Large deviations of the giant in spatial random graphs
Joost Jorritsma
joint with Júlia Komjáthy, Dieter Mitsche



Long-range phenomena in percolation, September '24
Supercritical bond percolation on \(\mathbb{Z}^d\)

[Gandolfi '88, Grimmett & Marstrand '90, Kesten & Zhang '90, Pisztora '96]
Surface-tension driven behavior
The largest component

Soft Poisson Boolean model
Edge set \(\mathcal{E}_\infty\)
- \(\{u\leftrightarrow v \}\Longleftrightarrow \{w_u^{1/d}+w_v^{1/d}\ge \|x_u-x_v\|\}\)
- Long-range parameter \(\alpha>1\),
- Percolation \(p\in(0,1]\)
Connection probability
$$\mathbb{P}\big(u\leftrightarrow v\mid \mathcal{V}_\infty\big)=\phantom{p\bigg(}\frac{ w_u^{1/d}+ w_v^{1/d}}{\|x_u-x_v\|}\wedge 1\phantom{\bigg)^{\alpha d}}$$
$$\mathbb{P}\big(u\leftrightarrow v\mid \mathcal{V}_\infty\big)=\phantom{p}\bigg(\frac{ w_u^{1/d}+ w_v^{1/d}}{\|x_u-x_v\|}\wedge 1\bigg)^{\alpha d}$$
Vertex set \(\mathcal{V}_\infty\)
- Locations: Poisson point process intensity \(\beta>0\)
- Power-law i.i.d. weights \(\tau>2\):
\(\mathbb{P}(w_v\ge w)=w^{-(\tau-1)}\), \(w\ge 1\)
$$\mathbb{P}\big(u\leftrightarrow v\mid \mathcal{V}_\infty\big)=p\bigg(\frac{w_u^{1/d}+ w_v^{1/d}}{\|x_u-x_v\|}\wedge 1\bigg)^{\alpha d}$$




$$(\mathrm{deg}(v) \mid w_v=w) \, = \, \mathrm{Poi}(c_{\alpha, d, \tau}p\beta \cdot w)$$
Soft Poisson Boolean model




Supercriticality assumption
- \(\theta=\theta_{d, \tau, \alpha, p}(\beta):=\mathbb{P}_{d, \tau, \alpha, p, \beta}^0(0\leftrightarrow \infty)\)
- \(\beta>\beta_c(\alpha, \tau, d, p)=\inf\{\beta: \theta(\beta)>0\}\)
Edge set \(\mathcal{E}_\infty\)
- \(\{u\leftrightarrow v \}\Longleftrightarrow \{w_u^{1/d}+w_v^{1/d}\ge \|x_u-x_v\|\}\)
- Long-range parameter \(\alpha>1\),
- Percolation \(p\in(0,1]\)
Vertex set \(\mathcal{V}_\infty\)
- Locations: Poisson point process intensity \(\beta>0\)
- Power-law i.i.d. weights \(\tau>2\):
\(\mathbb{P}(w_v\ge w)=w^{-(\tau-1)}\), \(w\ge 1\)
Soft Poisson Boolean model
Questions:
Fixed parameters:
- Point process on \(\mathbb{R}^d\) intensity \(\beta>0\)
- Power-law exponent \(\tau>2\)
- Long-range parameter \(\alpha>1\)
- Percolation \(p\in(0,1]\)
[Gracar, Lüchtrath, Mönch '21]
- When is \(\beta_c<\infty\)?
- \(d\ge 2\),
- Size of the largest connected component \(|\mathcal{C}_n^{(1)}|\)
- Law of large numbers (\(|\mathcal{C}_n^{(1)}|/n\to \theta\))?
- surface-tension behav. in \(\mathbb{P}(|\mathcal{C}_n^{(1)}|/n<\theta-\varepsilon)\)?
- exponential decay of \(\mathbb{P}(|\mathcal{C}_n^{(1)}|/n>\theta+\varepsilon)\)?
or \(d=1\), and \(\alpha\in(1, 2\vee\alpha_\tau)\)



