Minimizing movements schemes
with general movement limiters
Flavien Léger
joint works with Pierre-Cyril Aubin-Frankowski,
Gabriele Todeschi, François-Xavier Vialard
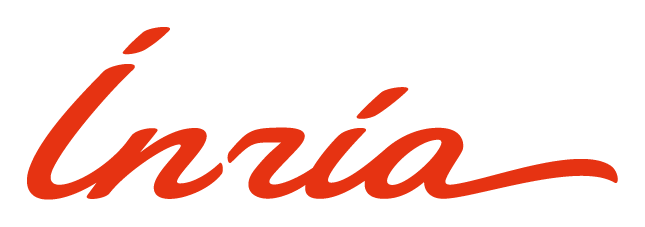
What I will present
Theory for minimizing movement schemes in infinite dimensions and in nonsmooth (nondifferentiable) settings, with a movement limiter given by a general cost function.
Main motivation: optimization on a space of measures P(M):
minimize E:P(M)→R∪{+∞}
Typical scheme:
where D(μ,ν)=
transport cost: W22(μ,ν), Tc(μ,ν),...
Bregman divergence: KL(μ,ν),...
Csiszár divergence: ∫M(μ−ν)2,...
...
What I will present
Theory for minimizing movement schemes in infinite dimensions and in nonsmooth (nondifferentiable) settings, with a movement limiter given by a general cost function.
1. Formulations for implicit and explicit schemes in a general setting
