowon Gweon, Karen Liu, Jiajun Wu, Li Fei-Fei
Rearrangement
"Definition 5. Manipulation refers to an agent’s control of its environment through selective contact."
Toward Robotic Manipulation
Matthew T. Mason, Annual Reviews 2018
Rearrangement is central to this!

BEHAVIOR-1K: A Benchmark for Embodied AI with 1,000 Everyday Activities and Realistic Simulation
Chengshu Li, Ruohan Zhang, Josiah Wong, Cem Gokmen, Sanjana Srivastava, Roberto Martín-Martín, Chen Wang, Gabrael Levine, Michael Lingelbach, Jiankai Sun, Mona Anvari, Minjune Hwang, Manasi Sharma, Arman Aydin, Dhruva Bansal, Samuel Hunter, Kyu-Young Kim, Alan Lou, Caleb R Matthews, Ivan Villa-Renteria, Jerry Huayang Tang, Claire Tang, Fei Xia, Silvio Savarese, Hyowon Gweon, Karen Liu, Jiajun Wu, Li Fei-Fei
picking up trash
emptying trash cans
sweeping floors
taking trash outside
raking leaves
putting dishes away after cleaning
clean your kitty litter box
picking up litter
disposing of lawn clippings
cleaning the pool
unloading shopping from car
removing ice from walkways
cleaning bedroom
cleaning debris out of car
Rearrangement is a hard problem!
Habitat Rearrangement Challenge 2022
Andrew Szot, Karmesh Yadav, Alex Clegg, Vincent-Pierre Berges, Aaron Gokaslan, Angel Chang, Manolis Savva, Zsolt Kira, Dhruv Batra
Hard
There are two tracks in the Habitat Rearrangement Challenge.
- Rearrange-Easy: The agent must rearrange one object. Furthermore, all containers (such as the fridge, cabinets, and drawers) start open, meaning the agent never needs to open containers to access objects or goals. The task planning in rearrange-easy is static with the same sequence of navigation to the object, picking the object, navigating to the goal, and then placing the object at the goal. The maximum episode length is 1500 time steps.
- Rearrange: The agent must rearrange one object, but containers may start closed or open. Since the object may start in closed receptacles, the agent may need to perform intermediate actions to access the object. For example, an apple may start in a closed fridge and have a goal position on the table. To rearrange the apple, the agent first needs to open the fridge before picking the apple. The agent is not provided with task information about if these intermediate open actions need to be executed. This information needs to be inferred from the egocentric observations and goal specification. The maximum episode length is 5000 time steps.
Rearrangement is a hard problem!
Habitat Rearrangement Challenge 2022
Andrew Szot, Karmesh Yadav, Alex Clegg, Vincent-Pierre Berges, Aaron Gokaslan, Angel Chang, Manolis Savva, Zsolt Kira, Dhruv Batra
Hard
There are two tracks in the Habitat Rearrangement Challenge.
- Rearrange-Easy: The agent must rearrange one object. Furthermore, all containers (such as the fridge, cabinets, and drawers) start open, meaning the agent never needs to open containers to access objects or goals. The task planning in rearrange-easy is static with the same sequence of navigation to the object, picking the object, navigating to the goal, and then placing the object at the goal. The maximum episode length is 1500 time steps.
- Rearrange: The agent must rearrange one object, but containers may start closed or open. Since the object may start in closed receptacles, the agent may need to perform intermediate actions to access the object. For example, an apple may start in a closed fridge and have a goal position on the table. To rearrange the apple, the agent first needs to open the fridge before picking the apple. The agent is not provided with task information about if these intermediate open actions need to be executed. This information needs to be inferred from the egocentric observations and goal specification. The maximum episode length is 5000 time steps.
Robots may benefit from some notion of "commonsense"
Rearrangement helps evaluate robot commonsense

Commonsense is the
dark matter of robotics

One option is to learn commonsense from the bottom up
1. Collect a big dataset
2. Train end-to-end deep networks
Transformers
or ConvNets

Actions
Pixels
- But useful robot data is expensive

Deep Learning is a Box

Interpolation
Extrapolation
Deep Learning is a Box

Interpolation
Extrapolation

Roboticist
Vision
NLP
Deep Learning is a Box

Interpolation
Extrapolation
Internet
Meanwhile in NLP...
Large Language Models

Large Language Models
Internet
Meanwhile in NLP...