Power-law weights
Constant weights
Lower tail*:
If \(\mathbb{E}[\# \text{edges of length } n^{1/d}]\to\infty\)
Main result: large deviations for the giant component
*log-corrections at phase transition
Upper tail:
If non-critical
*at continuity points of \(I(\theta+\varepsilon)\)
Biskup ['04]
Lower tail: a too small largest component

Goal 1:
+ local convergence (Giant is almost local)
[vdHofstad, vdHoorn, Maitra '21, vd Hofstad '21]
Goal 2: Prove the theorem
Bootstrapping: Goal 1 proved via method Goal 2
Edge set \(\mathcal{E}_\infty\)
- Long-range parameter \(\alpha>1\),
- Percolation \(p\in(0,1]\)
Connection probability
Vertex set \(\mathcal{V}_\infty\)
- Locations: Poisson point process intensity \(\beta>0\)
- Power-law i.i.d. weights \(\tau>2\):
\(\mathbb{P}(w_v\ge w)=w^{-(\tau-1)}\), \(w\ge 1\)
$$\mathbb{P}\big(u\leftrightarrow v\mid \mathcal{V}_\infty\big)=p\bigg(\frac{w_u^{1/d}+ w_v^{1/d}}{\|x_u-x_v\|}\wedge 1\bigg)^{\alpha d}$$




Goal 2 Prove


Power-law weights
Constant weights
Lower tail*:
If \(\mathbb{E}[\# \text{edges of length } n^{1/d}]\to\infty\)
Main result: large deviations for the giant component
*log-corrections at phase transition
Upper tail:
If non-critical
*at continuity points of \(I(\theta+\varepsilon)\)
Upper tail: polynomial decay when \(\tau<\infty\)

Related work:
- Sum of iid Par(\(\tau)\): principle of single jump.
- Large deviations # edges \(E_n\) (vdHvdHKMM '24)
\(\mathbb{P}(E_n>\mathbb{E}[E_n] +n\nu)=^\ast (1+o(1))c_\nu n^{-(\tau-2)\lceil\nu\rceil}\). - Inhomogeneous random graphs (J., Zwart '24+)
\(\mathbb{P}\big(|\mathcal{C}_n^{(1)}|/n>\rho\big)=^\ast (1+o(1))C_\rho n^{-(\tau-2)\lceil \widetilde h(\rho)\rceil}.\)
$$\mathbb{P}\big(u\leftrightarrow v\mid \mathcal{V}_\infty\big)=p\bigg(\frac{w_u^{1/d}+w_v^{1/d}}{\|x_u-x_v\|^d}\wedge 1\bigg)^{d\alpha}$$
Challenge: Capture dependency on percolation \(p\)



Geom. inhom. RG
Long-range percolation
Lower tail*:
If \(\mathbb{E}[\# \text{edges of length } n^{1/d}]\to\infty\)
Main result: large deviations for the giant component
*log-corrections at phase transition
Upper tail:
If non-critical, \(\rho\in(\theta, 1)\)
*at continuity points of \(I(\rho)\)
Thank you!

-
Cluster-size decay in supercritical long-range percolation;
With Júlia Komjáthy, Dieter Mitsche;
Electronic Journal of Probability (2024). -
Cluster-size decay in supercritical KSRGs;
With Júlia Komjáthy, Dieter Mitsche;
Preprint arXiv: 2303.00712. -
Large deviations of the giant in supercritical KSRGs;
With Júlia Komjáthy, Dieter Mitsche;
Preprint arXiv: 2404.02984.
Kernel-based spatial random graphs
Vertex set \(\mathcal{V}_\infty\)
- Spatial locations \(x_v\in\mathbb{R}^d\): PPP(1),
- i.i.d. weights \(w_v\ge 1\): Pareto(\(\tau\)),
Edge set \(\mathcal{E}_\infty\)
- Symmetric kernel \(\kappa(w_1, w_2)\),
- Edge-density \(\beta>0\),
- Long-range parameter \(\alpha>1\),
Connection probability
$$\mathbb{P}\big(u\leftrightarrow v\mid \mathcal{V}_\infty\big)=\bigg(\beta\frac{\kappa(w_u, w_v)}{\|x_u-x_v\|^d}\bigg)^\alpha\wedge 1$$
Vertex set \(\mathcal{V}_\infty\)
- Spatial locations \(x_v\in\mathbb{R}^d\): PPP(1), or \(\mathbb{Z}^d\),
- i.i.d. weights \(w_v\ge 1\): \(\mathbb{P}(w_v\ge w)=w^{-(\tau-1)}\),
Edge set \(\mathcal{E}_\infty\)
- Symmetric kernel \(\kappa(w_1, w_2)\),
- Edge-density \(\beta>0\),
- Long-range parameter \(\alpha>1\),