2. Theory for rates of convergence based on convexity along specific paths, and generalized “L-smoothness” (“L-Lipschitz gradients”) for explicit scheme
Implicit scheme
Minimize E:X→R∪{+∞}, where X is a set (set of measures, metric space...).
Use D:X×Y→R∪{+∞}, where Y is another set (often X=Y).
Algorithm
(Implicit scheme)
Rem: formulated as an alternating minimization
Motivations for general D(x,y):
Implicit scheme
- D(x,y) tailored to the problem
- Gradient flows “x˙(t)=−∇E(x(t))”
⏵ Define gradient flows in nonsmooth, metric settings: D=2τd2,τ→0
⏵ D(x,y) as a proxy for d2(x,y) (same behaviour on the diagonal):
(Ambrosio–Gigli–Savaré ’05)
(De Giorgi ’93)
Toy example: x˙(t)=−∇2u(x(t))−1∇E(x(t)), u:Rd→R strictly convex
Two approaches:
d= distance for Hessian metric ∇2u
Alternating minimization
Given Φ:X×Y→R∪{+∞} to minimize, define
Examples of AM: Sinkhorn, Expectation–Maximization, Projections onto convex set, SMACOF for multidimensional scaling...
Then alternating minimization of Φ⟺ implicit method with E and D:
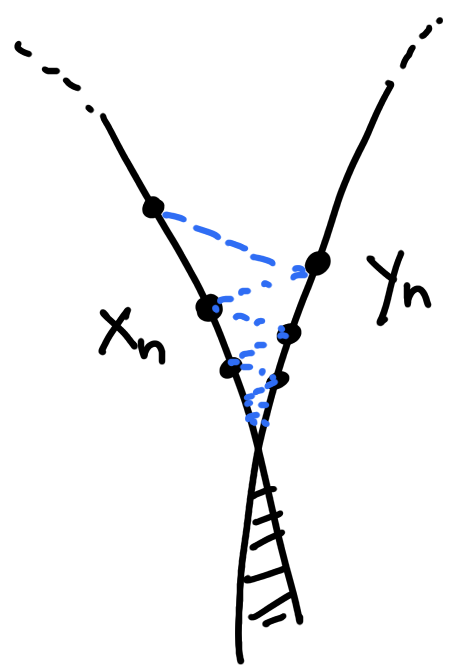
Explicit minimizing movements: warm-up
Two steps:
1) majorize: find the tangent parabola (“surrogate”)
2) minimize: minimize the surrogate
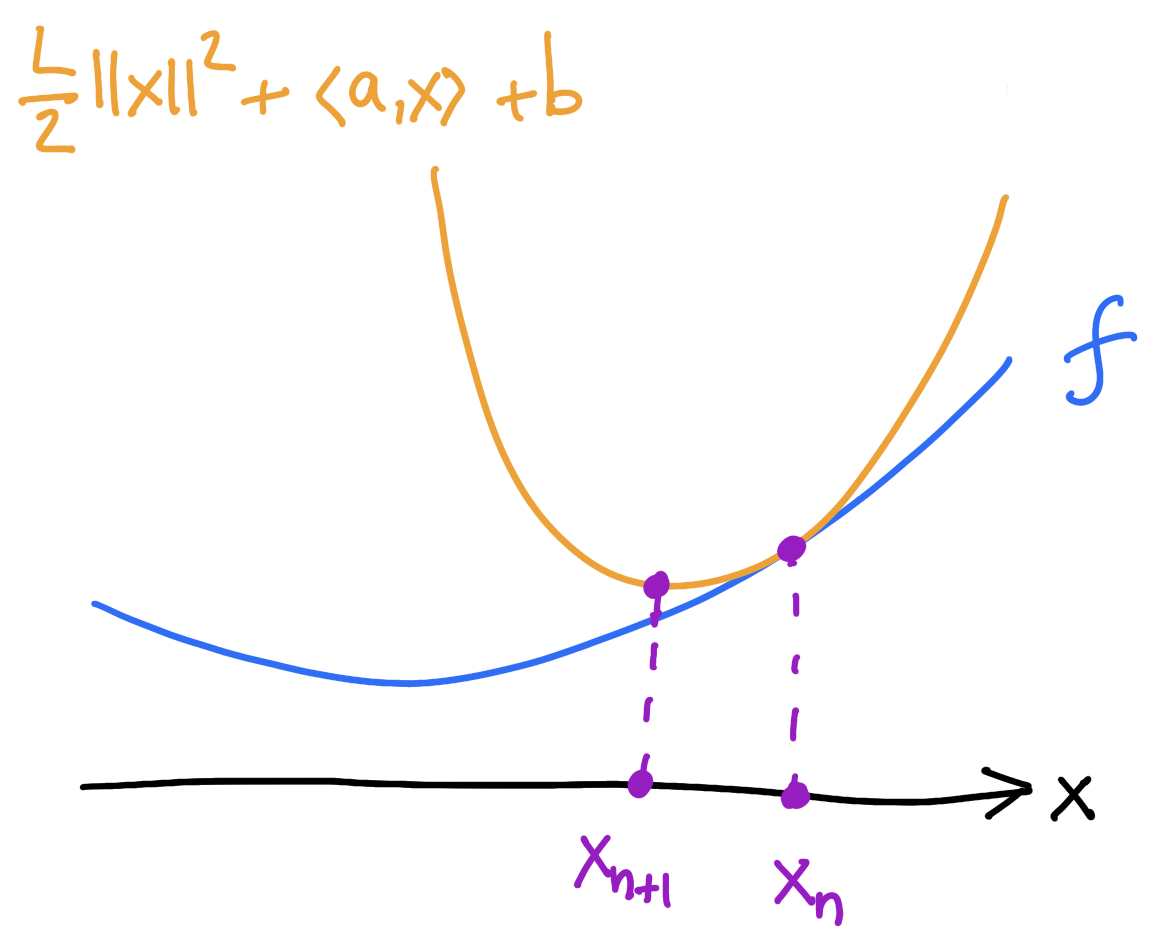
xn+1=xn−L1∇E(xn)
E:Rd→R
Gradient descent
“Nonsmooth” formulation of Gradient descent:
Warm-up question: how to formulate GD in a nonsmooth context? (ex: metric space)
Explicit minimizing movements: warm-up
Two steps:
1) majorize: find the tangent parabola (“surrogate”)
2) minimize: minimize the surrogate
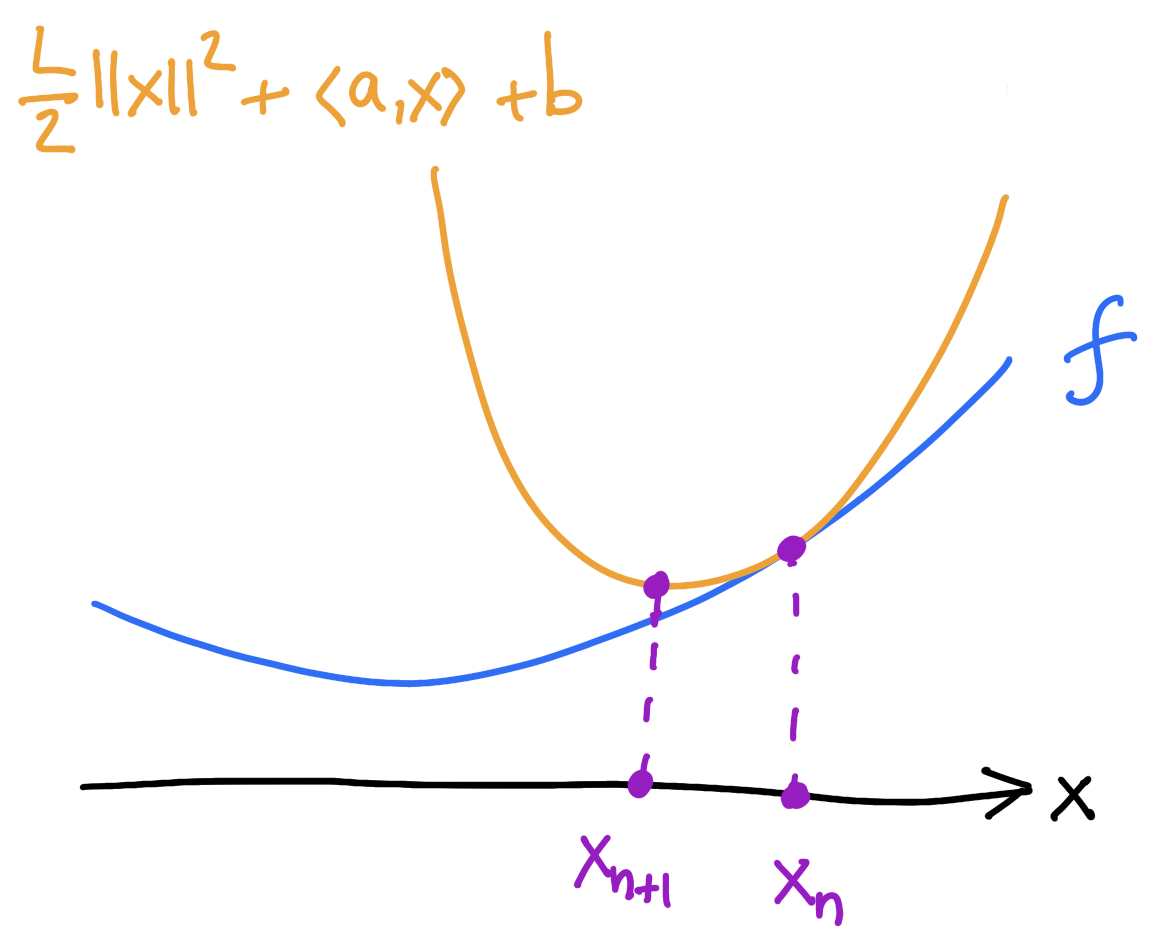
If E is L-smooth (∇2E≤LId×d) then it sits below the surrogate:
E(x)
≤
E(xn)+⟨∇E(xn),x−xn⟩+2L∥x−xn∥2
xn+1=xn−L1∇E(xn)
Explicit minimizing movements: c-concavity
∃h:Y→R∪{+∞}
Definition.
E is c-concave if
generalizes “L-smoothness”
Abstract setting:
Smallest such h is the c-transform
h(y)=supx∈XE(x)−D(x,y)
∃h:Y→R∪{+∞}
Definition.