Books
Recipes
Code
News
Articles
Dialogue
Demo
Quick Primer on Language Models
Tokens (inputs & outputs)
Transformers (models)
Attention Is All You Need, NeurIPS 2017
Ashish Vaswani, Noam Shazeer, Niki Parmar, Jakob Uszkoreit, Llion Jones, Aidan N. Gomez, Lukasz Kaiser, Illia Polosukhin
Quick Primer on Language Models
Tokens (inputs & outputs)
Transformers (models)
Pieces of words (BPE encoding)
big
bigger
per word:
biggest
small
smaller
smallest
big
er
per token:
est
small

Attention Is All You Need, NeurIPS 2017
Ashish Vaswani, Noam Shazeer, Niki Parmar, Jakob Uszkoreit, Llion Jones, Aidan N. Gomez, Lukasz Kaiser, Illia Polosukhin
Quick Primer on Language Models
Tokens (inputs & outputs)
Transformers (models)
Self-Attention
Pieces of words (BPE encoding)
big
bigger
per word:
biggest
small
smaller
smallest
big
er
per token:
est
small


Attention Is All You Need, NeurIPS 2017
Ashish Vaswani, Noam Shazeer, Niki Parmar, Jakob Uszkoreit, Llion Jones, Aidan N. Gomez, Lukasz Kaiser, Illia Polosukhin
Bigger is Better

Neural Language Models: Bigger is Better, WeCNLP 2018
Noam Shazeer
Bigger is Better
Neural Language Models: Bigger is Better, WeCNLP 2018
Noam Shazeer

Bigger is Better
Neural Language Models: Bigger is Better, WeCNLP 2018
Noam Shazeer

Bigger is Better
Neural Language Models: Bigger is Better, WeCNLP 2018
Noam Shazeer

Bigger is Better
Neural Language Models: Bigger is Better, WeCNLP 2018
Noam Shazeer

Somewhere in the space of interpolation
Example?
Lives robot planning
Somewhere in the space of interpolation
Example?
Lives robot planning
Can LLMs give us top down commonsense?
PaLM-SayCan & Socratic Models
Socratic Models: Composing Zero-Shot Multimodal Reasoning with Language
https://socraticmodels.github.io
LLMs on robots! Open research problem, but here's one way to do it...

Do As I Can, Not As I Say: Grounding Language in Robotic Affordances
https://say-can.github.io
Socratic Models: Composing Zero-Shot Multimodal Reasoning with Language
https://socraticmodels.github.io

Visual Language Model
CLIP, ALIGN, LiT,
SimVLM, ViLD, MDETR
Human input (task)
Do As I Can, Not As I Say: Grounding Language in Robotic Affordances
https://say-can.github.io
PaLM-SayCan & Socratic Models
LLMs on robots! Open research problem, but here's one way to do it...
Socratic Models: Composing Zero-Shot Multimodal Reasoning with Language
https://socraticmodels.github.io

Visual Language Model
CLIP, ALIGN, LiT,
SimVLM, ViLD, MDETR
Human input (task)
Large Language Model for Planning (e.g. SayCan)
Language-conditioned Policies
Do As I Can, Not As I Say: Grounding Language in Robotic Affordances
https://say-can.github.io
PaLM-SayCan & Socratic Models
LLMs on robots! Open research problem, but here's one way to do it...
Live Demo
Limits of language as a "state" representation
- Loses spatial precision
- Highly multimodal (lots of different ways to say the same thing)
- Not as information-rich as in-domain representations (e.g. images)
Limits of language as a "state" representation
- Loses spatial precision
- Highly multimodal (lots of different ways to say the same thing)
- Not as information-rich as in-domain representations (e.g. images)
Can we leverage continuous pre-trained word embedding spaces?

An Image is Worth One Word: Personalizing Text-to-Image Generation using Textual Inversion
Rinon Gal et al. 2022
Limits of language as a "state" representation
- Only for high level? what about control?
Perception
Planning
Control
Socratic Models
Inner Monologue
ALM + LLM + VLM
SayCan
Wenlong Huang et al, 2022
LLM
Imitation? RL?
Engineered?
Intuition and commonsense is not just a high-level thing
Intuition and commonsense is not just a high-level thing
Applies to low-level behaviors too
- Spatial: "move a little bit to the left"
- Temporal: "move faster"
- Functional: "balance yourself"
Demo
Intuition and commonsense is not just a high-level thing
Applies to low-level behaviors too
- Spatial: "move a little bit to the left"
- Temporal: "move faster"
- Functional: "balance yourself"
Seems to be stored in the depths of in language models... how to extract it?
Language models can write code
Code as a medium to express low-level commonsense
Live Demo
Language models can write code
Code as a medium to express more complex plans

Jacky Liang, Wenlong Huang, Fei Xia, Peng Xu, Karol Hausman, Brian Ichter, Pete Florence, Andy Zeng
code-as-policies.github.io
Code as Policies: Language Model Programs for Embodied Control


Live Demo
Language models can write code
Code as a medium to express more complex plans

