Screening of Budgeted Agents
Hedyeh Beyhaghi, Modibo Camara, Jason Hartline, Aleck Johnsen, Sheng Long
Office of Admissions




- Goal: admit high-skill students
- Method: Design a test




- Goal: admit high-skill students
- Method: Design a test
Office of Admissions
Switch perspectives ...
Text
Office of Admissions
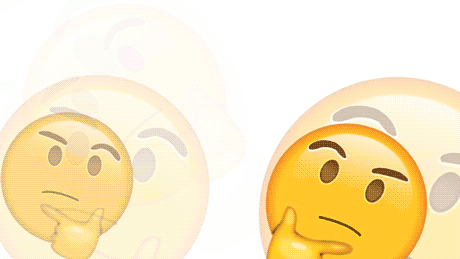




⛳: admit high-skilled students
max score: 75%
max score: 60%
max score: 93%
max score: 88%
skill : ???
budget: ???
skill : ???
budget: ???
skill : ???
budget: ???
skill : ???
budget: ???




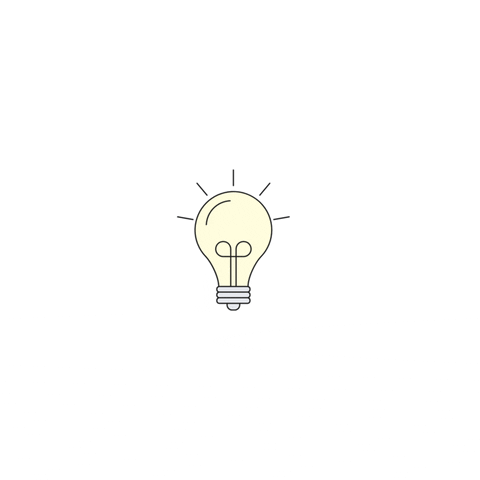
idea: admit those who score above 90
✅
✅
\(\to\) we argue that this is not the best admissions policy
❌
❌
max score: 60%
max score: 88%
max score: 72%
max score: 93%
skill : ???
budget: ???
skill : ???
budget: ???
skill : ???
budget: ???
skill : ???
budget: ???




max score: 75%
max score: 60%
max score: 93%
max score: 88%
Why not?
High
Low
High
Low
Budget
Skill
Recall:
- low score \(\neq\) low skill
- deterministic admissions policy cannot differentiate
Proposal: 🎲 Multi-track admissions policy
In the above example,
\(\to\) We argue that:
- multi-track admissions policy is optimal for budgeted agents
- In particular, multi-track admissions policy is better than deterministic threshold policy
Comparison
allocation
score
0
1
0.9
allocation
score
0
1
75
0.8
60
1
❓Why does multi-track work better?
low-skilled students
high-skilled students
When there are no constraints on effort:
Given deterministic policy:
- low-skill students, regardless of budget, won't participate
- Only the high-skill high-budget student can achieve positive utility
allocation
0
1
0.9
1
score
score
utility
high skill
low skill
Given probabilistic policy:
- low-skill students, regardless of budget, won't participate
- Only the high-skill high-budget student can achieve positive utility
high skill
low skill
Given probabilistic policy:
- low-skill students, regardless of budget, won't participate
- Only the high-skill high-budget student can achieve positive utility
❓Why does multi-track work better?
low-skilled students
When there are no constraints on effort:
Three options:
A. Score above 90 and admitted with certainty
B. Score between 70 and 90 and admitted w.p. 0.7
C. Don't apply.
low-skilled students
high-skilled students
preference direction
Suppose all students face the same budget \(\color{red}{b = 0.25}\)
Suppose all students face the same budget \(\color{red}{b = 0.25}\)
Compare this to the deterministic threshold:
Observe that we are admitting low-skilled students with less probability, compared to deterministic threshold
Per Hedyeh's suggestion:
-- what would a menu of lotteries look like?
-- what would people with different skill types and budget choose?
\(\to\) How does partial allocation separate high skill from low skill?
low-skilled students
high-skilled students
❓For which of these agents is their budget a tight constraint?
A: For the low-skilled agents
x
q
u
e
\(\to\) What if we introduce subsidies?
low-skilled students
high-skilled students
preference direction
Next step
- Introducing subsidies ...
To conclude:
- Multi-track admissions policy is optimal
- In particular, multi-track admissions policy is better than deterministic, single-track admissions policy
- Partial allocation helps differentiate between high-skilled and low-skilled students
Thank you for listening!