E is c-concave if
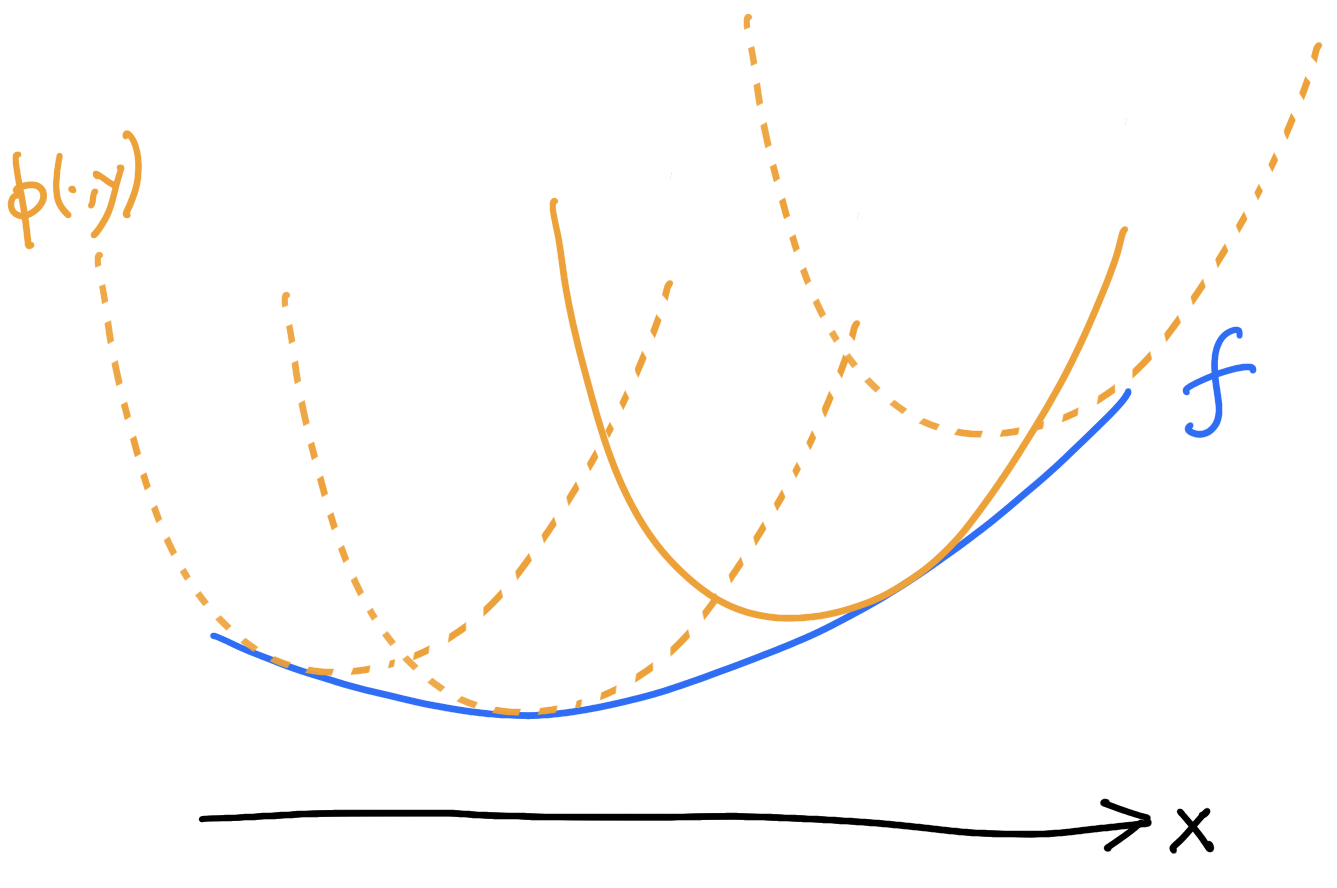
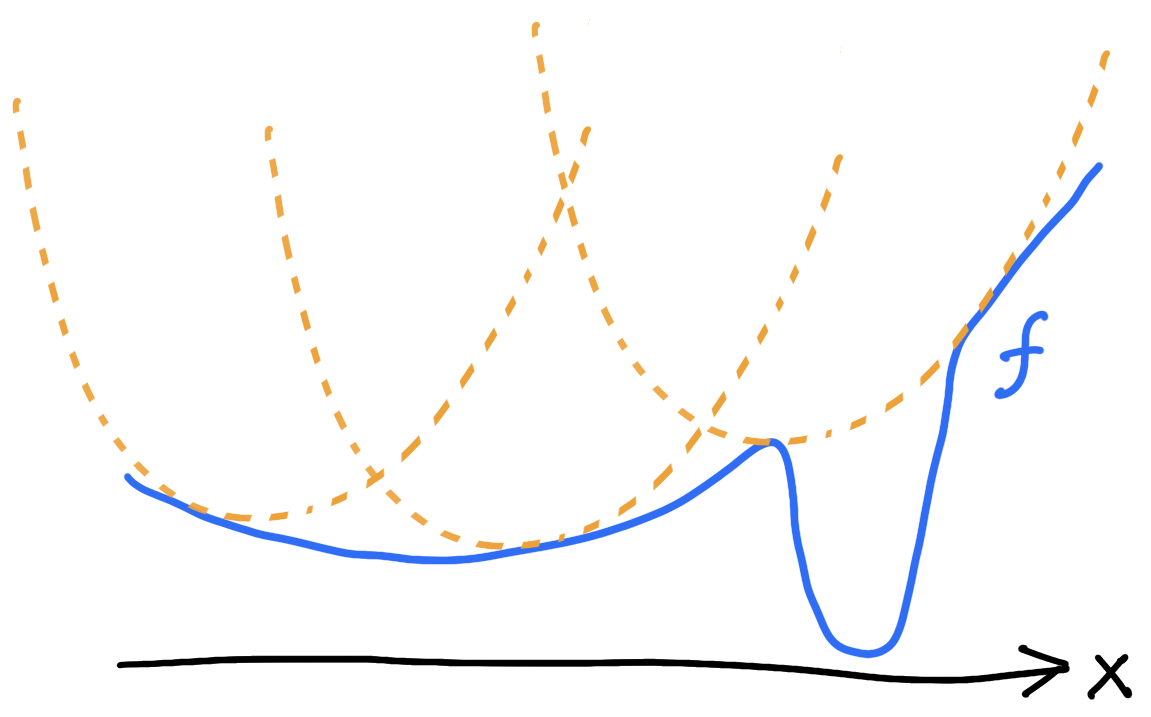
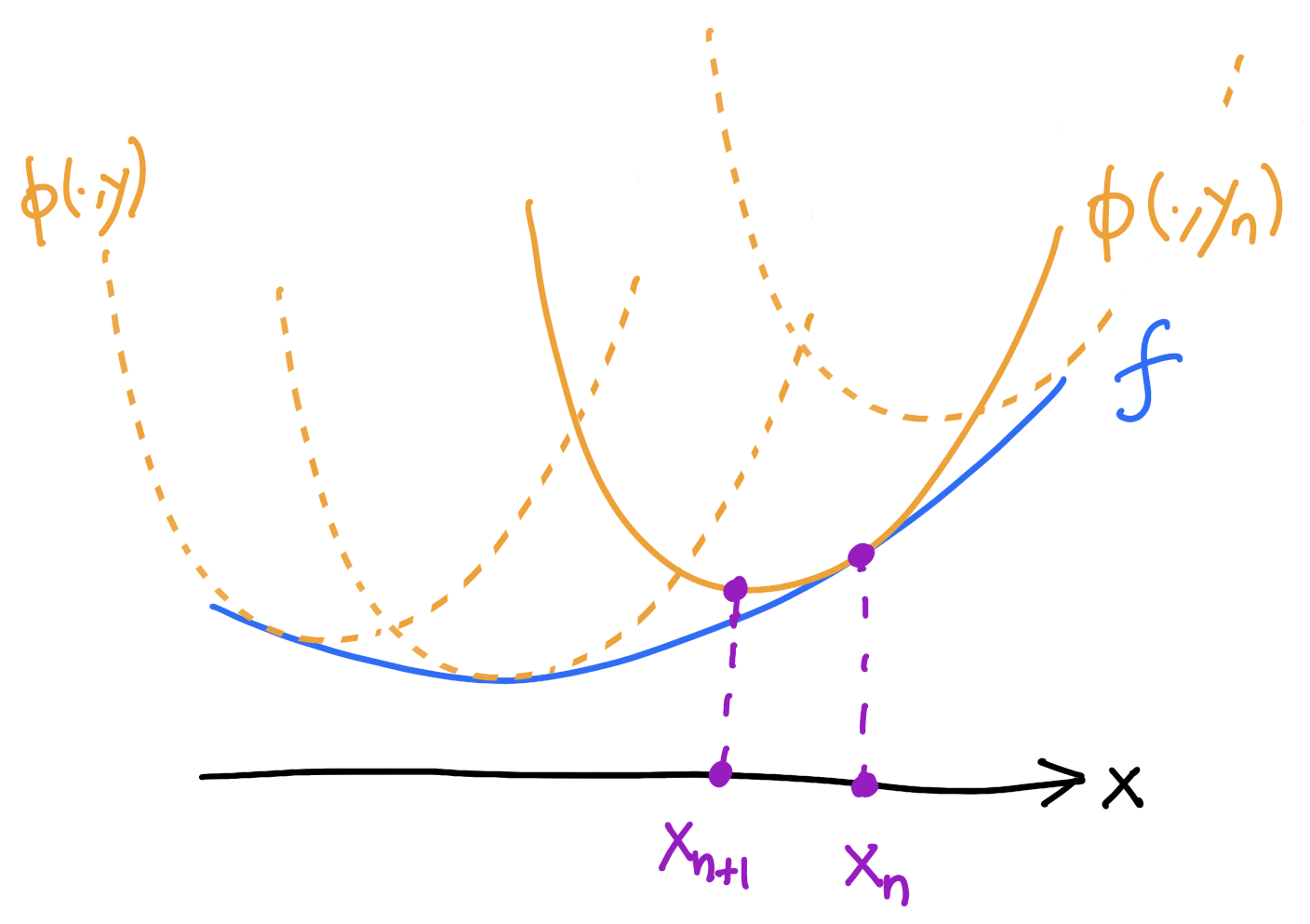
c-concave
not c-concave
Explicit minimizing movements: c-concavity
Differentiable NNCC setting. Suppose that ∀x∈X,∃y∈Y: ∇xD(x,y)=∇E(x) and
∇2E(x)≤∇xx2D(x,y).
Then E is c-concave.
Theorem.
(L–Aubin-Frankowski, à la Trudinger–Wang '06)
∃h:Y→R∪{+∞}
Definition.
E is c-concave if