Jacky Liang, Wenlong Huang, Fei Xia, Peng Xu, Karol Hausman, Brian Ichter, Pete Florence, Andy Zeng
code-as-policies.github.io
Code as Policies: Language Model Programs for Embodied Control



Live Demo
Language models can write code

Jacky Liang, Wenlong Huang, Fei Xia, Peng Xu, Karol Hausman, Brian Ichter, Pete Florence, Andy Zeng
code-as-policies.github.io
Code as Policies: Language Model Programs for Embodied Control
use NumPy,
SciPy code...
Language models can write code

Jacky Liang, Wenlong Huang, Fei Xia, Peng Xu, Karol Hausman, Brian Ichter, Pete Florence, Andy Zeng
code-as-policies.github.io
Code as Policies: Language Model Programs for Embodied Control



- PD controllers
- impedance controllers
Language models can write code

Jacky Liang, Wenlong Huang, Fei Xia, Peng Xu, Karol Hausman, Brian Ichter, Pete Florence, Andy Zeng
code-as-policies.github.io
Code as Policies: Language Model Programs for Embodied Control

Language models can write code

Jacky Liang, Wenlong Huang, Fei Xia, Peng Xu, Karol Hausman, Brian Ichter, Pete Florence, Andy Zeng
code-as-policies.github.io
Code as Policies: Language Model Programs for Embodied Control


What is the foundation model for robotics?
On the road to robot commonsense
Robot Learning
Language Models
Not a lot of robot data
Lots of Internet data




500 expert demos
5000 expert demos
50 expert demos
On the road to robot commonsense
Robot Learning
Language Models
Not a lot of robot data
Lots of Internet data






500 expert demos
5000 expert demos
50 expert demos
On the road to robot commonsense
Robot Learning
Language Models
- Finding other sources of data (sim, YouTube)
- Improve data efficiency with prior knowledge
Not a lot of robot data
Lots of Internet data






500 expert demos
5000 expert demos
50 expert demos
On the road to robot commonsense
Robot Learning
Language Models
- Finding other sources of data (sim, YouTube)
- Improve data efficiency with prior knowledge
Not a lot of robot data
Lots of Internet data






500 expert demos
5000 expert demos
50 expert demos
Embrace language to help close the gap!
Towards grounding everything in language
Language
Control
Vision
Tactile
Socratic Models: Composing Zero-Shot Multimodal Reasoning with Language
https://socraticmodels.github.io
Lots of data
Less data
Less data
"Language" as the glue for intelligent machines
Language
Perception
Planning
Control
Socratic Models: Composing Zero-Shot Multimodal Reasoning with Language
https://socraticmodels.github.io
"Language" as the glue for intelligent machines
Language
Perception
Planning
Control
Socratic Models: Composing Zero-Shot Multimodal Reasoning with Language
https://socraticmodels.github.io
We have some reason to believe that
"the structure of language is the structure of generalization"
To understand language is to understand generalization
https://evjang.com/2021/12/17/lang-generalization.html
Sapir–Whorf hypothesis
Towards grounding everything in language
Language
Perception
Planning
Control
Humans
Towards grounding everything in language
Language
Perception
Planning
Control
Humans
Not just for general robots,
but for human-centered intelligent machines!
Thank you!
Pete Florence
Adrian Wong
Johnny Lee




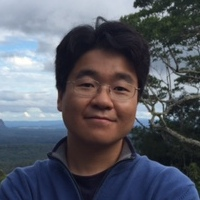






Vikas Sindhwani
Stefan Welker
Vincent Vanhoucke
Kevin Zakka
Michael Ryoo
Maria Attarian
Brian Ichter
Krzysztof Choromanski
Federico Tombari

Jacky Liang
Aveek Purohit

Wenlong Huang
Fei Xia
Peng Xu
Karol Hausman





and many others!
Scale alone might not be enough
Robot Learning
Language Models
Not a lot of robot data
Lots of Internet data
adapted from Tomás Lozano-Pérez
Machine learning is a box
... but robotics is a line
- Finding other sources of data (sim, YouTube)
- Improve data efficiency with prior knowledge
Different embodiments etc....
A possible middleground
Robot Learning
Language Models
Not a lot of robot data
Lots of Internet data
Embrace Compositionality
adapted from Tomás Lozano-Pérez
Machine learning is a box
... but robotics is a line
A possible middleground
Robot Learning
Language Models
Not a lot of robot data
Lots of Internet data
Embrace Compositionality
adapted from Tomás Lozano-Pérez
Machine learning is a box
... but robotics is a line
2. composing them
autonomously
1. build boxes
2022-NeurIPS-Rearrangement-Talk
By Andy Zeng
2022-NeurIPS-Rearrangement-Talk
- 482