Geom. Inhom. RG

Hyperbolic RG

Geom. RG

Long-range perc.

Scale-free Gilbert RG

Age-dependent RCM
Theorem. When \(\tau<2\) or \(\alpha<1\):
- Infinite degrees
- Bounded diameter
[Deijfen, v.d. Hofstad, Hooghiemstra '13], [Gracar, Grauer, Lüchtrath, Mörters '18]
[Heydenreich, Hulshof, J. '17], [Hirsch '17], [v.d. Hofstad, v.d. Hoorn, Maitra '22],
[J., Komjáthy, Mitsche, '23], [Lüchtrath '22]
Theorem. When \(\tau>2\) and \(\alpha>1\):
$$\mathbb{P}(\mathrm{deg}(0)\ge k)\sim k^{-(\tau-1)}.$$
\(\tau\) small: many hubs
\(\alpha\) small: many long edges
The interpolating kernel
Connection probability
$${\color{grey}\mathbb{P}\big(u\leftrightarrow v\mid \mathcal{V}_\infty\big)=\bigg(\beta}\frac{\kappa(w_u, w_v)}{\color{grey}\|x_u-x_v\|^d}{\color{grey}\bigg)^\alpha\wedge 1}$$
A parameterized kernel: \(\sigma\ge 0\)
$$\kappa_{\sigma}(w_u, w_v):=\max\{w_u, w_v\}\min\{w_u, w_v\}^\sigma$$
- \(\tau: \mathbb{P}(w_v\ge w)=w^{-(\tau-1)}.\)
- \(\sigma\): assortativity
- \(\sigma\): interpolation

SFP/GIRG

Hyperbolic RG


Age-dep. RCM
Scale-free Gilbert



Long-range percolation
Random geom. graph
Nearest-neighbor percolation
Remarks.
- \(\zeta_\ast<0\): subcritical*
-
\(d\ge 2\):
- \((d-1)/d\)
- partial results: upper bounds
- Product kernel: 2nd term