Explicit minimizing movements: c-concavity
Explicit minimizing movements
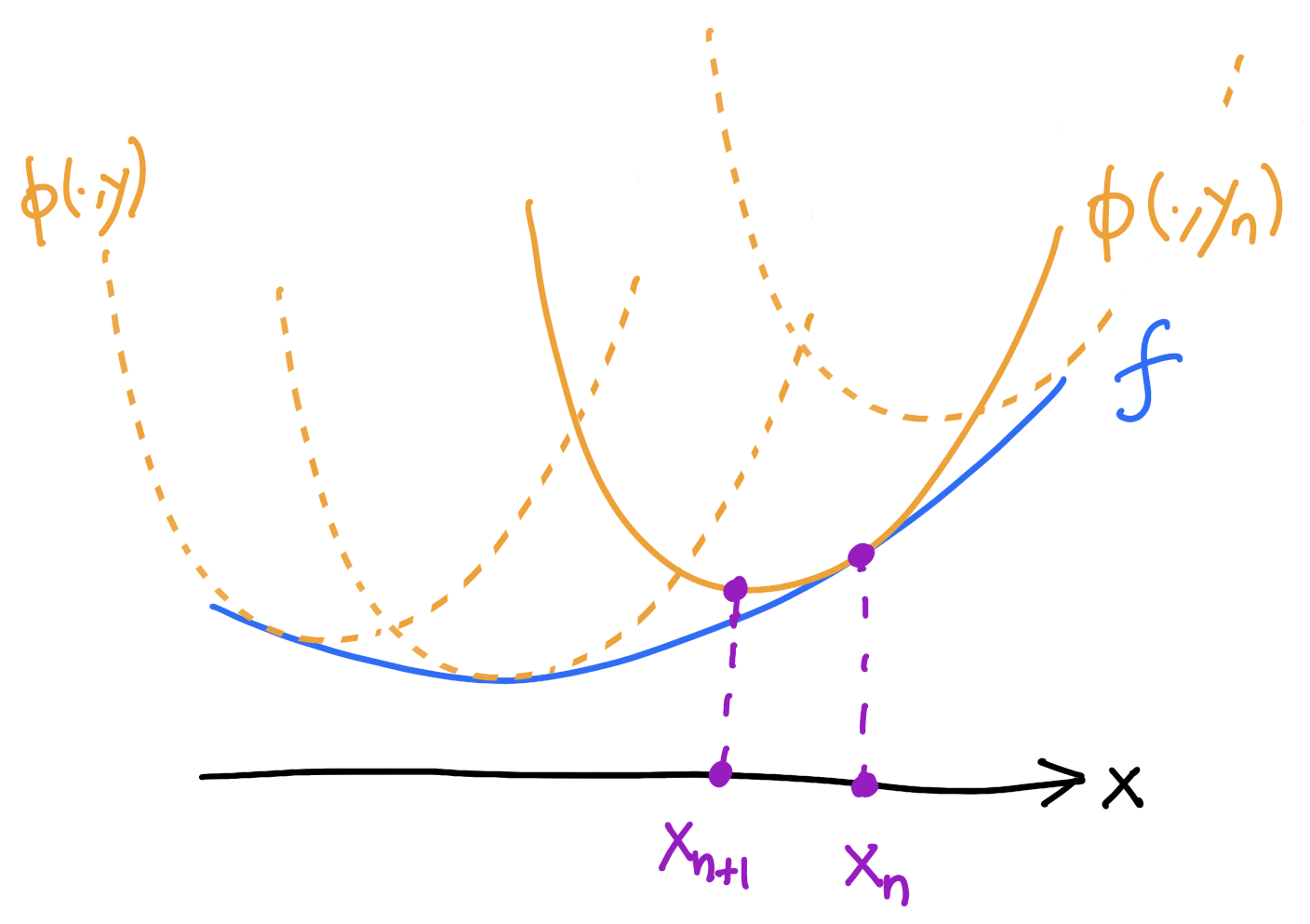
(majorize)
(minimize)
Algorithm.
(Explicit scheme)
Assume E c-concave.
(L–Aubin-Frankowski '23)
Explicit minimizing movements
X,Y smooth manifolds, D∈C1(X×Y), E∈C1(X) c-concave
Under certain assumptions, the explicit scheme can be written as
More: nonsmooth mirror descent, convergence rates for Newton
2. Convergence rates
EVI and convergence rates
Definition.
(Csiszár–Tusnády ’84)
(L–Aubin-Frankowski ’23)
Evolution Variational Inequality (or five-point property):
If (xn,yn) satisfy the EVI then
sublinear rates when μ=0
exponential rates when μ>0
Theorem.
(L–Aubin-Frankowski '23)
(Ambrosio–Gigli–Savaré ’05)
EVI
Take X=Y, D≥0, D(x,x)=0⟶yn+1=xn,
⏵ EVI as a property of E: ∃xn ∈x∈XargminE(x)+D(x,xn−1) s.t.
⏵ Proving the EVI: find a path x(s) along which E(x)−μD(x,xn)+D(x,xn−1)−D(x,xn) is convex ( → local to global)
Ex: E:Rd→R τμ-convex, D(x,y)=2τ1∥x−y∥2
3. A synthetic formulation of nonnegative cross-curvature
Variational c-segments and NNCC spaces
⏵ s↦(x(s),yˉ) is a variational c-segment if D(x(s),yˉ) is finite and
⏵ (X×Y,D) is a space with nonnegative cross-curvature (NNCC space) if variational c-segments always exist.