🪦 Slide Graveyard
Alice.
- ... is preparing for some exam
- ... has limited time to prepare

Office of Admissions




skill: ???
skill: ???
skill: ???
skill: ???
score: 75%
score: 60%
score: 93%
score: 88%
Why not?
✅
✅
\(\to\) we argue that this is not the best admissions rule
budget:
⌛
budget:⌛⌛⌛
budget:
⌛
budget:⌛⌛⌛
students may face constraints in budgets (on time)
❌
❌




Why not?
\(\to\) we argue that this is not the best admissions policy
students may face constraints in budgets (on time)
Introduction
- Given privately constrained public signals, how do we optimally allocate to agents of different types?
- Key idea: stochastic allocation enables higher allocation to a high type than to a low type, even when their observed signal is approximately equal due to budget constraints.
- Multi-dimensional, monopolistic screening problem
Menu
2-skill 2-budget agents pick from the menu
Explain why agents pick what they pick
Model
- Principal \(P\) observes a public signal of quality:
- \(P\) wants to hire agents with skills above threshold \(\tau \in \mathbb{R}_+\)
- \(P\) needs to design an allocation rule \(y:\mathbb{R}_+ \to [0, 1]\)
- Agent \(A\) has private type \((s, b)\)
- \(s\): skill; \(b\): budget on effort;
- \(s, b\) independent
- \(A\) wants to be hired.
\(\to\) \(A\)'s utility: \(u_A(x, e) := x- e\)
\(x\): probability of being hired / allocated
\(\to P\)'s utility from hiring \(A\): \(u_P := s - \tau\)
Main results
- An optimal mechanism for \(2\)-skill \(n\)-budget can be identified, and it takes the shape of a slanted-step function.
- Lottery menus leverages feasibility to improve allocation for high-skill agents
I. Deterministic threshold mechanism is not optimal
- A deterministic threshold mechanism sets a quality threshold \(\underline{q} \in \mathbb{R}_+\) and hires an agent if and only if the agent exhibits public quality \(q \geq \underline{q}\)
- When agents have different budgets, we can prove via counterexample that a deterministic threshold mechanism is not optimal.
II. Lottery menus leverage feasibility
to improve allocation for high-skill types
- A lottery menu mechanism is a (weakly) monotone allocation rule \(y\) with menu options \((\underline q, x)\) where \(x\) is the probability of allocation resulting from an agent exhibiting quality \(\underline q\).
- A menu option \((\underline q, x)\) is feasible for an agent \(A=(s,b)\) iff
- \((\underline q, x)\) is affordable: \(\underline q / s \leq b\)
- \((\underline q, x)\) is a rational choice: \(x - \underline q / s \geq 0\)
minimal effort to reach \(\underline q\)
II. Lottery menus leverage feasibility
to improve allocation for high-skill types
- A lottery menu mechanism is a (weakly) monotone allocation rule \(y\) with menu options \((\underline q, x)\) where \(x\) is the probability of allocation resulting from an agent exhibiting quality \(\underline q\).
- A menu option \((\underline q, x)\) is feasible for an agent \(A=(s,b)\) iff
- \((\underline q, x)\) is affordable: \(\underline q / s \leq b\)
- \((\underline q, x)\) is a rational choice: \(x - \underline q / s \geq 0\)
\((\underline q, s)\) is feasible for agent \(A=(s,b)\) iff \(\underline q / s \leq \min\{b, x\}\)
II. Lottery menus leverage feasibility
to improve allocation for high-skill types
\((\underline q, s)\) is feasible for agent \(A=(s,b)\) iff \(\underline q / s \leq \min\{b, x\}\)
Fixing \(\underline q\), if we decrease \(x\):
- For larger budgets \(b\), upperbound on \(\underline q / s\) will be set sooner by decreasing \(x \implies\) discriminates more against agents with larger budgets
Future directions
- What happens when we introduce a uniform subsidy (to budgets) to all agents?
- Extending the model from 2-skill \(n\)-budget to \(m\)-skill \(n\)-budget
- Extending the model from discrete type space to continuous type space
Thank you for listening!
Updates on Fair Mechanism Design
Dec 7, 2021
Motivation
For school admissions, we would like to design a more fair criteria than, say, just looking at the score and determining a threshold.