No known results
- \(|{\color{blue}\text{largest}}|/n\)
- \({\color{red}2^{\mathrm{nd}}\text{-largest}}\)
Example 1:
Scale-free Gilbert RG in \(d=1\)
Power-law degrees: \(\tau>2\)
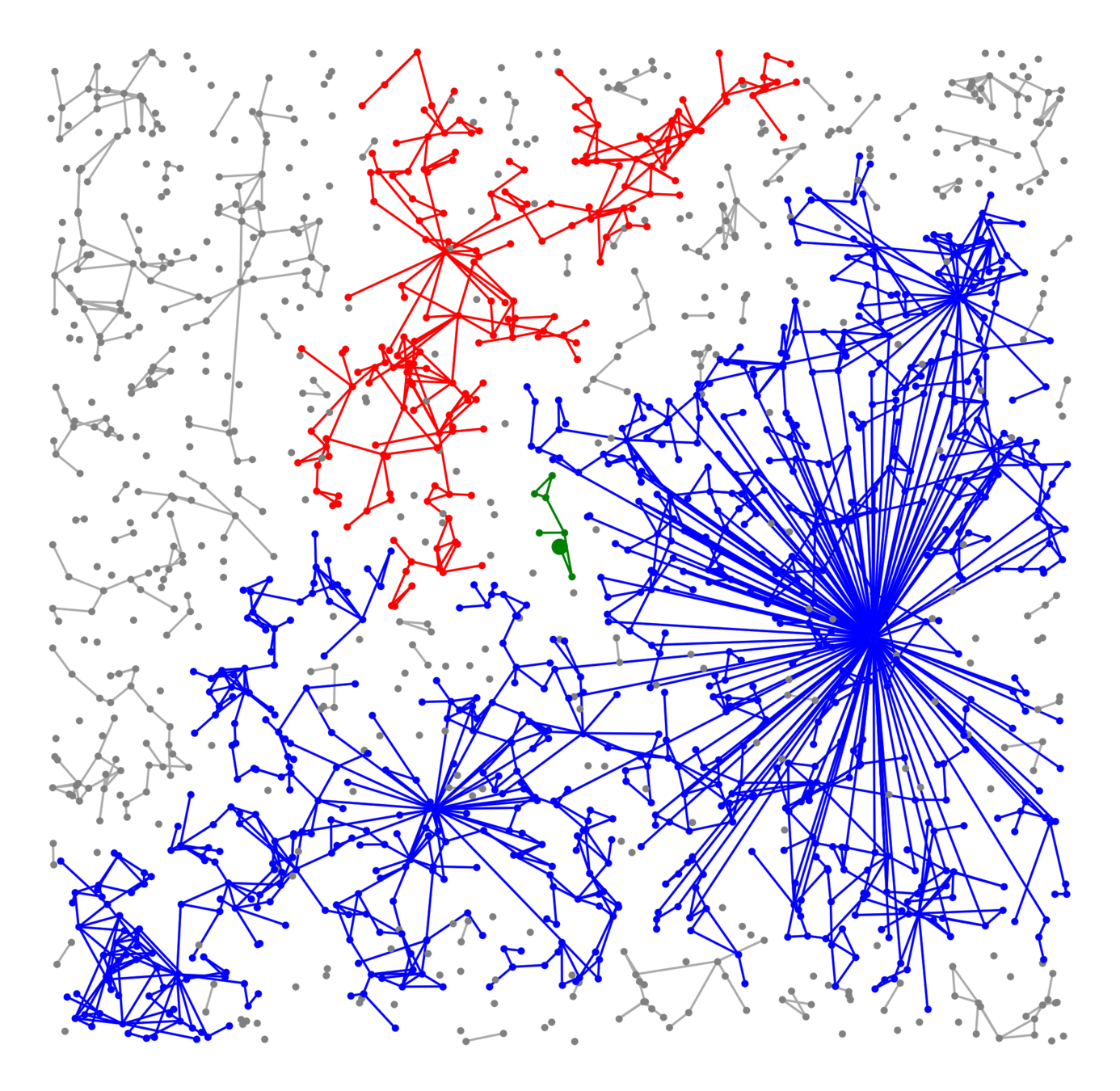
\(d\ge 2\)
* [Gracar, Lüchtrath, Mönch '22]
Theorem. (J., Komjáthy, Mitsche '23+)
Set
If \(\zeta_\ast>0\), then LLN for \(|{\color{blue}\text{largest}}|\), and
Long-range parameter: \(\alpha>1\)
\(\kappa_\mathrm{max}=w_u\vee w_v\)

\(\kappa_\mathrm{prod}=w_uw_v\)
Example 2:
Geom. Inhomog. RG in \(d=1\)
Lower bounds: cluster-size decay
Aim: Find minimal \(\zeta\) s.t.
Aim: Find \(\gamma\) s.t.
Lower bounds: cluster-size decay
Aim: Find minimal \(\zeta\) s.t.
Aim: Find \(\gamma\) s.t.
Lower bounds: large deviations
(FKG)




