

DeepMind Robotics (formerly known as Brain)
Learning Discontinuities for
Contact-Rich Manipulation
Andy Zeng
Embracing Contacts
ICRA 2023 Workshop

Manipulation
TossingBot
Manipulation
PaLM-SayCan
Learning to interact with the physical world (through contact)
RoboPianist
with machine learning from pixels
Discontinuities in contact-rich manipulation
and how we might go about modeling them
Continuous-Time Representations
Perception & State Estimation
Imitation Learning
Occlusions
Actions
Sensor Fusion


Discontinuities in contact-rich manipulation
and how we might go about modeling them
Continuous-Time Representations
Perception & State Estimation
Imitation Learning
Occlusions
Actions
Sensor Fusion


Implicit Behavior Cloning
Real human teleop trajectories
are full of discontinuities
Imitation Learning
BC policy learning as: instead of:
"Implicit Behavioral Cloning"
Pete Florence et al., CoRL 2021
Implicit Behavior Cloning
Real human teleop trajectories
are full of discontinuities
Imitation Learning
Learn a probability distribution over actions:
- Conditioned on observation (raw images)
- Uniformly sampled negatives
BC policy learning as: instead of:
"Implicit Behavioral Cloning"
Pete Florence et al., CoRL 2021
Implicit Behavior Cloning
Learn a probability distribution over actions:
- Conditioned on observation (raw images)
- Uniformly sampled negatives
BC policy learning as: instead of:

Semi-Algebraic Approximation using Christoffel-Darboux Kernel
Marx et al., Springer 2021

"Implicit Behavioral Cloning"
Pete Florence et al., CoRL 2021
Implicit Behavior Cloning
+ Can represent multi-modal actions
+ More sample efficiently learn discontinuous trajectories
BC policy learning as: instead of:

Fly left or right around the tree?


The Convergence Rate of Neural Networks for Learned Functions of Different Frequencies
Ronen Basri et al., NeurIPS 2019
subtle but decisive maneuvers
"Implicit Behavioral Cloning"
Pete Florence et al., CoRL 2021
Discontinuities in contact-rich manipulation
and how we might go about modeling them
Continuous-Time Representations
Perception & State Estimation
Imitation Learning
Occlusions
Actions
Sensor Fusion


Discontinuities in contact-rich manipulation
and how we might go about modeling them
Continuous-Time Representations
Perception & State Estimation
Imitation Learning
Occlusions
Actions
Sensor Fusion


Occlusions in Perception & State Estimation



Occlusions appear as
discontinuities in image space
3D data: self-occlusions

Contact points are often occluded
Partial observability
Deformable Object State Estimation with Implicit SDFs
"VIRDO: Visio-Tactile Implicit Representations of Deformable Objects"
"VIRDO++: Real-World, Visuo-Tactile Dynamics and Perception of Deformable Objects"
Youngsun Wi, Pete Florence, Andy Zeng, Nima Fazeli. ICRA & CoRL 2022



Deformable Object State Estimation with Implicit SDFs
"VIRDO: Visio-Tactile Implicit Representations of Deformable Objects"
"VIRDO++: Real-World, Visuo-Tactile Dynamics and Perception of Deformable Objects"
Youngsun Wi, Pete Florence, Andy Zeng, Nima Fazeli. ICRA & CoRL 2022




Deformable Object State Estimation with Implicit SDFs
"VIRDO: Visio-Tactile Implicit Representations of Deformable Objects"
"VIRDO++: Real-World, Visuo-Tactile Dynamics and Perception of Deformable Objects"
Youngsun Wi, Pete Florence, Andy Zeng, Nima Fazeli. ICRA & CoRL 2022



Discontinuities in contact-rich manipulation
and how we might go about modeling them
Continuous-Time Representations
Perception & State Estimation
Imitation Learning
Occlusions
Actions
Sensor Fusion


Discontinuities in contact-rich manipulation
and how we might go about modeling them
Continuous-Time Representations
Perception & State Estimation
Imitation Learning
Occlusions
Actions
Sensor Fusion


Observation data may appear discontinuous
"Multiscale Sensor Fusion and Continuous Control with Neural CDEs"
Sumeet Singh, Francis McCann Ramirez, Jacob Varley, Andy Zeng, Vikas Sindhwani. ICRA 2022

but the underlying process might not be
input: 30 Hz camera images
input: 100 Hz F/T readings...
output: 50 Hz actions?
Observation data may appear discontinuous
"Multiscale Sensor Fusion and Continuous Control with Neural CDEs"
Sumeet Singh, Francis McCann Ramirez, Jacob Varley, Andy Zeng, Vikas Sindhwani. ICRA 2022

but the underlying process might not be

input: 30 Hz camera images
input: 100 Hz F/T readings...
output: 50 Hz actions?
Time-continuous policies?

observation t=1
observation t=0
action t=0.5?
Observation data may appear discontinuous
"Multiscale Sensor Fusion and Continuous Control with Neural CDEs"
Sumeet Singh, Francis McCann Ramirez, Jacob Varley, Andy Zeng, Vikas Sindhwani. ICRA 2022

but the underlying process might not be


Observation data may appear discontinuous
"Multiscale Sensor Fusion and Continuous Control with Neural CDEs"
Sumeet Singh, Francis McCann Ramirez, Jacob Varley, Andy Zeng, Vikas Sindhwani. ICRA 2022

but the underlying process might not be




task success & completion
T = time between readings

Observation data may appear discontinuous
"Multiscale Sensor Fusion and Continuous Control with Neural CDEs"
Sumeet Singh, Francis McCann Ramirez, Jacob Varley, Andy Zeng, Vikas Sindhwani. ICRA 2022

but the underlying process might not be





task success & completion
rate of image frame dropout
T = time between readings

Discontinuities in contact-rich manipulation
and how we might go about modeling them
Continuous-Time Representations
Perception & State Estimation
Imitation Learning
Occlusions
Actions
Sensor Fusion


Manipulation without contact?
our objective is to affect change in the world
Jimmy Wu, Xingyuan Sun, Andy Zeng, Shuran Song, Szymon Rusinkiewicz, Thomas Funkhouser
IROS & RA-L 2022



Learning Pneumatic Non-Prehensile Manipulation with a Mobile Blower


Thank you!
Pete Florence
Youngsun Wi
Johnny Lee




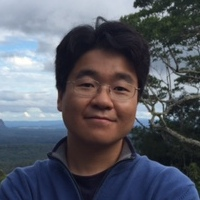




Vikas Sindhwani
Jimmy Wu
Vincent Vanhoucke
Kevin Zakka
Michael Ryoo
Maria Attarian
Brian Ichter
Krzysztof Choromanski
Federico Tombari

Jacky Liang
Sumeet Singh
Wenlong Huang
Fei Xia
Peng Xu
Karol Hausman





and many others!



2023-ICRA-workshop-contact
By Andy Zeng
2023-ICRA-workshop-contact
- 240