Review
- Agent \(i\) has skill \(s_i\), but principal only observes quality = skill \(\times\) effort
- Principal wants agents above a certain quality threshold \(\hat{q}\)
- Effort required for agent to reach said skill threshold \(\hat{q}\): \[e_i = \frac{\hat{q}}{s_i}\]
- Agent \(i\) is constrained by her budget on effort: \[e_i \in [0, b_i] \]
- Agent \(i\)'s utility \[u_i = x_i - e_i\]
- where \(x_i\) is the probability that she gets allocated
Review
- Agent \(i\) has
- skill \(s_i\)
- budget \(b_i\)
- effort \(e_i \in [0, b_i]\)
- Agent gets allocated with probability \(x_i\)
- Agent's utility \[u^A_i = x_i - e_i\]
- Principal observes agents' quality:
\(q_i = s_i \times e_i\)
- Principal wants agents above a certain skill threshold \(\hat{q}\)
- Principal's utility \[u^P =\sum_i (s_i - \hat{q})\]
What will the agent do?
- Suppose we offer agents a menu of options to choose from
- Each option is a (quality threshold, allocation probability) tuple, \((\hat{q}, x)\)
- Agent with skill \(s\) will consider option \((\hat{q}, x)\) if
- effort required to reach \(\hat{q}\) is not going to exceed her budget, and
- utility is non-negative when exerting effort (i.e. individual rationality)
- \(\hat{q}_j/s \leq b\), and
- \(u = x_j - \hat{q}_j/s \geq 0\), or equivalently, \(\hat{q}_j/s \leq x_j\)
- subscript \(_j\) because we can have multiple options
Option \((\hat{q}_j, x_j)\) is feasible for agent if \(\hat{q}_j/s \leq \min \{b, x_j\}\)
Putting this into a graph...
Option \((\hat{q}_j, x_j)\) is feasible for agent if \(\hat{q}_j/s \leq \min \{b, x_j\}\)
\(x\)
\(q\)
\(1\)
Putting this into a graph...
i.e. visualizing agent's effort
\(x\)
\(q\)
\(1\)
option \((\hat{q}_j, x_j)\) is feasible if \(\hat{q}_j/s \leq \min \{b, x_j\}\)
\(x\)
\(q\)
\(1\)
Consider a single agent with skill \(s\);
When \(b=1\):
\(s\)
\(k = 1/s\)
option \((\hat{q}_j, x_j)\) is feasible if \(\hat{q}_j/s \leq \min \{b, x_j\}\)
\(x\)
\(q\)
\(1\)
Consider a single agent with skill \(s\);
When \(b=1\):
\(\implies\) Question: which is a feasible option?
\(s\)
\(k = 1/s\)
\((\hat{q}_1, x_1)\)
\((\hat{q}_2, x_2)\)
option \((\hat{q}_j, x_j)\) is feasible if \(\hat{q}_j/s \leq \min \{b, x_j\}\)
\(x\)
\(q\)
\(1\)
Consider a single agent with skill \(s\);
When \(b=1\):
\(\implies\) Question: which is a feasible option?
\(s\)
\(k = 1/s\)
\((\hat{q}_1, x_1)\)
\(\hat{q}_2\)
\(x_2\)
\(x\)
\(q\)
\(1\)
Consider a single agent with skill \(s\);
When \(b=1\):
\(\implies\) Question: which is a feasible option?
\(s\)
\(k = 1/s\)
\((\hat{q}_1, x_1)\)
\(\hat{q}_2\)
\(x_2\)
\(\hat{q}_2 / s\)
option \((\hat{q}_j, x_j)\) is feasible if \(\hat{q}_j/s \leq \min \{b, x_j\}\)
Answer: \((\hat{q}_2, x_2)\) is not feasible
\(x\)
\(q\)
\(1\)
Consider a single agent with skill \(s\);
When \(b=1\):
\(\implies\) Question: which is a feasible option?
\(s\)
\(k = 1/s\)
\((\hat{q}_1, x_1)\)
\((\hat{q}_2, x_2)\)
option \((\hat{q}_j, x_j)\) is feasible if \(\hat{q}_j/s \leq \min \{b, x_j\}\)
\(x\)
\(q\)
\(1\)
Consider a single agent with skill \(s\);
When \(b=1\):
\(\implies\) Question: which is a feasible option?