Lower bounds: \(|\mathcal{C}_n^{(2)}|\)
\(\delta\) small
Kernel-based spatial random graphs
Vertex set \(\mathcal{V}_\infty\)
-
Spatial locations, either
- Lattice \(\mathbb{Z}^d\)
- Poisson point process (unit intensity)
- Power-law i.i.d. weights \(w_v\ge 1\):
\(\mathbb{P}(w_v\ge w)=w^{-(\tau-1)}\),

Upper bound: \(|\mathcal{C}_n^{(2)}|\)












Soft Poisson Boolean model
Long-range percolation
Bond percolation on \(\mathbb{Z}^d\)
Lower tail:
- surface tension
- vertex boundary
Long-range percolation
Vertex set \(\mathcal{V}_\infty\)
-
Spatial locations, either
- Lattice \(\mathbb{Z}^d\)
- Poisson point process (unit intensity)

Edge set \(\mathcal{E}_\infty\)
- Long-range parameter \(\alpha>1\),
- Edge-density \(\beta>0\),
- Percolation \(p\in(0,1]\)
Connection probability
$$\mathbb{P}\big(u\leftrightarrow v\mid \mathcal{V}_\infty\big)=p\bigg(\frac{\beta}{\|x_u-x_v\|^d}\wedge 1\bigg)^\alpha$$
$$\mathbb{P}\big(u\leftrightarrow v\mid \mathcal{V}_\infty\big)=\phantom{p}\bigg(\frac{\beta}{\|x_u-x_v\|^d}\wedge 1\bigg)^\alpha$$
$$\mathbb{P}\big(u\leftrightarrow v\mid \mathcal{V}_\infty\big)=\phantom{p}\bigg(\frac{\beta}{\|x_u-x_v\|^d}\wedge 1\bigg)^\alpha$$
$$\mathbb{P}\big(u\leftrightarrow v\mid \mathcal{V}_\infty\big)=\phantom{p}\bigg(\frac{\beta}{\|x_u-x_v\|^d}\phantom{\wedge 1}\bigg)^\alpha$$
$$\mathbb{P}\big(u\leftrightarrow v\mid \mathcal{V}_\infty\big)=\phantom{p\bigg(}\frac{1}{\|x_u-x_v\|^d}\phantom{\bigg)^\alpha\wedge 1}$$
$$\mathbb{P}\big(u\leftrightarrow v\mid \mathcal{V}_\infty\big)=\phantom{p}\bigg(\frac{1}{\|x_u-x_v\|^d}\phantom{\wedge 1}\bigg)^\alpha$$



Soft Poisson Boolean model


Edge set \(\mathcal{E}_\infty\)
- Long-range parameter \(\alpha>1\),
- Percolation \(p\in(0,1]\)
Connection probability
$$\mathbb{P}\big(u\leftrightarrow v\mid \mathcal{V}_\infty\big)=p\bigg(\frac{ \phantom{w_u^{1/d}+ w_v^{1/d}}}{\|x_u-x_v\|}\wedge 1\bigg)^{\alpha d}$$
Vertex set \(\mathcal{V}_\infty\)
- Spatial locations: Poisson point process intensity \(\beta>0\)
- Power-law i.i.d. weights \(w_v\ge 1\):
\(\mathbb{P}(w_v\ge w)=w^{-(\tau-1)}\),
$$\mathbb{P}\big(u\leftrightarrow v\mid \mathcal{V}_\infty\big)=p\bigg(\frac{w_u^{1/d}+ w_v^{1/d}}{\|x_u-x_v\|}\wedge 1\bigg)^{\alpha d}$$
$$\mathbb{P}\big(u\leftrightarrow v\mid \mathcal{V}_\infty\big)=p\bigg(\frac{ {\color{darkred}w_u^{1/d}+w_v^{1/d}}}{\|x_u-x_v\|}\wedge 1\bigg)^{\alpha d}$$
Kernel-based spatial random graphs
Edge set \(\mathcal{E}_\infty\)
- Long-range parameter \(\alpha>1\),
- Edge-density \(\beta>0\),
- Percolation \(p\in(0,1]\)