X,Y two arbitrary sets, D:X×Y→R∪{±∞}.
Definition.
(L–Todeschi–Vialard '24)
Origins in regularity of optimal transport
(Ma–Trudinger–Wang ’05)
(Trudinger–Wang ’09)
(Kim–McCann ’10)
convexity of the set of c-concave functions
(Figalli–Kim–McCann '11)
Properties of NNCC spaces
Stable by products
Stable by quotients with “equidistant fibers”
(connect to: Kim–McCann '12)
(L–Todeschi–Vialard '24)
(finite) collection of NNCC spaces (Xa×Ya,ca)a=1..n,
X=X1×⋯×Xn,Y=Y1×⋯×Yn,c(x,y)=c1(x1,y1)+⋯+cn(xn,yn)
(X×Y,c) is NNCC.
c:X×Y→[−∞,+∞], P1:X→X, P2:Y→Y s.t. “equidistant fibers”
Then: (X×Y,c) NNCC ⟹ (X×Y,c) NNCC
(connect to: Kim–McCann '12)
Quotients:
Products:
Properties of NNCC spaces
Metric cost c(x,y)=d2(x,y) NNCC⟹PC
(connect to: Ambrosio–Gigli–Savaré ’05)
(connect to: Loeper ’09)
(L–Todeschi–Vialard '24)
Application: transport costs
“Proof.”
(X×Y,c) NNCC ⟹ (P(X)×P(Y),Tc) NNCC
Ex: W22 on Rn, on Sn, OT with Bregman costs...
Variational c-segments ≈ generalized geodesics
X,Y Polish, c:X×Y→R∪{+∞} lsc
1. (U,V)↦Ec(U,V)=∫Ωc(U(ω),V(ω))dP(ω) is NNCC when (X×Y,c) is NNCC “product of NNCC”
2. law(U)=μinfEc(U,V)=law(V)=νinfEc(U,V)=Tc(μ,ν) “equidistant fibers”
Theorem.
(L–Todeschi–Vialard '24)
Examples
Gromov–Wasserstein
Costs on measures. The following are NNCC:
Relative entropy KL(μ,ν)=∫log(dνdμ)dμ,
Hellinger D(μ,ν)=∫(dλdμ−dλdν)2dλ,
Fisher–Rao = length space associated with Hellinger
(G×G,GW2) is NCCC
X=[X,f,μ] and Y=[Y,g,ν]∈G
Any Hilbert or Bregman cost is NNCC:
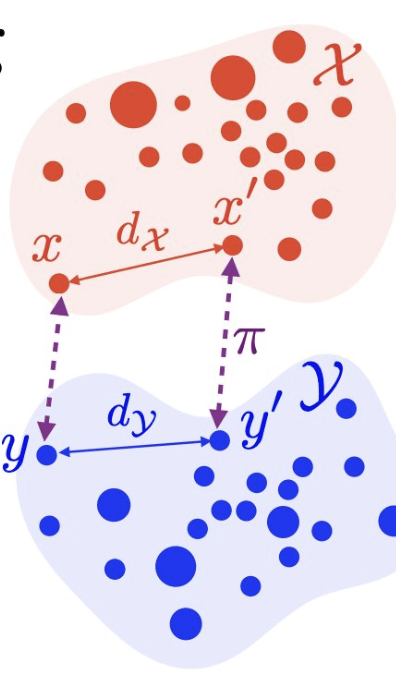
G. Peyré
Convergence rates for minimizing movements
Suppose that for each x∈X and n≥0,
Then sublinear (μ=0) or linear (μ>0) convergence rates.
⏵ there exists a variational c-segment s↦(x(s),yn) on (X×Y,D) with x(0)=xn and x(1)=x
⏵ s↦E(x(s))−μD(x(s),yn+1) is convex
⏵ s→0+limsD(x(s),yn+1)=0
Theorem.
(L–Aubin-Frankowski '23)
Thank you!
(Lyon 2025-01-29)
By Flavien Léger
(Lyon 2025-01-29)
- 68