\(s\)
\(k = 1/s\)
\(\hat{q}_1\)
\(x_1\)
\((\hat{q}_2, x_2)\)
option \((\hat{q}_j, x_j)\) is feasible if \(\hat{q}_j/s \leq \min \{b, x_j\}\)
\(x\)
\(q\)
\(1\)
Consider a single agent with skill \(s\);
When \(b=1\):
\(\implies\) Question: which is a feasible option?
\(s\)
\(k = 1/s\)
\(\hat{q}_1\)
\(x_1\)
\(\hat{q}_1/s\)
\((\hat{q}_2, x_2)\)
option \((\hat{q}_j, x_j)\) is feasible if \(\hat{q}_j/s \leq \min \{b, x_j\}\)
Answer: \((\hat{q}_1, x_1)\) is feasible
\(x\)
\(q\)
\(1\)
Consider a single agent with skill \(s\);
When \(b=1\):
\(\implies\) Question: What about utility?
\(s\)
\(k = 1/s\)
\(\hat{q}_1\)
\(x_1\)
\(\hat{q}_1/s\)
\((\hat{q}_2, x_2)\)
option \((\hat{q}_j, x_j)\) is feasible if \(\hat{q}_j/s \leq \min \{b, x_j\}\)
Recall: utility \(u = x_i - \hat{q_j}/s_i\)
utility
effort
\(x\)
\(q\)
\(1\)
Consider a single agent with skill \(s\);
When \(b=1\):
\(\implies\) Question: What about zero-utility curve?
\(s\)
\(k = 1/s\)
option \((\hat{q}_j, x_j)\) is feasible if \(\hat{q}_j/s \leq \min \{b, x_j\}\)
Recall: utility \(u = x_i - \hat{q_j}/s_i\)
zero-utility \(\implies x_i - \hat{q}_j/s_i = 0 \implies x_i = \hat{q}_j / s_i\)
zero-utility curve
\(x\)
\(q\)
\(1\)
Generalizing the feasible region
\(s\)
\(k = 1/s\)
When \(b=1\),

feasible region
\(x\)
\(q\)
\(1\)
Generalizing the feasible region
\(s\)
\(k = 1/s\)
When \(b<1\),

\(s\cdot b\)
feasible region
\(\implies\) budget is limiting
\(x\)
\(q\)
\(1\)
Generalizing the feasible region
\(s\)
\(k = 1/s\)
When \(b>1\),

\(s\cdot b\)
\(\implies\) budget is no longer limiting for agent
feasible region
\(x\)
\(q\)
\(1\)
\(s\)
\(k = 1/s\)
What about agents with different budgets?
Suppose we have agents with same skills but different budgets
\(s\cdot b_L\)
\(s\cdot b_H\)
\(b=1\)
\(x\)
\(q\)
\(1\)
\(s\)
\(k = 1/s\)
What about agents with different budgets?
Suppose we have agents with same skills but different budgets
\(s\cdot b_L\)
\(s\cdot b_H\)
\(b=1\)
feasible for \(b_H\) but not for \(b_L\)
\(x\)
\(q\)
\(1\)
\(s\)
\(k = 1/s\)
What about agents with different skills?
Suppose we have agents with low skills \(s_L\) and high skills \(s_H\) but they face the same budget
\(x\)
\(q\)
\(1\)
\(s_H\)
\(1/s_H\)
What about agents with different skills?
Suppose we have agents with low skills \(s_L\) and high skills \(s_H\) but they face the same budget
\(s_L\)
\(1/s_L\)
feasible for \(s_H\) but not for \(s_L\)
What is the optimal menu?
- Recall: principal's utility is \(\sum_i (s_i - \hat{q})\).
- Ideally, we want all agents with high skills.
- However, we can't tell high-skilled agents from low-skilled agents because all we observe is quality.
- Start with the simple case of two kinds of skill types and two kinds of budget types
- An agent can either be high-skilled \(s_H\) or low-skilled \(s_L\)
- An agent can either have a high budget \(b_H\) or a low budget \(b_L\)
- WLOG, suppose \(b_L < 1\)
What is the optimal menu?
- If \(s_L\cdot b_H \leq s_H\cdot b_L\):
- Posting a single threshold of \(\hat{q} = s_L\cdot b_H + \epsilon\) is optimal because the threshold allows us to filter out all agents with low skills
- The more interesting case -- \(s_L\cdot b_H > s_H\cdot b_L\):
- Just looking at quality won't help us differ high-skilled agents from low-skilled agents
Principal's utility is \(\sum_i (s_i - \hat{q})\).