Connection probability
Vertex set \(\mathcal{V}_\infty\)
-
Spatial locations, either
- Lattice \(\mathbb{Z}^d\)
- Poisson point process (unit intensity)
- Power-law i.i.d. weights \(w_v\ge 1\):
\(\mathbb{P}(w_v\ge w)=w^{-(\tau-1)}\),
$$\mathbb{P}\big(u\leftrightarrow v\mid \mathcal{V}_\infty\big)=p\bigg(\frac{\beta\cdot (w_u\cdot w_v)}{\|x_u-x_v\|^d}\wedge 1\bigg)^\alpha$$


Geom. inhom. RG
Long-range percolation
Lower tail*:
If \(\mathbb{E}[\# \text{edges of length } n^{1/d}]\to\infty\)
Remainder: Lower tail LRP - Upper tail GIRG
*log-corrections at phase transition
Upper tail:
If non-critical, \(\rho\in(\theta, 1)\)
*at continuity points of \(I(\rho)\)
Upper bounds
Challenge: Delocalized components
# possibilities for \(|{\color{red}2^{\mathrm{nd}}\text{-largest}}|\ge k\)

Components in supercritical graphs
-
Largest component \({\color{blue}\mathcal{C}_n^{(1)}}\):
- Linear in box size
- Law of large numbers
- Lower tail large deviations
- Upper tail large deviations
Questions




Components in supercritical graphs
-
Largest component \({\color{blue}\mathcal{C}_n^{(1)}}\):
- Linear in box size
- Law of large numbers
- Lower tail large deviations
- Upper tail large deviations
Questions




Previous results
[Alexander & Chayes & Chayes '90], [Grimmett & Marstrand, '90], [Kesten & Zhang '90], ..., [Deuschel, Pisztora, '96], [Biskup '04], [Penrose '05], [Sly & Crawford'12], [Kiwi & Mitsche '17], [Lichev, Lodewijks, Mitsche, Schapira '22], [Bläsius, Friedrich, Ruff, Zeiff, '23]



Conjecture: \(\exists \zeta\in \big[\tfrac{d-1}{d},1\big)\):


Lower bounds
Upper bounds

Upper bounds

What about other value \(\zeta_\ast\)?
Description of weight distribution in large components
Prevent "small-to-large" merging
Components in supercritical graphs
- Largest component \({\color{blue}\mathcal{C}_n^{(1)}}\):
- Linear in box size
- Law of large numbers
- Lower tail large deviations
- Upper tail large deviations
Answered questions (\(d=1\))




(Second-)largest component in supercritical spatial random graphs
- Cluster-size decay
- Second-largest component
Open problems:
-
Largest component:
- Linear in box size
- Law of large numbers
- Large deviations
Answered questions (\(d=1\))
- Phase transition boundaries.
- Partial results \(d\ge 2\)
- Extension from PPP to grid
- Central limit theorem






- \(\zeta\in\big[(d-1)/d, 1\big)\)
Lower bounds
Upper bounds




Small
Large
Lower bounds
Upper bounds

Lower bounds
Upper bounds
Lower bounds
Upper bounds

Remarks.
- \(\zeta_\ast<0\): subcritical*
-
\(d\ge 2\):
- \((d-1)/d\)
- partial results: upper bounds
- Product kernel: 2nd term
Power-law degrees: \(\tau>2\)
\(d\ge 2\)
* [Gracar, Lüchtrath, Mönch '22]
Theorem. (J., Komjáthy, Mitsche '23+)
Set
If \(\zeta_\ast>0\), then LLN for \(|{\color{blue}\text{largest}}|\), and
Long-range parameter: \(\alpha>1\)

Example 2:
Geom. Inhomog. RG in \(d=1\)






Hyperbolic random graph
Scale-free percolation
Long-range percolation
Scale-free Gilbert RG
Random geom. graph
Nearest-neighbor percolation

Largest component in spatial inhomogeneous random graphs
By joostjor
Largest component in spatial inhomogeneous random graphs
- 85