Simple menu 1 ("keep all high-skilled")
- Post quality threshold \(\hat{q} = s_L\cdot b_L + \epsilon\)
- Assign with certainty for those who reached the threshold
\(x\)
\(q\)
\(1\)
\(s_H\cdot b_H\)
\(s_L\cdot b_H\)
\(s_H\cdot b_L\)
\(s_L\cdot b_L\)
Simple menu 1
- Post quality threshold \(\hat{q} = s_L\cdot b_L + \epsilon\)
- Assign with certainty for those who reached the threshold
\(x\)
\(q\)
\(1\)
\(s_H\cdot b_H\)
\(s_L\cdot b_H\)
\(s_H\cdot b_L\)
\(s_L\cdot b_L\)
low skill people who reached \(\hat{q}\)
(because they have high budgets)
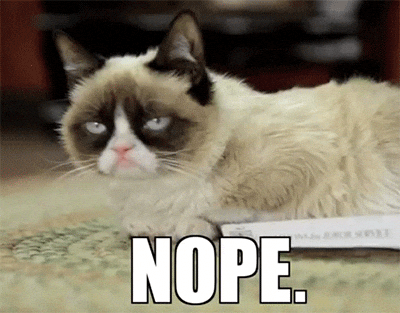
How can we improve?
Simple menu 2 ("no low skill")
\(x\)
\(q\)
\(1\)
\(s_H\cdot b_H\)
\(s_L\cdot b_H\)
\(s_H\cdot b_L\)
\(s_L\cdot b_L\)
Note: \(b_L < b_H < 1\)
\(s_L\)
\(s_H\)
feasible region for \(s_L\)
Simple menu 2 ("no low skill")
\(x\)
\(q\)
\(1\)
\(s_H\cdot b_H\)
\(s_L\cdot b_H\)
\(s_H\cdot b_L\)
\(s_L\cdot b_L\)
- Post menu options that are outside \(s_L\)'s feasible region, but inside \(s_H\)'s feasible region
\(s_L\)
\(s_H\)
Formally:
\((s_Lb_H+\epsilon, 1)\)
\((s_Hb_L, s_Hb_L/s_L - \epsilon)\)
\(x\)
\(q\)
\(1\)
\(s_H\cdot b_j\)
\(s_L\cdot b_i\)
\(s_L\)
\(s_H\)
Generalizing to 2-skill \(n\)-budgets
- low-skilled agents: \(s_Lb_k < s_Lb_i\) will pick (0, 0); \(s_Lb_k \geq s_Lb_i\) will pick purple point;
- high-skilled agents: \(s_Lb_k < s_Lb_j\) will pick dot on their vertical line; \(s_Lb_k \geq s_Hb_j\) will pick orange point
Next step
- Generalizing to \(n\) skills 2 budget
- Generalizing to \(n\) skills \(m\) budgets
- Continuous skills and budgets
\(x\)
\(q\)
\(1\)
\(s_H\cdot b_H\)
\(s_L\cdot b_H\)
\(s_H\cdot b_i\)
\(s_L\cdot b_i\)
\(s_L\)
\(s_H\)
Generalizing to 2-skill \(n\)-budgets
Same idea: keep quality threshold outside \(s_L\)'s feasible region
Menu with jumps
\(x\)
\(q\)
\(1\)
\(s_H\cdot b_H\)
\(s_L\cdot b_H\)
\(s_H\cdot b_L\)
\(s_L\cdot b_L\)
Text
Menu with jumps
\(x\)
\(q\)
\(1\)
\(s_H\cdot b_H\)
\(s_L\cdot b_H\)
\(s_H\cdot b_L\)
\(s_L\cdot b_L\)
Text
Simple menu 2
\(x\)
\(q\)
\(1\)
\(s_H\cdot b_H\)
\(s_L\cdot b_H\)
\(s_H\cdot b_L\)
\(s_L\cdot b_L\)
- Post quality threshold \(\hat{q} = s_H\cdot b_L - \epsilon\)
- Assign with certainty for those who reached the threshold
What is the optimal menu?
We want to hire no low skill agents, and as many high skill agents as possible
\(x\)
\(q\)
\(1\)
\(s_H\cdot b_H\)
\(s_L\cdot b_H\)
\(s_H\cdot b_L\)
\(s_L\cdot b_L\)
two skills, same limiting budget
What is the optimal menu?
We want to hire no low skill agents, and as many high skill agents as possible
\(x\)
\(q\)
\(1\)
\(s_H\cdot b_H\)
\(s_L\cdot b_H\)
\(s_H\cdot b_L\)
\(s_L\cdot b_L\)
expressing optimal menu in math: ...
What is the optimal menu?
Suppose we have two kinds of budget \(b_L, b_H\) and two kinds of skill \(s_L, s_H\).
\(x\)
\(q\)
\(1\)
\(s_H\cdot b_H\)
\(s_L\cdot b_H\)
\(s_H\cdot b_L\)
\(s_L\cdot b_L\)
What is the optimal menu?
Suppose we have two kinds of budget \(b_L, b_H\) and two kinds of skill \(s_L, s_H\).
\(x\)
\(q\)
\(1\)
\(s_H\cdot b_H\)
\(s_L\cdot b_H\)
\(s_H\cdot b_L\)
\(s_L\cdot b_L\)
More specifically,
- Principal's first-best is to hire everyone who is above a certain threshold
- Principal's utility is \[ u^P(x,e) = \sum_i x_i \cdot (s_i - t) \] where \(t \geq 0\) is some threshold
- For a utility maximizing principal, they will only pick agents with \(s_i \geq t\)
Public Budget
- Suppose we have a single public budget \(b\) for all agents; i.e. \(F^b\) contains one element
- all agents face the same budget \(b\) and we know it
- Suppose that allocation rule \(g\) has a deterministic quality threshold \(\tau\)
- We argue that there always exists a threshold \(\tau = t\) that achieves the first-best
- i.e. Principal awards agents with \(s_i \geq t\)
Proof.
- To achieve threshold \(\tau\), agent \(i\) will need to put in effort at least \(\tau / s_i\) - think of this as min effort required to achieve \(\tau\)
- Recall that agent \(i\)'s utility is \(\underbrace{g(q_i)}_{\in [0, 1]} - e_i\)
- \(\implies\) Agent \(i\)'s max possible utility is \(1 - \tau / s_i\) if she wants to reach quality threshold \(\tau\)
- To reach threshold \(t\), agent \(i\)'s max possible utility is \(1 - t/s_i\) and she will only put in effort if \(1 - t/s_i \geq 0\)
Proof cont'd.
- To reach threshold \(t\), agent \(i\)'s max possible utility is \(1 - t/s_i\) and she will only put in effort if \(1 - t/s_i \geq 0\)
- \(\implies s_i \geq t\) - i.e. only agents with skill \(s_i \geq t\) will put in effort
- Therefore, Principal's utility \(\sum_i x_i \cdot (s_i - t)\) is maximized because we're only summing up non-negative quantities
Next step
- Derive a concrete example with two populations where using a single threshold fails
4 cases
\(1 - t/s_i \geq 0\)
\(1 - t/s_i < 0\)
(max possible utility)
\(t/s_i \leq b\)
\(t/s_i > b\)
(min effort vs budget)
\(e_i = t/s_i\)
\(e_i = 0\)
\(e_i = b\)
\(e_i = 0\)
(case 1)
(case 2)
will put in effort
Formally,
- Agent \(i = 1, ... , n\)
- For each agent \(i\), she has a
- skill \(s_i \sim F^s\)
- budget \(b_i \sim F^b\)
- effort \(e_i \in [0, b_i] \)
- \(F^s ,F^b\) are known
- We observe each agent's quality: \(q_i = \underbrace{s_i}_{\text{skill}} \times \underbrace{e_i}_{\text{effort}}\)
Formally,
- \(x_i \in [0, 1]\) indicates the probability of agent \(i\) receiving the reward; normalize reward to 1
- Agent \(i\)'s utility: \(u_i^A (x_i, e_i) = x_i - e_i\)
- Allocation rule \(g: q_i \to [0, 1]\)
Agent's effort
- To achieve quality threshold \(\tau\), we only need effort \(\tau / s_i\)
\(\to\) When will an agent put in effort?
- Given \(\tau\), agent will put in effort
- \(e_i = 0\) if \(1 - \tau/s_i < 0\)
- Agent puts in no effort if maximal attainable utility at \(\tau\) is negative
- \(e_i = \min \{b, \tau/s_i\}\) otherwise
- \(e_i = 0\) if \(1 - \tau/s_i < 0\)
Deriving \(\tau\)
- Recall that the principal will award agents with skill \(s_i \geq t \to \) awarded agent at the boundary has skill \(s_i = t\)
- For an agent at the boundary, she either has a binding budget (\(e_i = \frac{\tau}{t} = b\)) or zero utility (\(1 - \frac{\tau}{t} = 0\)).
Case 1: \(e = \tau / t = b\)
- From \(\tau/t = b\), we have \(\tau = tb\)
- Suppose \( b > 1\).
- Utility is \( 1 - \tau / t = 1 - b < 0\) -- This cannot happen. Therefore, \(b \leq 1\).
- \(\to \tau / t \leq 1 \to \tau \leq t\)
- In general, utility \(= 1 - \tau / t \geq 0 \to t \geq \tau\)
- Therefore, \(\tau = t\)
Case 2: \(1 - \tau / t = 0\)
- Solving for the above equation, we have that \(\tau = t\)
\(t / s_i \in (b, \infty)\)
- In words: For agent \(i\), the minimum effort required to achieve quality threshold \(t\) is greater than the budget for effort
- Agent \(i\) with skill s.t. \(s_i \cdot b < t\) will not be selected by the principal \(\implies g(s_i \cdot b) = 0\)
- \(\implies\) Agent \(i\) will not exert effort; \(e = 0\)
- \(\implies\) Quality \(q_i = 0 < t\), Principal will not select such an agent
\(0\)
\(b\)
\(\frac{t}{s_i}\)
\(\frac{t}{s_i}\)
\(t / s_i \in (0, b]\)
- In words: For agent \(i\), the minimum effort required to achieve quality threshold \(t\) is between 0 and budget
- To maximize agent's utility, she will put in effort \(e_i = t/s_i \leq b\), and her utility is \(g(s_i \cdot e_i) - e_i = g(t) - t/s_i \)
- Recall that \(g(t) = x_i \in [0, 1]\), therefore agent \(i\)'s maximum possible utility is \(1 - t/s_i\)
- Agent will not put in effort if \(1 - t/s_i < 0\)
- \(\implies 1 < t/s_i \leq b\)
-
Agent will put in effort if \(1 - t/s_i \geq 0\)
- \(\implies b > 1 \geq t/s_i\)
\(0\)
\(b\)
\(\frac{t}{s_i}\)
\(\frac{t}{s_i}\)
(scratch paper)
* utility is non-negative: \(1 - \tau / t \geq 0\)
* effort \(\leq\) budget: \(\tau / t \leq b \)
When budget binds: \(\tau = tb \to 1 - b \geq 0 \to b \leq 1\)
\(q_i = s_i \times e_i\)
utility = \(g(q_i) - e_i = x_i - e_i\)
\(0\)
\(b\)
\(\frac{t}{s_i}\)
\(\frac{t}{s_i}\)
Public budget
- If \(b \leq 1\) (i.e. budget is binding):
- \(t\) is used when \( \tau / t \leq b \to \tau \leq tb \)
- Agent exerts effort and meets threshold \(\tau\) if her utility is positive, that is, \(1 - \tau / t \geq 0 \to t \geq \tau \)
- Since \( b \leq 1\), \( \tau \leq tb \) is binding, and suppose we set \(\tau = tb\)
- This ensures that agents with \( s \geq t\) will choose to exert effort \(b\), meet the threshold, and are given rewards
Public budget
- Moreover, for agents with skills \(s < t\), they will put in effort \(e \leq b\)
From a MD pov,
- The principal could have different objective functions to maximize
- For example, principal could maximize the sum of qualities
- similar to maximizing revenue in an all-pay auction
- Another example would be to maximize social welfare
Variant 2
- Principal's first-best is to hire the top \(k\) candidates
- \(s_i \geq 0\)
- \( C = k\) is now binding
- Allocation rule \(g\) maps observed qualities, reported budgets \(\hat{b}\), reported skills \(\hat{s}\) to allocation \(x\)
Variant 2
- Suppose allocation rule \(g\) ignores reports for now and only uses observed qualities
- Assume for now that the probability of a tie (in terms of quality?) is 0
- Agents care about their probability of being in the top \(k\)
- Denote the largest quality by \(q^{(1)}\) and the smallest quality by \(q^{(n)}\)
Variant 2
- For agent \(i\), her interim utility is \[\Pr_F[q_i \geq q^{(k)}] - e_i\]
Fair Mechanism Design
By Sheng Long
Fair Mechanism Design
- 40